
SenseDynamics
Predicting aerodynamics forces from sensor data
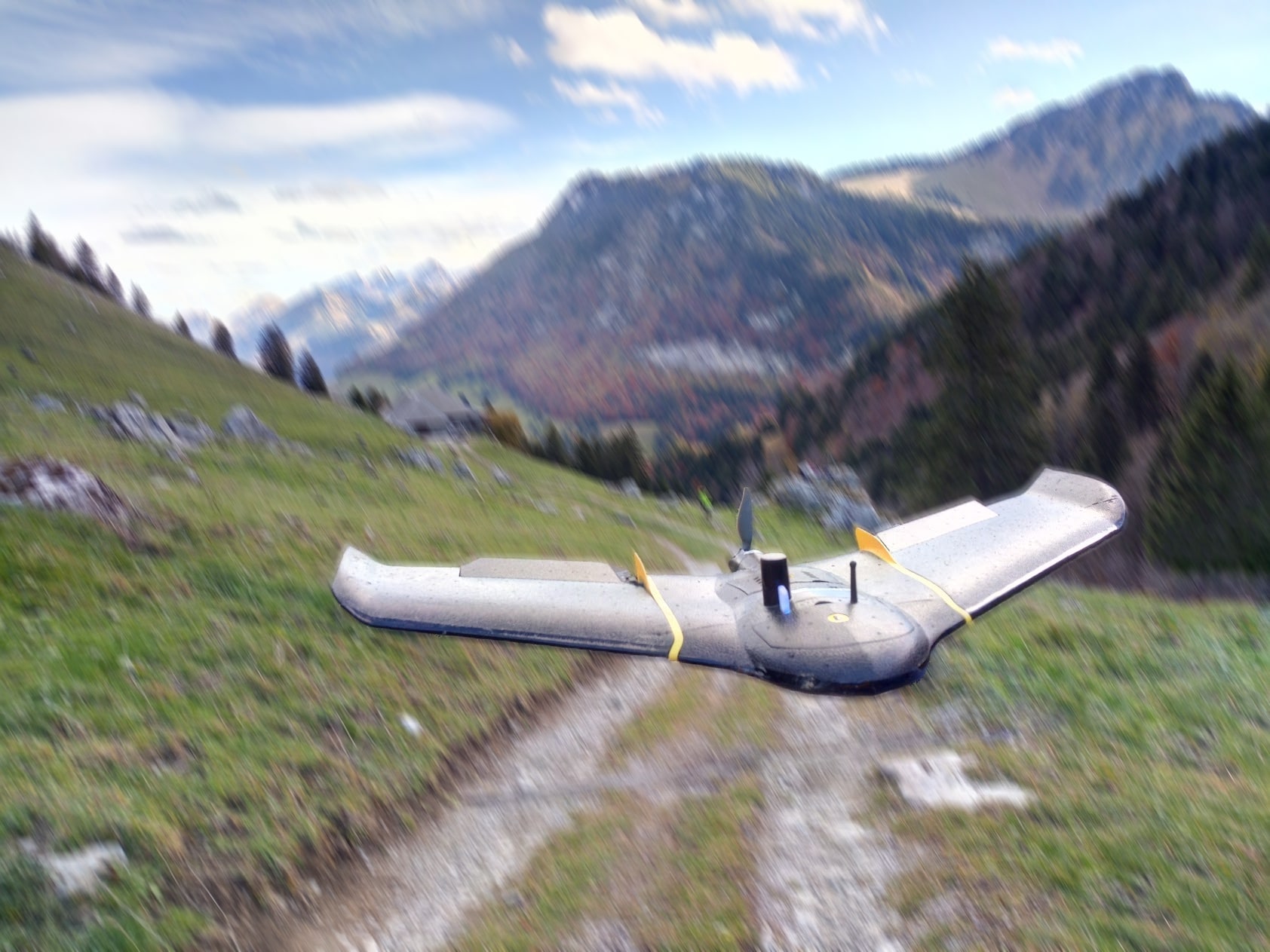
Abstract
To advance state-of-the-art Machine Learning capabilities when creating precise surrogate models of transient nonlinear physical phenomena related to aerodynamics with known (quantified probability density function describing it) and traceable uncertainty. Create the capability to reconstruct such complex physical transient phenomenon with a minimum amount of discrete real sensorial input of finite and calibrated precision.
People
Collaborators
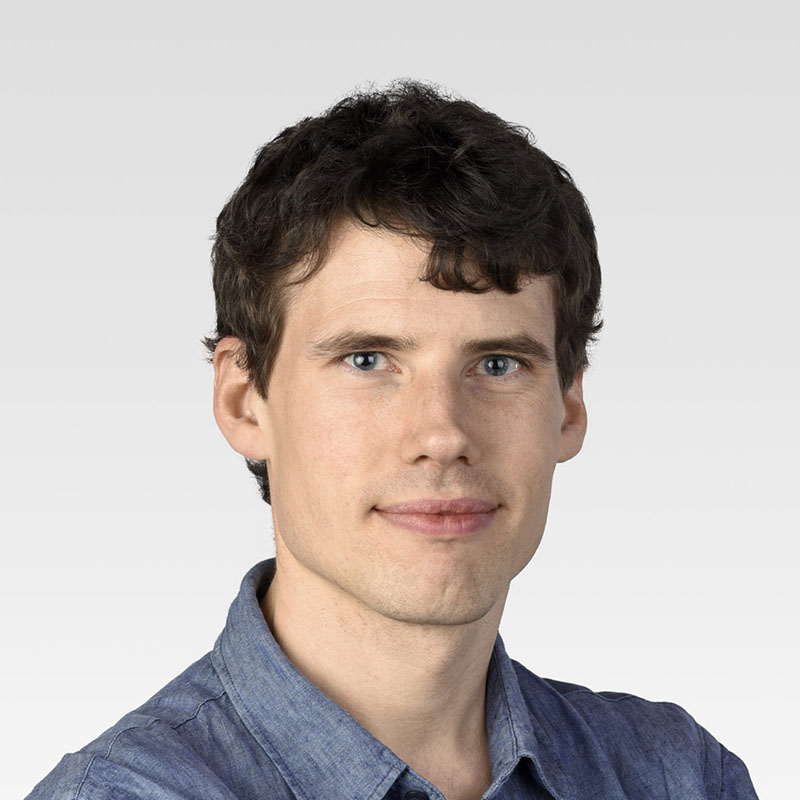
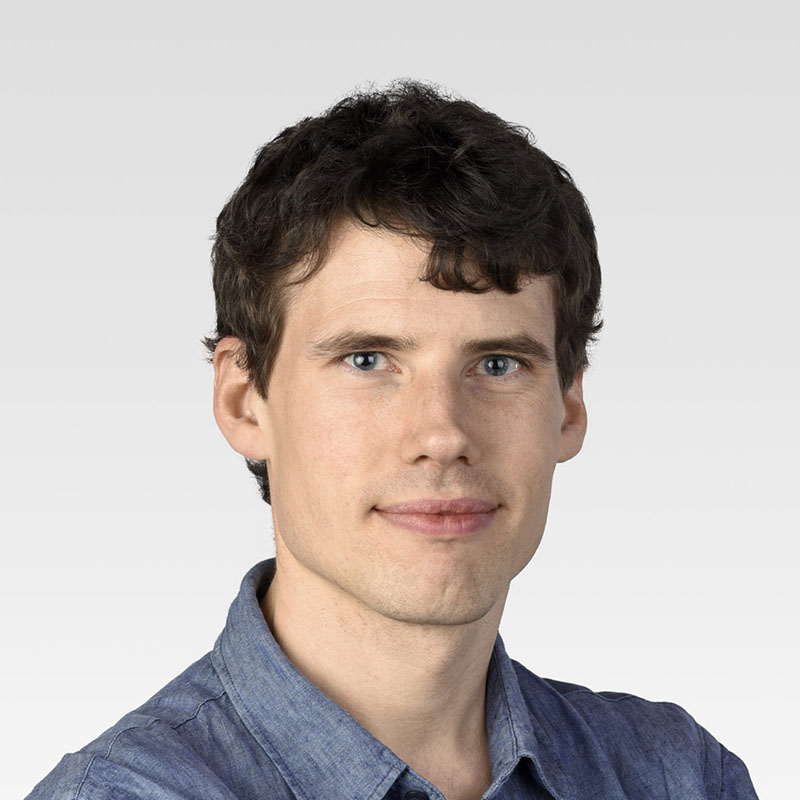
Christian joined SDSC in 2019 to design data-driven solutions to support academic research projects. His research interests comprise inference of probabilistic models, with a focus on nonparametric models, and stochastic processes. He holds a PhD in Computational Neuroscience from the Technical University Berlin, Germany, a MSc from the Bernstein Center for Computational Neuroscience Berlin, and a BSc in Cognitive Science from the University of Osnabrück, Germany. During the course of his studies, he additionally conducted research at Khalifa University (UAE), Strathclyde University (UK), and the RIKEN Brain Science Institute and the Kyoto University in Japan. Before joining SDSC, he was an intern at Amazon CoreAI in Berlin.
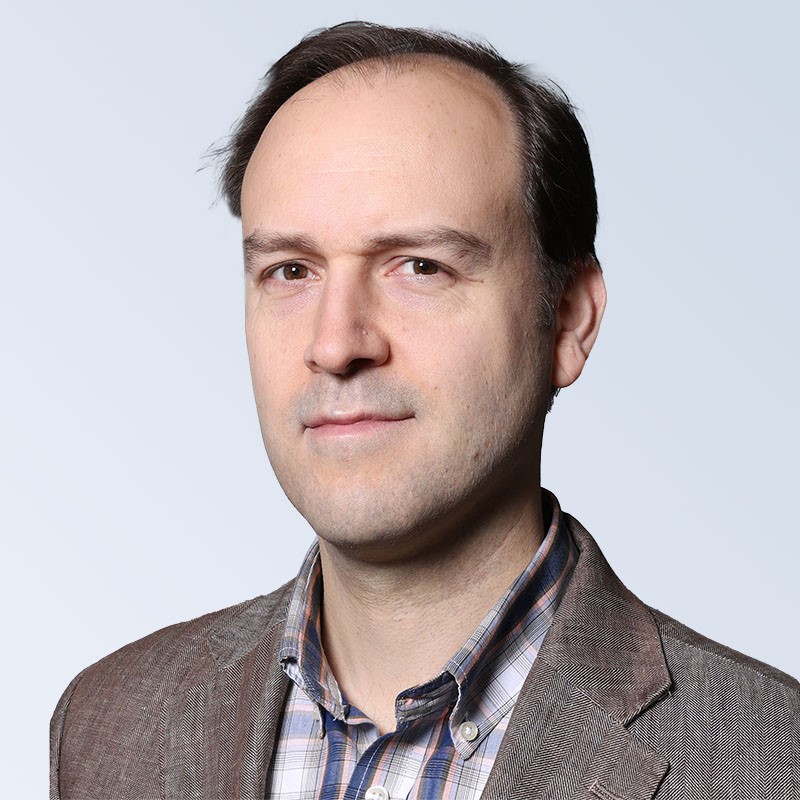
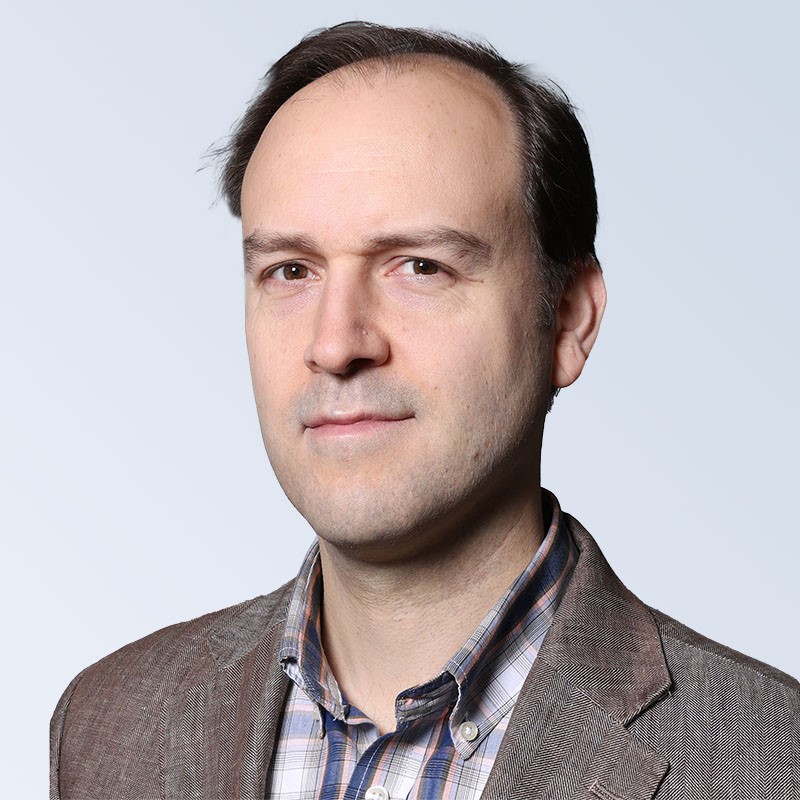
Guillaume Obozinski graduated with a PhD in Statistics from UC Berkeley in 2009. He did his postdoc and held until 2012 a researcher position in the Willow and Sierra teams at INRIA and Ecole Normale Supérieure in Paris. He was then Research Faculty at Ecole des Ponts ParisTech until 2018. Guillaume has broad interests in statistics and machine learning and worked over time on sparse modeling, optimization for large scale learning, graphical models, relational learning and semantic embeddings, with applications in various domains from computational biology to computer vision.
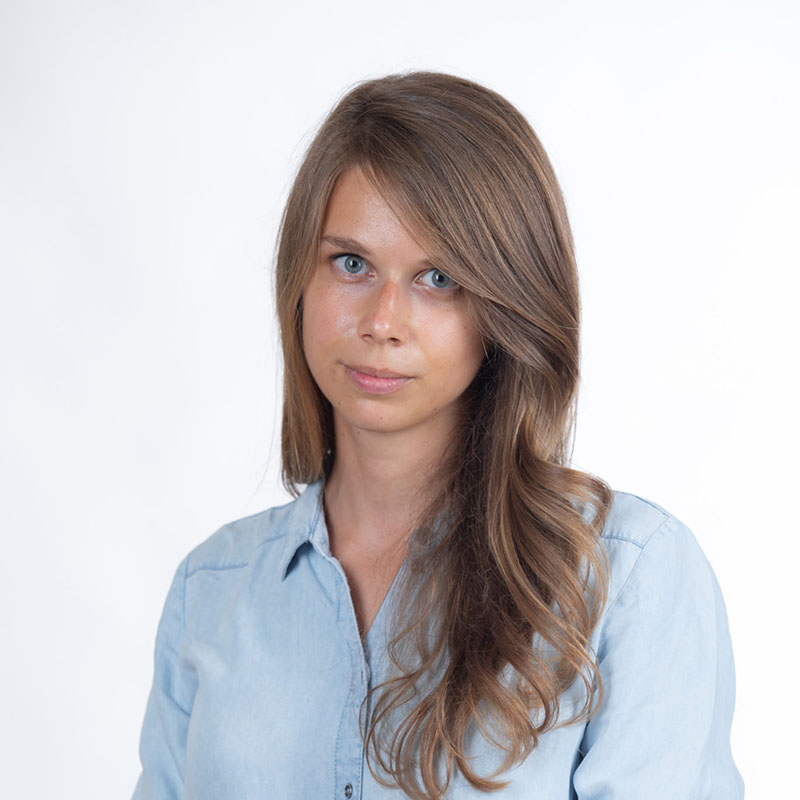
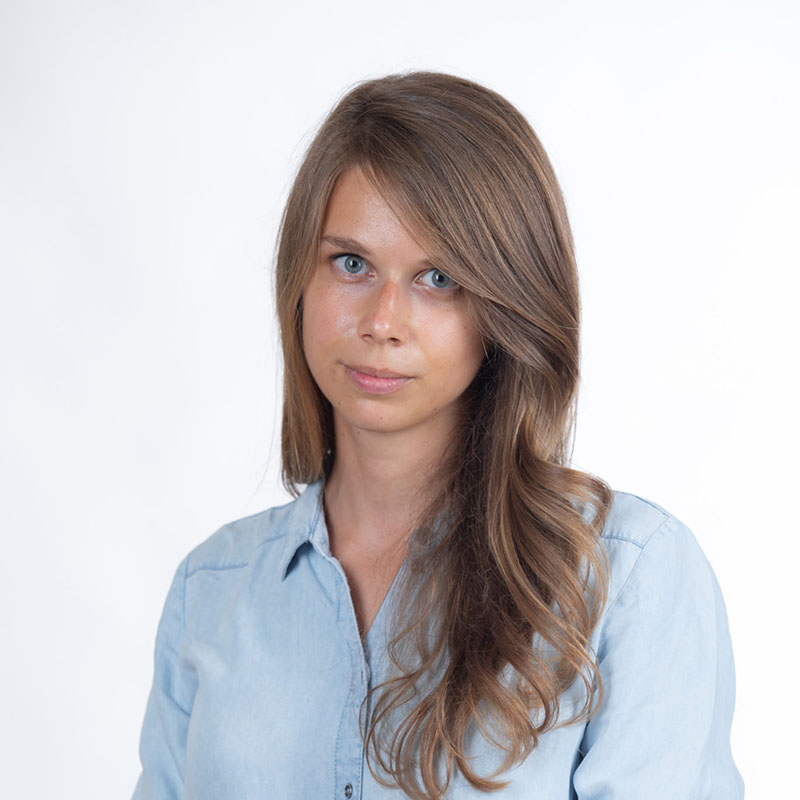
Natasa is a computer scientist by training, with a Bachelor degree in Computer Science and Engineering and Master degree in Embedded Systems from Ss. Cyril and Methodius University in Skopje, North Macedonia. She finished her doctoral studies in Information Systems at UNIL- HEC Lausanne, under the supervision of Valérie Chavez-Demoilun. During her studies she did an internship at NATO and Facebook AI Research. Her research interest focuses on causal inference, generative models, uncertainty and interpretability.
PI | Partners:
description
Nowadays, autonomous navigation of aircraft vehicles relies mostly on systems like GPS providing a signal to determine the vehicle’s position. To avoid an uncontrolled flight in the case of signal interruption, robust fallback algorithms are required. Data-driven strategies should allow for accurate navigation by using information that remains available, such as sensor measurements on board.
Despite the notable advances in artificial intelligence, recently developed algorithms frequently fail to fulfil robustness requirements to employ data-driven systems in the real world.
Therefore, to mindfully tackle the question of autonomous navigation, in the SenseDynamics project, the SDSC supports a highly multidisciplinary research collaboration established between three traditionally unrelated laboratories at EPFL (TOPO – topography and navigation, UNFOLD – unsteady experimental aerodynamics, MCSS – computational mathematics and simulation science), and the Swiss Federal Bureau of Meteorology and Standardisation METAS. Each of these groups is essential in the effort towards unmanned aerial vehicles that would be also GPS-free, navigating through air only by a machine learning model which uses data from a limited number of sensors.
In achieving these objectives, the SenseDynamics project uses high-fidelity data recorded in an experiment from diverse movements of an airfoil of a wing in a wind tunnel, provided by the UNFOLD group. Additional simulated data is provided by the MCSS, which is cheaper, less accurate, but indeed matches closely the expected physical behaviour under diverse unseen setups. The SDSC takes on the challenge to combine these multi-fidelity data sources, and design a machine learning model able to predict the necessary forces. At the same time, the model should fulfil the desired reliability and robustness criteria, which are finally being validated by a pipeline set up in METAS.
In a broader perspective, the SenseDynamics project should demonstrate how machine learning and data science – guided by domain expertise – can provide effective and practical solutions for real world challenges.
Presentation
Gallery
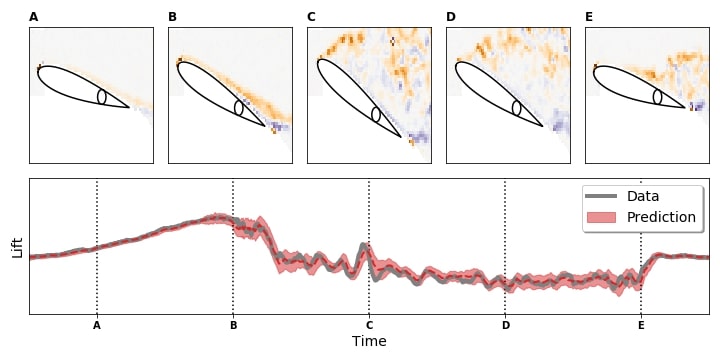
Annexe
Additionnal resources
Bibliography
- Deparday, J., Mulleners, K. (2019). Modeling the interplay between the shear layer and leading edge suction during dynamic stall Physics of Fluids 31(10), 107104. https://dx.doi.org/10.1063/1.5121312
- Mulleners, K., Raffel, M. (2013). Dynamic stall development Experiments in Fluids 54(2), 1469 1477. https://dx.doi.org/10.1007/s00348-013-1469-7
- Mulleners, K., Raffel, M. (2012). The onset of dynamic stall revisited Experiments in Fluids 52(3), 779 793. https://dx.doi.org/10.1007/s00348-011-1118-y
- Leishman, J. (2002). Challenges in modelling the unsteady aerodynamics of wind turbines Wind Energy 5(2-3), 85 132. https://dx.doi.org/10.1002/we.62
- Alsalman, M., Colvert, B., Kanso, E. (2018). Training bioinspired sensors to classify flows. Bioinspiration & biomimetics 14(1), 016009. https://dx.doi.org/10.1088/1748-3190/aaef1d
- Colvert, B., Alsalman, M., Kanso, E. (2017). Classifying vortex wakes using neural networks arXiv 13(2), 025003. https://dx.doi.org/10.1088/1748-3190/aaa787
- Tuna, B., Kurelek, J., Yarusevych, S. (2019). Surface-Pressure-Based Estimation of the Velocity Field in a Separation Bubble AIAA Journal 57(9), 3825-3837. https://dx.doi.org/10.2514/1.j0580
Publications
Related Pages
More projects
CLIMIS4AVAL
News
Latest news
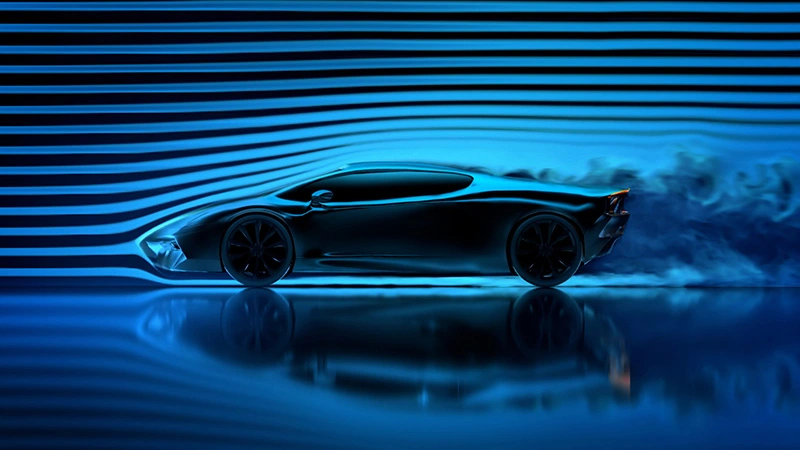
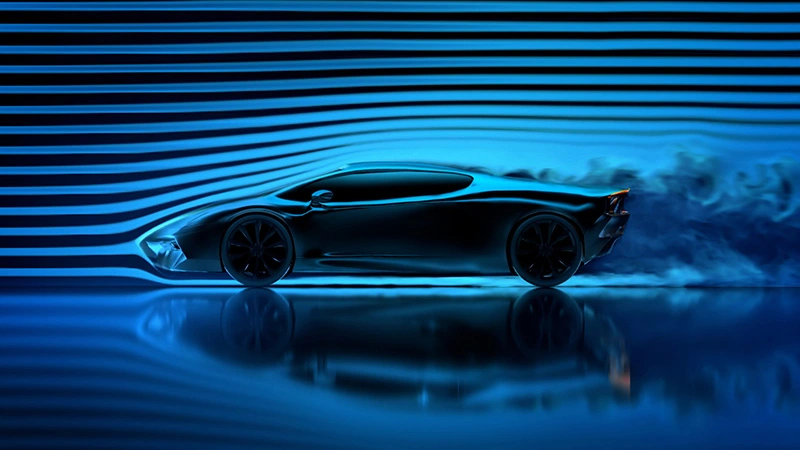
Smartair | An active learning algorithm for real-time acquisition and regression of flow field data
Smartair | An active learning algorithm for real-time acquisition and regression of flow field data
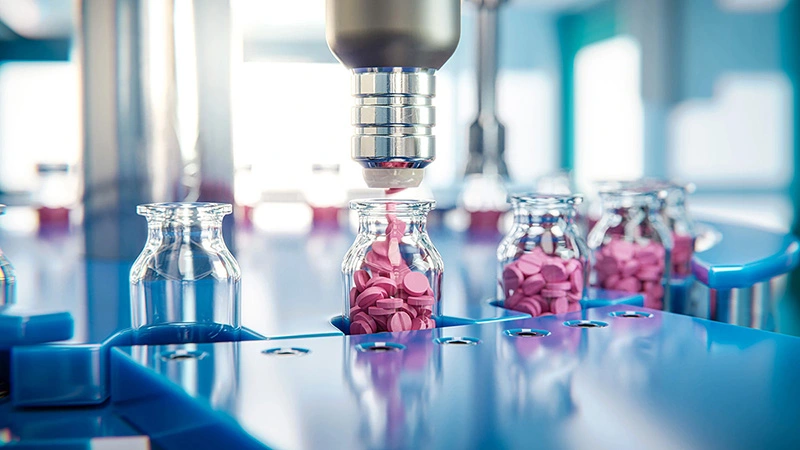
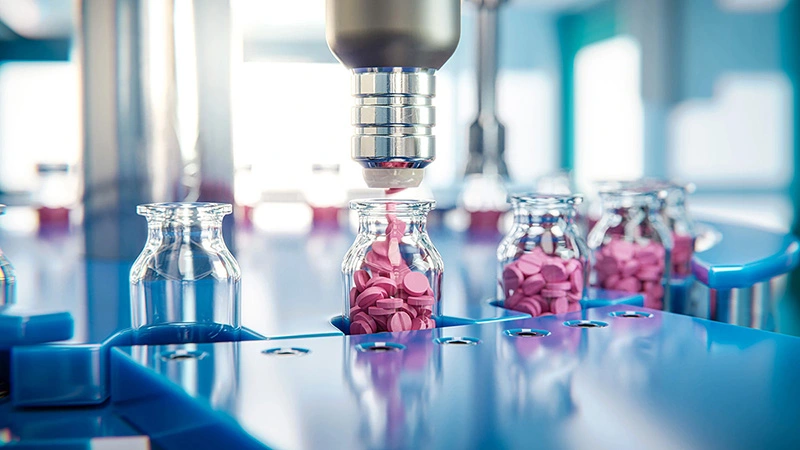
The Promise of AI in Pharmaceutical Manufacturing
The Promise of AI in Pharmaceutical Manufacturing
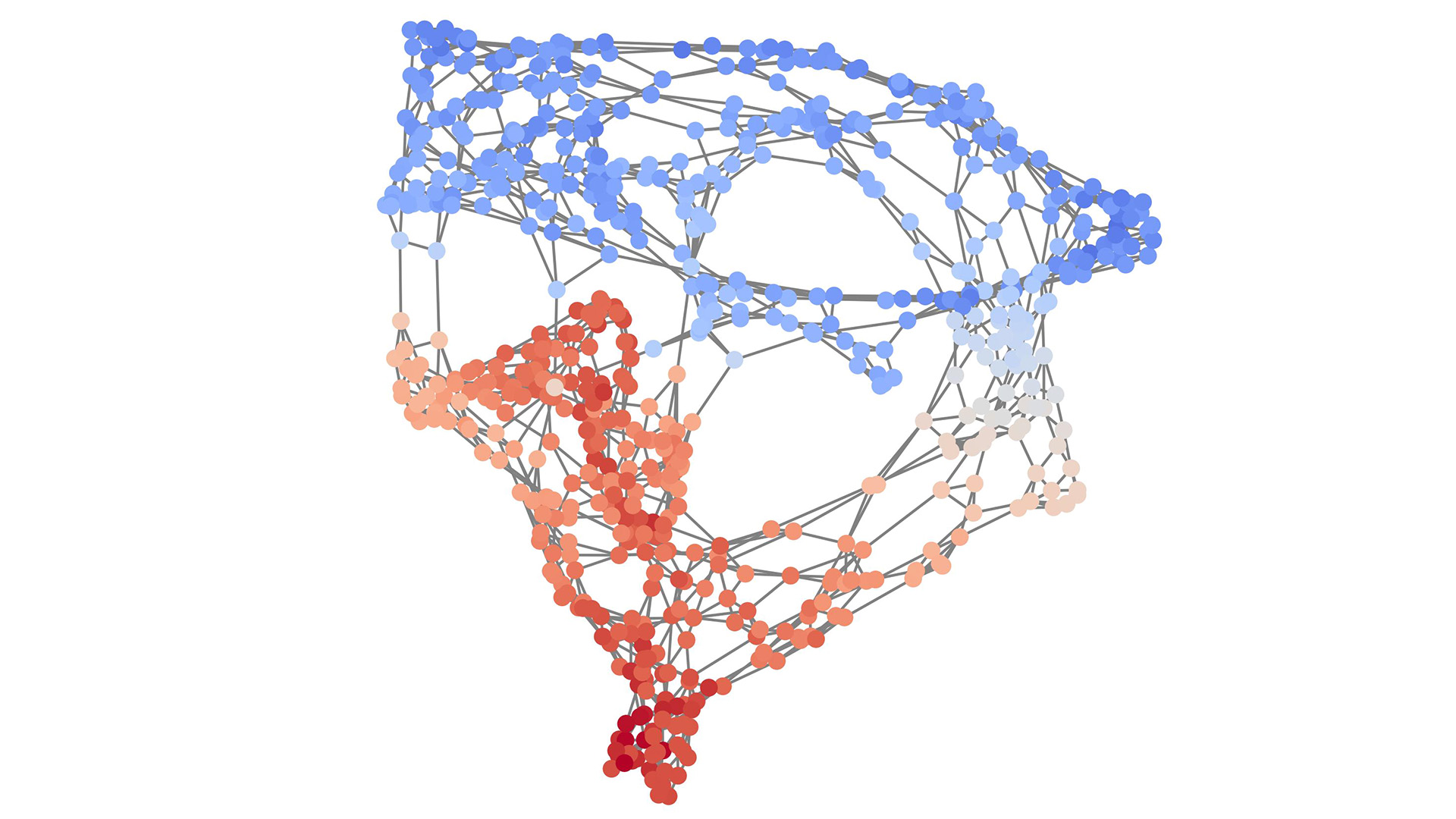
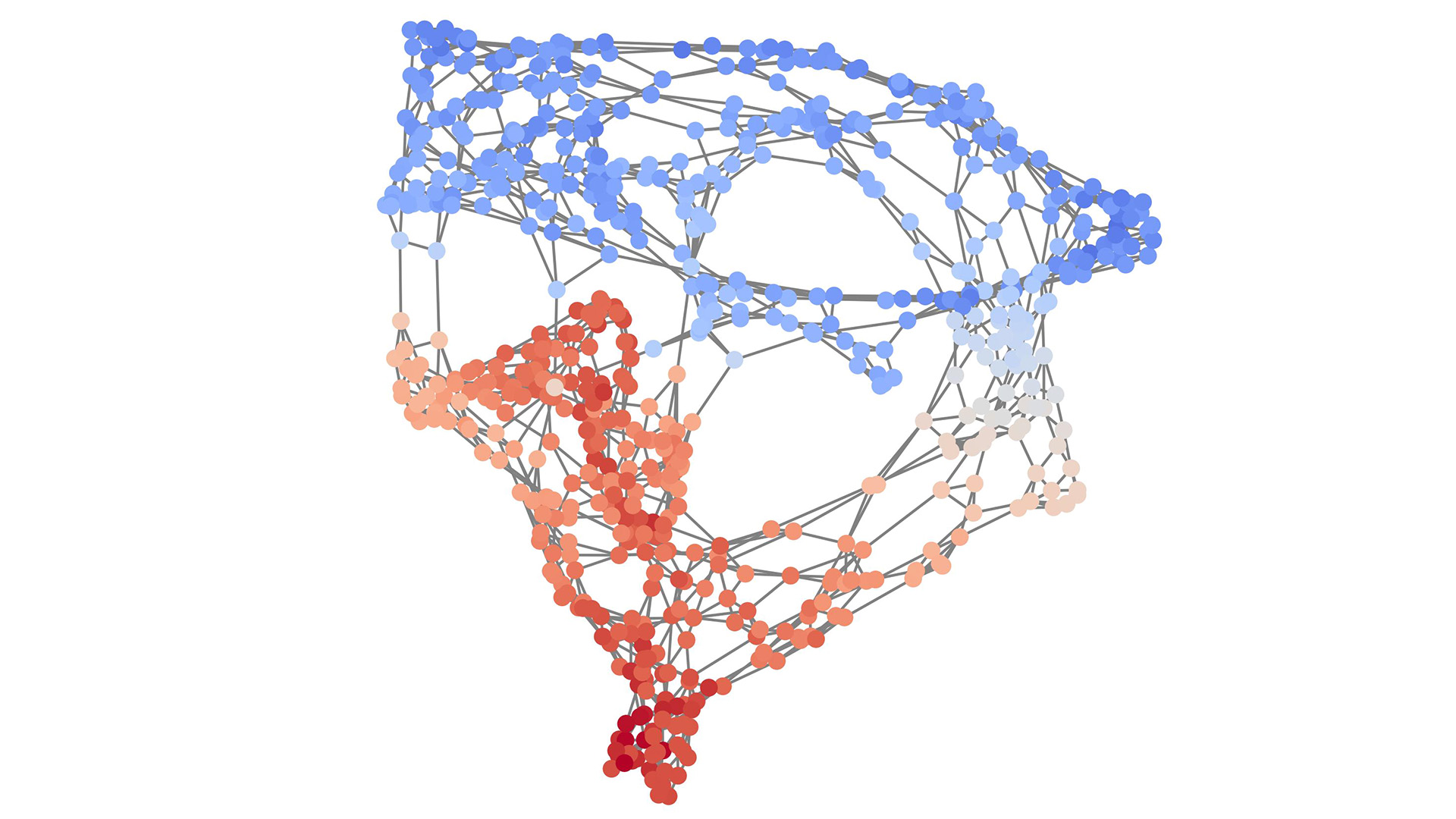
Efficient and scalable graph generation through iterative local expansion
Efficient and scalable graph generation through iterative local expansion
Contact us
Let’s talk Data Science
Do you need our services or expertise?
Contact us for your next Data Science project!