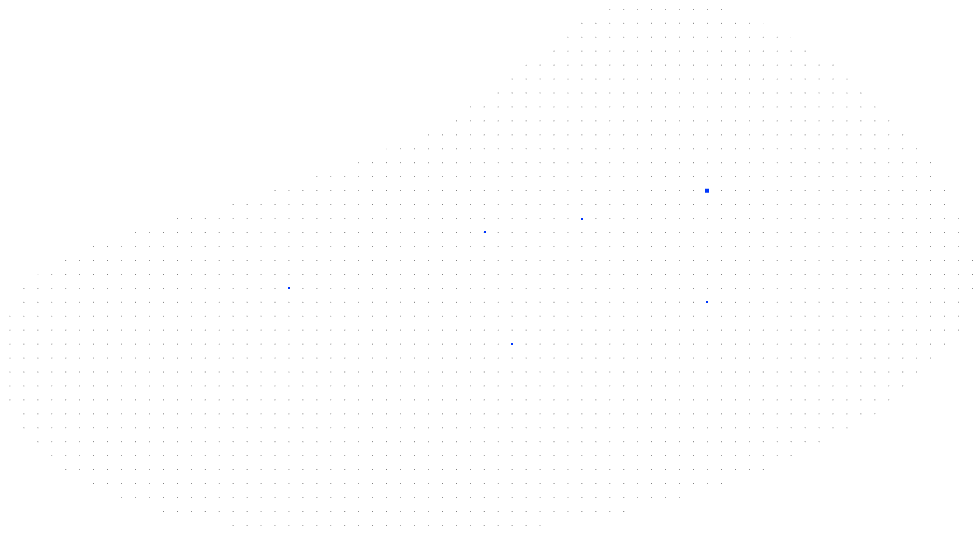
Citizen-Controlled
Citizen-controlled Data Science for Multiple Sclerosis Research

Abstract
Multiple Sclerosis (MS) is a complex chronic disease whose manifestation depends on clinical, environmental and individual factors and for which prediction of individual progression is poor and often treatment decisions are hampered by the lack of objective parameters (e.g., related to fatigue).
MS data was employed as the use case within the MIDATA project which aims at developing an ethically fair and secure data infrastructure that permits collection, integration and analysis of diverse types of data under the control of the citizen/patient.
The task of SDSC within this project was to extract data from the doctor's reports collected and stored with the hospital software kisim. A doctor's report is a semi-structured text of a few to several dozen lines where each line is associated with a topic such as diagnosis, current state, history, MRI or medication. The neurology clinic at the university hospital of Zurich USZ has developed and is maintaining the database seantis to store MS patients records in a structured manner. So far, the seantis database has been filled manually by transcribing information from the doctor's reports to the corresponding fields.
People
Collaborators
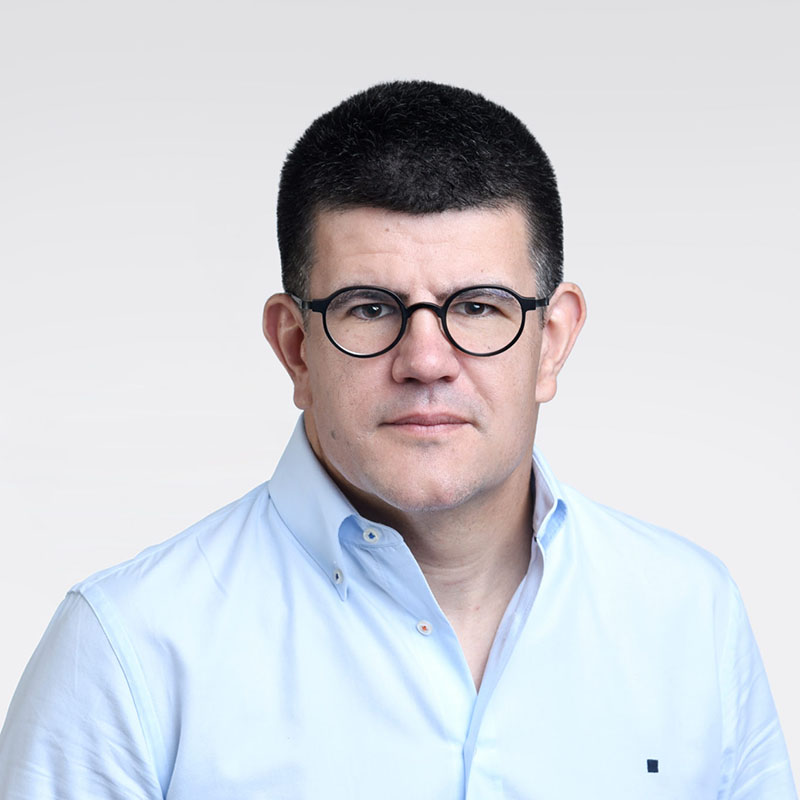
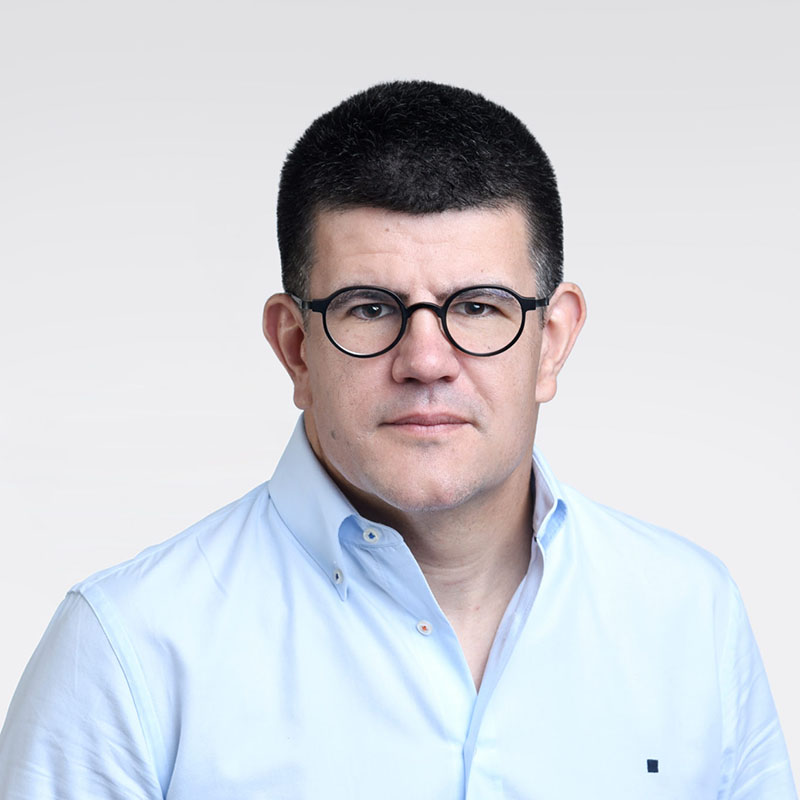
Fernando Perez-Cruz received a PhD. in Electrical Engineering from the Technical University of Madrid. He is Titular Professor in the Computer Science Department at ETH Zurich and Head of Machine Learning Research and AI at Spiden. He has been a member of the technical staff at Bell Labs and a Machine Learning Research Scientist at Amazon. Fernando has been a visiting professor at Princeton University under a Marie Curie Fellowship and an associate professor at University Carlos III in Madrid. He held positions at the Gatsby Unit (London), Max Planck Institute for Biological Cybernetics (Tuebingen), and BioWulf Technologies (New York). Fernando Perez-Cruz has served as Chief Data Scientist at the SDSC from 2018 to 2023, and Deputy Executive Director of the SDSC from 2022 to 2023
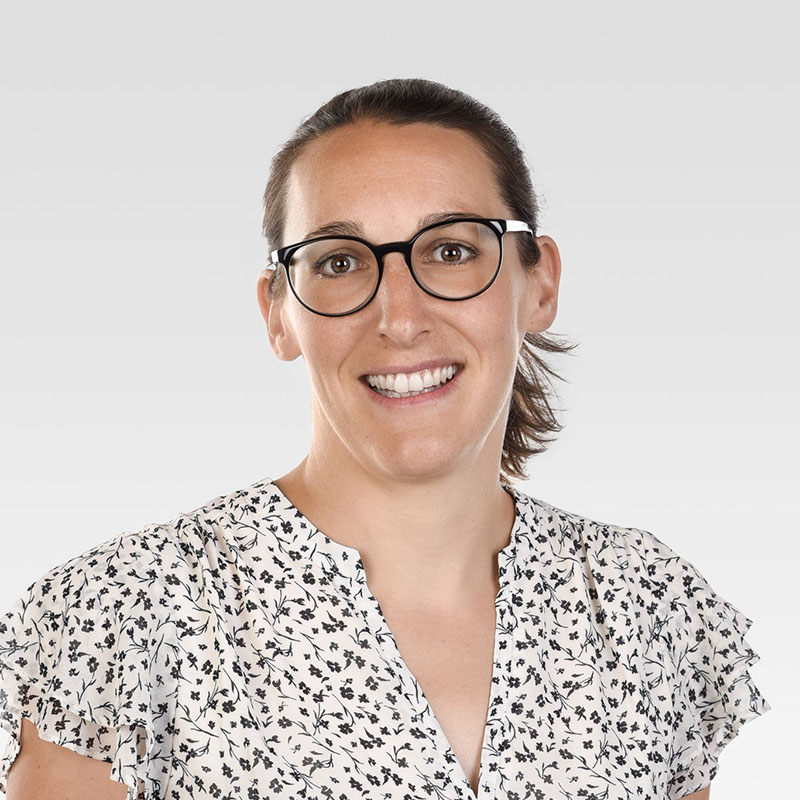
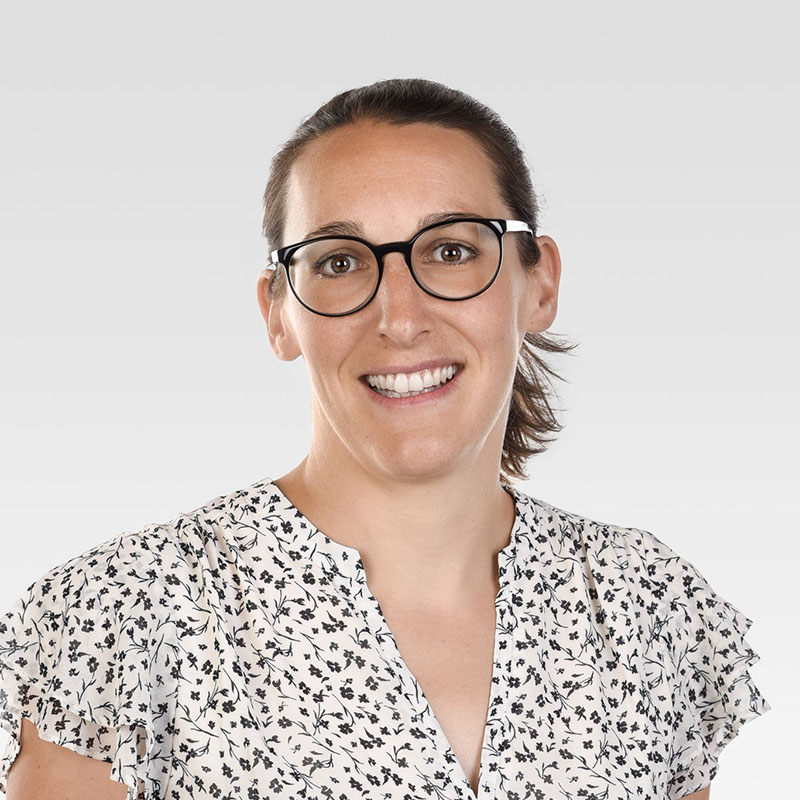
Lili obtained the MSc in Statistics from ETH in 2018. She wrote her Master thesis at the Swiss Data Science Center applying topic modelling to political data. She rejoined the center in May 2020 after a year as a statistical consultant at the Seminar for Statistics at ETH. With her MSc in Chemical Engineering, she worked as a process engineer in the glass industry for several years. She is interested in interdisciplinary projects where data science can help uncover new insights.


Luis is originally from Spain, where he completed his bachelor's studies in Electrical engineering, and the Ms.C. on signal theory and communications, both at the University of Seville. During his Ph.D. he started focusing on machine learning methods, more specifically message passing techniques for channel coding, and Bayesian methods for channel equalization. He carried it out between the University of Seville and the University Carlos III in Madrid, also spending some time at the EPFL, Switzerland, and Bell Labs, USA, where he worked on advanced techniques for optical channel coding. When he completed his Ph.D. in 2013, he moved to the Luxembourg Center on Systems Biomedicine, where he switched his interest to neuroscience, neuroimaging, life sciences, etc., and the application of machine learning techniques to these fields. During his 4 and a half years there as a Postdoc, he worked on many different problems as a data scientist, encompassing topics such as microscopy image analysis, neuroimaging, single-cell gene expression analysis, etc. He joined the SDSC in April 2018. As Lead Data Scientist, Luis coordinates projects in various domains. Several projects focus on the application of natural language processing and knowledge graphs to the study of different phenomena in social and political sciences. In the domains of architecture and engineering, Luis is responsible for projects centered on the application of novel generative methods to parametric modeling. Finally, Luis also coordinates different projects in robotics, ranging from collaborative robotic construction to deformable object manipulation.
PI | Partners:
description
Goal:
Semi-automatic update of the MS database seantis using the doctor's reports.
Approach:
- Build embedding of doctor's reports using Doc2Vec where one text line corresponds to one document.
- Multi-class classification of text lines using embedding vectors as features and manually assigned labels as targets. This intermediate step allows to predict text line labels for new unseen doctor's reports.
- For specific parts of seantis (MS diagnosis, MRI information, ...), tailored classification procedures were developed to predict columns of interest, e.g. MS diagnosis type, type of MRI (spinal or cranial) and whether new and/or contrast medium enhancing lesions were detected.
Impact:
Facilitate the update of the seantis database by providing predictions for fields of interest based on extracted information from doctor's reports.
Presentation
Gallery
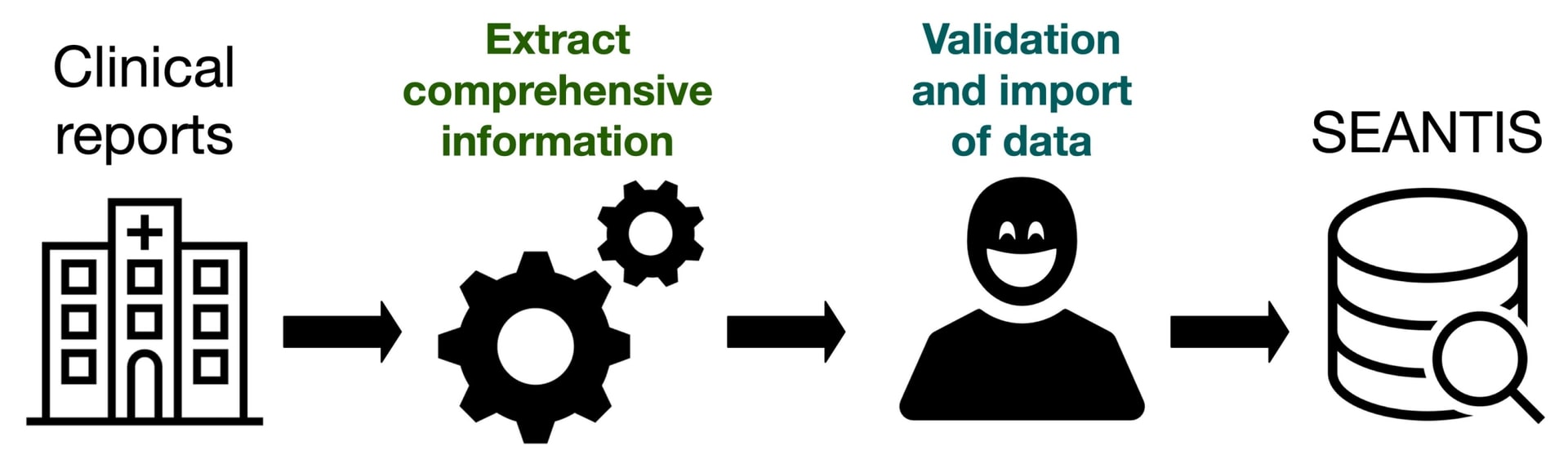
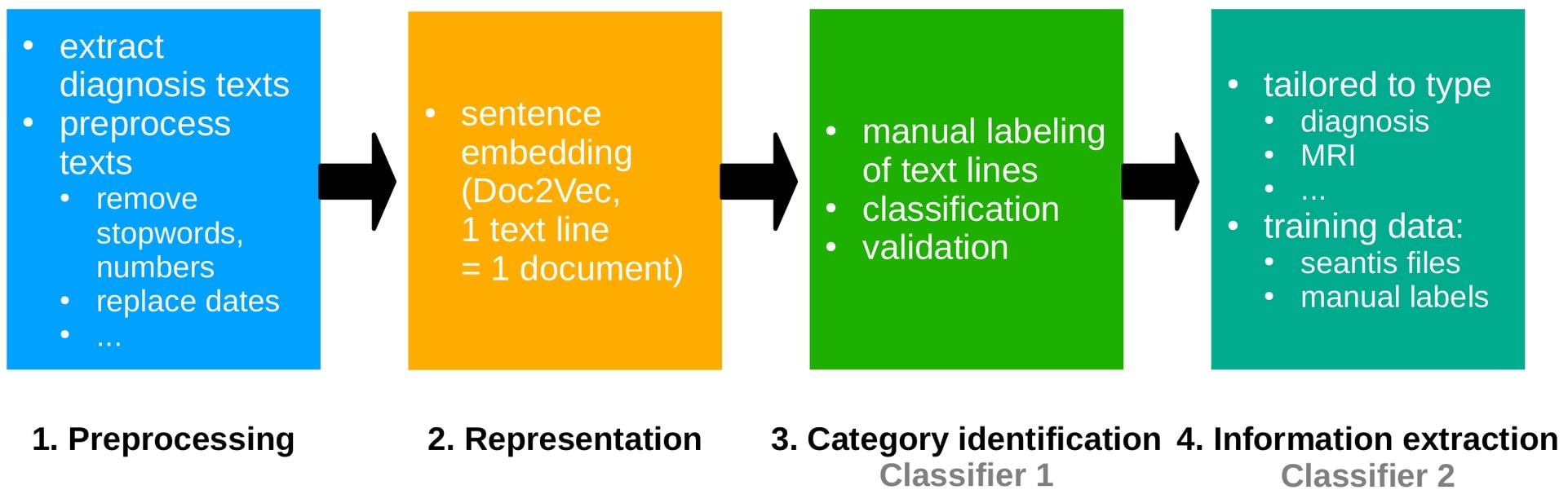
Annexe
Additional resources
Bibliography
Publications
Related Pages
More projects
STIMO
News
Latest news


Smartair | An active learning algorithm for real-time acquisition and regression of flow field data
Smartair | An active learning algorithm for real-time acquisition and regression of flow field data
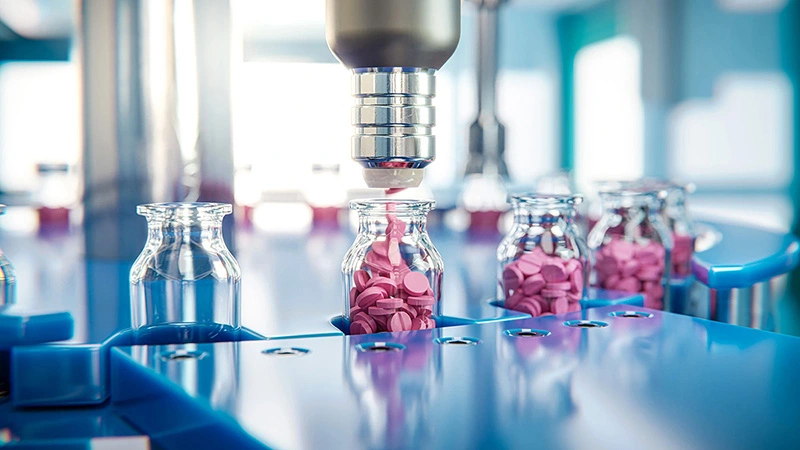
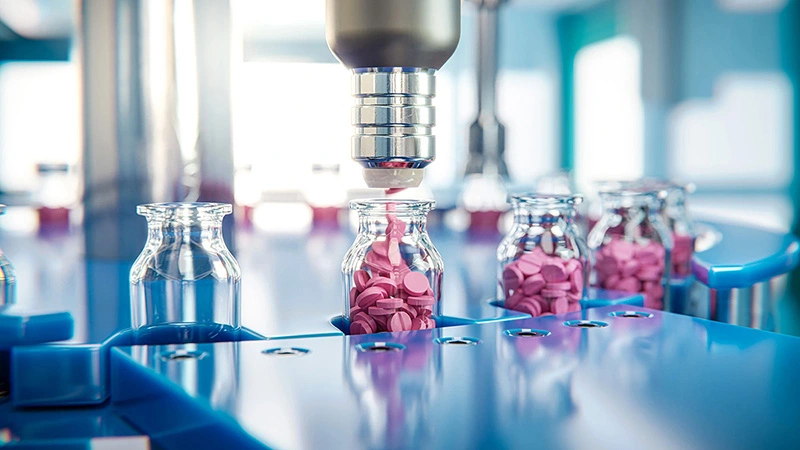
The Promise of AI in Pharmaceutical Manufacturing
The Promise of AI in Pharmaceutical Manufacturing


Efficient and scalable graph generation through iterative local expansion
Efficient and scalable graph generation through iterative local expansion
Contact us
Let’s talk Data Science
Do you need our services or expertise?
Contact us for your next Data Science project!