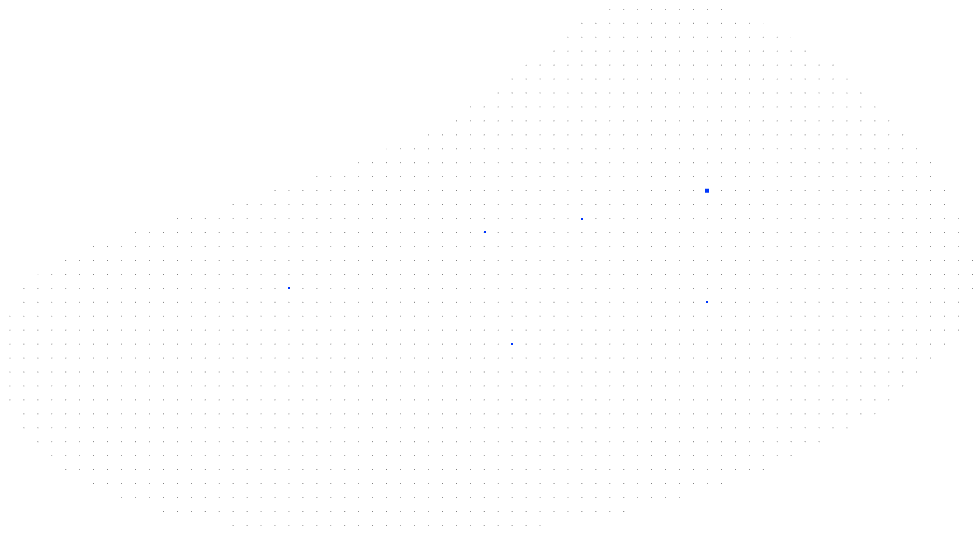
ADOPT
AI for Detecting Ocean Plastic Pollution with Tracking

Abstract
Marine litter is a growing ecologic concern that needs to be addressed at a global scale. While satellite and meteorological data is available in abundance, few advances have been made towards continuous monitoring of marine litter aggregated in floating patches on the oceans. To address this, we propose ADOPT, a hybrid model to automatically identify marine litter from satellite imagery and predict its location given meteorological information. In the first instance, ADOPT contains a deep learning-based detection module to identify aggregations of floating debris. In a second instance, it employs a physical drift estimation module to project the pathway of detected debris in space and time. These modules ingest Sentinel-2 and PlanetScope satellite time series as well as meteorological data to provide up-to-date, high-resolution estimates of the locations of floating objects. This is crucial for on-site ship-based cleanup efforts that rely on accurate and timely information on floating litter islands for efficient collection. Automating floating debris detection poses several challenges: the domain shift between the globally distributed satellite scenes is strong; the small footprint of debris induces a severely imbalanced dataset; a visual identification of debris is difficult even for experts; noisy and incomplete labels pose challenges to automated machine learning methods. The two-layered strategy tackles these issues, where the segmentation module is aided by a machine-learning-based tracking module. Addressing this real-world challenge in a physically sound manner will provide a unique opportunity to deploy methods beyond idealized benchmarks, and with ecological impact, providing cleanup missions with up-to-date and physically plausible marine litter location estimations.
People
Collaborators


Christian joined SDSC in 2019 to design data-driven solutions to support academic research projects. His research interests comprise inference of probabilistic models, with a focus on nonparametric models, and stochastic processes. He holds a PhD in Computational Neuroscience from the Technical University Berlin, Germany, a MSc from the Bernstein Center for Computational Neuroscience Berlin, and a BSc in Cognitive Science from the University of Osnabrück, Germany. During the course of his studies, he additionally conducted research at Khalifa University (UAE), Strathclyde University (UK), and the RIKEN Brain Science Institute and the Kyoto University in Japan. Before joining SDSC, he was an intern at Amazon CoreAI in Berlin.
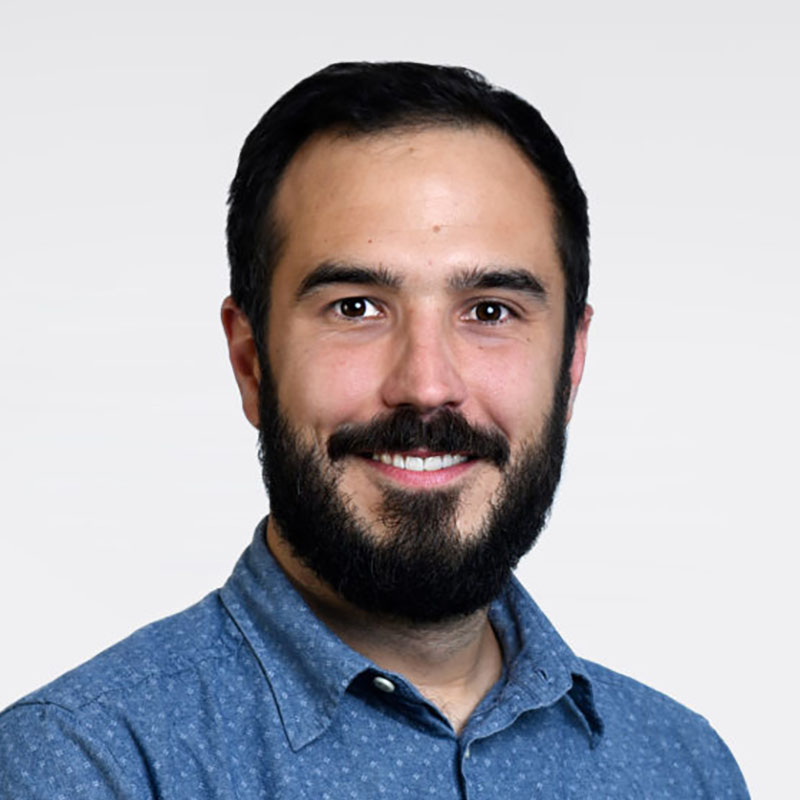
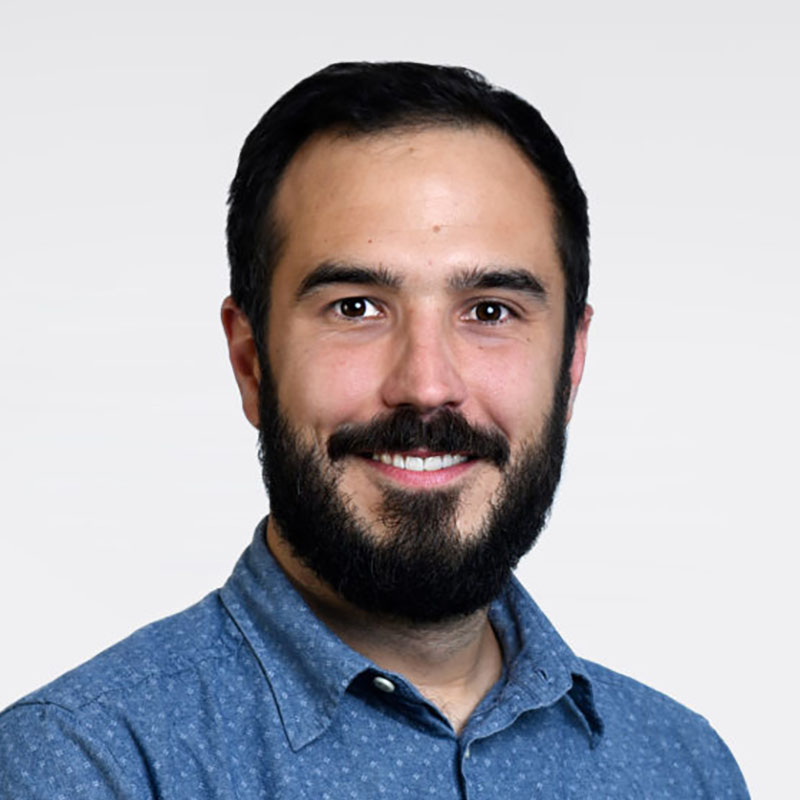
Michele received a Ph.D. in Environmental Sciences from the University of Lausanne (Switzerland) in 2013. He was then a visiting postdoc in the CALVIN group, Institute of Perception, Action and Behaviour of the School of Informatics at the University of Edinburgh, Scotland (2014-2016). He then joined the Multimodal Remote Sensing and the Geocomputation groups at the Geography department of the University of Zurich, Switzerland (2016-2017). His main research activities were at the interface of computer vision, machine and deep learning for the extraction of information from aerial photos, satellite optical images and geospatial data in general.
PI | Partners:
EPFL, Environmental Computational Science and Earth Observation Laboratory:
- Prof. Devis Tuia
- Dr. Emmanuele Dalsasso
description
Motivation
Marine litter is accumulating at alarming rates, with 19 to 23 million metric tonnes dispersed in 2016 alone (Borrelle et al., 2020). Plastic artifacts constitute 75% of marine litter, exceeding 5 trillion objects in numbers (Eriksen et al., 2014) and are causing a serious threat to marine ecosystems, climate, and the human food chain. To understand the dynamics of plastic debris and finally remove it from the eco-system it is key to automatize large-scale detection.
Proposed Approach / Solution
This project aims at tracking plastic debris in the ocean with satellite imagery. This involves two modules (see Figure 1):
1) Debris detection: Utilizing Segmentation algorithms, e.g. UNET++ (Russwurm et al., 2023), to efficiently detect plastic debris on satellite images. Since data are scarce, semi-supervised approaches are a promising direction to explore,
2) Debris tracking: To learn the dynamics of plastic debris, models that predict the effective flow field based on currents, wind, and waves. After identifying the location of debris the model should predict likely positions of the debris the next time satellite imagery is available (1-5 days). The envisioned method should account for uncertainty of the predictions, such that a search area is identified for the segmentation module to look next (see Figure 2 for an example prediction with a Hidden Markov Model).
Impact
The final product, an “AI for Detecting Ocean Plastic Pollution with Tracking” (ADOPT), will be able to not only detect litter objects with high accuracy but also provide a timely estimate of future locations, thanks to its ability to process images of different satellite sensors and the inclusion of a physically sound ocean drift module for tracking.
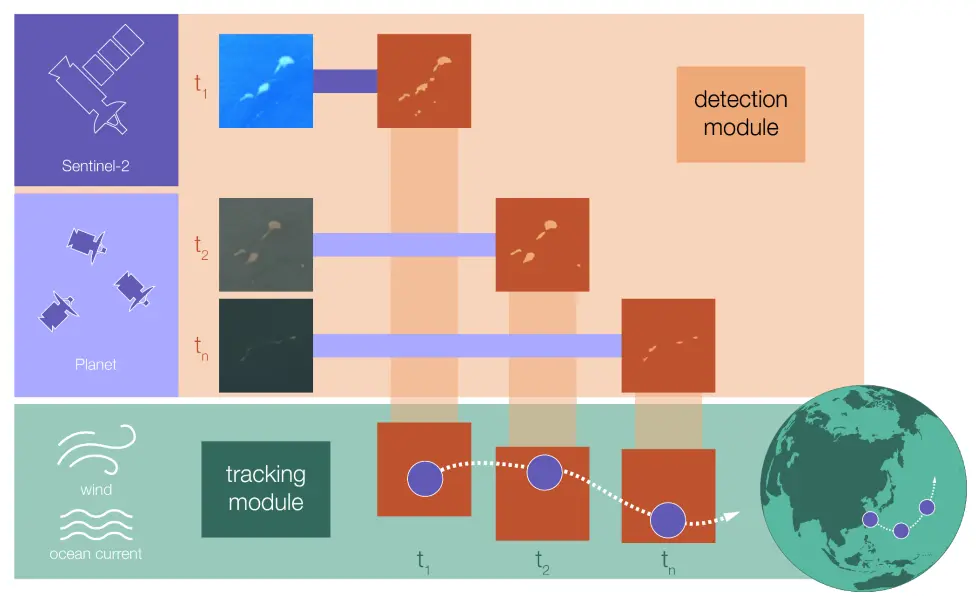

Presentation
Gallery
Annexe
Additional resources
Bibliography
- Borrelle, S. B., Ringma, J., Law, K. L., Monnahan, C. C., Lebreton, L., McGivern, A., ... & Rochman, C. M. (2020). Predicted growth in plastic waste exceeds efforts to mitigate plastic pollution. Science, 369(6510), 1515-1518. DOI: 10.1126/science.aba3656
- Eriksen, M., Lebreton, L. C., Carson, H. S., Thiel, M., Moore, C. J., Borerro, J. C., ... & Reisser, J. (2014). Plastic pollution in the world's oceans: more than 5 trillion plastic pieces weighing over 250,000 tons afloat at sea. PloS one, 9(12), e111913. DOI: 10.1371/journal.pone.0111913
- Carmo, R., Mifdal, J., & Rußwurm, M. (2021, September). Detecting macro floating objects on coastal water bodies using Sentinel-2 data. In OCEANS 2021: San Diego–Porto (pp. 1-7). IEEE. DOI: 10.23919/OCEANS44145.2021.9705668
- Russwurm, M. C., Gül, D., & Tuia, D. (2023, April). Improved marine debris detection in satellite imagery with automatic refinement of coarse hand annotations. In 11th International Conference on Learning Representations (ICLR) Workshops.
Publications
Related Pages
More projects
MAGNIFY
News
Latest news
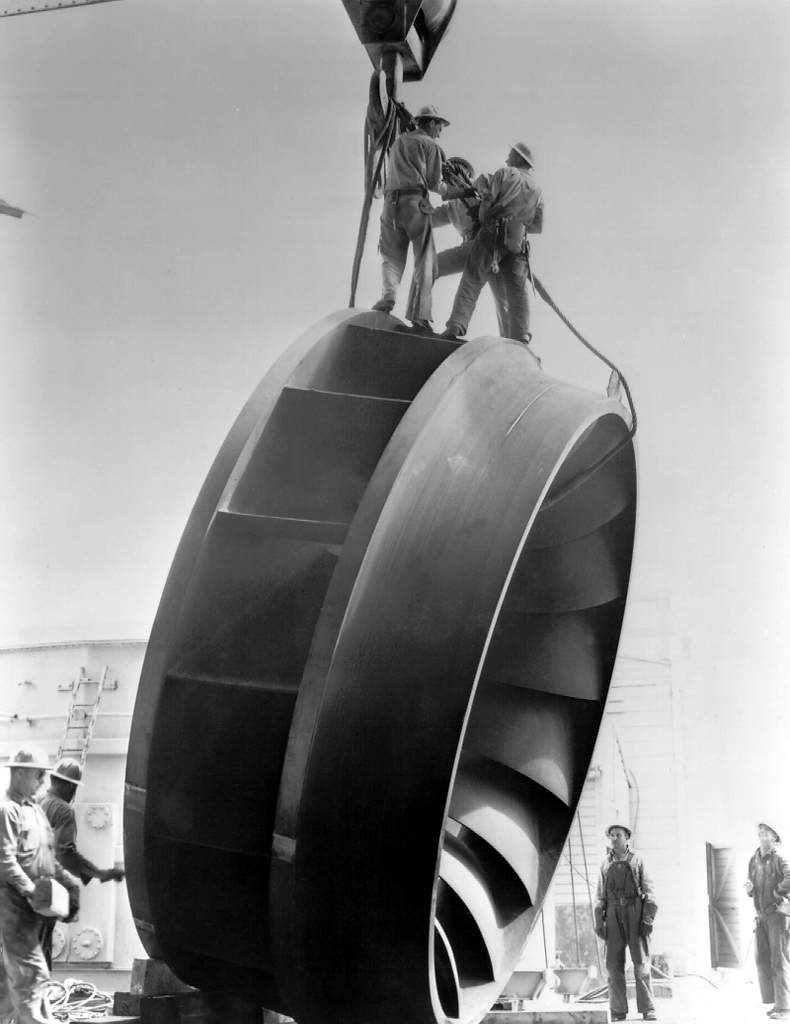
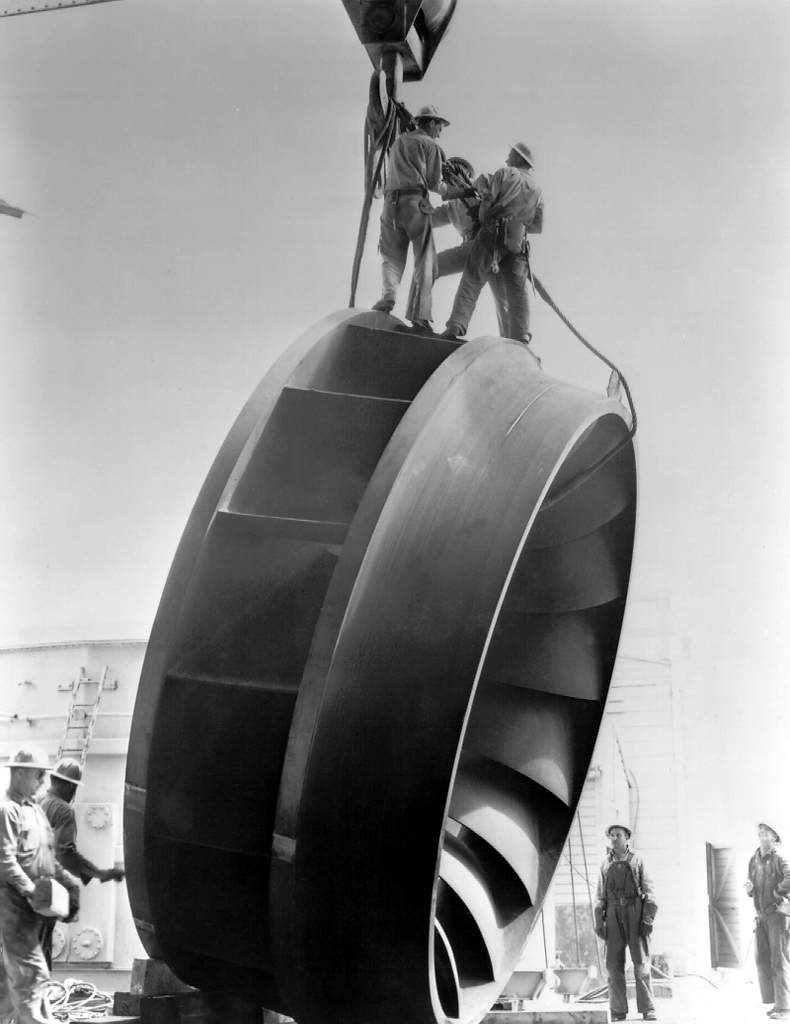
PAIRED-HYDRO | Increasing the Lifespan of Hydropower Turbines with Machine Learning
PAIRED-HYDRO | Increasing the Lifespan of Hydropower Turbines with Machine Learning


First National Calls: 50 selected projects to start in 2025
First National Calls: 50 selected projects to start in 2025
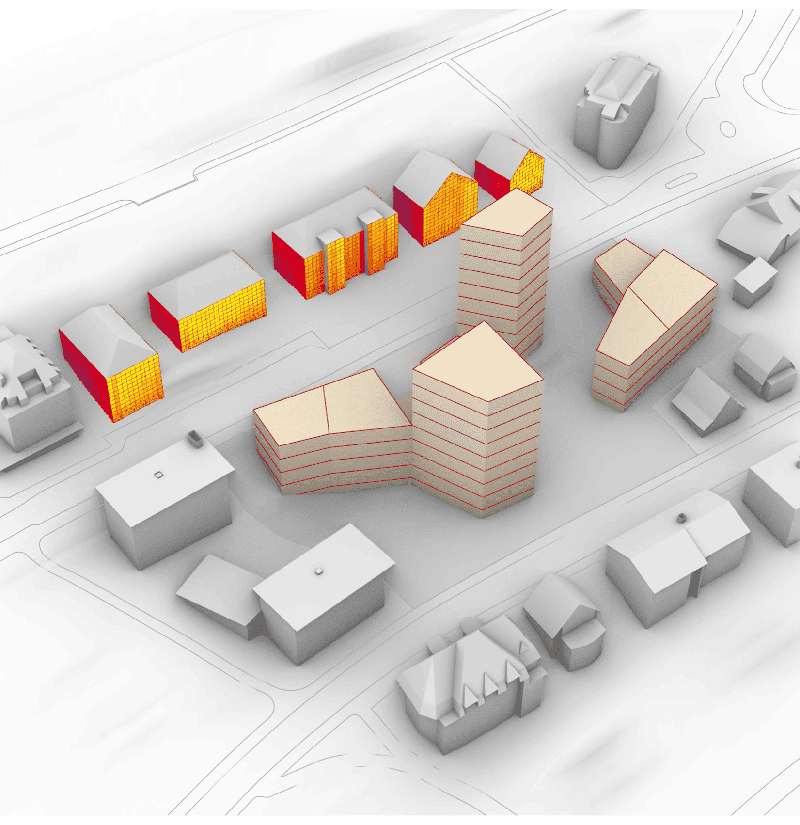
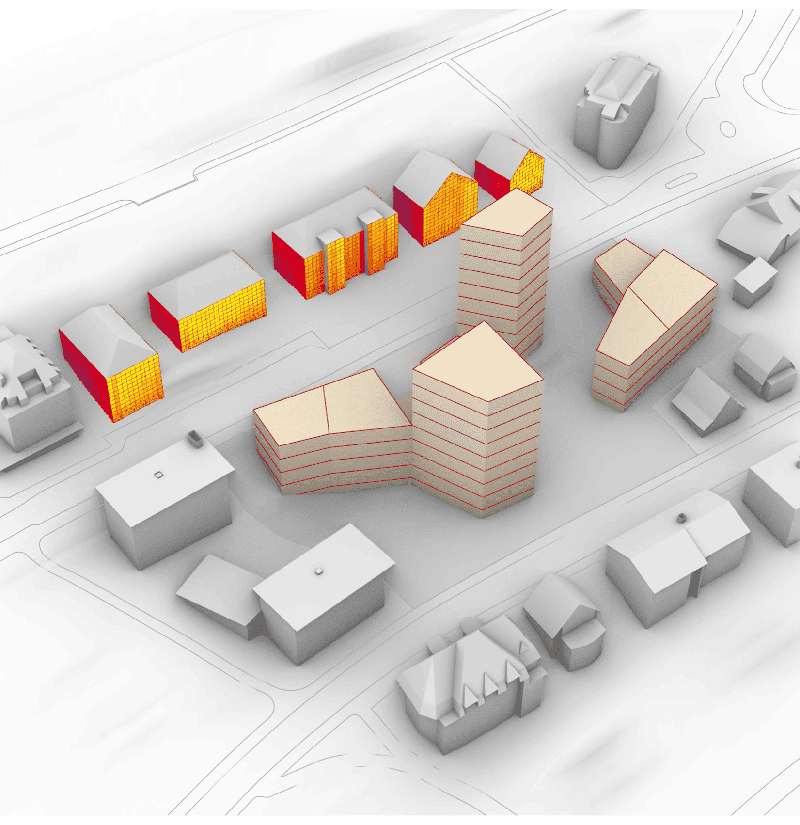
AIXD | Generative AI toolbox for architects and engineers
AIXD | Generative AI toolbox for architects and engineers
Contact us
Let’s talk Data Science
Do you need our services or expertise?
Contact us for your next Data Science project!