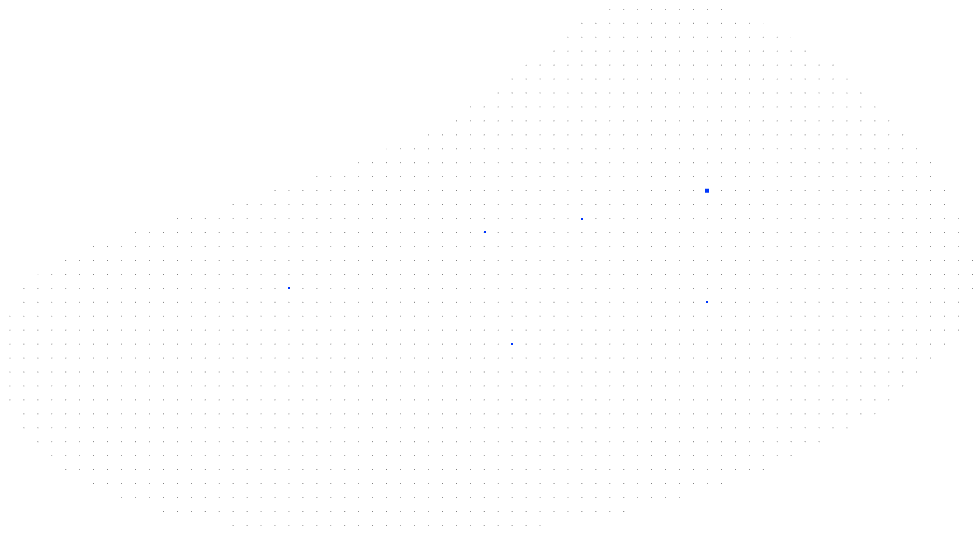
EAGLE
Enhanced understanding of Alpine mass movements Gathered through machine LEarning
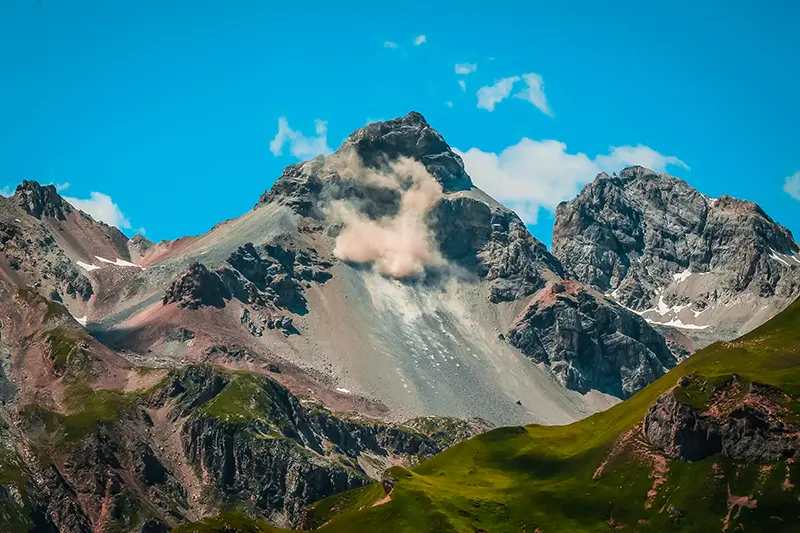
Abstract
Slow-moving mass movements, such as landslides and debris flows, pose serious hazards in alpine environments, threatening lives and infrastructure. Current detection methods rely heavily on manual analysis of Earth Observation (EO) data, limiting scalability and efficiency. The project EAGLE (Enhanced understanding of Alpine mass movements Gathered through machine LEarning) aims to leverage deep learning techniques to automate the detection and mapping of these geohazards using Interferometric Synthetic Aperture Radar (InSAR) data. We construct a high-quality dataset of manually annotated mass movements using Sentinel-1 wrapped interferograms from ascending and descending orbits. Advanced segmentation models—including U-Net, DeepLabv3, SegFormer, and residual shrinkage networks—are trained and evaluated against expert labels. Furthermore, we explore the integration of complementary EO sources, such as optical/multispectral imagery and DEM derivatives, that hopefully further enhances model performance. If successful, our approach promises more accurate, timely, and large-scale monitoring of alpine mass movements, contributing to improved hazard assessment, early warning systems, and climate-resilient infrastructure planning in mountainous environments worldwide.
People
Collaborators
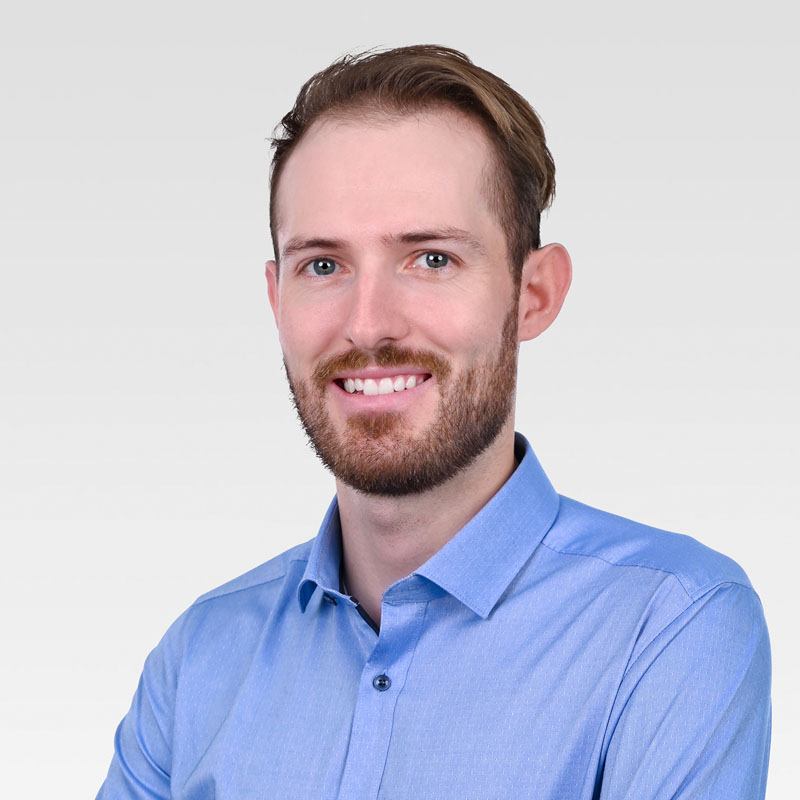
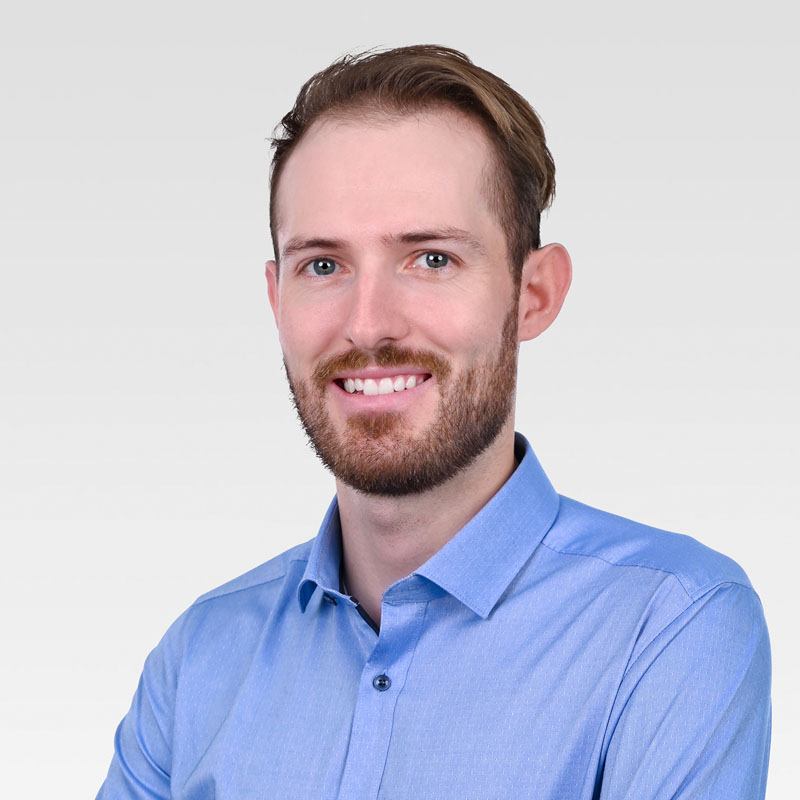
Before joining the SDSC as a Data Scientist in April 2023, Alessandro obtained his master’s degree in Computer Science with a focus on Machine Learning from ETH Zurich. In his master’s thesis, he worked on a joint project by the SDSC and the SLF Davos in which he automated the process of avalanche danger forecasting in the Swiss Alps using state-of-the-art machine learning techniques. In his free time, Alessandro loves alpine sports like ski-touring, climbing, and mountaineering.
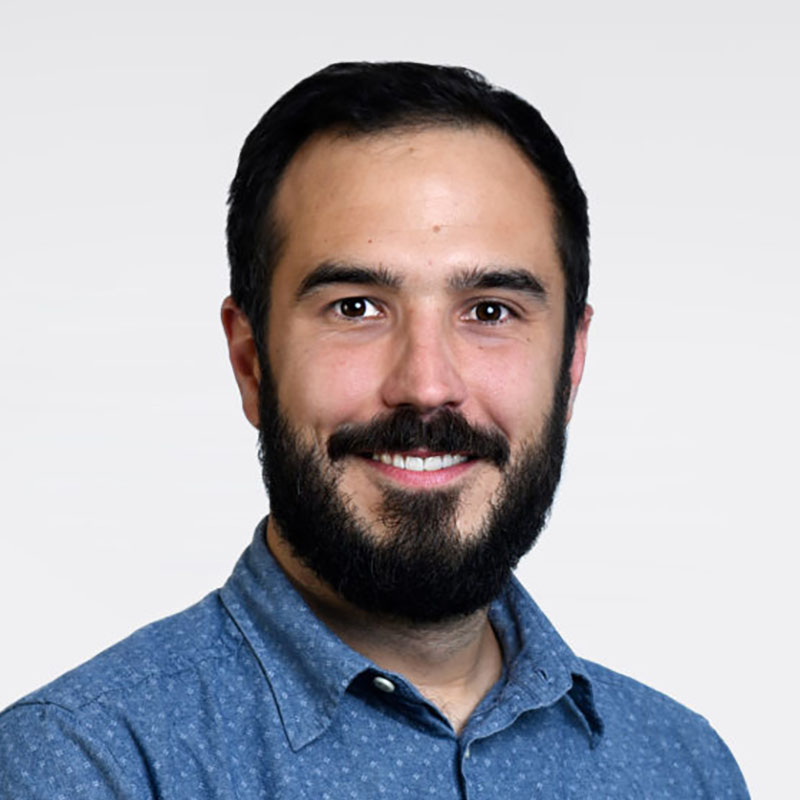
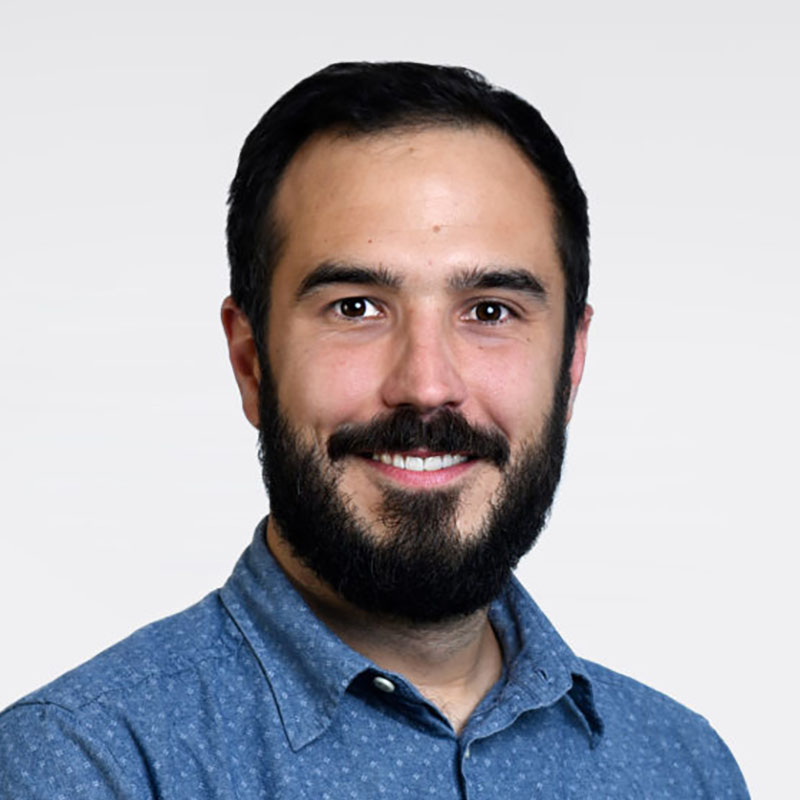
Michele received a Ph.D. in Environmental Sciences from the University of Lausanne (Switzerland) in 2013. He was then a visiting postdoc in the CALVIN group, Institute of Perception, Action and Behaviour of the School of Informatics at the University of Edinburgh, Scotland (2014-2016). He then joined the Multimodal Remote Sensing and the Geocomputation groups at the Geography department of the University of Zurich, Switzerland (2016-2017). His main research activities were at the interface of computer vision, machine and deep learning for the extraction of information from aerial photos, satellite optical images and geospatial data in general.
PI | Partners:
description
Motivation
Slow-moving mass movements pose a major concern in the Swiss Alps, since they can result in significant hazards such as landslides, rock avalanches, and debris flows, endangering both human lives and infrastructure. Currently, in Switzerland, the detection and monitoring of these processes heavily rely on manual methods, where experts analyse Earth Observation (EO) data or conduct field measurements. Large constellations of satellites are constantly circling the Earth, capturing and storing high-resolution EO data, leading to an exponentially growing archive of data. Thus, the core objective of our project is to harness the power of machine learning to unlock the potential of this rich repository of EO data. By developing innovative algorithms and techniques, we aim to automate the detection and monitoring processes, providing more accurate countrywide mass movement catalogues for the Swiss Alps.
Proposed Approach / Solution
We aim to develop deep learning-based approaches for mapping alpine landforms of interest using various Earth observation (EO) data, with a particular focus on Interferometric Synthetic Aperture Radar (InSAR) data (see Figure 1). While some models have demonstrated promising mapping capabilities using optical images (Prakash et al., 2021; Prakash et al., 2020) or stacked InSAR data (Liang et al., 2023), only a few have explored the direct mapping of mass movements from wrapped InSAR data (Bralet et al., 2024).

Our approach involves creating a comprehensive dataset of manually mapped mass movements using Sentinel-1 wrapped interferograms from both ascending and descending tracks, covering a range of temporal baselines in the Swiss Alps. This dataset is then used to train advanced segmentation models specifically designed for InSAR data, including U-Net, DeepLabv3, SegFormer, and deep residual shrinkage models. These models are evaluated against expert annotations on previously unseen imagery. Additionally, our work includes the integration of other EO data, such as optical imagery and digital elevation model derivatives. Finally, we are exploring novel techniques to classify the dynamics and activities associated with these processes.
Impact
Automating the mapping of slow-moving mass movements will significantly improve the accuracy, consistency, and timeliness of hazard detection in the Swiss Alps. Our approach enables the creation of large-scale, up-to-date catalogues, supporting early warning systems, infrastructure planning, and disaster response. This contributes to more effective risk management and increased resilience in alpine regions.
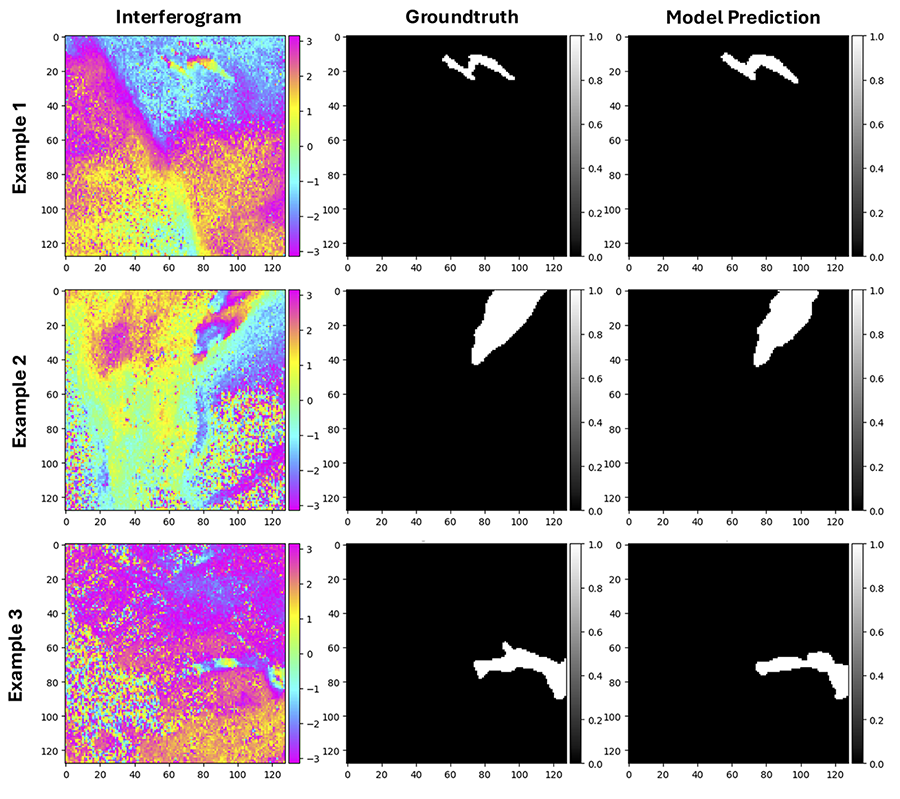
Presentation
Gallery
Annexe
Additional resources
Bibliography
- Prakash, N., Manconi, A., & Loew, S. (2021). A new strategy to map landslides with a generalized convolutional neural network. Scientific Reports, 11, 9722. https://doi.org/10.1038/s41598-021-89015-8
- Prakash, N., Manconi, A., & Loew, S. (2020). Mapping Landslides on EO Data: Performance of Deep Learning Models vs. Traditional Machine Learning Models. Remote Sensing, 12(3), 346. https://doi.org/10.3390/rs12030346
- Liang, Y., Zhang, Y., Li, Y., & Xiong, J. (2023). Automatic Identification for the Boundaries of InSAR Anomalous Deformation Areas Based on Semantic Segmentation Model. Remote Sensing, 15(21), 5262. https://doi.org/10.3390/rs15215262
- Bralet, A., Trouvé, E., Chanussot, J., & Atto, A. M. (2024). ISSLIDE: A New InSAR Dataset for Slow SLIding Area DEtection With Machine Learning. IEEE Geoscience and Remote Sensing Letters, 21, 1-5. https://doi.org/10.1109/LGRS.2024.3365299
Publications
More projects
MAGNIFY
News
Latest news


First National Calls: 50 selected projects to start in 2025
First National Calls: 50 selected projects to start in 2025
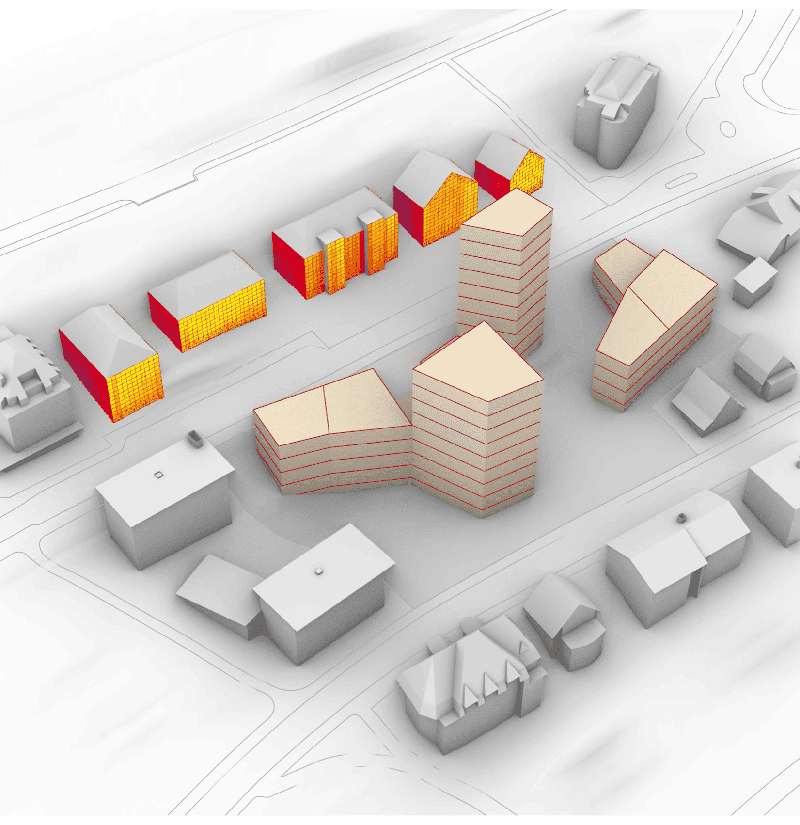
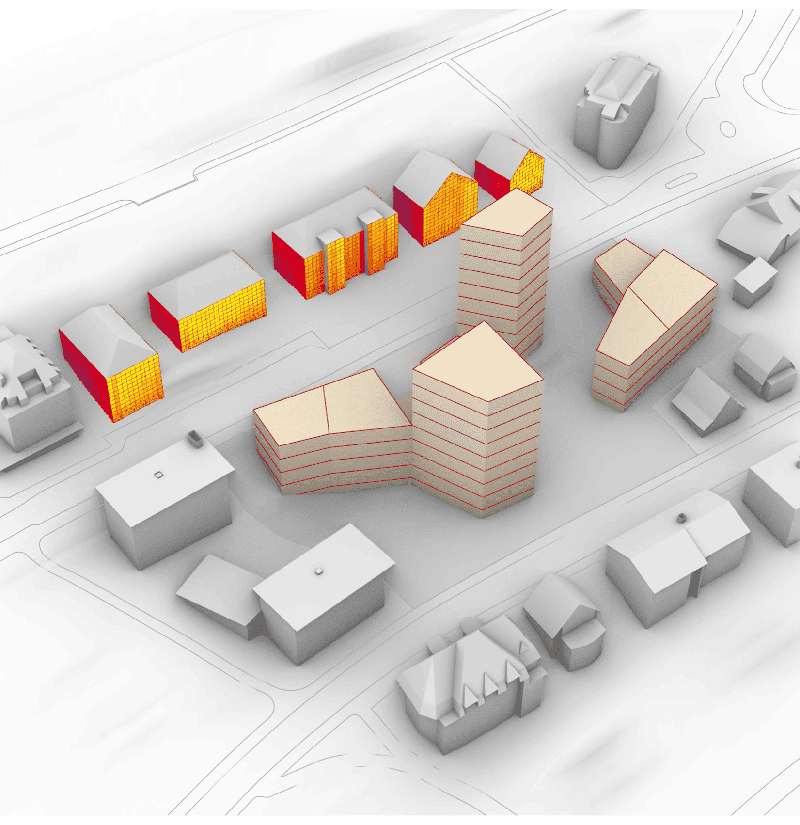
AIXD | Generative AI toolbox for architects and engineers
AIXD | Generative AI toolbox for architects and engineers


Smartair | An active learning algorithm for real-time acquisition and regression of flow field data
Smartair | An active learning algorithm for real-time acquisition and regression of flow field data
Contact us
Let’s talk Data Science
Do you need our services or expertise?
Contact us for your next Data Science project!