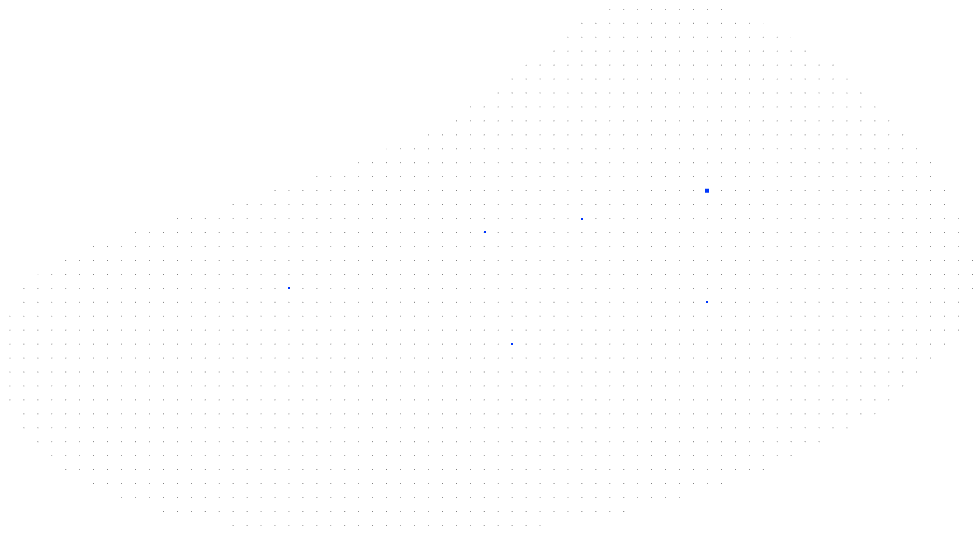
FastPoints2Mesh
Data-Driven Inference of Mesh-based Representations for Deformable Objects from Unstructured Point Clouds

Abstract
Combining robotic manipulation with computer vision holds great promise for automating daily tasks of ever-increasing complexity. However, versatile manipulation of complex or deformable objects is still beyond current robotic capabilities, primarily because fast and robust shape estimation for soft materials remains a challenging and largely open problem. We propose to tackle this challenge with a physics-informed mesh representation, building on recent advances in machine learning to achieve real-time point cloud to mesh reconstruction. Recent methods make it efficient and effective to learn from point cloud representation and show robustness to input perturbation and corruption. We aim to reconstruct in real-time the mesh of a deforming object by bringing suitable physical properties such as deformability, inertia, and density conservation into the loss function of the point cloud to mesh reconstruction neural network. We will procure a large-scale simulated dataset and enhance it with real-world data for training neural networks. The simulated dataset will be based on datasets of CAD object models (ShapeNet, PartNet, etc.) that are available online. The resulting pre-trained network is expected to greatly improve both the surface reconstruction accuracy and the frame rate of real-time mesh reconstruction. Such a quick and accurate meshing solution will be an important foundation for computer vision and robotics. The tracking of complex objects in unstructured environments is crucial for dexterous robotic manipulation and real-world interactions, which will strongly impact many application domains, including medicine, logistics, food processing, and agriculture.
People
Collaborators
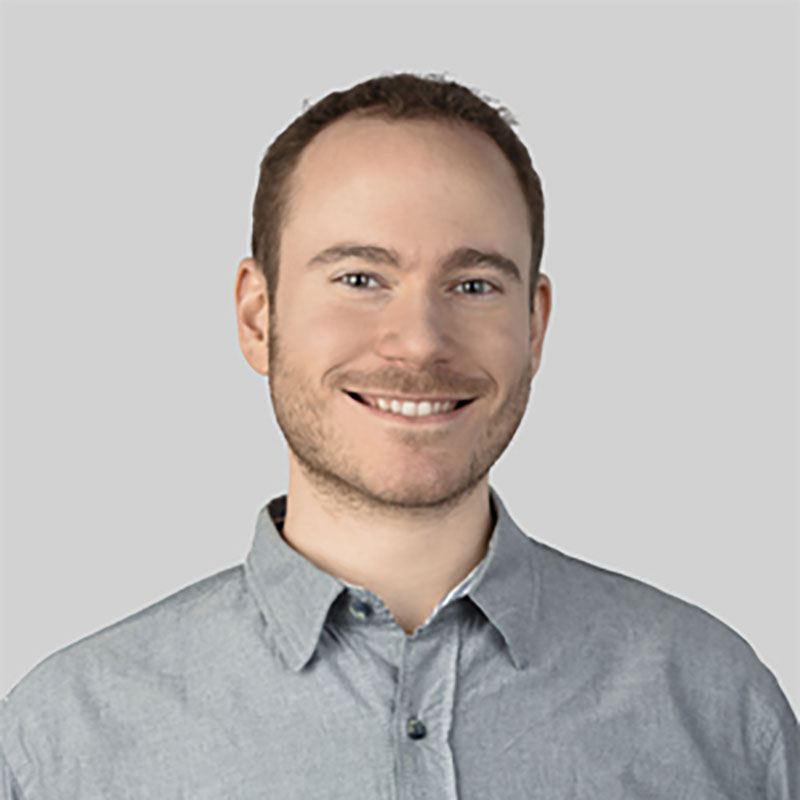
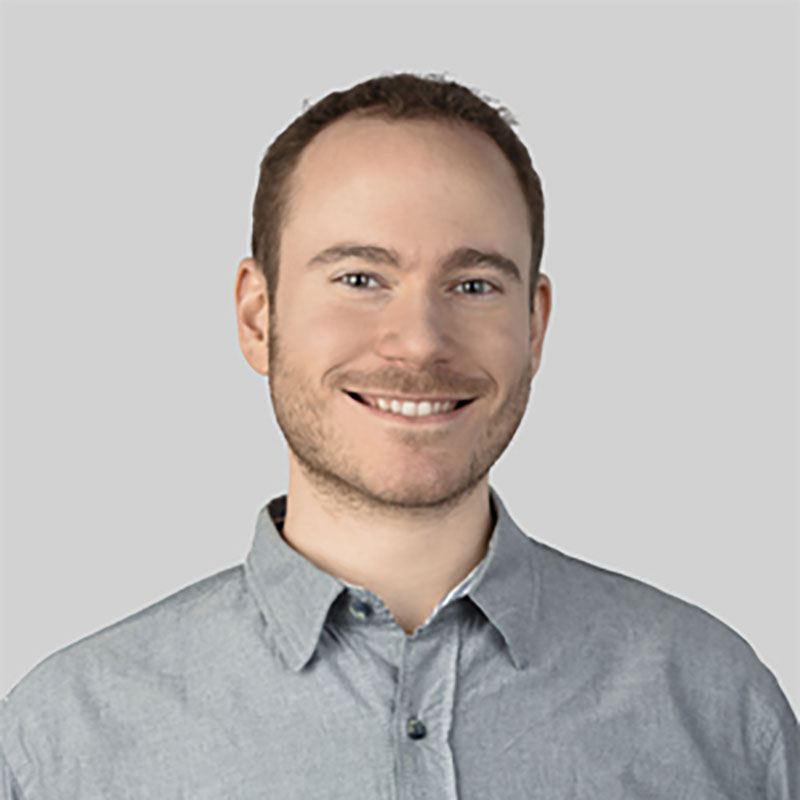
Firat completed his undergraduate studies in Electronics Engineering at Sabanci University. He later received his MSc. in Electrical and Electronics Engineering from EPFL. He conducted his doctoral studies on medical image segmentation in Computer Vision Lab at ETH Zurich. In between, he visited INRIA (Sophia Antipolis, France) and ABB Corporate Research Center (Baden, Switzerland). His research interests revolve around computer vision and machine learning, with a focus on the medical domain. He has been with SDSC since 2019.
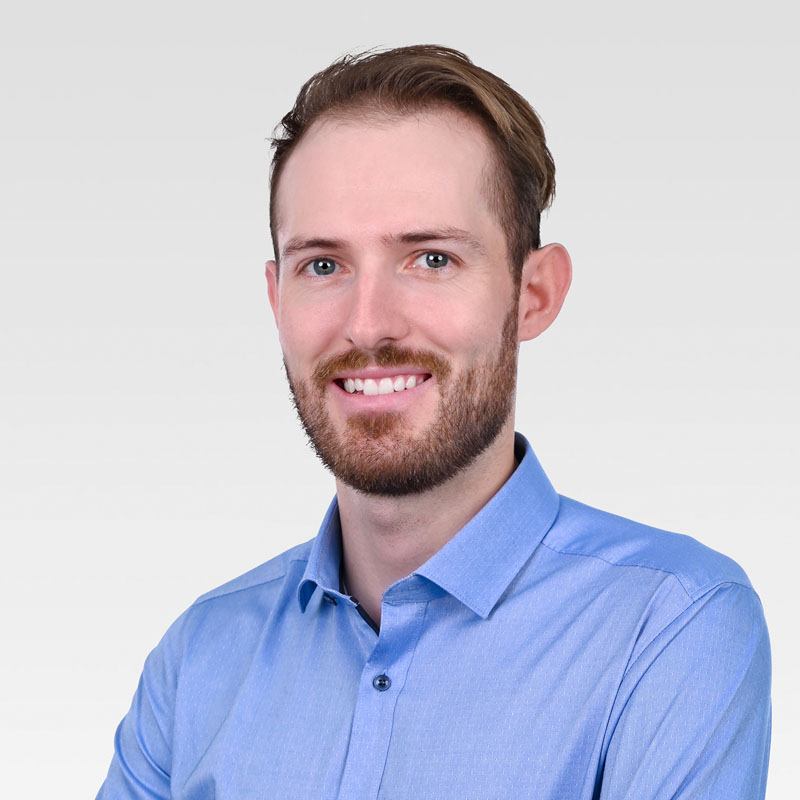
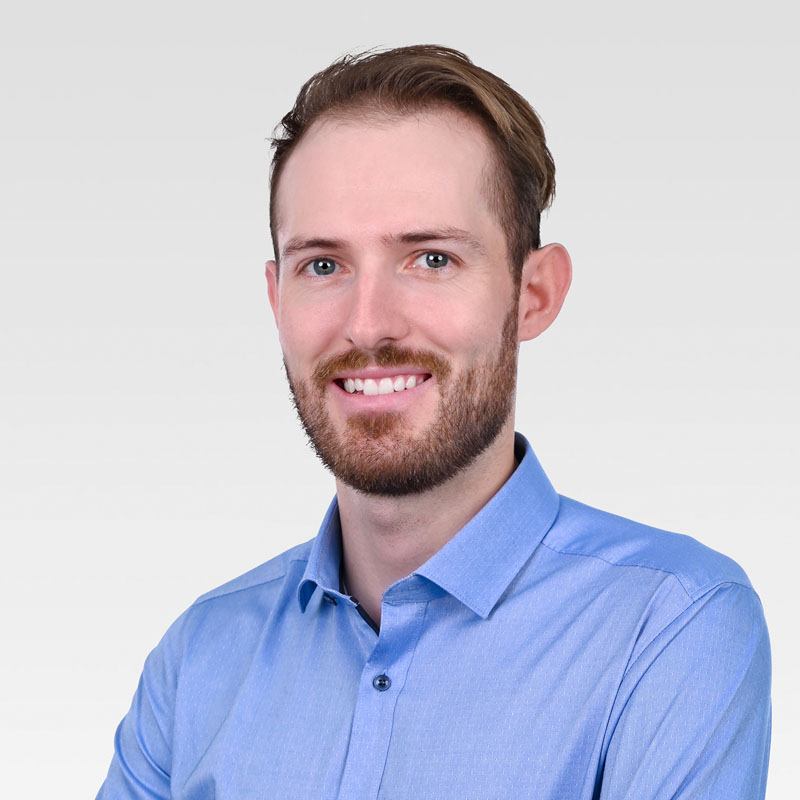
Before joining the SDSC as a Data Scientist in April 2023, Alessandro obtained his master’s degree in Computer Science with a focus on Machine Learning from ETH Zurich. In his master’s thesis, he worked on a joint project by the SDSC and the SLF Davos in which he automated the process of avalanche danger forecasting in the Swiss Alps using state-of-the-art machine learning techniques. In his free time, Alessandro loves alpine sports like ski-touring, climbing, and mountaineering.


Luis is originally from Spain, where he completed his bachelor's studies in Electrical engineering, and the Ms.C. on signal theory and communications, both at the University of Seville. During his Ph.D. he started focusing on machine learning methods, more specifically message passing techniques for channel coding, and Bayesian methods for channel equalization. He carried it out between the University of Seville and the University Carlos III in Madrid, also spending some time at the EPFL, Switzerland, and Bell Labs, USA, where he worked on advanced techniques for optical channel coding. When he completed his Ph.D. in 2013, he moved to the Luxembourg Center on Systems Biomedicine, where he switched his interest to neuroscience, neuroimaging, life sciences, etc., and the application of machine learning techniques to these fields. During his 4 and a half years there as a Postdoc, he worked on many different problems as a data scientist, encompassing topics such as microscopy image analysis, neuroimaging, single-cell gene expression analysis, etc. He joined the SDSC in April 2018. As Lead Data Scientist, Luis coordinates projects in various domains. Several projects focus on the application of natural language processing and knowledge graphs to the study of different phenomena in social and political sciences. In the domains of architecture and engineering, Luis is responsible for projects centered on the application of novel generative methods to parametric modeling. Finally, Luis also coordinates different projects in robotics, ranging from collaborative robotic construction to deformable object manipulation.


Mathieu Salzmann is the Deputy Chief Data Scientist of the Swiss Data Science Center and a Senior Scientist and Lecturer at EPFL. He received his PhD from EPFL in 2009 and, between 2009 and 2015, has held post-doctoral and researcher positions at UC Berkeley, TTI-Chicago, and NICTA and the Australian National University. From May 2020 to February 2024, he was also a part-time Senior GNC Engineer at ClearSpace. Mathieu Salzmann has broad interests in machine learning and deep learning, with a particular focus on computer vision. He has published over 100 articles at top-tier peer-reviewed machine learning and computer vision venues and is a strong believer in collaborative work.
description
Motivation
Recent advancements in computer vision could allow for robotic manipulation of complex objects (see Fig. 1 for some robotic manipulators). Along this line, inferring functional and physical properties of previously unseen objects from visual data play a key role to assess effective strategies for manipulation.
Proposed Approach / Solution
The project explores fast point cloud to mesh reconstruction of both soft robot arms (manipulators) and objects of interest (objects in the scene). A parallel effort is for generating a large-scale dataset with complex objects being manipulated. This dataset includes two types of data. One is the real-world collected data with both daily objects such as plush toys shown in Figure 2, and 3D printed deformable objects for easy reproduction [1]. The other is the simulated data based on large-scale 3D model datasets (ShapeNet, PartNet, etc.) that are publicly available online. This would allow for optimizing data-driven models that can infer functional and/or physical properties based on vision alone. We are also exploring approaches for simulating capture systems in an effort to bridge the gap between simulated and real-world data.
Impact
Fast mesh reconstruction is an important foundation for tracking and interacting with complex objects in unstructured scenes. Its impact would surpass the robotics field alone, with applications in medicine, logistics, food processing and agriculture. The generated dataset can promote future novel work from the community and allow for benchmarking findings.


Presentation
Gallery
Annexe
Additional resources
Bibliography
- Obrist, Jan, et al. "PokeFlex: A Real-World Dataset of Volumetric Deformable Objects for Robotics." arXiv preprint arXiv:2410.07688 (2025).
Publications
Related Pages
More projects
MAGNIFY
News
Latest news


First National Calls: 50 selected projects to start in 2025
First National Calls: 50 selected projects to start in 2025
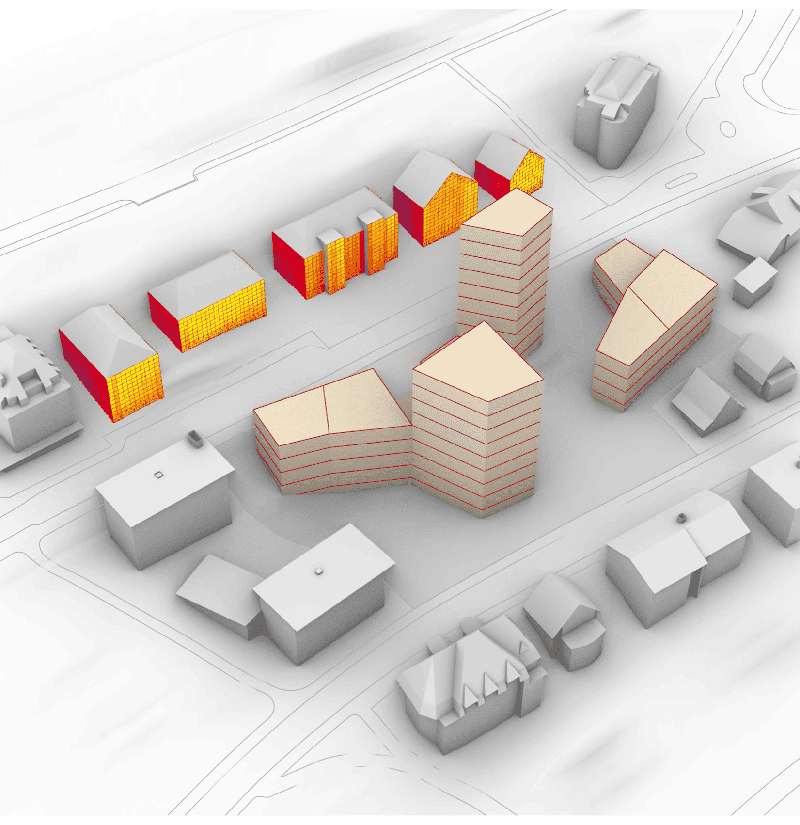
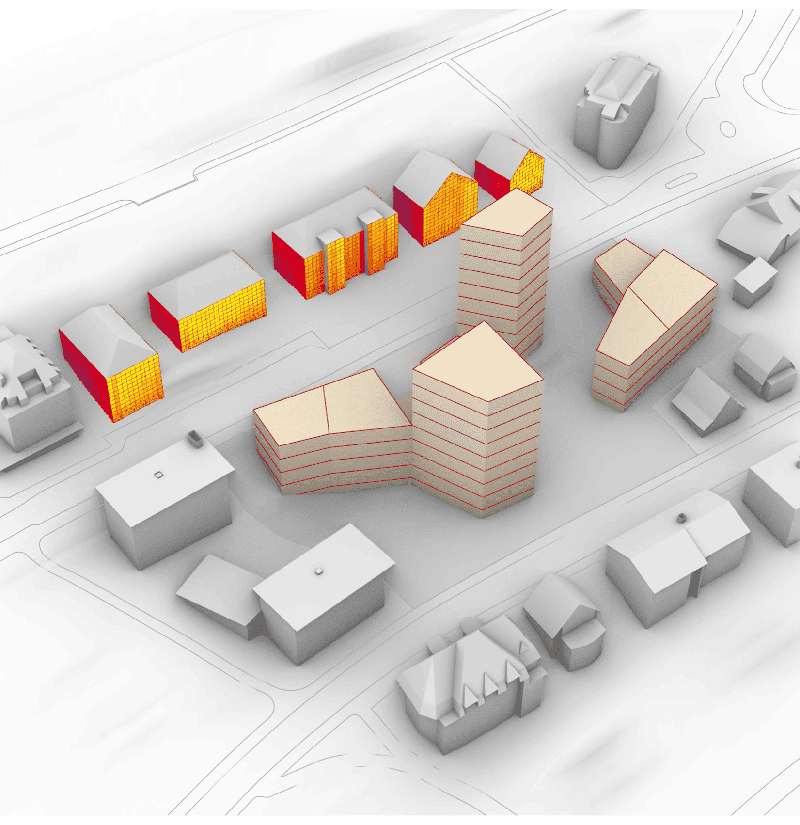
AIXD | Generative AI toolbox for architects and engineers
AIXD | Generative AI toolbox for architects and engineers


Smartair | An active learning algorithm for real-time acquisition and regression of flow field data
Smartair | An active learning algorithm for real-time acquisition and regression of flow field data
Contact us
Let’s talk Data Science
Do you need our services or expertise?
Contact us for your next Data Science project!