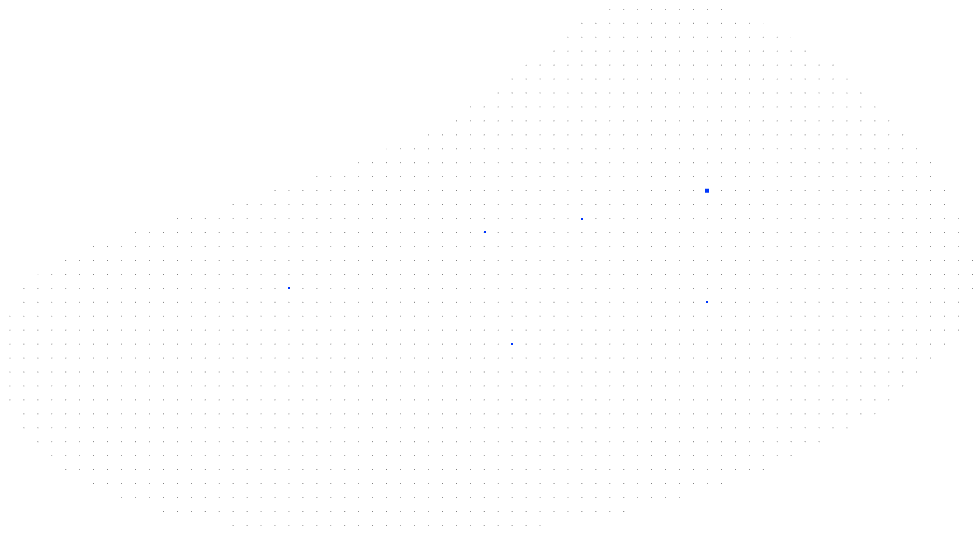
DrSCS
Predicting subclonal drug response from single-cell sequencing for precision oncology
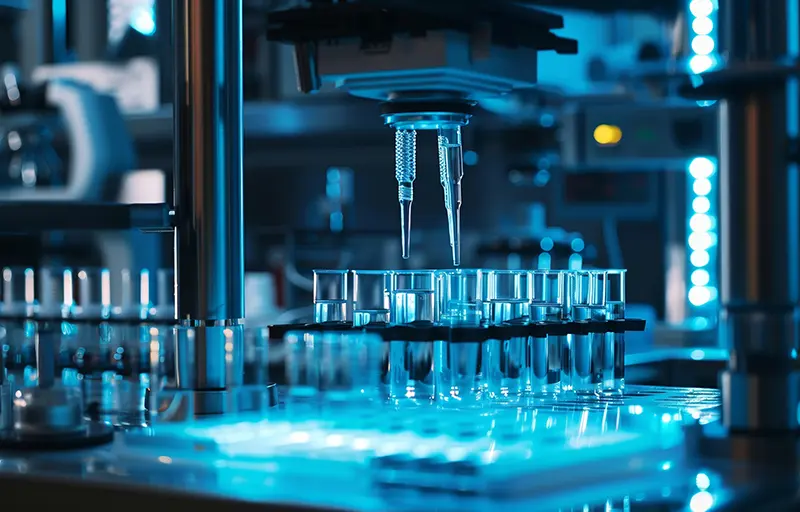
Abstract
Tumors evolve as heterogeneous populations of cells, which may be distinguished by different genomic aberrations and transcriptomic differences. The resulting intra-tumor heterogeneity plays an important role in cancer patient relapse and treatment failure, so that obtaining a clear understanding of each patient's tumor composition and evolutionary history is key for personalised therapies. Single-cell DNA sequencing now provides the possibility to resolve tumor heterogeneity at the highest resolution of individual tumor cells, while single-cell RNA sequencing offers expression profiling of tumor subpopulations as well as characterisation of the immune compartment of the tumor microenvironment. In parallel, ex vivo drug screening gives a direct measurement of the aggregate response of tumor cells to various compounds.
With 136 samples profiled in a recent collaboration, we developed and incorporated new prediction models for the drug response of the individual subclones in each patient's tumor. Our approach account for the tumor heterogeneity and for potentially different responses of constituent tumor parts to treatment. To integrate the different data modalities, we first integrate the single-cell DNA and RNA information with joint modelling of the tumor evolution to more accurately understand its composition in terms of the existing subpopulations and their expression and copy number profiles. By additionally integrating drug response information, we can, for the first time, build and test drug prediction models at the level of tumor subclones. The potential of understanding the effect of different treatments on the heterogeneous parts of a tumor and thereby designing treatments for the full composite tumor could have great impact in aiding precision oncology.
This project is co-funded by PHRT.
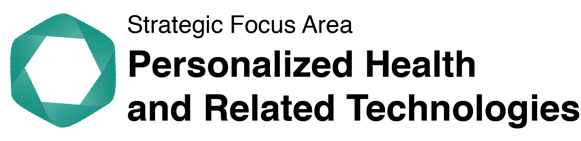
People
Collaborators


Quentin graduated with an engineering degree in mathematics and computer science from École des Ponts ParisTech in 2019. After a 6-month experience at the Center for Data Science of the New York University working on applied Machine Learning for medical imaging, he did a PhD in Statistics at Gustave Eiffel University (Paris). During his PhD, Quentin worked on random graphs and selective inference. His recent cross-disciplinary collaborations involve applications in biology and hydrology.
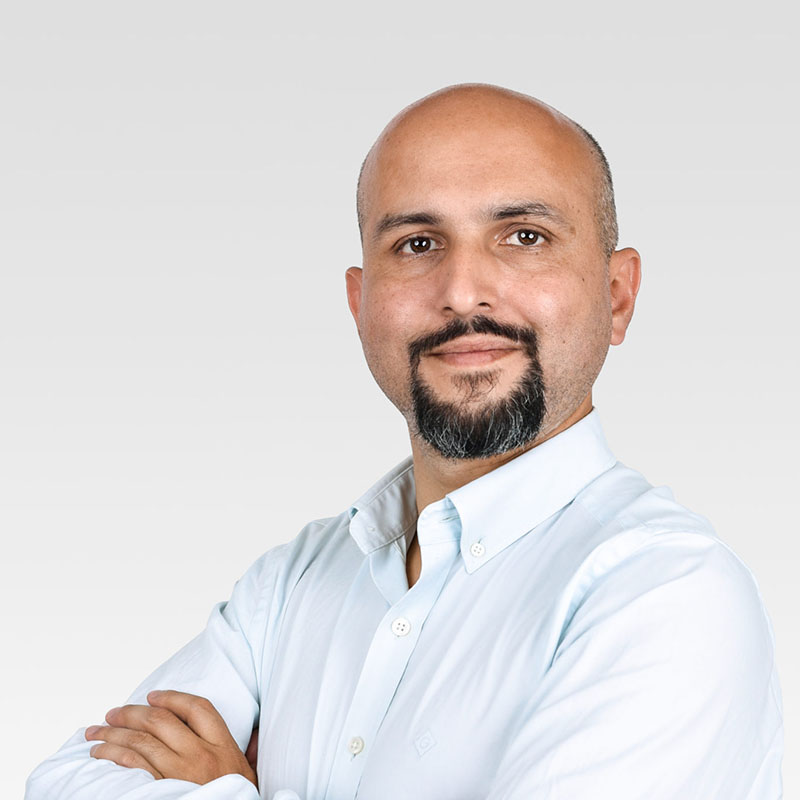
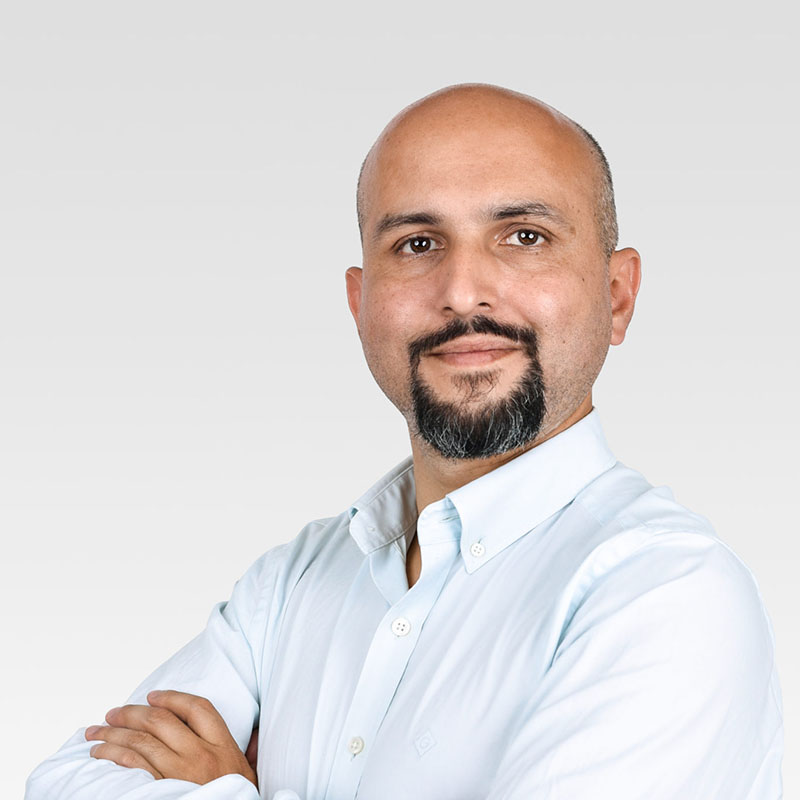
Daniel worked as a postdoctoral researcher on critical event prediction for the University Hospital in Zurich. In addition, Daniel has worked as a postdoctoral researcher in Lausanne, delivering algorithms for Bayesian inference in big panel data. Previously in Paris, he developed models for automated scientific discovery. He obtained a Ph.D. from the University of Edinburgh, funded by a Microsoft Research scholarship. His interest relates primarily to attacking applied biomedicine problems from different angles, frequentist statistics, Bayesian statistics, and Machine Learning.
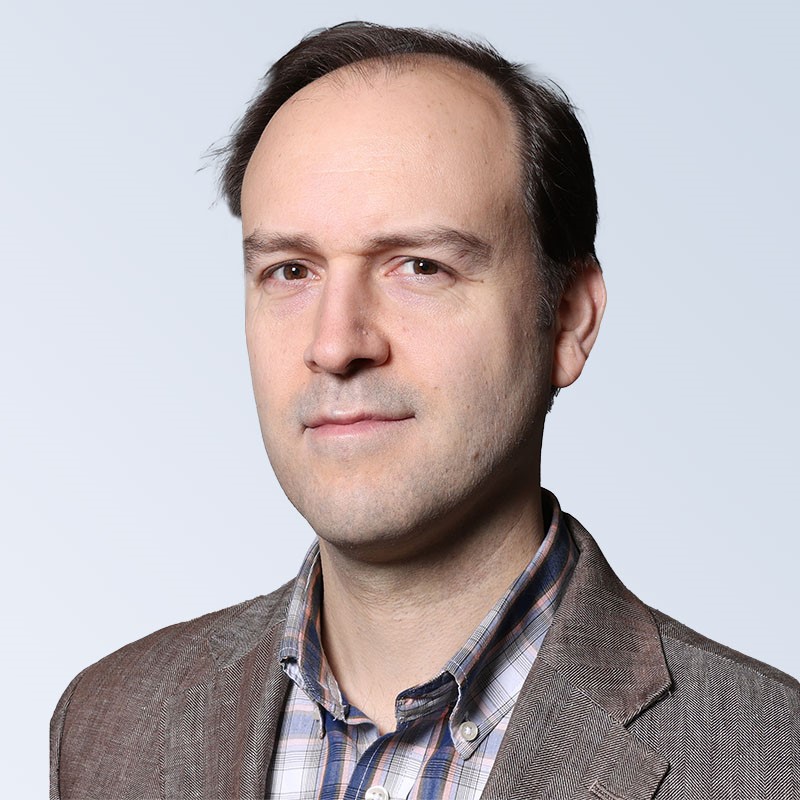
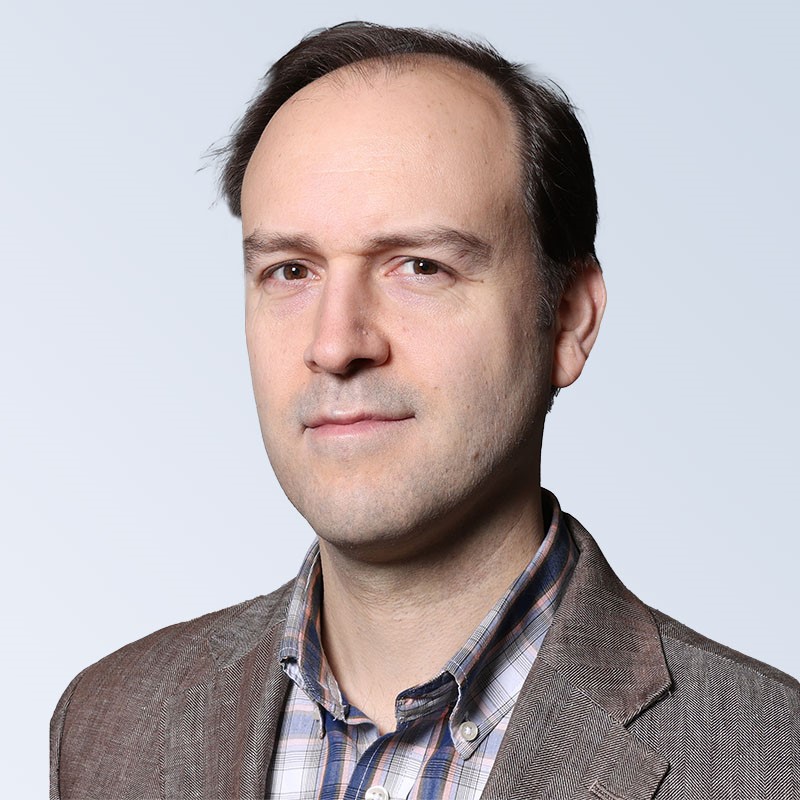
Guillaume Obozinski graduated with a PhD in Statistics from UC Berkeley in 2009. He did his postdoc and held until 2012 a researcher position in the Willow and Sierra teams at INRIA and Ecole Normale Supérieure in Paris. He was then Research Faculty at Ecole des Ponts ParisTech until 2018. Guillaume has broad interests in statistics and machine learning and worked over time on sparse modeling, optimization for large scale learning, graphical models, relational learning and semantic embeddings, with applications in various domains from computational biology to computer vision.
description
Motivation
During tumor progression, cancers may evolve into a complex system of heterogeneous subpopulations of cells harbouring different genomic aberration. Diversity and heterogeneity within a tumor is a considerable cause of treatment failure and relapse, since subclonal cell populations may be resistant to treatment and therefore progress. Effective treatment should target all subpopulations, or evolve with the tumor to adapt as new clones become dominant. Uncovering the genetic makeup and evolutionary history of each tumor and linking these molecular alterations to the chances of reatment success is thus key to developing targeted therapies.
Proposed Approach / Solution
We introduce scClone2DR, a novel method that leverages single-cell DNA and RNA sequencing data alongside ex vivo drug screening results. Our approach aims to predict drug impact at the subclonal level, thereby accounting for the diverse cellular populations within tumors. scClone2DR operates as a generative probabilistic model, incorporating information from both single-cell DNA and RNA sequencing datasets to accurately model tumor heterogeneity. By considering subclone proportions and cell counts in drug screening wells, our method offers a comprehensive understanding of the tumor response to different compounds. We perform extensive validation using simulated and real world data as obtained from the Tumor Profiler Consortium.
Impact
The impact of our work is at least threefold:
- Clinical impact: scClone2DR equips practitioners with tools to prescribe personalized treatments that effectively target all tumor subpopulations within a patient. Unlike existing approaches that often only reduce the size of the largest tumor clones, scClone2DR ensures that even small, drug-resistant subclones are accounted for—helping to prevent long-term relapse.
- Research impact: The interpretability of scClone2DR enables oncology researchers to explore the drug–gene associations identified by the model. This not only helps validate the model by comparing its predictions with existing literature, but also opens opportunities to uncover novel mechanisms of drug action.
- Experimental impact: Thanks to its generative nature, scClone2DR makes it possible to evaluate how many replicates per drug are needed in pharmascopy data to reliably assess model performance using only observed data. Our work, particularly on simulated datasets, has shown that scClone2DR can accurately estimate parameters—but may struggle to confidently assess performance if the number of replicates is too low, due to inherent noise in pharmascopy measurements.

Presentation
Gallery
Annexe
Additional resources
Bibliography
- Kuipers, J., Tuncel, M. A., Ferreira, P., Jahn, K., & Beerenwinkel, N. (2020). Single-cell copy number calling and event history reconstruction. doi:10.1101/2020.04.28.065755
- Ferreira, P. F., Kuipers, J., & Beerenwinkel, N. (2021). Mapping single-cell transcriptomes to copy number evolutionary trees. doi:10.1101/2021.11.04.467244
- Bertolini, A., Prummer, M., Tuncel, M. A., Menzel, U., Rosano-González, M. L., Kuipers, J., … Singer, F. (2022). scAmpi-A versatile pipeline for single-cell RNA-seq analysis from basics to clinics. PLoS Computational Biology, 18(6), e1010097. doi:10.1371/journal.pcbi.1010097
- Irmisch, A., Bonilla, X., Chevrier, S., Lehmann, K.-V., Singer, F., Toussaint, N. C., … Levesque, M. P. (2021). The Tumor Profiler Study: integrated, multi-omic, functional tumor profiling for clinical decision support. Cancer Cell, 39(3), 288–293. doi:10.1016/j.ccell.2021.01.004
Publications
Related Pages
More projects
MAGNIFY
News
Latest news


First National Calls: 50 selected projects to start in 2025
First National Calls: 50 selected projects to start in 2025
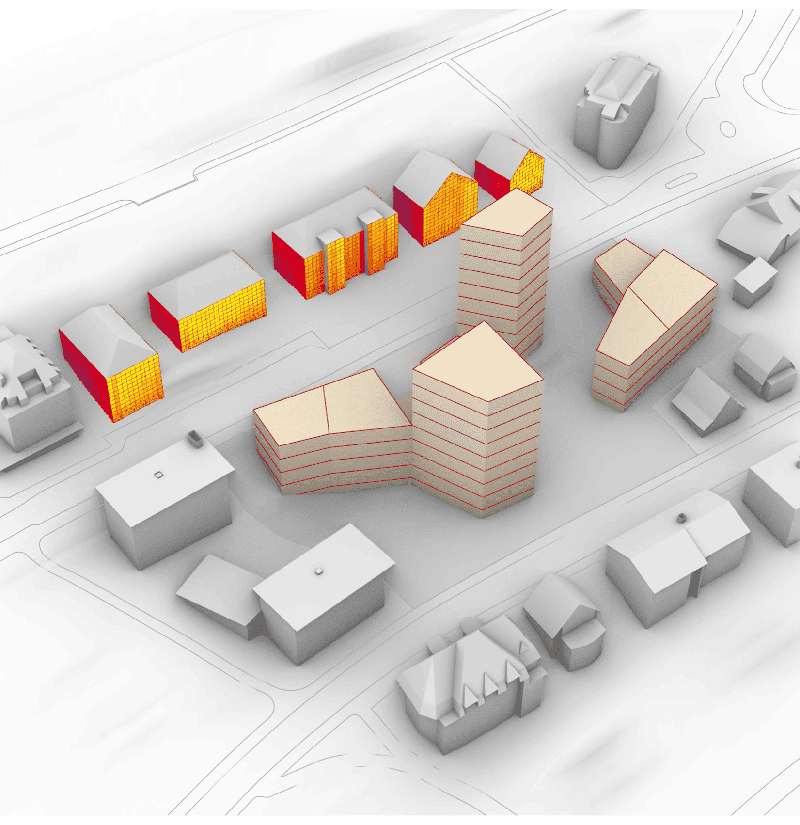
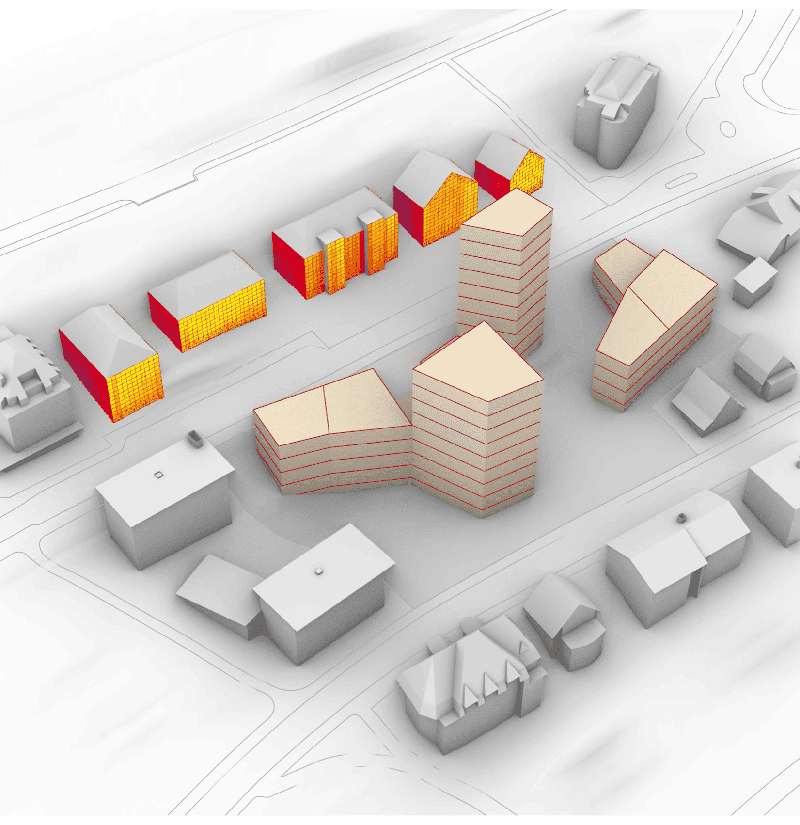
AIXD | Generative AI toolbox for architects and engineers
AIXD | Generative AI toolbox for architects and engineers


Smartair | An active learning algorithm for real-time acquisition and regression of flow field data
Smartair | An active learning algorithm for real-time acquisition and regression of flow field data
Contact us
Let’s talk Data Science
Do you need our services or expertise?
Contact us for your next Data Science project!