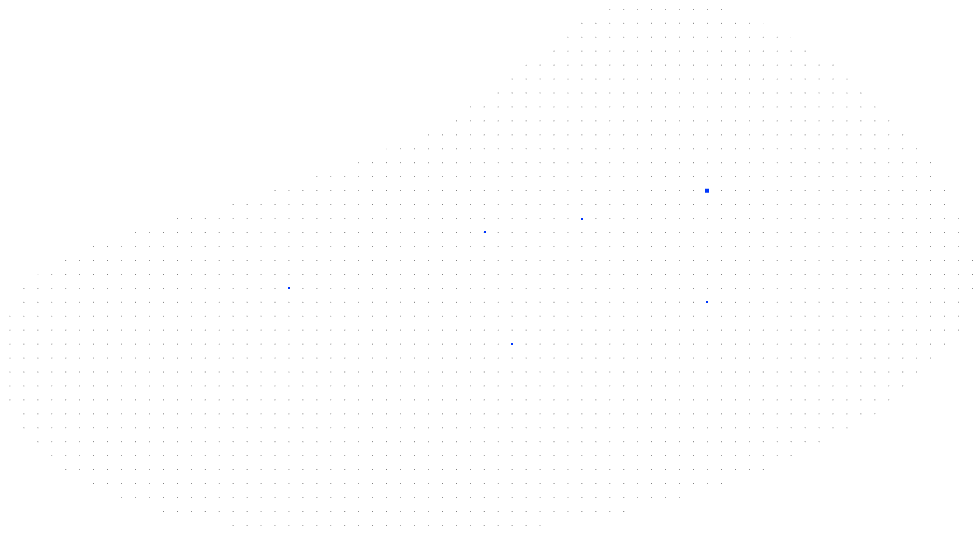
TREMA
Transforming real estate management with AI
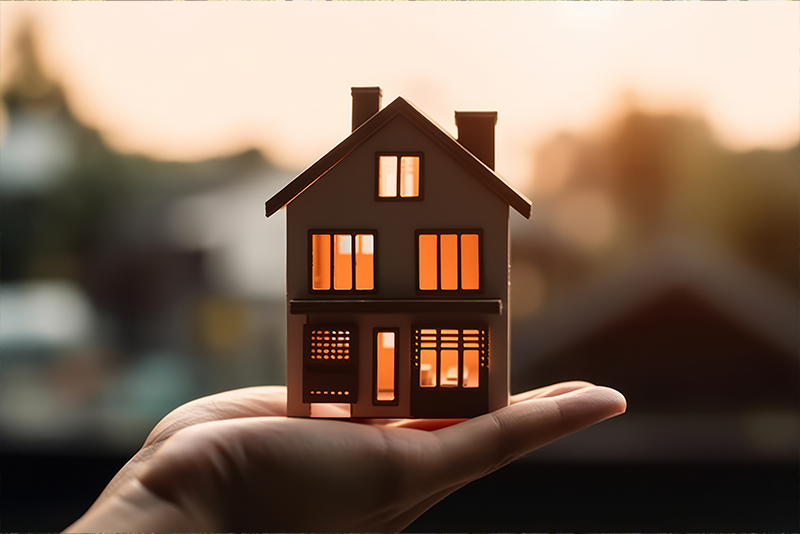
Abstract
The collaboration with our industry partner, Xelidoni, specialized in damage assessment for home rentals, aims to automatically detect property damage from images or videos captured with handheld cameras.
Currently, inspections in the home rental industry involve detailed documentation and expert estimations, often taking several hours per property and costing hundreds of Swiss francs per inspection. Our goal is to develop data-driven methodologies to significantly reduce inspection times, lower costs for property managers, and enhance accuracy in the damage appraisal process.
In this InnoCheque project, the SDSC focused on identifying effective data acquisition strategies, highlighting the shortcomings of public benchmarks, analyzing the current dataset provided by Xelidoni, assessing the limitations of state-of-the-art damage detection methods, discussing their feasibility in the home rental context, and exploring future research directions.
People
Collaborators


Isinsu joined the SDSC in May 2022. She obtained a Bachelor’s degree in Computer Science from Middle East Technical University, Turkey, and later received a Master’s degree in Computer Science from EPFL. She conducted her doctoral studies in the Computer Vision Lab at EPFL with a focus on human body pose estimation and motion prediction using deep learning-based methods. Her research interests include self- and semi-supervised learning for image-based analysis of the human body and motion, with applications ranging from athletic training to healthcare.


Thibaut holds a B.Sc in Computer Science from HEIG-VD. Before joining the SDSC, he worked in startups where he developped a diverse skill set combining cloud infrastructure, database and application development. Thibaut is very enthusiatic about new technologies and best coding practices and he is looking forward to supporting the team and its projects.


Alessandro joined the SDSC in March 2019 as a data scientist focused on industry collaborations. His mission is to support corporates in leveraging the power of their data by adopting analytical approaches and data-centric solutions. His background is in biomedical engineering, with a PhD in neuroscience from the University of Tübingen. Before joining the center, he worked as a postdoc at the Max Planck Institute for Biological Cybernetics, at the EPFL Laboratory of Cognitive Neuroscience in Geneva, and as data scientist for a private ecommerce company.


Mathieu Salzmann is the Deputy Chief Data Scientist of the Swiss Data Science Center and a Senior Scientist and Lecturer at EPFL. He received his PhD from EPFL in 2009 and, between 2009 and 2015, has held post-doctoral and researcher positions at UC Berkeley, TTI-Chicago, and NICTA and the Australian National University. From May 2020 to February 2024, he was also a part-time Senior GNC Engineer at ClearSpace. Mathieu Salzmann has broad interests in machine learning and deep learning, with a particular focus on computer vision. He has published over 100 articles at top-tier peer-reviewed machine learning and computer vision venues and is a strong believer in collaborative work.
description
Motivation
In the home rental industry, current damage assessment practices rely heavily on human inspectors, involving manual documentation and expert judgment. This process is time-consuming—often taking several hours per property—and incurs significant costs, especially when conducted at scale. To address these inefficiencies, our goal is to develop an AI-based damage assessment system that leverages visual recognition and anomaly detection techniques to automate inspections, reduce appraisal times from hours to minutes, and lower costs for property managers without compromising accuracy.
Proposed Approach / Solution
We explored the feasibility of leveraging AI to automatically detect damages incurred during home rentals by performing the following tasks:
- Review of state-of-the-art damage detection methods and relevant public datasets: We explored three main damage detection approaches, including supervised defect classification, unsupervised anomaly detection, and language-guided anomaly detection.
- Analysis of Xelidoni’s dataset and preliminary experiments using AnomalyCLIP: We propose building a dedicated dataset comprising approximately 5,000 normal and 500–750 anomalous images, covering a balanced range of high-, medium-, and low-priority indoor damages, annotated with anomaly labels and some bounding boxes. To ensure robustness and compatibility with public benchmarks, the dataset will include diverse scenes under different lighting conditions, varying damage severities, multiple viewing angles, and high-resolution images.
In addition, we tested AnomalyCLIP on normal and defective images of furnished flats, achieving strong zero-shot detection but encountering minor false positives on reflective surfaces. Since the model was trained on industrial datasets, fine-tuning it on a dedicated home damage dataset could improve performance. Future work could explore pretraining strategies and refining CLIP-based methods for better differentiation of defect types.
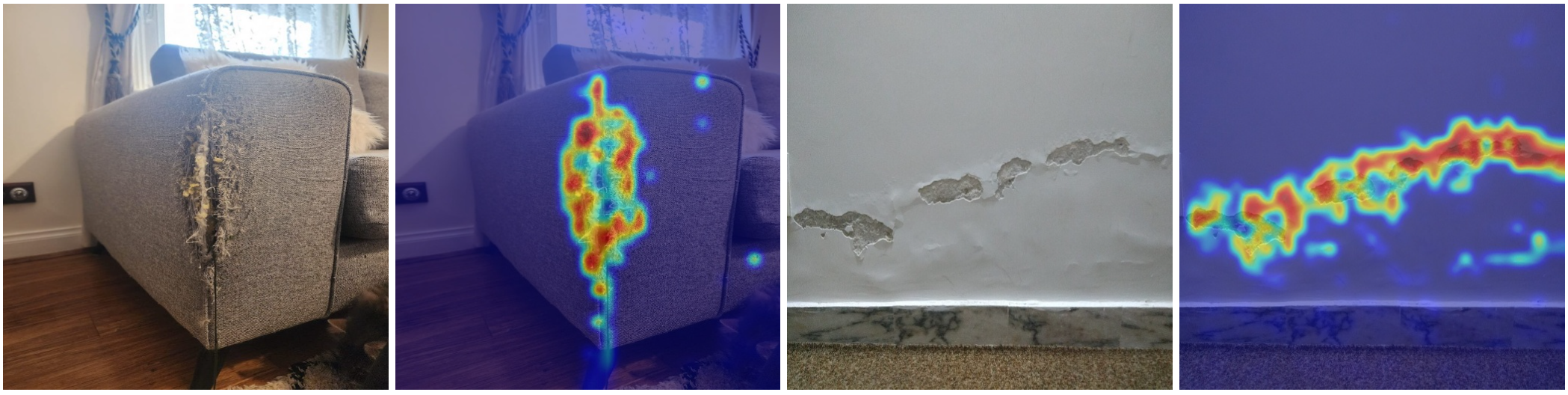
Impact
The AI-based damage assessment has the potential to transform the home rental industry by automating a traditionally time-consuming and costly inspection process. By leveraging visual recognition and anomaly detection techniques, the project aims to reduce inspection times from hours to minutes while lowering associated costs for property managers. This approach not only improves operational efficiency but also enhances the consistency and accuracy of damage appraisals across rental properties.
Presentation
Gallery
Annexe
Cover image source: Adobe Stock
Additional resources
Bibliography
- Lis, K., Nakka, K., Fua, P., & Salzmann, M. Detecting the Unexpected via Image Resynthesis. In ICCV, 2019.
- Radford, A., Kim, J. W., Hallacy, C., Ramesh, A., Goh, G., Agarwal, S., Sastry, G., Askell, A., Mishkin, P., Clark, J., Krueger, G., & Sutskever, I. Learning transferable visual models from natural language supervision. In International Conference on Machine Learning, 2021.
- Pantoja-Rosero, B. G., Oner, D., Kozinski, M., Achanta, R., Fua, P., Perez-Cruz, F., & Beyer, K. TOPO-Loss for continuity-preserving crack detection using deep learning. Construction and Building Materials, 344, 2022.
- Zhou, Q., Pang, G., Tian, Y., He, S., & Chen, J. AnomalyCLIP: Object-agnostic Prompt Learning for Zero-shot Anomaly Detection. In ICLR, 2024.
- Zhu, J., & Pang, G. Toward Generalist Anomaly Detection via In-context Residual Learning with Few-shot Sample Prompts. In CVPR, 2024.
Publications
Related Pages
More projects
MAGNIFY
News
Latest news
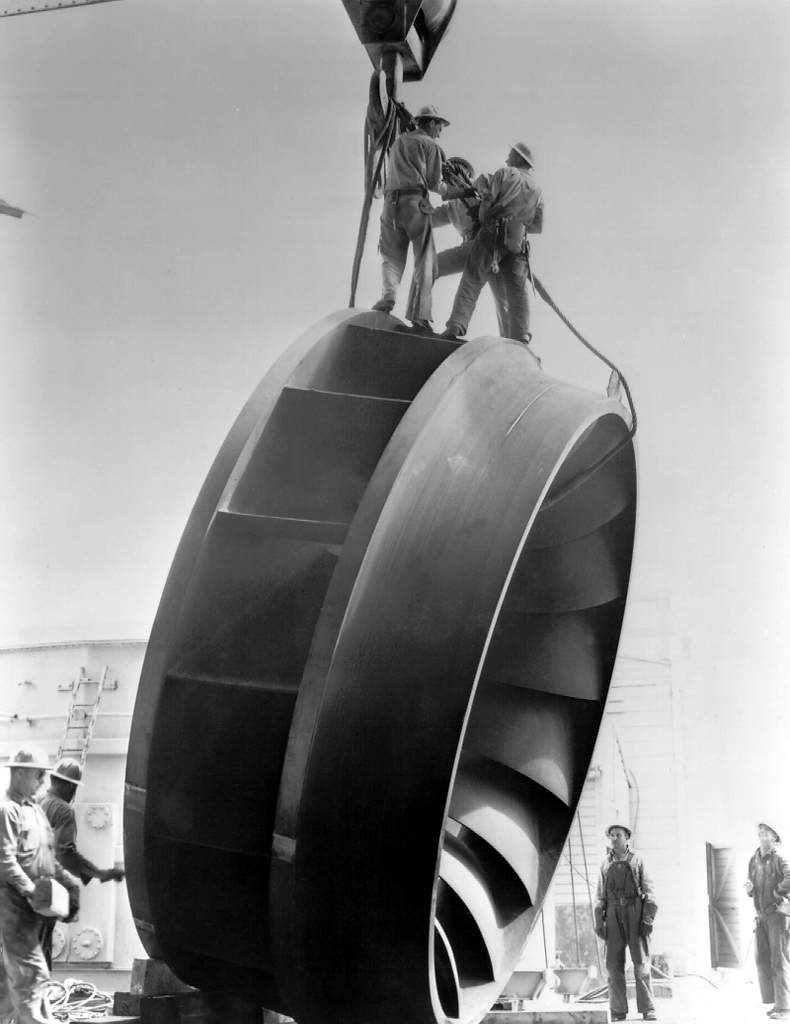
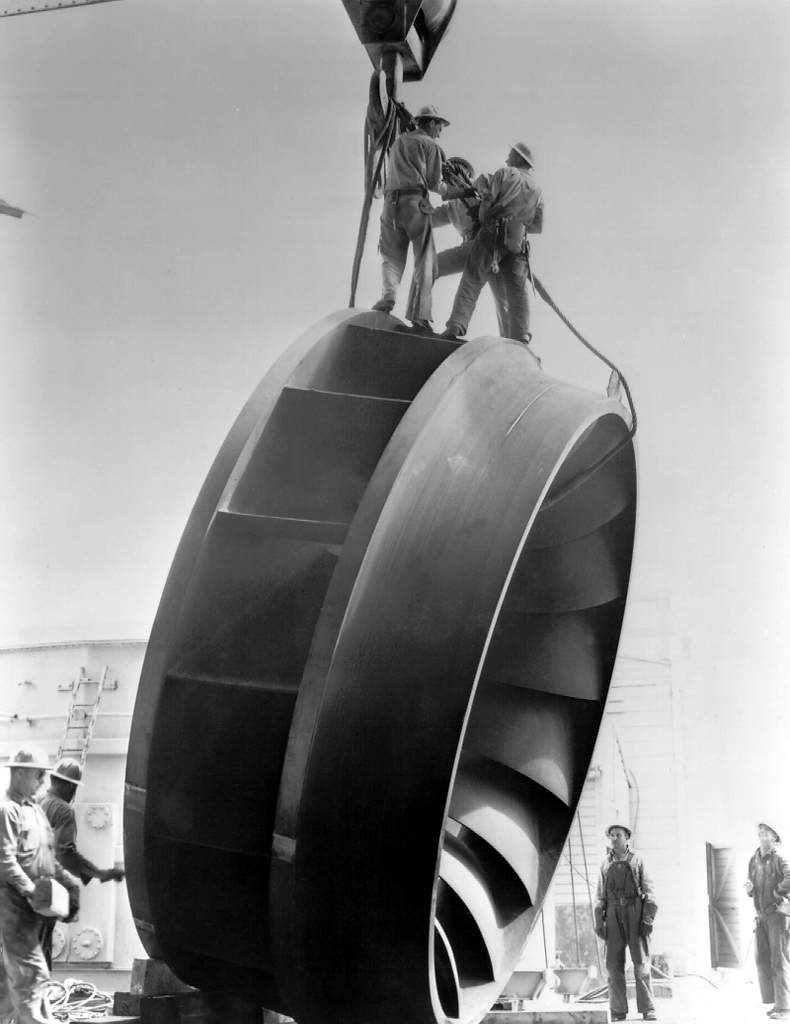
PAIRED-HYDRO | Increasing the Lifespan of Hydropower Turbines with Machine Learning
PAIRED-HYDRO | Increasing the Lifespan of Hydropower Turbines with Machine Learning


First National Calls: 50 selected projects to start in 2025
First National Calls: 50 selected projects to start in 2025
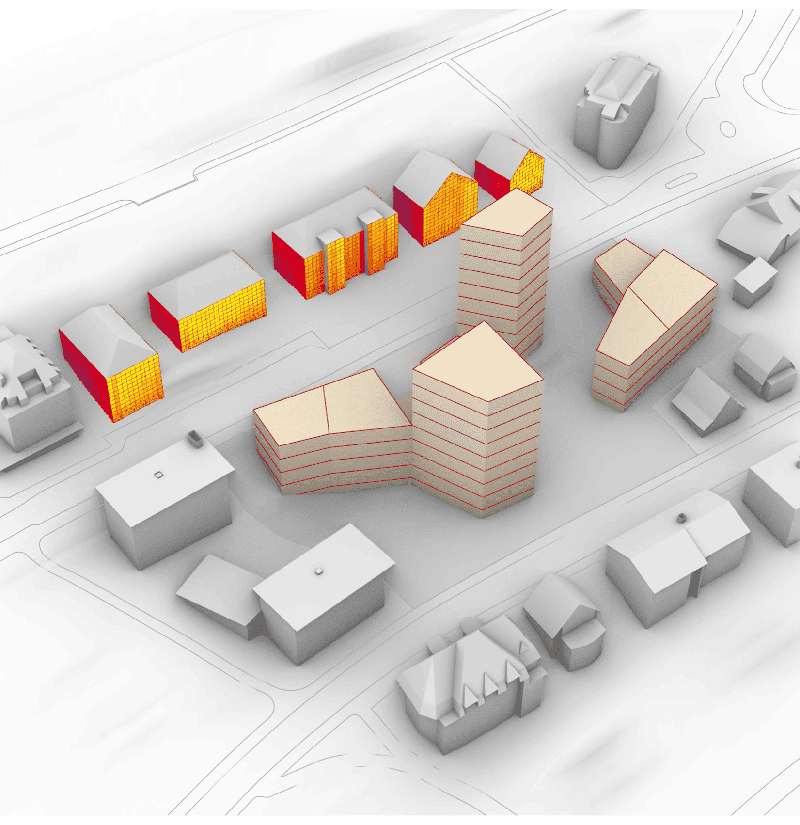
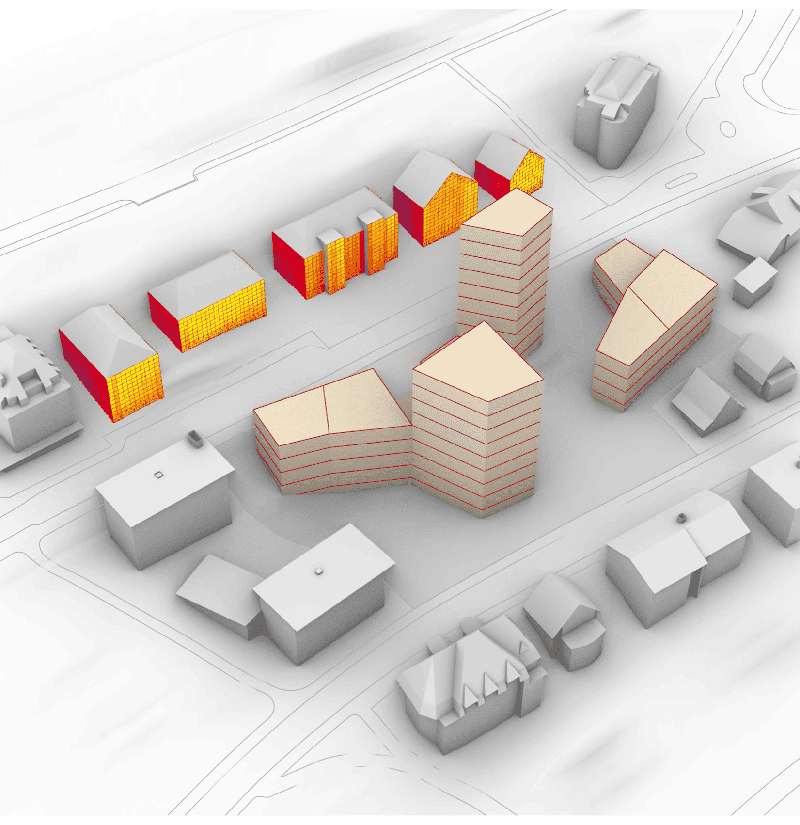
AIXD | Generative AI toolbox for architects and engineers
AIXD | Generative AI toolbox for architects and engineers
Contact us
Let’s talk Data Science
Do you need our services or expertise?
Contact us for your next Data Science project!