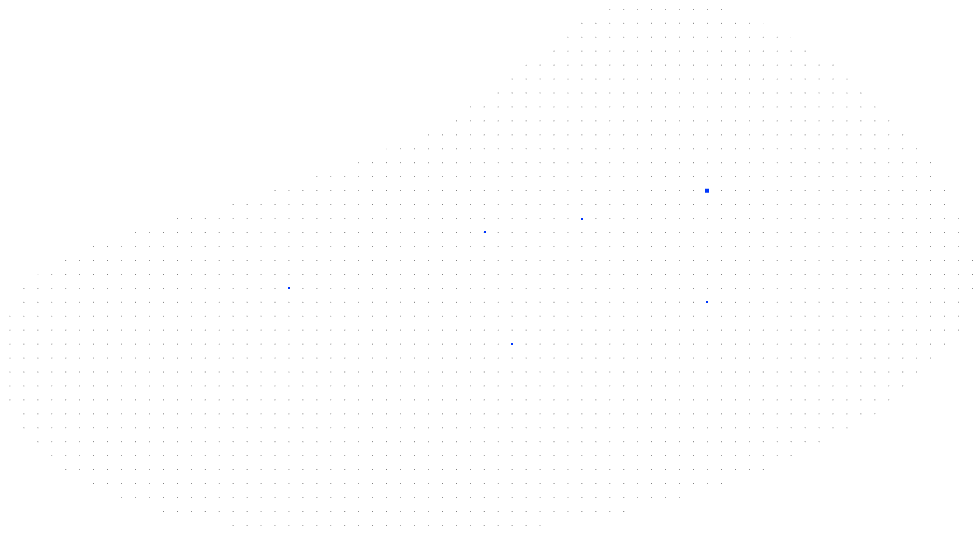
SPI-PAMIR

Abstract
Among the most iconic mountain ranges of the Third Pole are the Pamir Mountains, a keystone connecting the Central Asian (e.g. Tien Shan) and Himalayan mountain chains (e.g. Karakoram, Kunlun and Hindu Kush). The Pamir Mountains in Tajikistan supply water to an arid region stretching to the Aral Sea, and buffer seasonal water shortage with snow, glacier and permafrost thaw. The Pamirs’ cryosphere is thus of key importance, particularly given the ongoing challenges with water management, agriculture and energy production. Still, the response of the Pamir cryosphere and its ecosystems to climate change remains poorly understood, and the anomalous state of its glaciers exhibiting both health and decay, as well as the extent of thawing permafrost contributing additional water availability, remains an unsolved puzzle.
Permafrost is ground that remains continuously at or below 0°C for at least two consecutive years. In the collaboration between the PAMIR project and the SDSC, we will model permafrost probability for the Pamir region. The aim is to develop a machine learning surrogate of a computationally costly ground thermal model that can be applied to create maps of permafrost presence for the entire Pamir region.
People
Collaborators
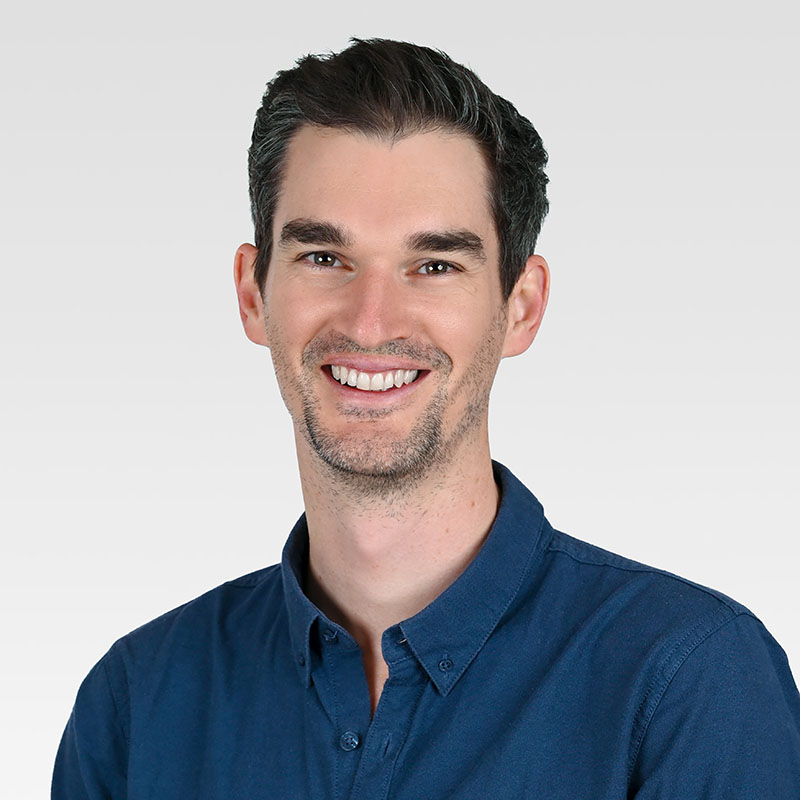
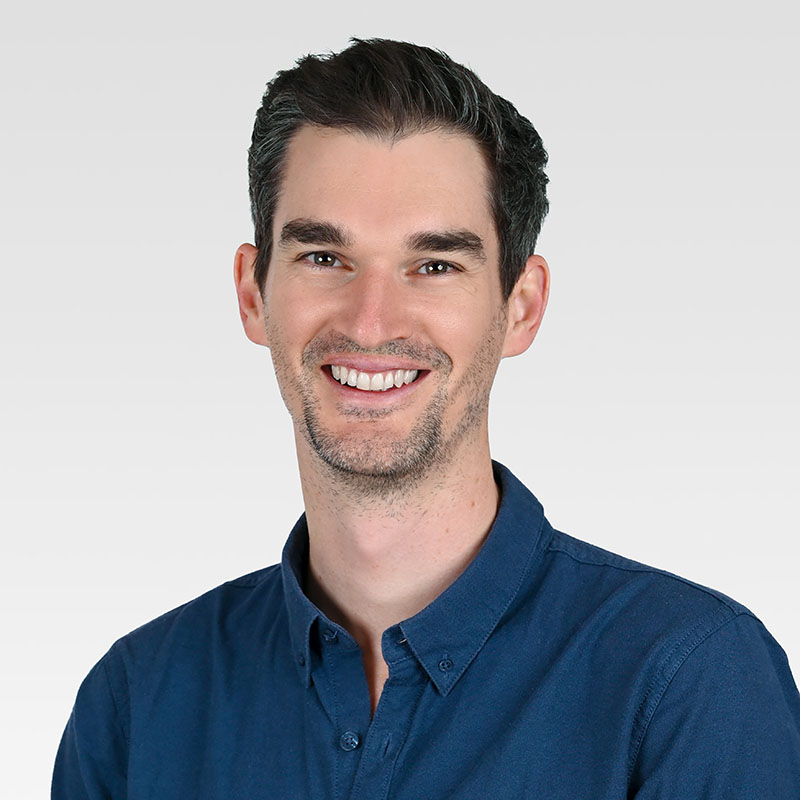
Luke completed his PhD in oceanography at the University of Cape Town in 2017.
After a short stint at the Council for Scientific and Industrial Research in Cape Town, he joined the Environmental Physics group at ETH Zürich as a postdoc in 2019. Here, his research focussed on ocean biogeochemistry. Specifically, he used machine learning approaches to estimate surface ocean pH and surface ocean carbon fluxes from satellite and ship-based observations.
Luke has also been involved developing scientific data processing tools for several autonomous observation platforms.
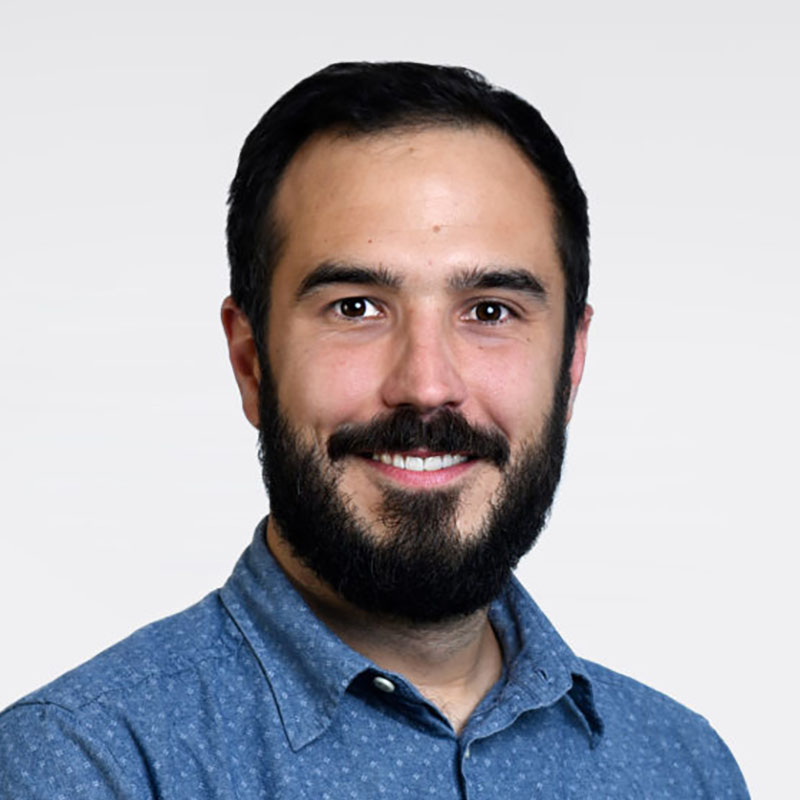
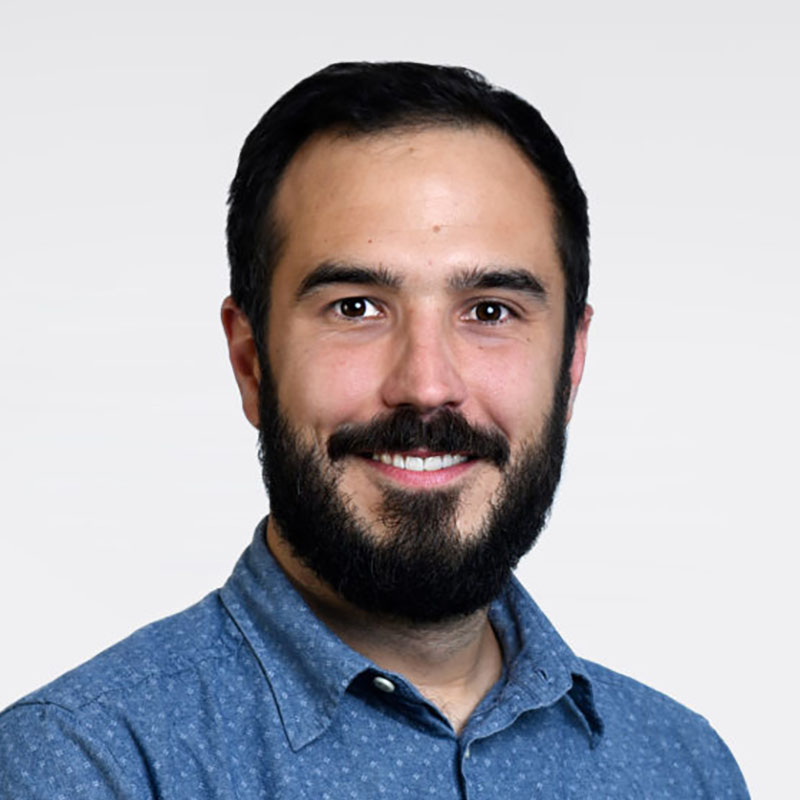
Michele received a Ph.D. in Environmental Sciences from the University of Lausanne (Switzerland) in 2013. He was then a visiting postdoc in the CALVIN group, Institute of Perception, Action and Behaviour of the School of Informatics at the University of Edinburgh, Scotland (2014-2016). He then joined the Multimodal Remote Sensing and the Geocomputation groups at the Geography department of the University of Zurich, Switzerland (2016-2017). His main research activities were at the interface of computer vision, machine and deep learning for the extraction of information from aerial photos, satellite optical images and geospatial data in general.
description
Motivation
Permafrost modelling is a challenging task that integrates decades of hourly climatic conditions. The current state of the art approach, a land surface model with elements of radiative transfer, is computationally costly at large scales. Various techniques have been developed to reduce the computational cost of the approach to make it feasible to run on a large compute cluster (Fiddes and Gruber, 2012). Machine learning offers a way to further reduce this computational cost.
Proposed Approach / Solution
The SDSC will aid the PAMIR project by creating baseline maps of permafrost and state variables for the Pamir mountain range (Figure 1) with a simple implementation of the CryoGrid model (Westermann et al., 2022). Smaller regions will be mapped with a pseudo-spatial implementation of CryoGrid (Fiddes et al., 2015) using local data to determine valid ranges for parameters (Mathys et al., 2024). These outputs will serve as training data for a machine learning surrogate. The surrogate will then be used to predict permafrost at high resolution (~ 100 m) for the entire region.
Impact
Permafrost could play an important part of the water cycle in the Pamir region, which is the reservoir for the arid downstream regions (Figure 2). Being able to map the presence and changing state of permafrost (increasing or decreasing) will help to understand the vulnerability of this region to climate change.

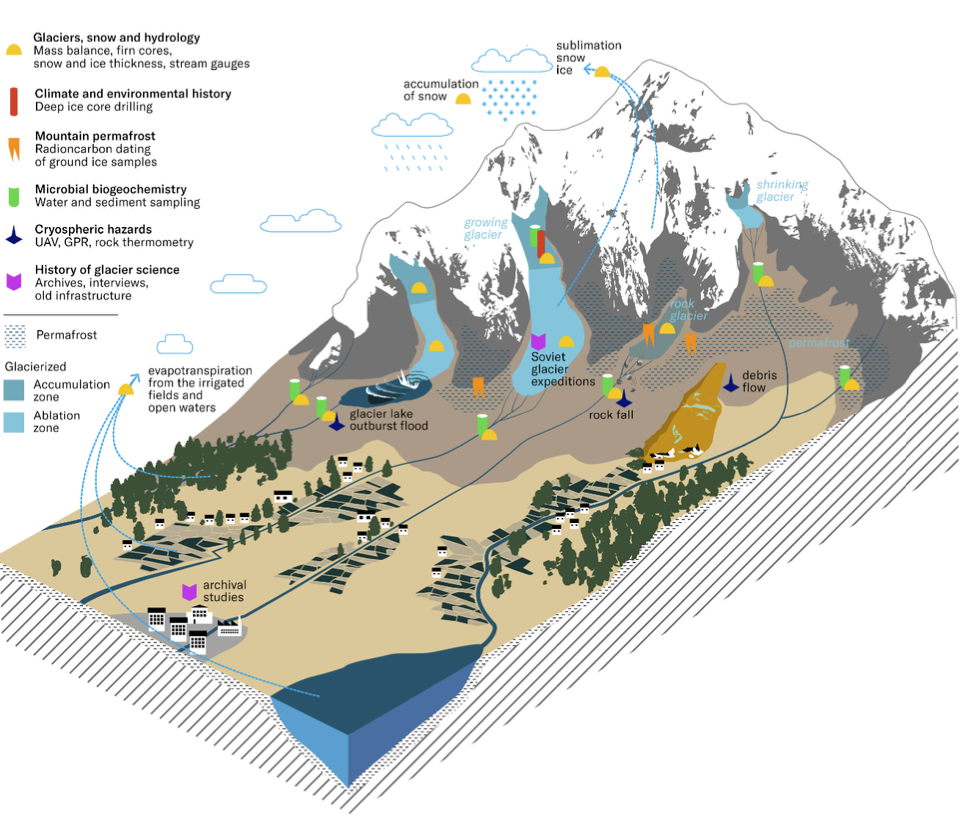
Presentation
Gallery
Annexe
Cover image source: Jason Klimatsas
Additional resources
Bibliography
- Westermann, S., Ingeman-Nielsen, T., Scheer, J., Aalstad, K., Aga, J., Chaudhary, N., Etzelmüller, B., Filhol, S., Kääb, A., Renette, C., Schmidt, L. S., Schuler, T. V., Zweigel, R. B., Martin, L., Morard, S., Ben-Asher, M., Angelopoulos, M., Boike, J., Groenke, B., … Langer, M. (2023). The CryoGrid community model (version 1.0) – a multi-physics toolbox for climate-driven simulations in the terrestrial cryosphere. Geoscientific Model Development, 16(9), 2607–2647. https://doi.org/10.5194/gmd-16-2607-2023
- Mathys, T., Azimshoev, M., Bektursunov, Z., Hauck, C., Hilbich, C., Duishonakunov, M., Kayumov, A., Kassatkin, N., Kapitsa, V., Martin, L. C. P., Mollaret, C., Navruzshoev, H., Pohl, E., Saks, T., Silmonov, I., Musaev, T., Usubaliev, R., & Hoelzle, M. (2024). Quantifying permafrost ground ice contents in the tien shan and pamir (central asia): A petrophysical joint inversion approach using the geometric mean model. EGUsphere, 2024, 1–52. https://doi.org/10.5194/egusphere-2024-2795
- Fiddes, J., & Gruber, S. (2012). TopoSUB: A tool for efficient large area numerical modelling in complex topography at sub-grid scales. Geoscientific Model Development, 5(5), 1245–1257. https://doi.org/10.5194/gmd-5-1245-2012
- Fiddes, J., Endrizzi, S., & Gruber, S. (2015). Large-area land surface simulations in heterogeneous terrain driven by global data sets: Application to mountain permafrost. The Cryosphere, 9(1), 411–426. https://doi.org/10.5194/tc-9-411-2015
Publications
Related Pages
More projects
MAGNIFY
News
Latest news
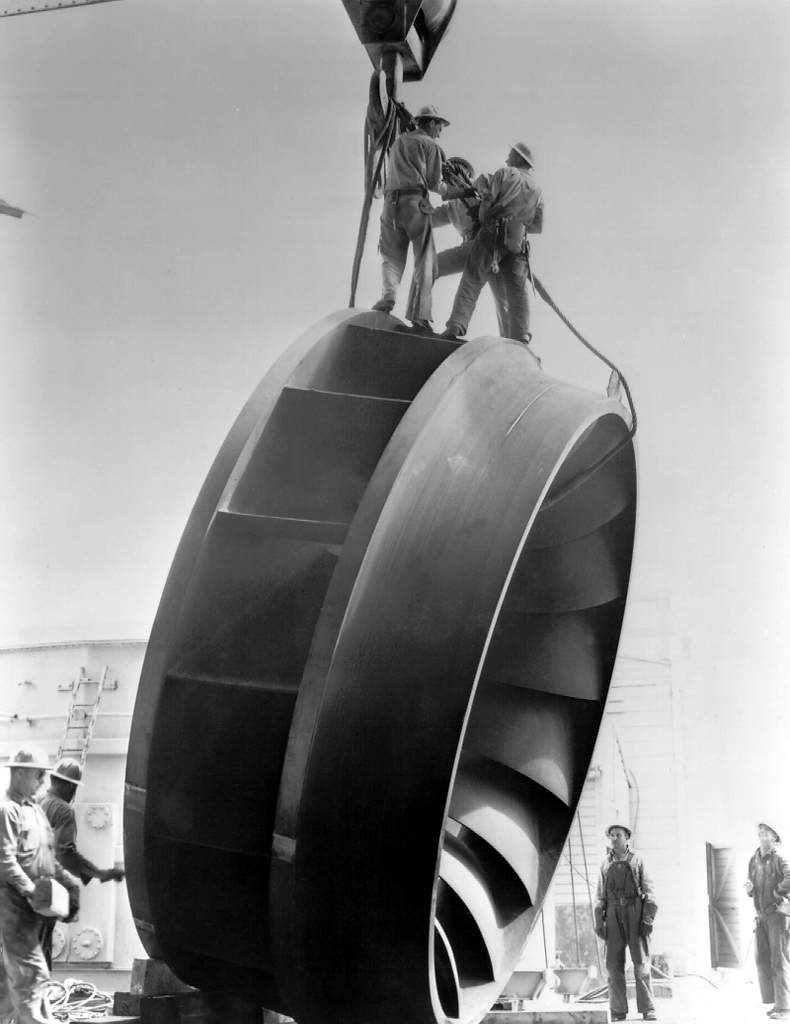
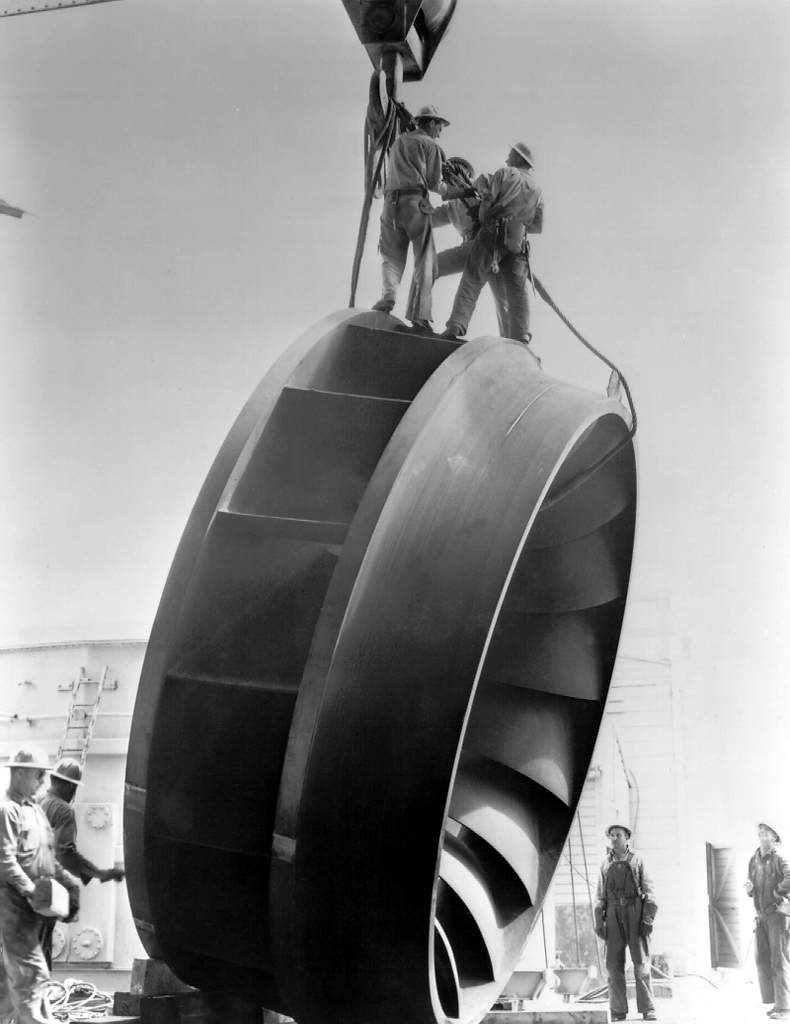
PAIRED-HYDRO | Increasing the Lifespan of Hydropower Turbines with Machine Learning
PAIRED-HYDRO | Increasing the Lifespan of Hydropower Turbines with Machine Learning


First National Calls: 50 selected projects to start in 2025
First National Calls: 50 selected projects to start in 2025
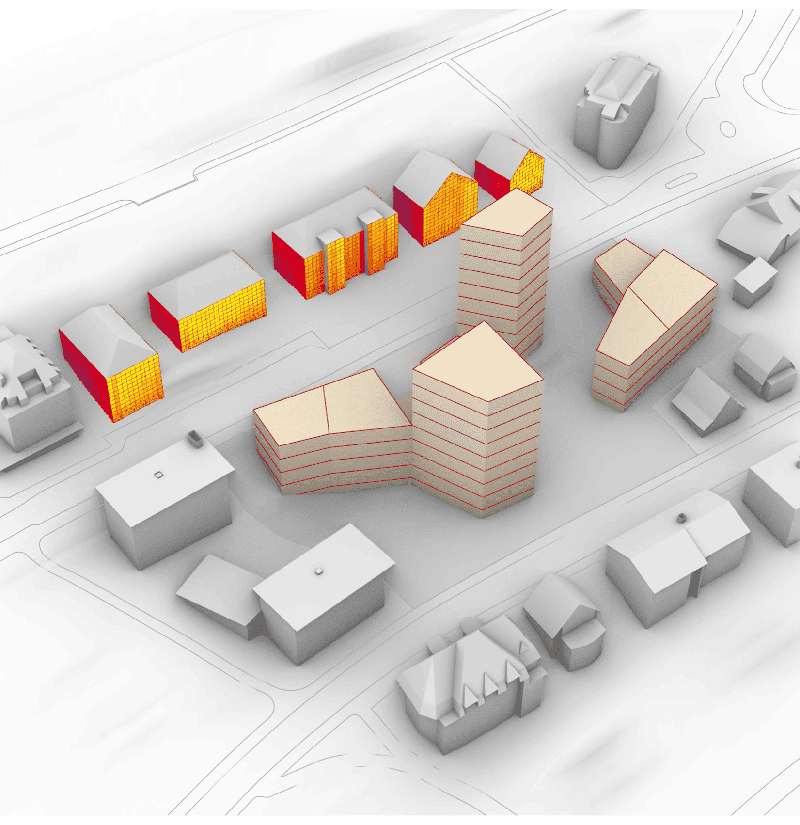
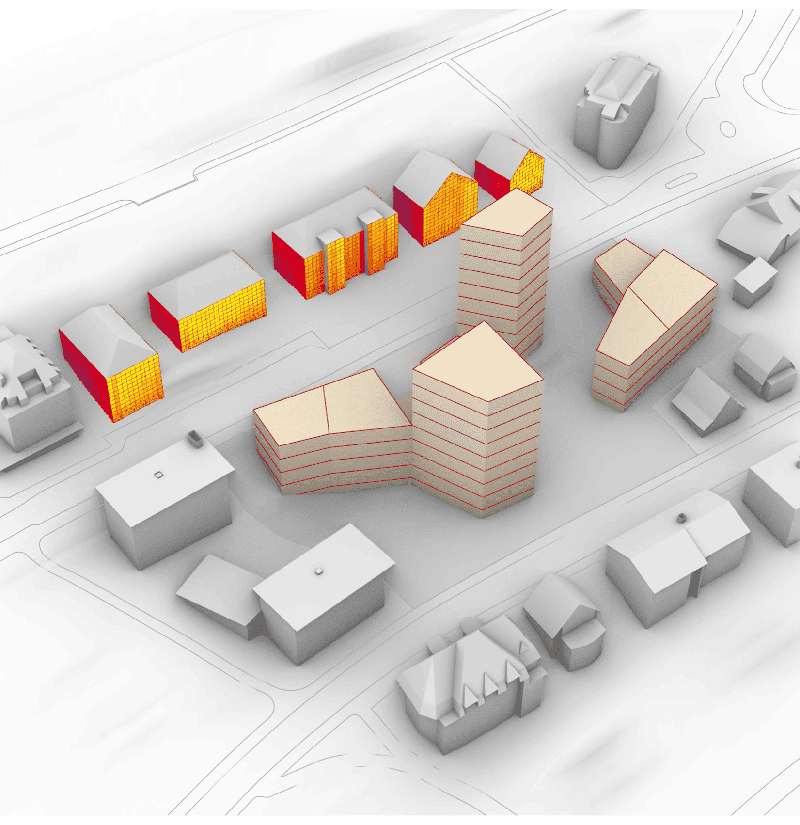
AIXD | Generative AI toolbox for architects and engineers
AIXD | Generative AI toolbox for architects and engineers
Contact us
Let’s talk Data Science
Do you need our services or expertise?
Contact us for your next Data Science project!