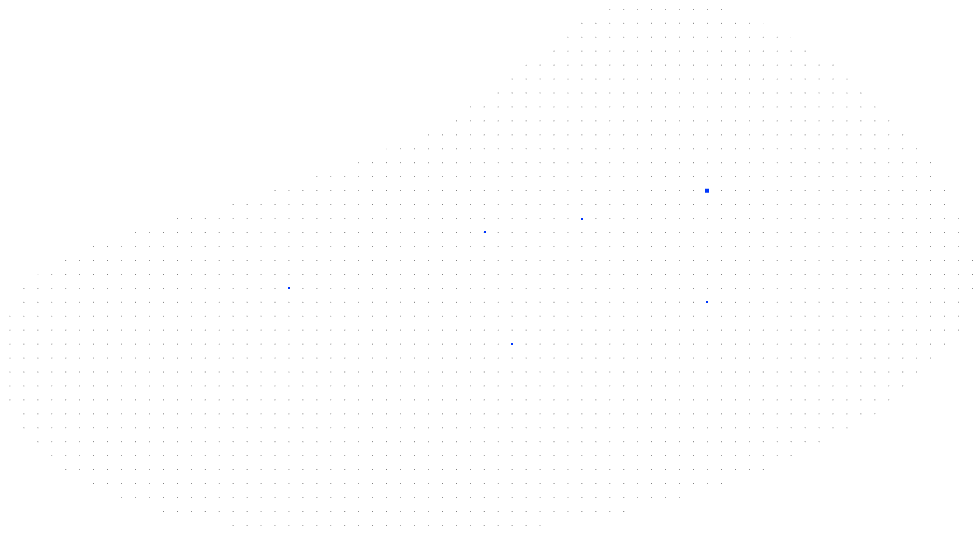
COPE
COnstraints on near-term warming ProjEctions via distributionally robust statistical and machine learning

Abstract
Climate model projections of 21st century warming are uncertain and some climate models predict very high warming rates over the next few decades. Recent research has attempted to narrow the uncertainty range of future warming based on historical temperature trends. However, key climate system uncertainties limit our confidence in such observations-based constraints: (i) the magnitude and phase of multidecadal internal variability, (ii) anthropogenic and volcanic aerosol forcing, and (iii) potential model biases in representing the climatological base state, the strength of pattern effects, unforced variability or forced response patterns.
Here, we propose to develop robust constraints on near-future projections of climate warming via integrating climate model simulations with observations using distributionally robust statistical and machine learning (StatML) methods. Previous work within the SDSC-funded DASH has led to an improved understanding and attribution of the past climate record, from identifying historical forced vs. internal climate variability, separation of specific forced responses, and towards employing the concept of distributional robustness to multidecadal variability, thus reducing climate model spread.
We propose to leverage these developments for constraining near-term future warming projections, and projections of future temperature and precipitation at the regional scale via dynamical adjustment. A key challenge is to incorporate the concept of distributional robustness in climate science, with the potential to achieve robustness towards model structural uncertainty that will facilitate the transfer across climate models and towards observations.
Overall, the project will narrow uncertainties in near-term future climate, and advance methods using distributional robustness that are likely to transfer to many fields within Earth system science where imperfect process-based models are used and combined with observational data.
People
Collaborators


Victor has joined the SDSC in 2020 to design solutions for data-driven optimization problems. His research interests lie at the crossroad of machine learning and decision-making. This contains several topics such as stochastic optimization, reinforcement learning, combinatorial optimization, and probabilistic graphical models. Victor received a PhD in operations research and machine learning from Ecole des Ponts Paristech in 2020. Before that, he completed a master degree in Operation Research and Machine learning at Ecole des Ponts Paristech and a bachelor degree in Applied Mathematics and Computer Sciences.
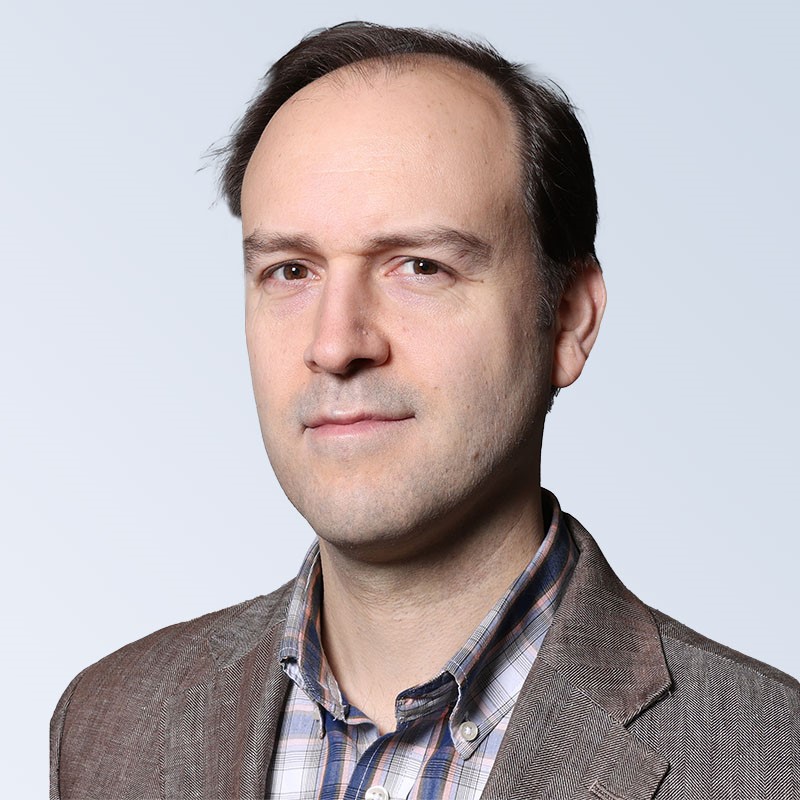
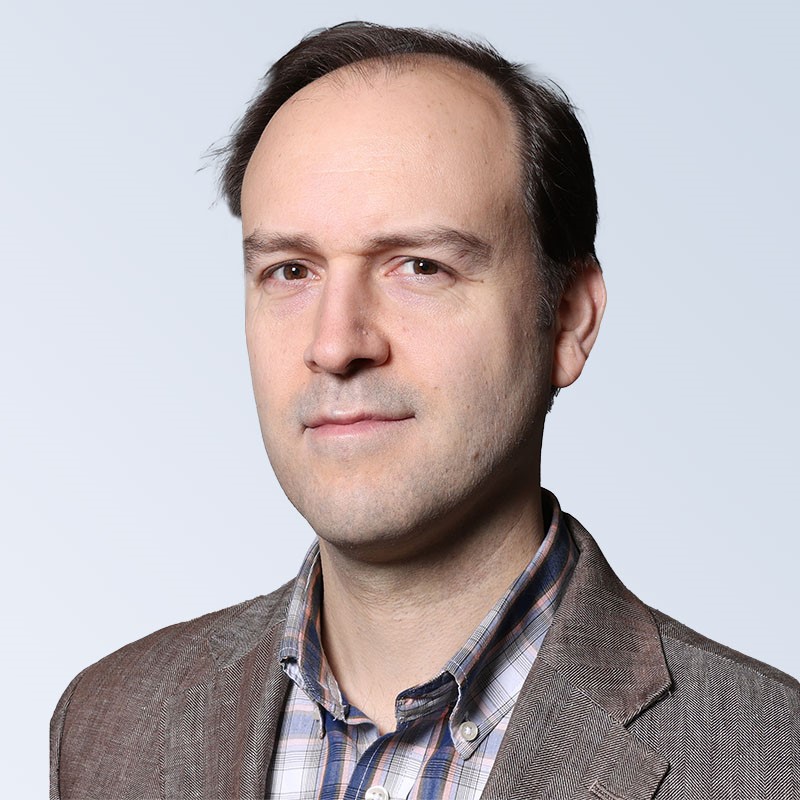
Guillaume Obozinski graduated with a PhD in Statistics from UC Berkeley in 2009. He did his postdoc and held until 2012 a researcher position in the Willow and Sierra teams at INRIA and Ecole Normale Supérieure in Paris. He was then Research Faculty at Ecole des Ponts ParisTech until 2018. Guillaume has broad interests in statistics and machine learning and worked over time on sparse modeling, optimization for large scale learning, graphical models, relational learning and semantic embeddings, with applications in various domains from computational biology to computer vision.
description
Motivation
Existing climate models provide good insights for understanding temperature forecasting. Since they are based on different atmospheric assumptions, they provide different predictions. Leveraging the variety of climate models, the project aims at building machine learning models that are robust towards the uncertainty of the models. This raises the question of defining the statistical robustness in such a context.
Proposed Approach / Solution
SDSC role is to mathematically formulate this key idea of statistical robustness towards model uncertainty. The current work focuses on developing linear models with robust model weights. These linear models take anomalies as input (time series of maps) and return the spatial forced response (time series of maps). The goal is to learn a worst-case combination of weights assigned to climates models, which leads to robust prediction of forced response using the linear coefficients (see fingerprint in Figure 1). We evaluate the learned model by training on all except one climate models and then testing it on the remaining one. The evaluation shows that we can decrease the worst-case error.
Impact
The project will develop robust statistical models for the near future warming predictions at global and regional scales, with careful consideration of uncertainties, based on an improved understanding of the historical climate record. The project’s impact will be highly relevant for near-term climate policy, and for climate impact studies because of refined warming estimates at the regional scale.
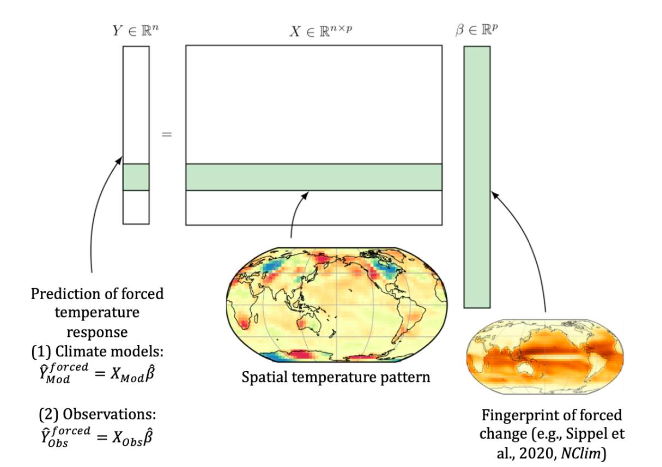
Presentation
Gallery
Annexe
Cover image source: Adobe Stock
Additional resources
Bibliography
- Sippel, S., Meinshausen, N., Székely, E., Fischer, E., Pendergrass, A.G., Lehner, F. and Knutti, R., 2021b. Robust detection of forced warming in the presence of potentially large climate variability. Science advances, 7(43): eabh4429.
- Székely, E., Sippel, S., Knutti, R., Obozinski, G. and Meinshausen, N., 2019. A direct approach to detection and attribution of climate change. arXiv preprint arXiv:1910.03346.
- Székely, E., Sippel, S., Meinshausen, N., Obozinski, G. and Knutti, R., 2022. Robustness constraints for detection and attribution of climate change under uncertainty. in preparation.
- Sippel, S., Meinshausen, N., Székely, E., Fischer, E., Pendergrass, A., Lehner, F. and Knutti, R., 2021a. Robust detection of forced warming in the presence of potentially large climatevariability. Science Advances, In Review.
- Sippel, S., Fischer, E.M., Scherrer, S.C., Meinshausen, N. and Knutti, R., 2020a. Late 1980s abrupt cold season temperature change in Europe consistent with circulation variability and long-term warming. Environmental Research Letters, 15(9): 094056.
- Sippel, S., Meinshausen, N., Fischer, E.M., Székely, E. and Knutti, R., 2020b. Climate change now detectable from any single day of weather at global scale. Nature climate change, 10(1): 35-41.
Publications
Related Pages
More projects
MAGNIFY
News
Latest news
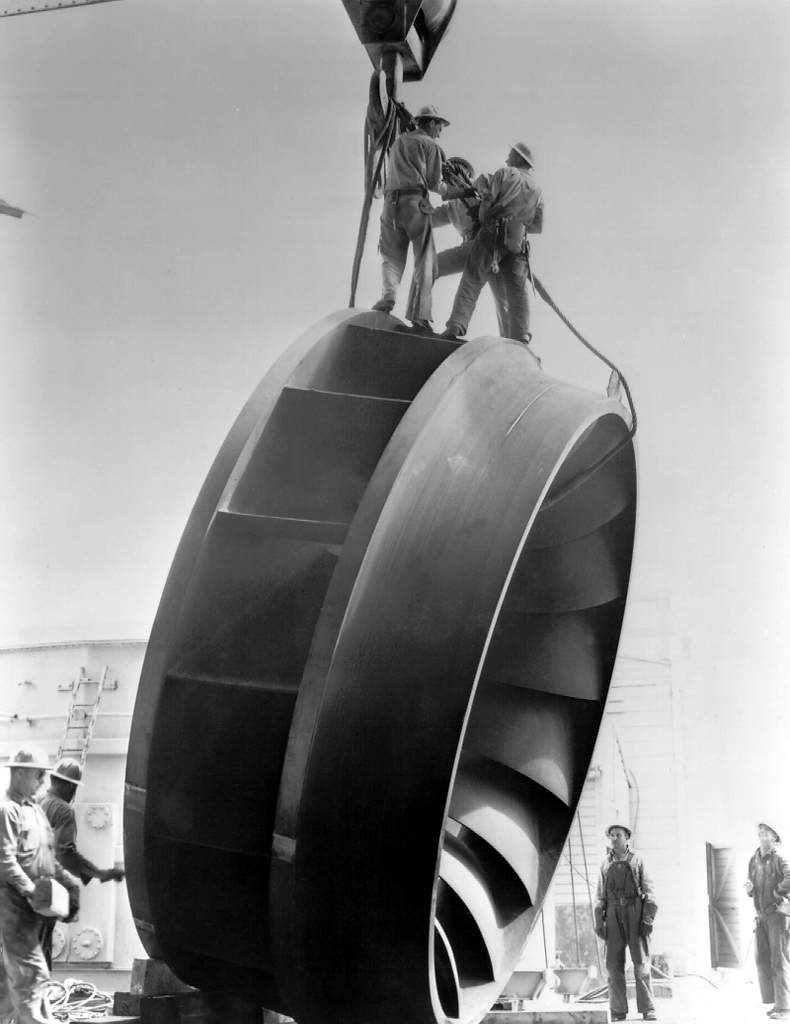
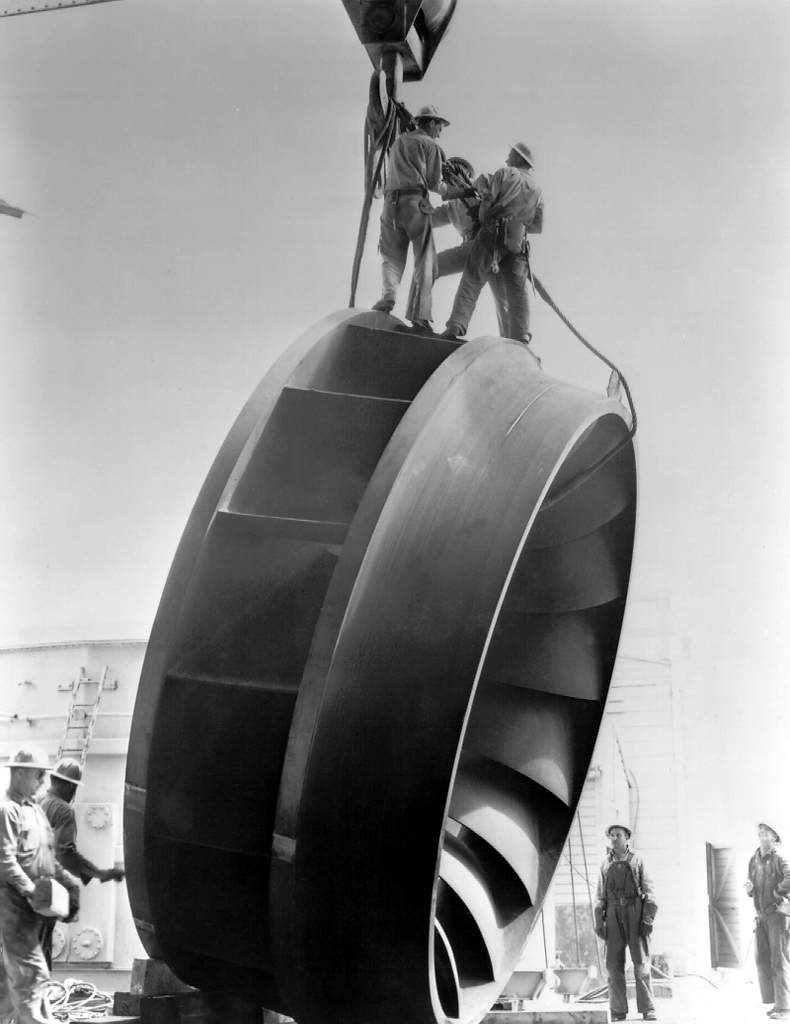
PAIRED-HYDRO | Increasing the Lifespan of Hydropower Turbines with Machine Learning
PAIRED-HYDRO | Increasing the Lifespan of Hydropower Turbines with Machine Learning


First National Calls: 50 selected projects to start in 2025
First National Calls: 50 selected projects to start in 2025
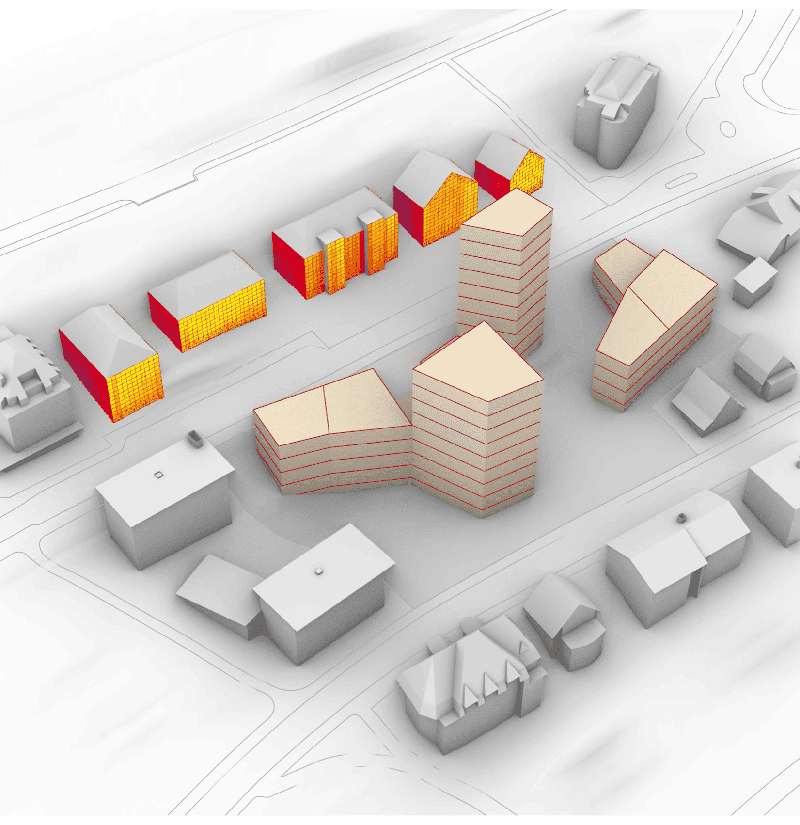
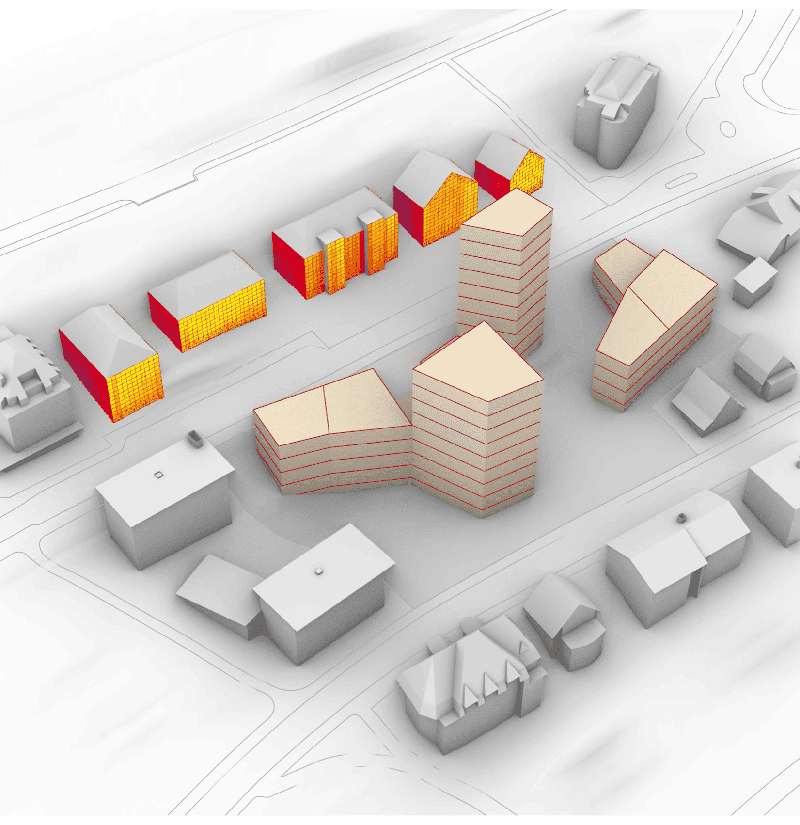
AIXD | Generative AI toolbox for architects and engineers
AIXD | Generative AI toolbox for architects and engineers
Contact us
Let’s talk Data Science
Do you need our services or expertise?
Contact us for your next Data Science project!