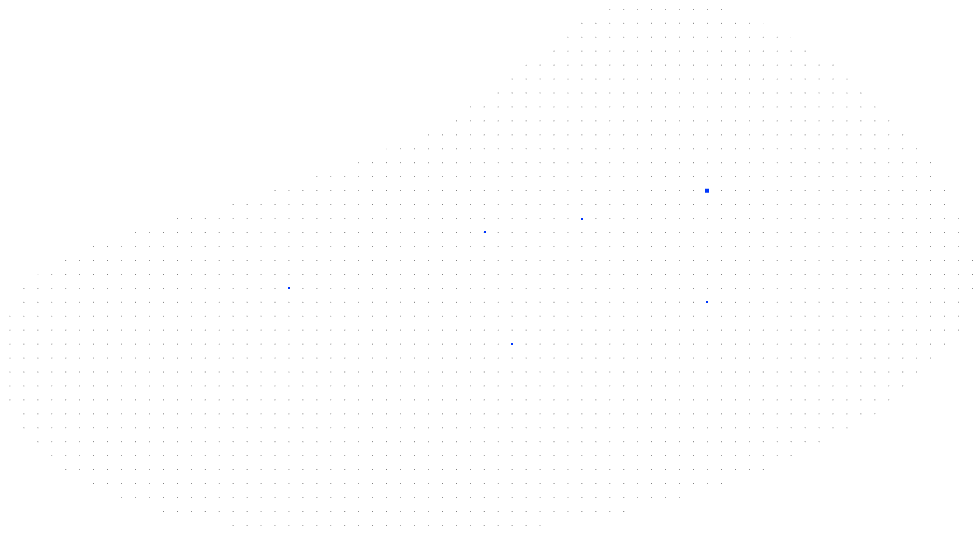
WATRES
A Data-Driven approach to estimate WATershed RESponses

Abstract
Water flowing through the landscape as groundwater or streamflow is made of innumerable water parcels of different ages (or residence times), which enter through precipitation and mix along their journey. Rivers often react quickly to rainfall events and can cause water quantity problems like
floods. But rivers are also known to transport significant amounts of “old” water, which is stored for years before being discharged and can have a large influence on water quality. While the timing of watershed responses is key to our understanding of flood generation and water quality processes, quantifying these responses is complex because they can be nonlinear, time-variable and may take irregular shapes that are difficult to predict a priori. The sensor revolution now provides both flow and tracer measurements at high resolution, but these technical advances have not been matched by data analysis techniques that can unleash the full power of the new data. Current methodologies typically rely on strong assumptions (e.g., stationarity) and on models that are calibrated against data but not yet data-driven. The goal of this project was to develop a new knowledge-guided but data-driven methodology to estimate the timing of watershed responses. This methodology leverages streamflow data from over 150 sites across Switzerland and streamflow concentration data from the highest-resolution Swiss water quality dataset.
This goal of this project was to develop a statistical learning algorithm to quantify water residence time distributions (and the associated uncertainty) from water quantity and water quality data. The algorithms has been applied to real-world watersheds to quantify the characteristic landscape responses in terms of water flow and water age. This project enabled us to make progress on a critical scientific challenge with significant societal relevance—ensuring water security in the face of floods and droughts, and promoting the fair distribution of this essential resource.
People
Collaborators


Quentin graduated with an engineering degree in mathematics and computer science from École des Ponts ParisTech in 2019. After a 6-month experience at the Center for Data Science of the New York University working on applied Machine Learning for medical imaging, he did a PhD in Statistics at Gustave Eiffel University (Paris). During his PhD, Quentin worked on random graphs and selective inference. His recent cross-disciplinary collaborations involve applications in biology and hydrology.
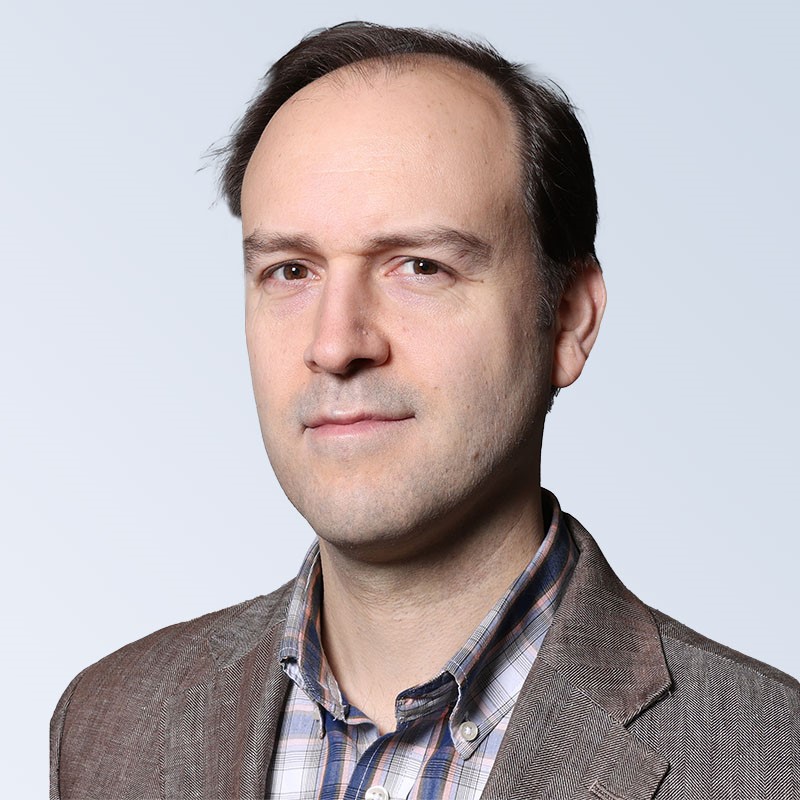
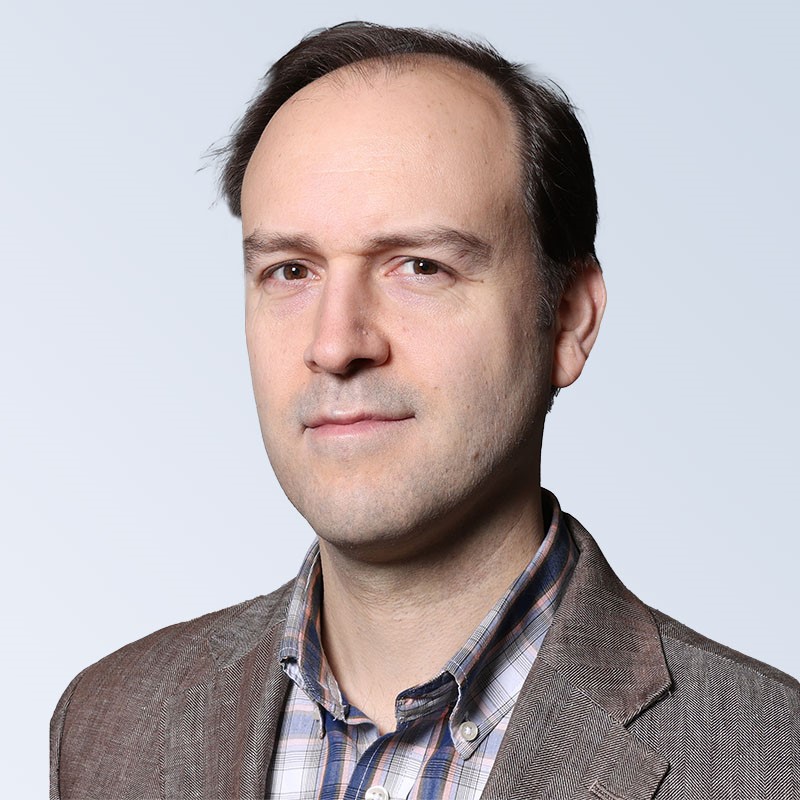
Guillaume Obozinski graduated with a PhD in Statistics from UC Berkeley in 2009. He did his postdoc and held until 2012 a researcher position in the Willow and Sierra teams at INRIA and Ecole Normale Supérieure in Paris. He was then Research Faculty at Ecole des Ponts ParisTech until 2018. Guillaume has broad interests in statistics and machine learning and worked over time on sparse modeling, optimization for large scale learning, graphical models, relational learning and semantic embeddings, with applications in various domains from computational biology to computer vision.
description
Motivation
Watershed responses are key to our understanding of flood generation and water quality processes, but quantifying these responses is complex because they can be nonlinear, time-variable and may take irregular shapes that are difficult to predict a priori. The sensor revolution now provides both flow and tracer measurements at high resolution, but these technical advances have not been matched by data analysis techniques that can unleash the full power of the new data.
Proposed Approach / Solution
The goal of this project was to develop a new knowledge-guided but data-driven methodology to estimate the response of watersheds in terms of water quantity and water quality (see Figure 1).
- For the water quantity problem, we developed a novel data-driven approach for estimating impulse response functions using Generalized Additive Models. This method captures the complex, nonlinear relationships between precipitation and runoff, providing a flexible and interpretable framework for systematically analyzing hydrological responses.
- For the water quality problem, we integrated concepts from StorAge Selection (SAS)-based methods, survival analysis, and mixture modeling to create a physically grounded, data-driven model for estimating transit time distributions.
Impact
Our models have been validated using synthetic datasets, demonstrating their ability to accurately recover the latent objects of interest—namely, transfer functions for the quantity problem and transit time distributions for the quality problem. We further applied our methods to real-world catchments, providing valuable insights into hydrological behavior. The results obtained from real data align well with those from state-of-the-art approaches, reinforcing confidence in the predictive capabilities of our models.
Future work will aim to fully harness the potential of these models by extending their application to a broader range of sites and tackling key hydrological questions. In particular, we seek to understand which factors—such as climate, topography, and watershed size—govern the timescales of flow and transport responses in real-world watersheds.
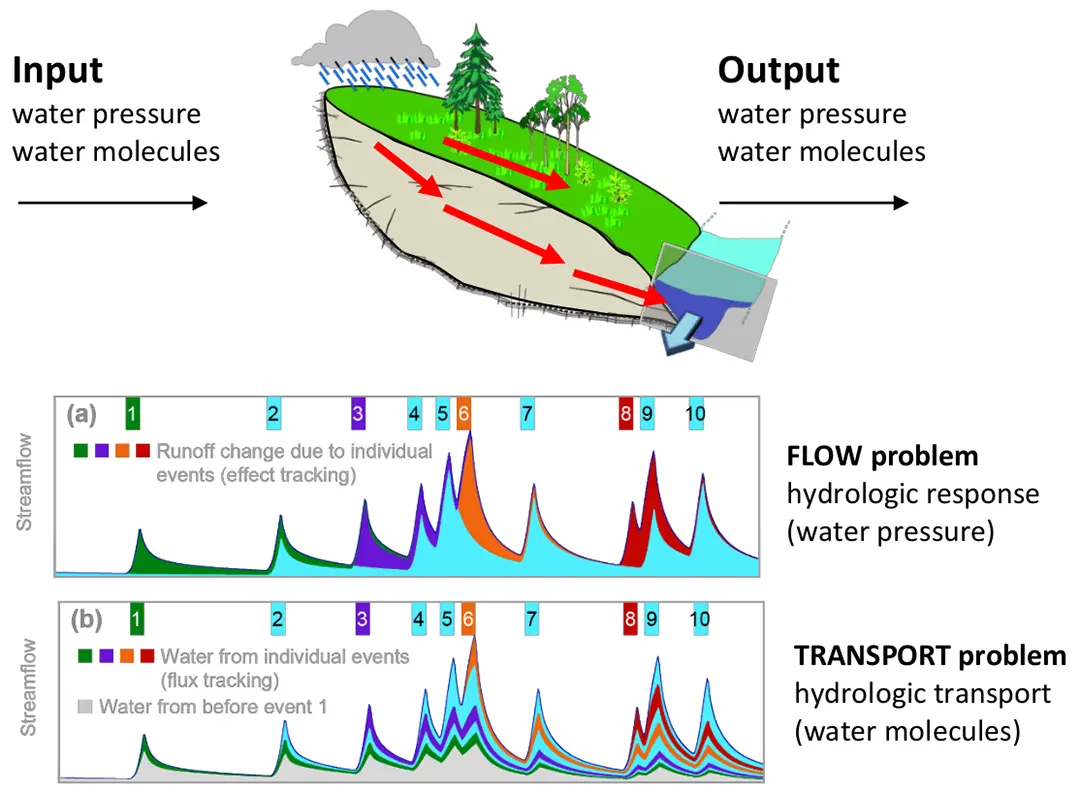
Presentation
Gallery
Annexe
Additional resources
Bibliography
- Kirchner, J. W. (2019). Quantifying new water fractions and transit time distributions using ensemble hydrograph separation: theory and benchmark tests. Hydrology and Earth System Sciences, 23(1), 303–349. doi:10.5194/hess-23-303-2019
- Benettin, P., & Bertuzzo, E. (2018). tran-SAS v1.0: a numerical model to compute catchment-scale hydrologic transport using StorAge Selection functions. Geoscientific Model Development, 11(4), 1627–1639. doi:10.5194/gmd-11-1627-2018
- Harman, C. J. (2015). Time-variable transit time distributions and transport: Theory and application to storage-dependent transport of chloride in a watershed. Water Resources Research, 51(1), 1–30. doi:10.1002/2014wr015707
- Kirchner, J. W. (2022). Impulse response functions for nonlinear, nonstationary, and heterogeneous systems, estimated by deconvolution and demixing of noisy time series. Sensors (Basel, Switzerland), 22(9), 3291. doi:10.3390/s22093291
Publications
Related Pages
More projects
MAGNIFY
News
Latest news


First National Calls: 50 selected projects to start in 2025
First National Calls: 50 selected projects to start in 2025
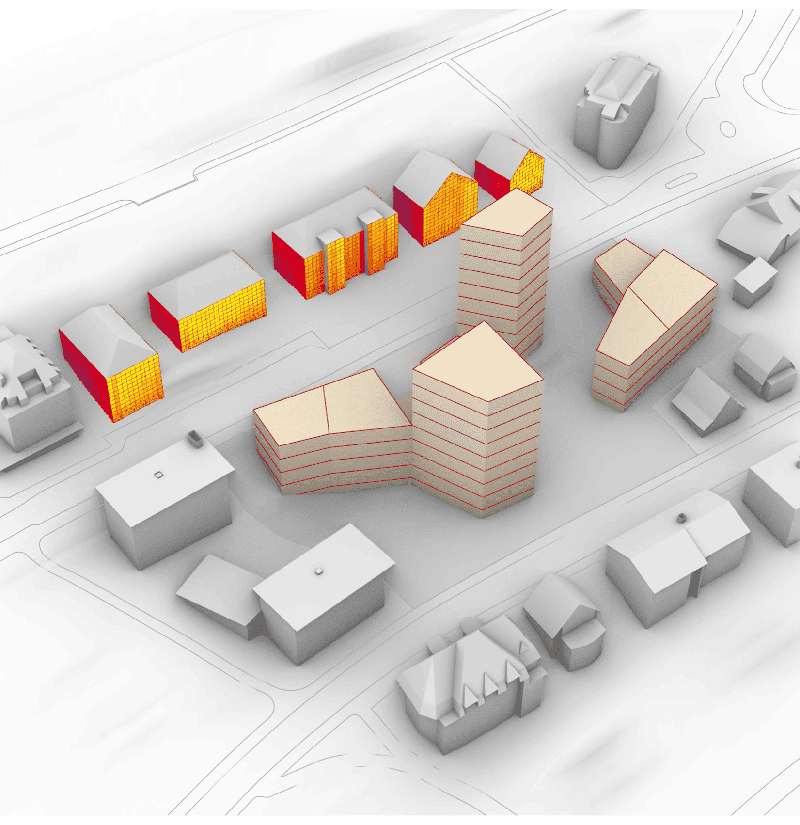
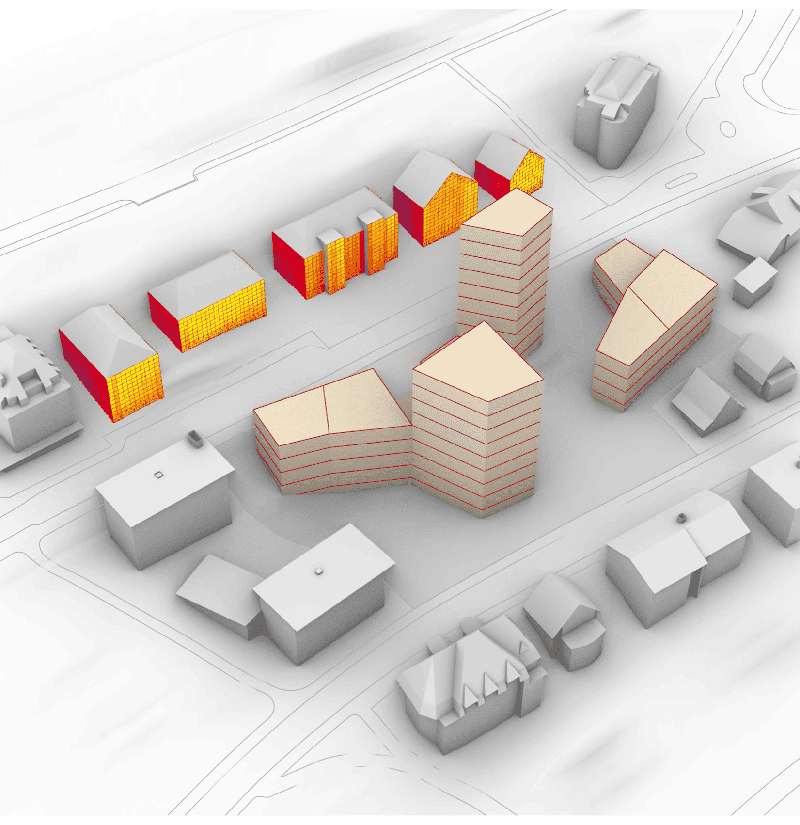
AIXD | Generative AI toolbox for architects and engineers
AIXD | Generative AI toolbox for architects and engineers


Smartair | An active learning algorithm for real-time acquisition and regression of flow field data
Smartair | An active learning algorithm for real-time acquisition and regression of flow field data
Contact us
Let’s talk Data Science
Do you need our services or expertise?
Contact us for your next Data Science project!