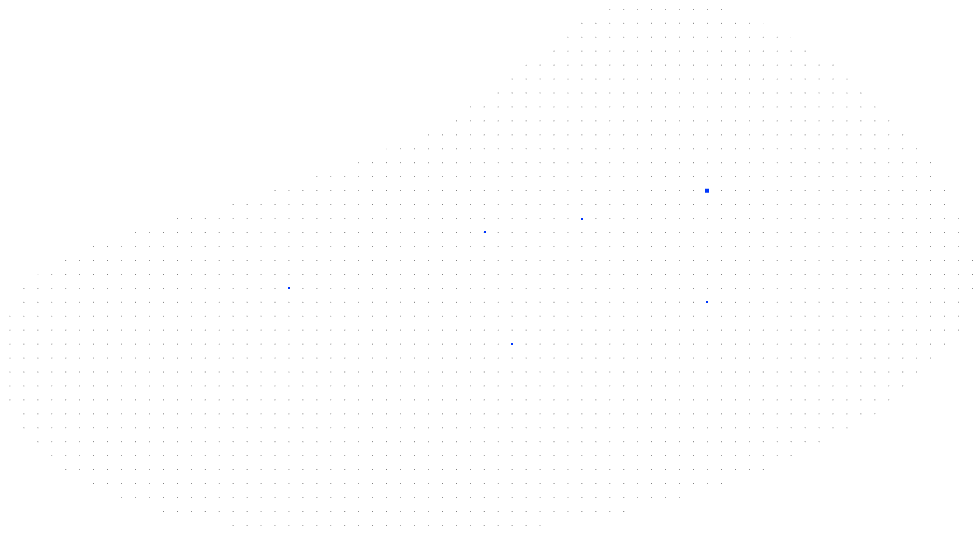
MMAI-MS
Multi-Modal Analysis and Integration of MS Patient Data

Abstract
This project aims to create computational models for predicting the course of the Multiple Sclerosis (MS) disease and stratifying for treatment responses to support clinicians. Based on an already collected data corpus and ongoing data collection, we will adapt and develop novel machine learning methods to exploit the variety of data modalities focusing on disease state characterization and disease progression modeling. This includes natural language processing (NLP) for feature extraction from clinical data, automatic feature detection in magnetic resonance images (MRI), models for longer-term temporal data, and integrative models that combine information from different data sources. Together, these models will allow generating reports for longitudinal comparisons of MS patients to support patient care.
This project is co-funded by PHRT.
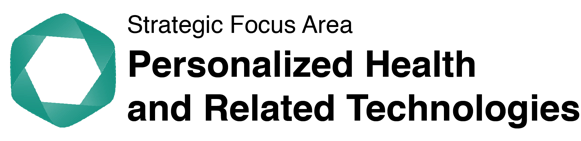
People
Collaborators
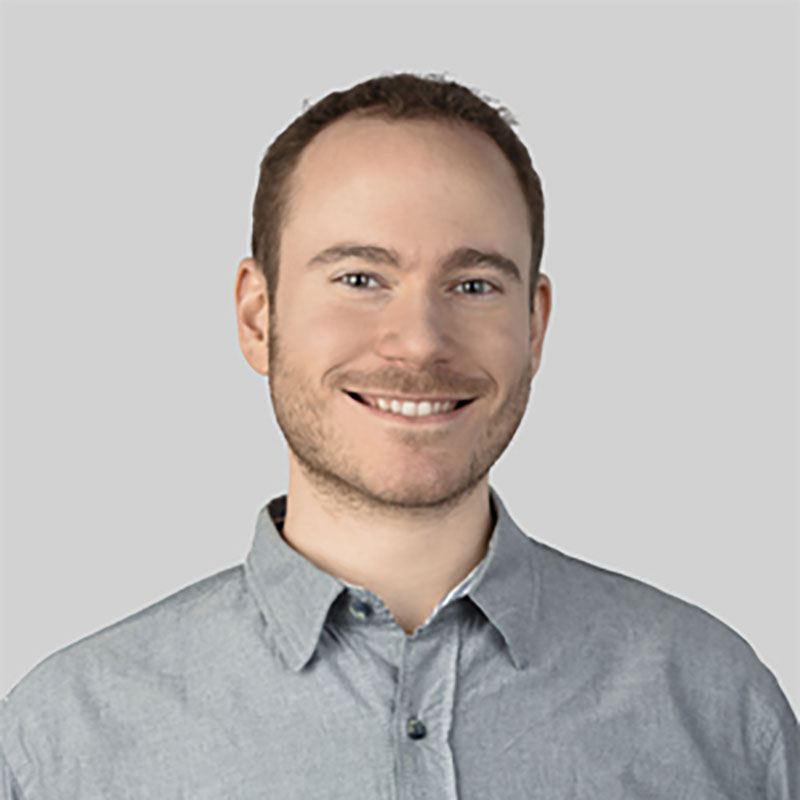
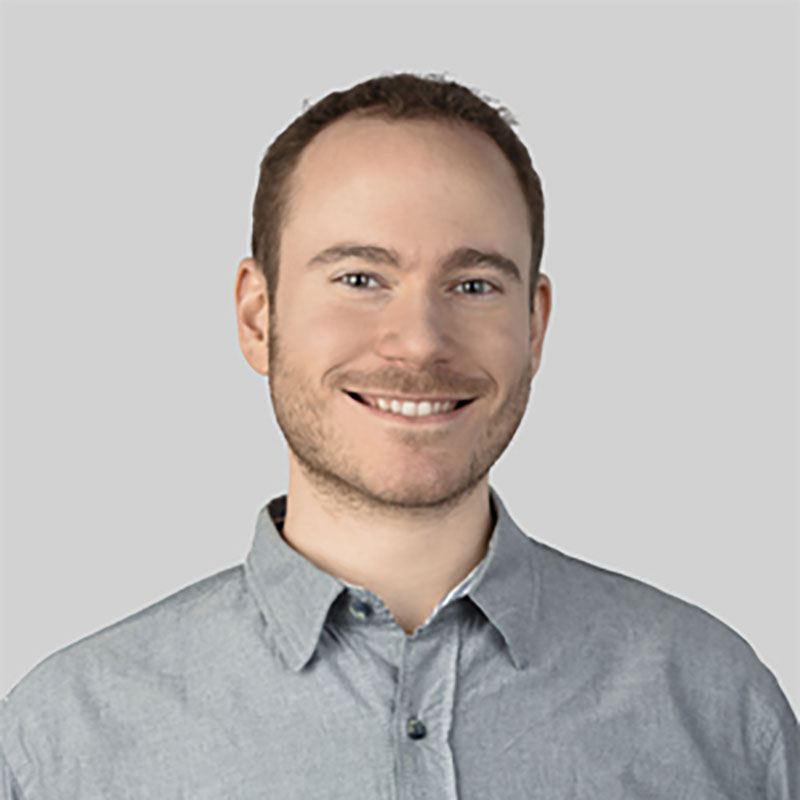
Firat completed his undergraduate studies in Electronics Engineering at Sabanci University. He later received his MSc. in Electrical and Electronics Engineering from EPFL. He conducted his doctoral studies on medical image segmentation in Computer Vision Lab at ETH Zurich. In between, he visited INRIA (Sophia Antipolis, France) and ABB Corporate Research Center (Baden, Switzerland). His research interests revolve around computer vision and machine learning, with a focus on the medical domain. He has been with SDSC since 2019.


Luis is originally from Spain, where he completed his bachelor's studies in Electrical engineering, and the Ms.C. on signal theory and communications, both at the University of Seville. During his Ph.D. he started focusing on machine learning methods, more specifically message passing techniques for channel coding, and Bayesian methods for channel equalization. He carried it out between the University of Seville and the University Carlos III in Madrid, also spending some time at the EPFL, Switzerland, and Bell Labs, USA, where he worked on advanced techniques for optical channel coding. When he completed his Ph.D. in 2013, he moved to the Luxembourg Center on Systems Biomedicine, where he switched his interest to neuroscience, neuroimaging, life sciences, etc., and the application of machine learning techniques to these fields. During his 4 and a half years there as a Postdoc, he worked on many different problems as a data scientist, encompassing topics such as microscopy image analysis, neuroimaging, single-cell gene expression analysis, etc. He joined the SDSC in April 2018. As Lead Data Scientist, Luis coordinates projects in various domains. Several projects focus on the application of natural language processing and knowledge graphs to the study of different phenomena in social and political sciences. In the domains of architecture and engineering, Luis is responsible for projects centered on the application of novel generative methods to parametric modeling. Finally, Luis also coordinates different projects in robotics, ranging from collaborative robotic construction to deformable object manipulation.
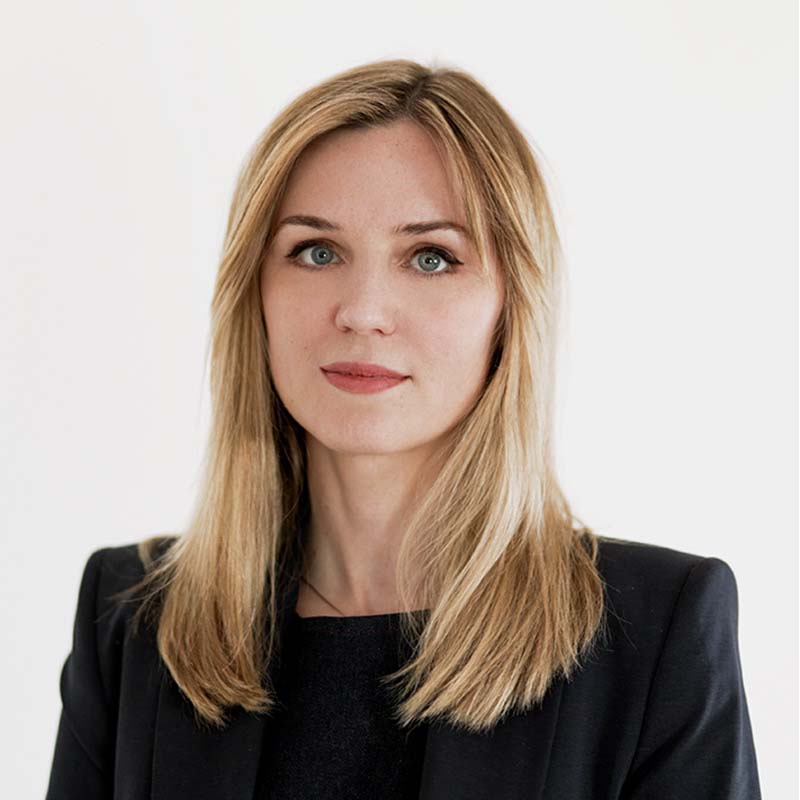
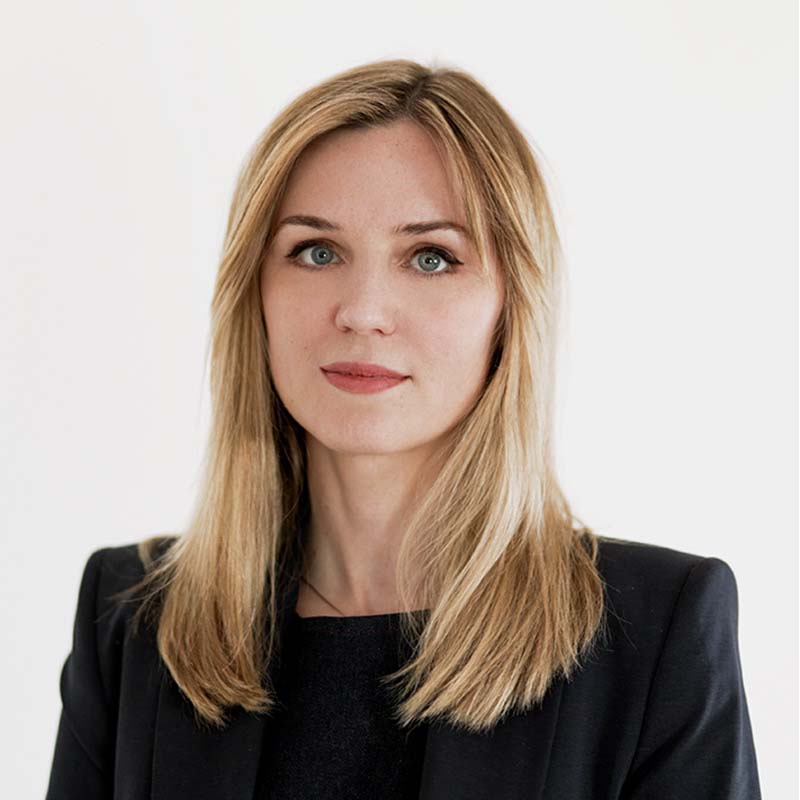
Ekaterina received her PhD in Computer Science from Moscow Institute for Physics and Technology, Russia. Afterwards, she worked as a researcher at the Institute for Information Transmission Problems in Moscow and later as a postdoctoral researcher in the Stochastic Group at the Faculty of Mathematics at University Duisburg-Essen, Germany. She has experience with various applied projects on signal processing, predictive modelling, macroeconomic modelling and forecasting, and social network analysis. She joined the SDSC in November 2019. Her interests include machine learning, non-parametric statistical estimation, structural adaptive inference, and Bayesian modelling.
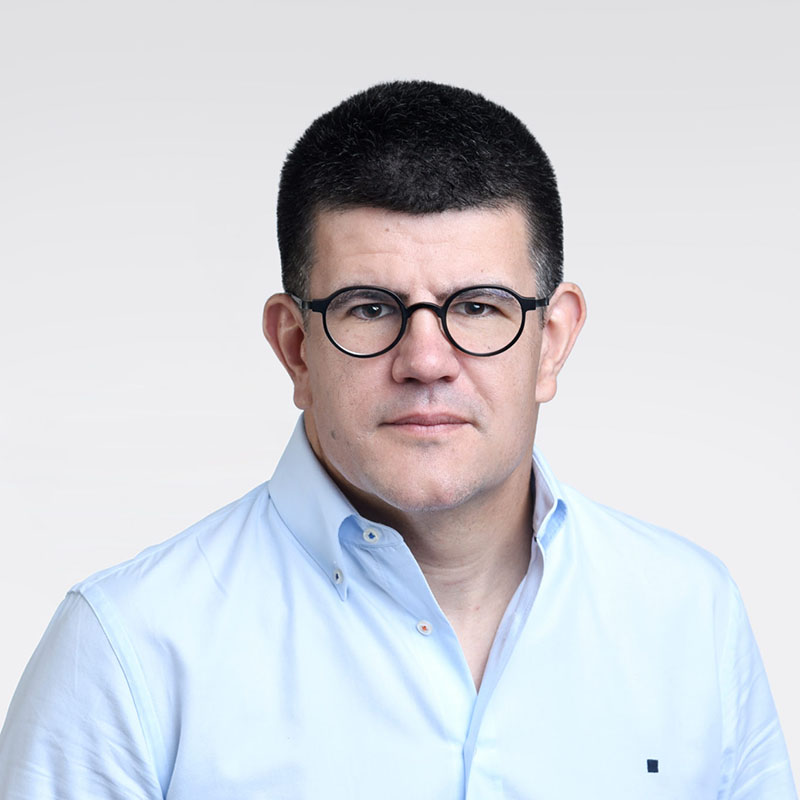
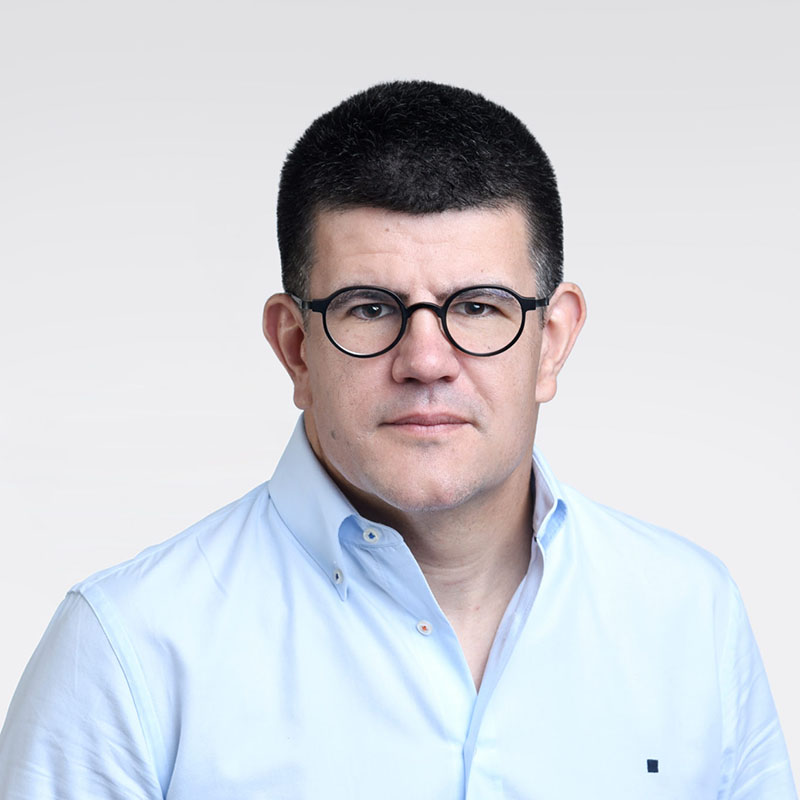
Fernando Perez-Cruz received a PhD. in Electrical Engineering from the Technical University of Madrid. He is Titular Professor in the Computer Science Department at ETH Zurich and Head of Machine Learning Research and AI at Spiden. He has been a member of the technical staff at Bell Labs and a Machine Learning Research Scientist at Amazon. Fernando has been a visiting professor at Princeton University under a Marie Curie Fellowship and an associate professor at University Carlos III in Madrid. He held positions at the Gatsby Unit (London), Max Planck Institute for Biological Cybernetics (Tuebingen), and BioWulf Technologies (New York). Fernando Perez-Cruz has served as Chief Data Scientist at the SDSC from 2018 to 2023, and Deputy Executive Director of the SDSC from 2022 to 2023
PI | Partners:
ETH Zurich, Department of Computer Science
- Prof. Dr. Christian Holz
- Prof. Dr. Gunnar Rätsch
- Dr. Cristóbal Esteban
- Dr. Martina Baumann
- Dr. Rita Kuznetsova
- Dr. Neda Davoudi
- Dr. Shkurta Gashi
- Dr. Liliana Barrios
- Max Möbus
More info: siplab.org | bmi.inf.ethz.ch
University Hospital Zurich, Neuroimmunology Department:
- Prof. Dr. med. Roland Martin
- Prof. Dr. Andreas Lutteroti
- Dr. med. Dr. sc. nat. Veronika Kana
- Dr. med. univ. Marc Hilty
- Prof. Dr. med. Patrick Roth
description
Motivation
Multiple Sclerosis presents with a wide range of symptoms, imaging findings, and underlying mechanisms of inflammation and nerve damage. Among these, fatigue and poor sleep quality are particularly common and significantly impact patients’ quality of life and long-term outcomes. As a result, accurately assessing disease activity and progression in individual patients remains a major challenge that requires a deep understanding of the key drivers of the disease and how they evolve over time
Proposed Approach / Solution
Data preprocessing and feature extraction from mobile and wearable devices was performed and integrated with other data from the patients. Interpretable statistical and machine learning methods were developed to determine the ability of derived features to distinguish people with MS from healthy controls, recognize MS disability and fatigue levels, and to quantify the effect of sleep quality.
Impact
The proposed analysis demonstrates a potential of using the collected physiological signals from wearables to monitor different aspects of multiple sclerosis status and progression.
Presentation
Gallery
Annexe
Cover image source: Getty Images
Additional resources
Bibliography
Publications
Related Pages
More projects
MAGNIFY
News
Latest news
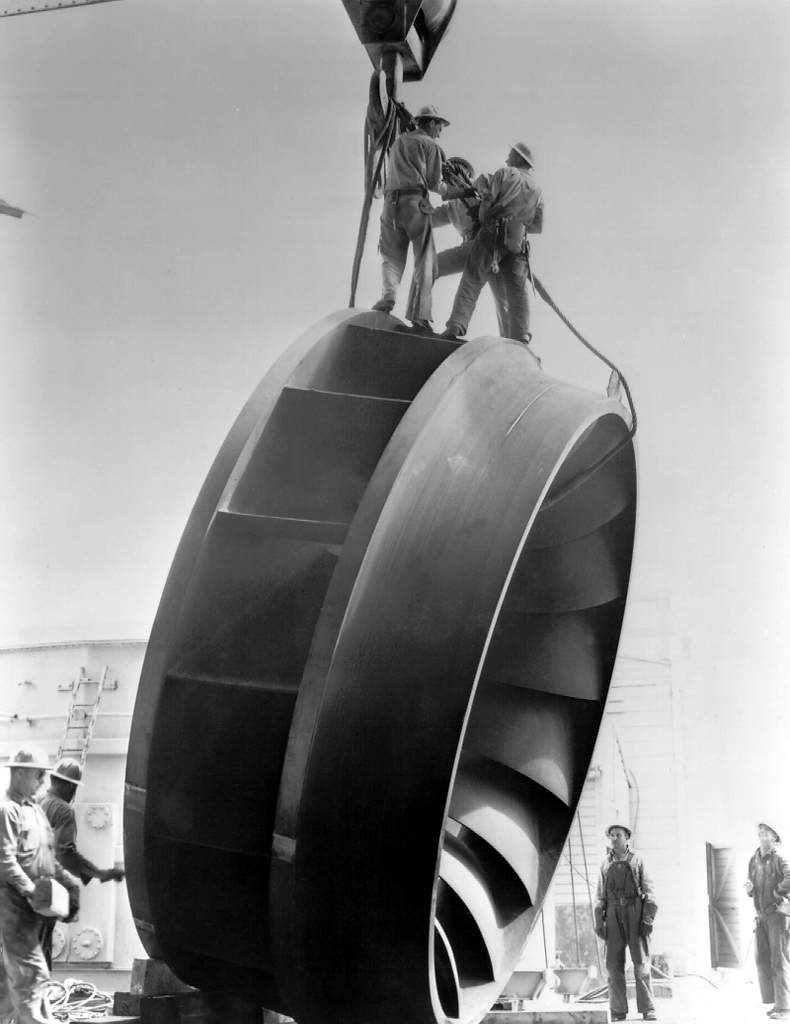
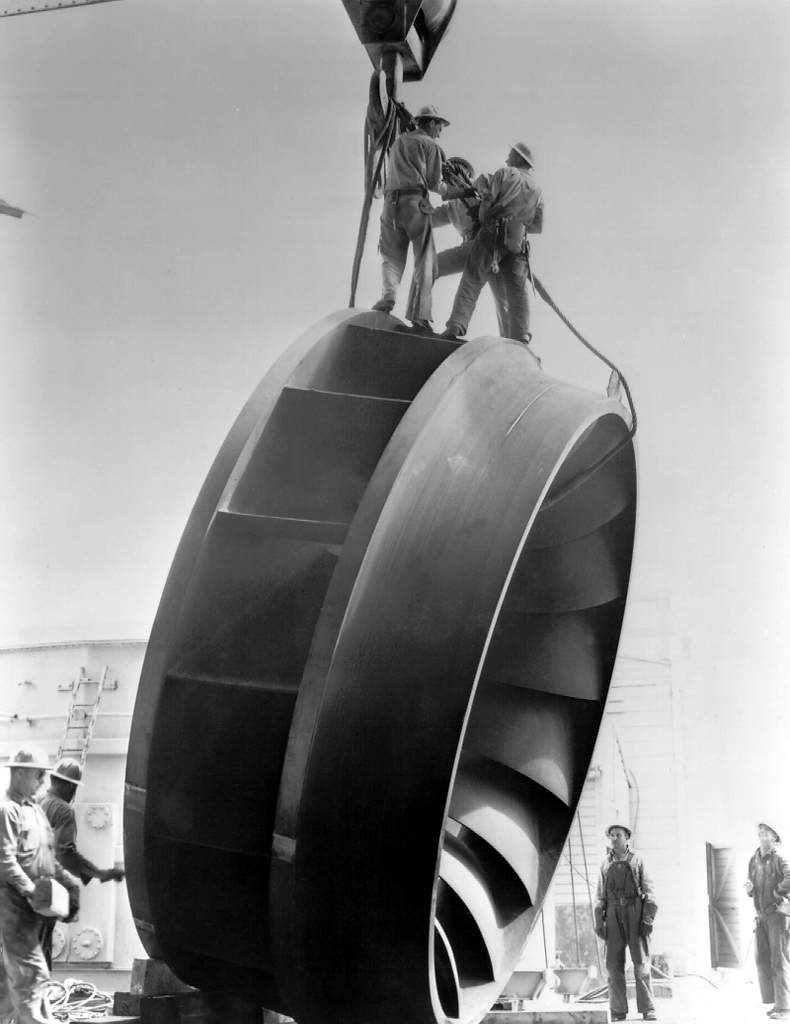
PAIRED-HYDRO | Increasing the Lifespan of Hydropower Turbines with Machine Learning
PAIRED-HYDRO | Increasing the Lifespan of Hydropower Turbines with Machine Learning


First National Calls: 50 selected projects to start in 2025
First National Calls: 50 selected projects to start in 2025
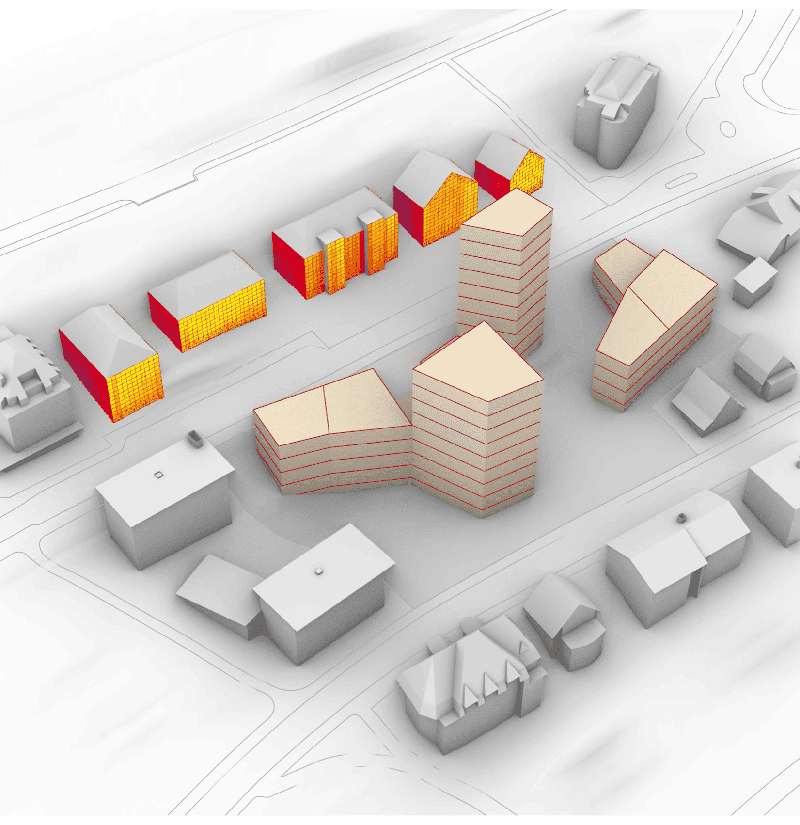
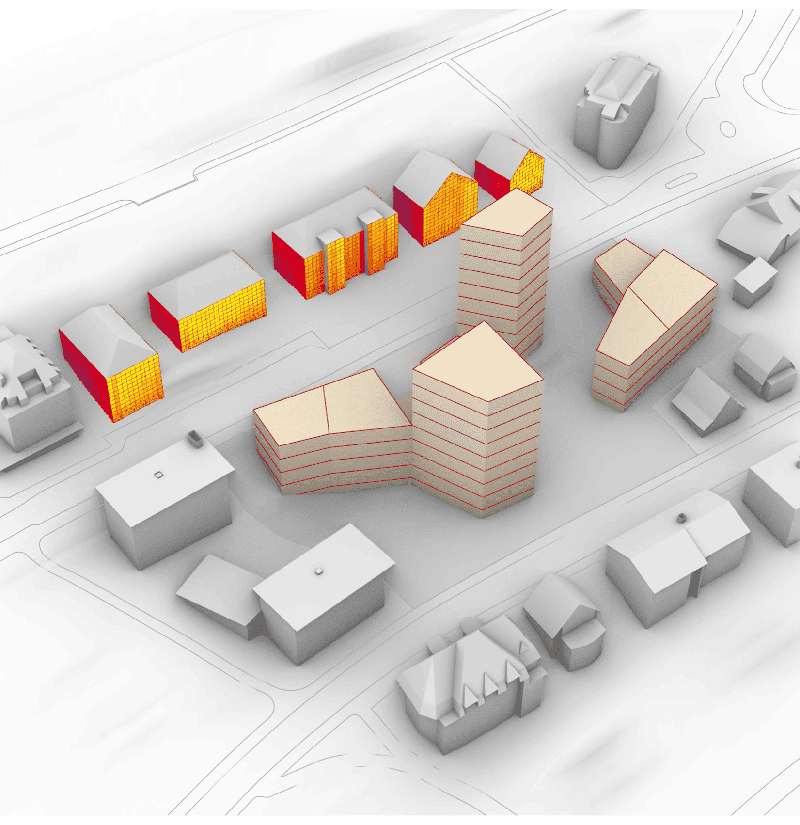
AIXD | Generative AI toolbox for architects and engineers
AIXD | Generative AI toolbox for architects and engineers
Contact us
Let’s talk Data Science
Do you need our services or expertise?
Contact us for your next Data Science project!