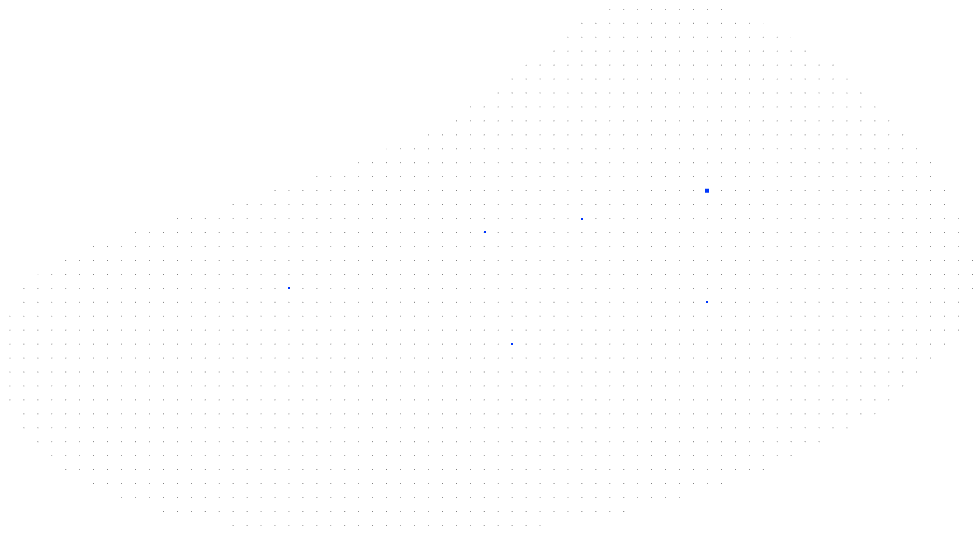
ease.ml
integrating AutoML into SDSC Eco-Systems
Started
January 5, 2019
Status
Share this project

Abstract
People
Collaborators
SDSC Team:
No items found.
PI | Partners:
Co-PIs:
- Prof. Dr. Ce Zhang, ETH Zürich
- Prof. Andreas Krause, ETH Zürich
description
Objectives:
- Integrate ease.ml into the SDSC eco-system (in particular, the RENKU platform) and provide model selection functionalities to a subset—mainly deep-learning based applications over images, text, and time series—of SDSC applications;
- Work closely with the SDSC to further advance the state of the art of AutoML, including overcoming a number of computer science challenges that mainly involve developing novel techniques for scalable Bayesian optimization;
- Pursue the emerging direction of how to automatically manage ML pipelines in a data-driven fashion.
Presentation
Download Presentation
Gallery
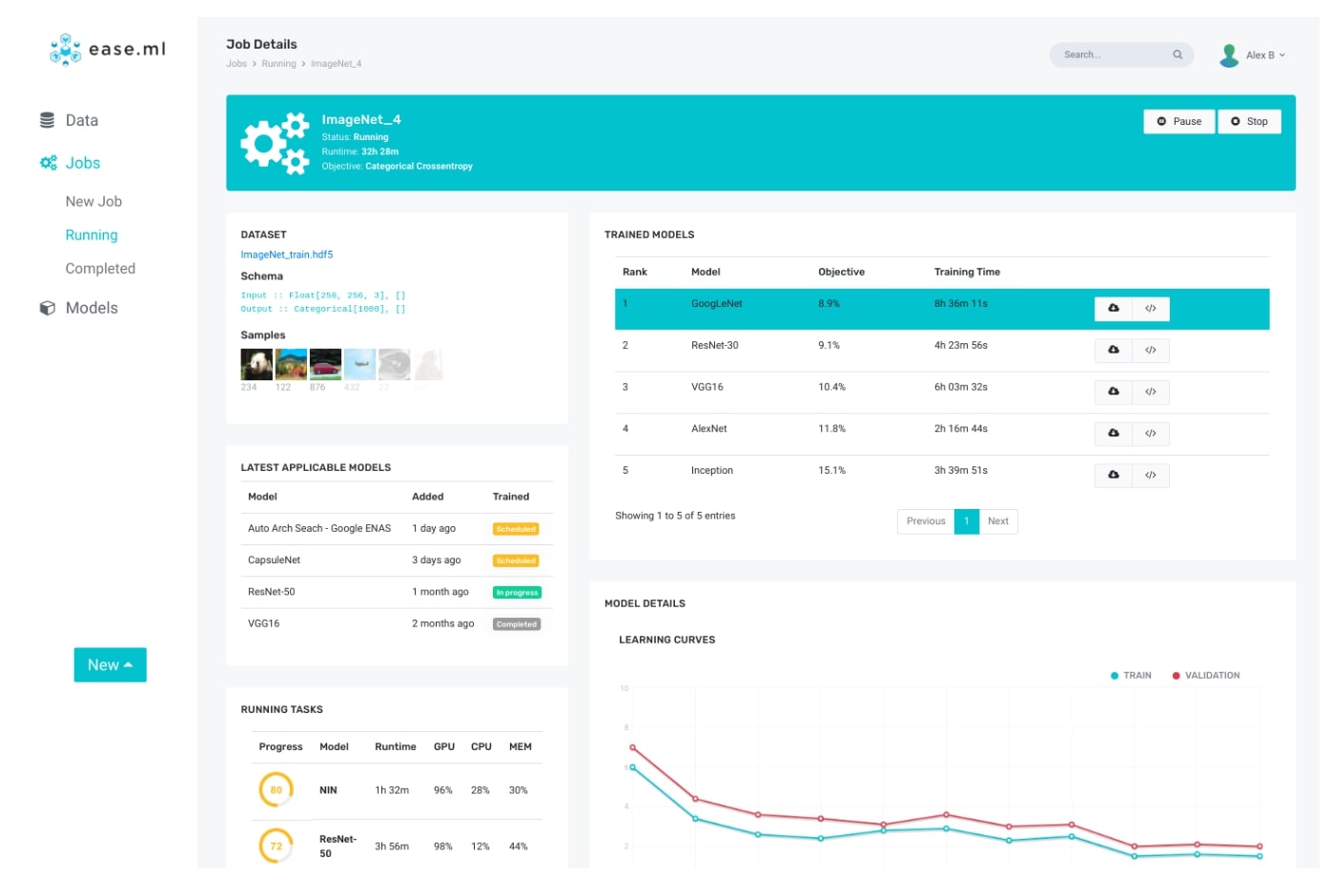
Annexe
Additional resources
Bibliography
Publications
Related Pages
More projects
STIMO
In Progress
Personalized epidural electrical stimulation of the lumbar spinal cord for clinically applicable therapy to restore mobility after paralyzing spinal cord injury
No items found.
News
Latest news


May 1, 2024
Smartair | An active learning algorithm for real-time acquisition and regression of flow field data
Smartair | An active learning algorithm for real-time acquisition and regression of flow field data
We’ve developed a smart solution for wind tunnel testing that learns as it works, providing accurate results faster. It provides an accurate mean flow field and turbulence field reconstruction while shortening the sampling time.
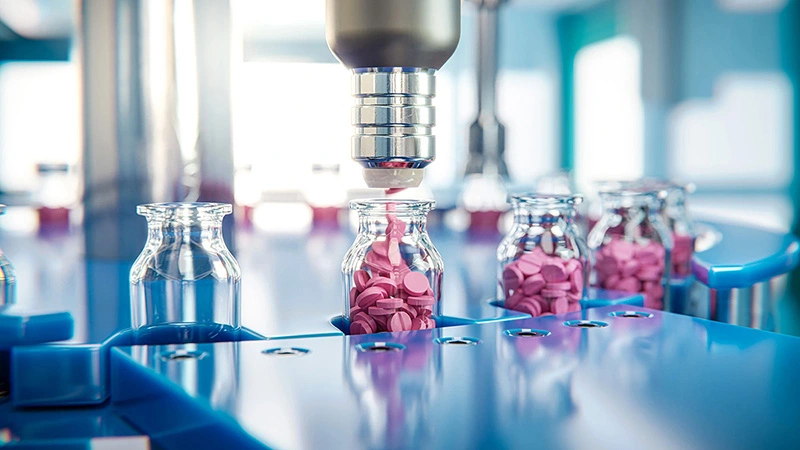
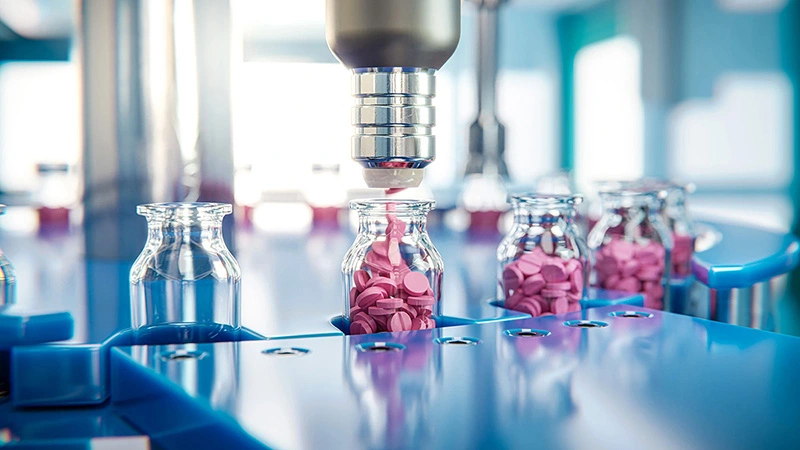
April 22, 2024
The Promise of AI in Pharmaceutical Manufacturing
The Promise of AI in Pharmaceutical Manufacturing
Innovation in pharmaceutical manufacturing raises key questions: How will AI change our operations? What does this mean for the skills of our workforce? How will it reshape our collaborative efforts? And crucially, how can we fully leverage these changes?


March 20, 2024
Efficient and scalable graph generation through iterative local expansion
Efficient and scalable graph generation through iterative local expansion
Have you ever considered the complexity of generating large-scale, intricate graphs akin to those that represent the vast relational structures of our world? Our research introduces a pioneering approach to graph generation that tackles the scalability and complexity of creating such expansive, real-world graphs.
Contact us
Let’s talk Data Science
Do you need our services or expertise?
Contact us for your next Data Science project!