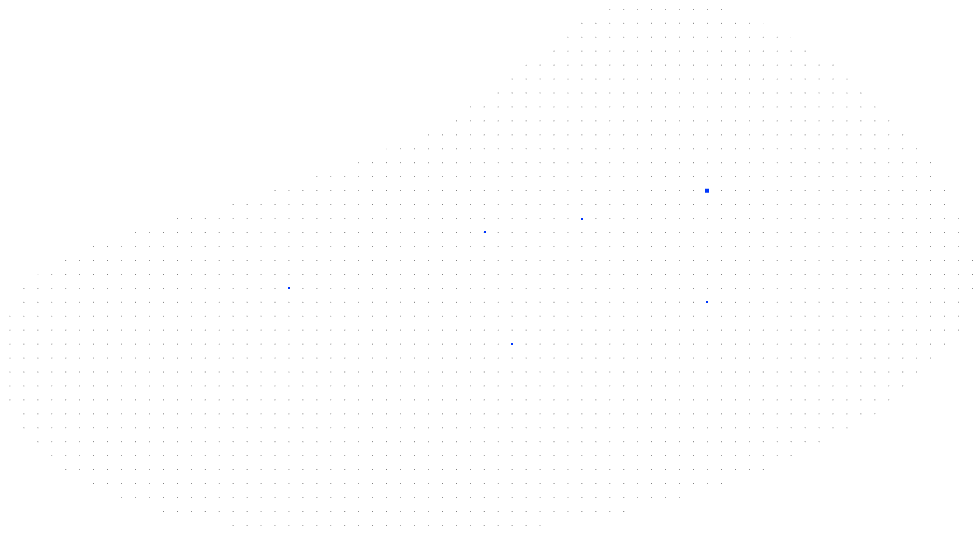
Fore[st]cast
Nowcast and predict forest condition
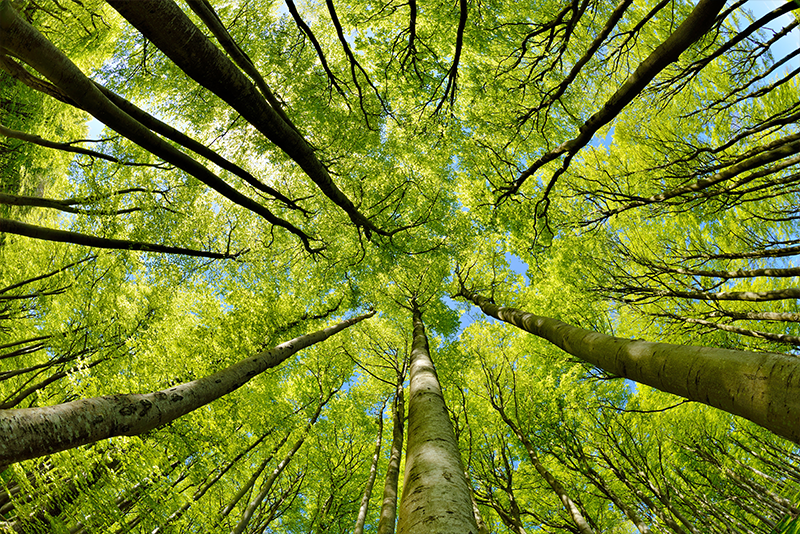
Abstract
Forests host about 90% of the world’s terrestrial biomass in the form of carbon and are an important pool for global biodiversity. We need long-term monitoring but near real-time data processing to fulfill modern society’s needs, both present and future.
This project is a step toward near real-time assessment of the condition of our forests, as has traditionally been done in meteorology or hydrology. The goal is to further develop a platform that reports on the current and predicted condition of trees (e.g., growth and drought stress) in relation to environmental variables.
With the TreeNet network, which is part of a long-term and large-scale forest infrastructure at WSL, we have developed a monitoring system that measures the responses of trees to their environment (air and soil) in near real-time and fully automated. This network consists of dendrometers installed on trees, measuring stem radius changes with high precision.
The data are transmitted at 10 minutes intervals to a central server, where they are cleaned, processed and converted into so-called nowcasts of drought severity and growth signals. What we still lack is the prediction of growth and tree water deficit, both temporally on scales from days to seasons and spatially at the scale of Switzerland beyond the point locations with active dendrometers.
People
Collaborators


William obtained a PhD in Statistics in 2015 jointly from the University of Geneva and the University of Sydney. He then worked as a post-doctoral research fellow at Dalhousie University as part of a Canadian Statistical Sciences Institute collaborative research team. He was an Assistant Professor of Statistics at Stevens Institute of Technology in Hoboken, New Jersey, before joining the SDSC in September 2020. His research interests include robust statistics, non-parametric methods, and spatio-temporal modeling. His recent cross-disciplinary collaborations involve applications in marine biology, volcanology, and fisheries science.
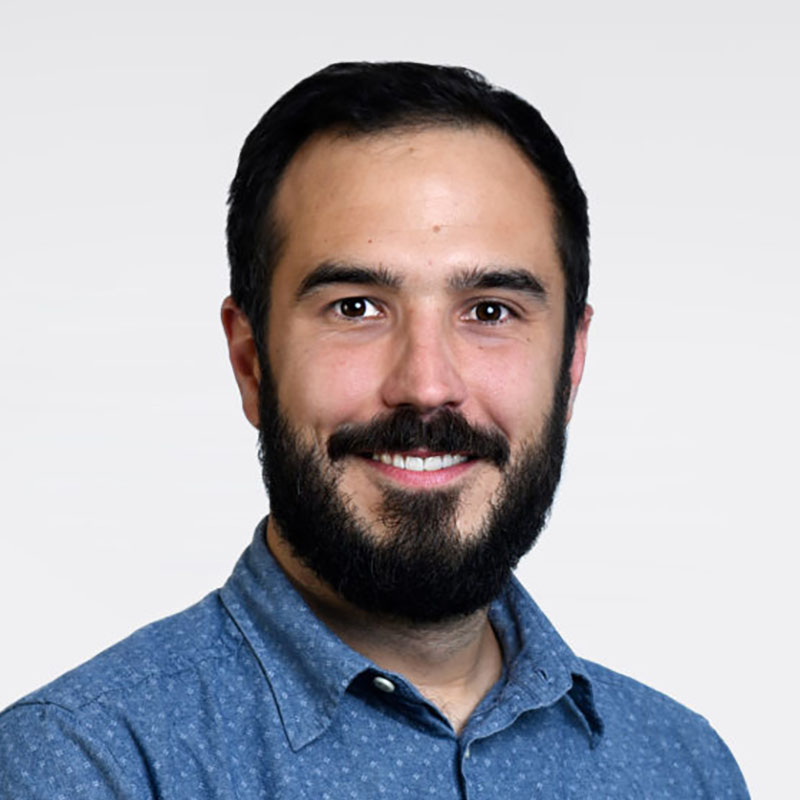
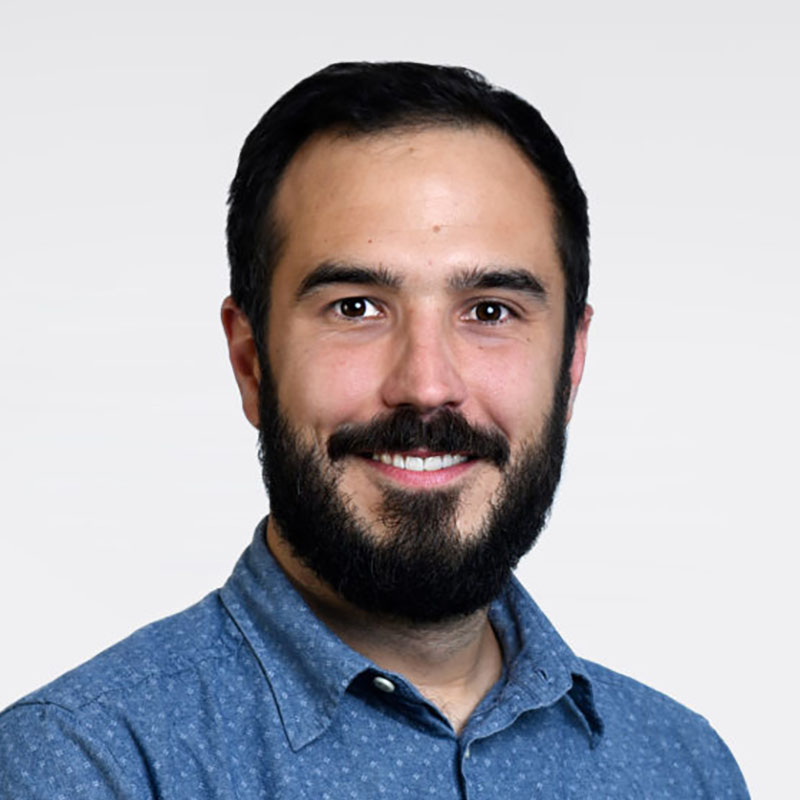
Michele received a Ph.D. in Environmental Sciences from the University of Lausanne (Switzerland) in 2013. He was then a visiting postdoc in the CALVIN group, Institute of Perception, Action and Behaviour of the School of Informatics at the University of Edinburgh, Scotland (2014-2016). He then joined the Multimodal Remote Sensing and the Geocomputation groups at the Geography department of the University of Zurich, Switzerland (2016-2017). His main research activities were at the interface of computer vision, machine and deep learning for the extraction of information from aerial photos, satellite optical images and geospatial data in general.
PI | Partners:
WSL, Forest Dynamics Group:
- Dr. Roman Zweifel
- Dr. Mirko Lukovic
- Dr. Jan Svoboda
- Dr. Katrin Meusburger
- Prof. Dr. Arthur Gessler
description
Motivation
The main current tasks are about the prediction of tree water deficit (TWD) measured at sparse locations in Switzerland but at regular time intervals. Two models are being developed in parallel: one focuses on temporal forecasts and one on spatial prediction. The output from these models would then be fed to the TreeNet online platform as well as integrated with various drought monitoring platforms in Switzerland.
Proposed Approach / Solution
SDSC is involved in the development of predictive models tailored for TWD in the context of sparse spatial locations but with relatively high temporal frequency. The first model is an adaptation of the Neural Hierarchical Interpolation for Time Series Forecasting (N-HiTS) model introduced by Challu et al. (2023). This recurrent neural network architecture relies on harmonic bases to represent processes at various temporal scales, similar to Fourier bases in spectral analysis. By leveraging both meteorological and environmental variables, as well as past histories of TWD series, this N-HiTS model can forecast TWD for trees with dendrometers with impressive accuracy. The second model is a long short-term memory (LSTM) neural network tailored for spatial prediction while modeling time series at locations with TWD records. The reason for using a recurrent model for spatial prediction is that trees with dendrometers are only at a few sites which do not cover Switzerland well. Thus only variables computed on a regular grid over Switzerland are used as input features to allow the model to learn reliable relations in time in hopes to then predict at arbitrary locations. These predictions would in turn be processed over particular regions, such as large hydrological catchments, to create maps of TWD used in drought monitoring platforms.
Impact
When both models achieve satisfactory performance, both in temporal and spatial prediction of TWD, outputs would be directly usable in forest health monitoring, biodiversity forecasts, and drought monitoring platforms. The latter is notably important for the national system for drought monitoring, forecasting and warning developed by the Swiss Federal Office for the Environment (FOEN) jointly with MeteoSwiss and Swisstopo, and whose first iteration is to be deployed in 2025. Using tree water deficit as an indirect measure of (lack of) water availability in the soil, in addition to more direct indices like predicted precipitation and air temperature, would be crucial for agricultural planning and securing drinking water supplies.
Presentation
Gallery
Annexe
Cover image source: Adobe Stock
Additional resources
Bibliography
- Zweifel, R., Pappas, C., Peters, R. L., Babst, F., Balanzategui, D., Basler, D., Bastos, A., Beloiu, M., Buchmann, N., Bose, A. K., and others. (2023). Networking the forest infrastructure towards near real-time monitoring–A white paper. Science of the Total Environment, 872, 162167. https://doi.org/10.1016/j.scitotenv.2023.162167
- Zweifel, R., Etzold, S., Basler, D., Bischoff, R., Braun, S., Buchmann, N., Conedera, M., Fonti, P., Gessler, A., Haeni, M., and others. (2021). TreeNet – The biological drought and growth indicator network. Frontiers in Forests and Global Change, 4, 776905. https://doi.org/10.3389/ffgc.2021.776905
- Luković, M., Zweifel, R., Thiry, G., Zhang, C., & Schubert, M. (2022). Reconstructing radial stem size changes of trees with machine learning. Journal of the Royal Society Interface, 19 (194), 20220349. https://doi.org/10.1098/rsif.2022.0349
Publications
Related Pages
More projects
MAGNIFY
News
Latest news
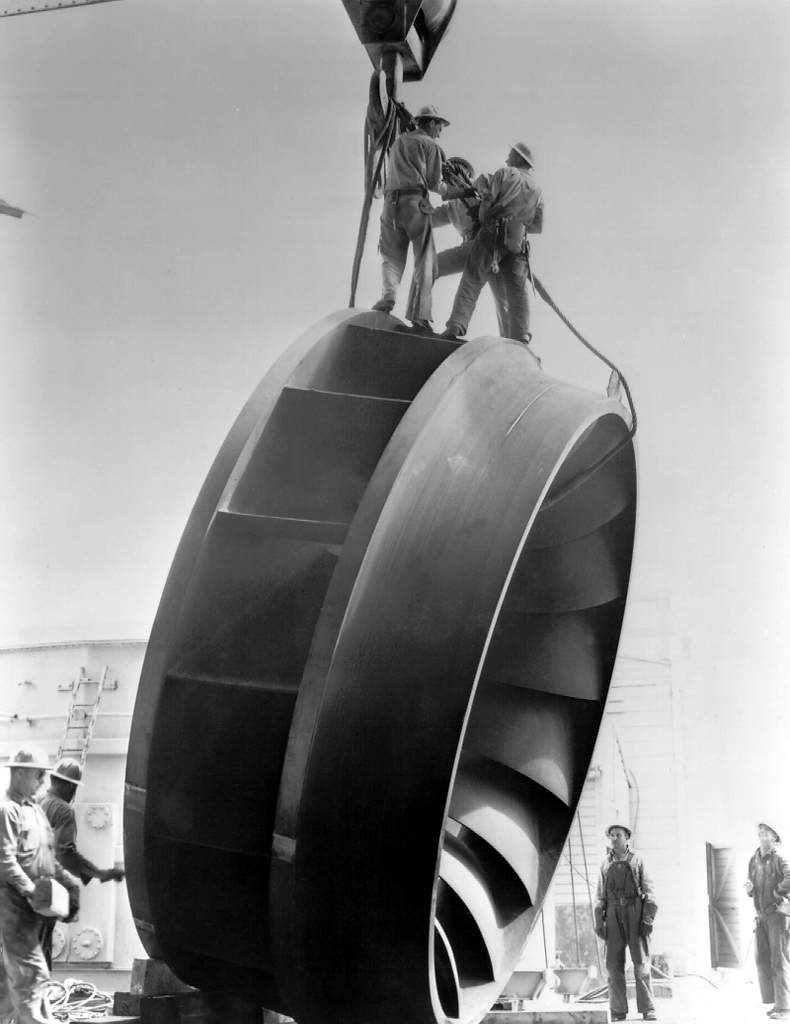
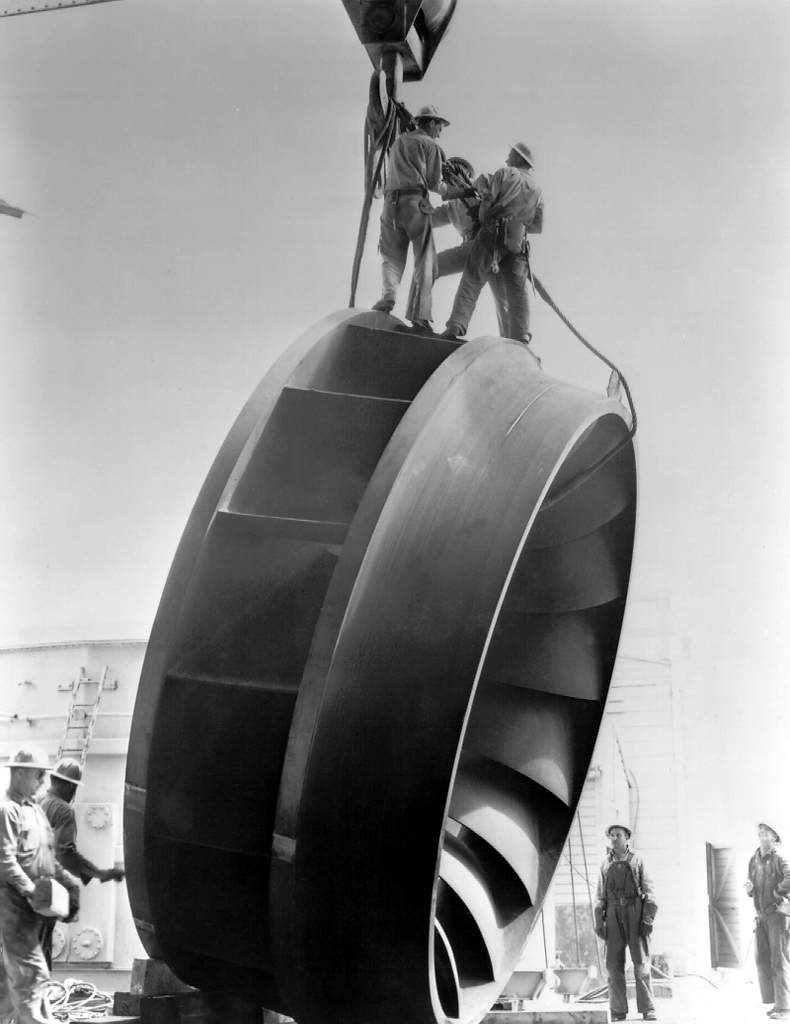
PAIRED-HYDRO | Increasing the Lifespan of Hydropower Turbines with Machine Learning
PAIRED-HYDRO | Increasing the Lifespan of Hydropower Turbines with Machine Learning


First National Calls: 50 selected projects to start in 2025
First National Calls: 50 selected projects to start in 2025
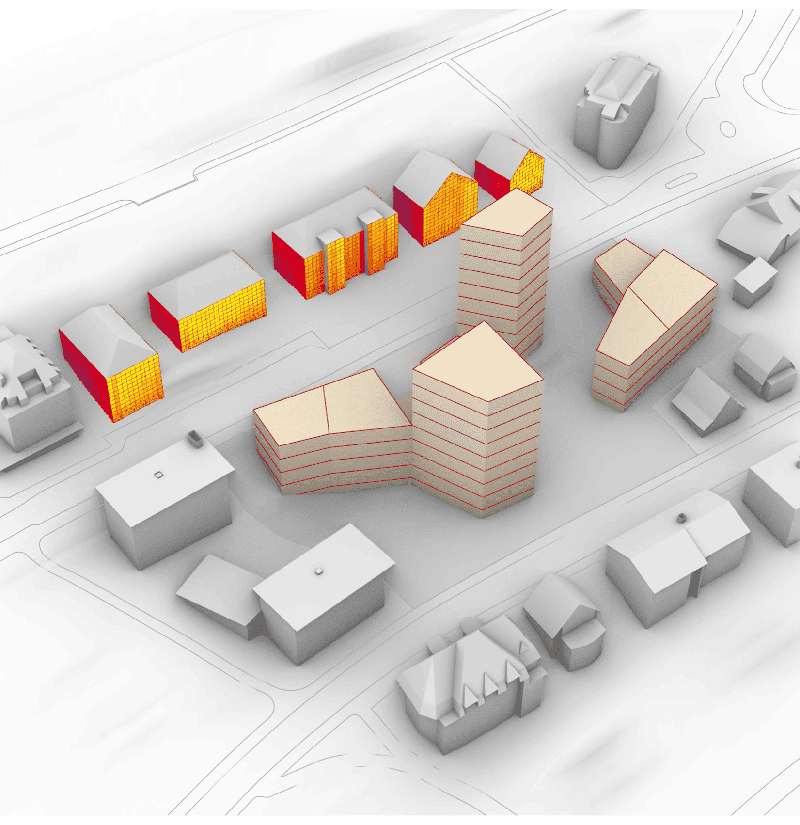
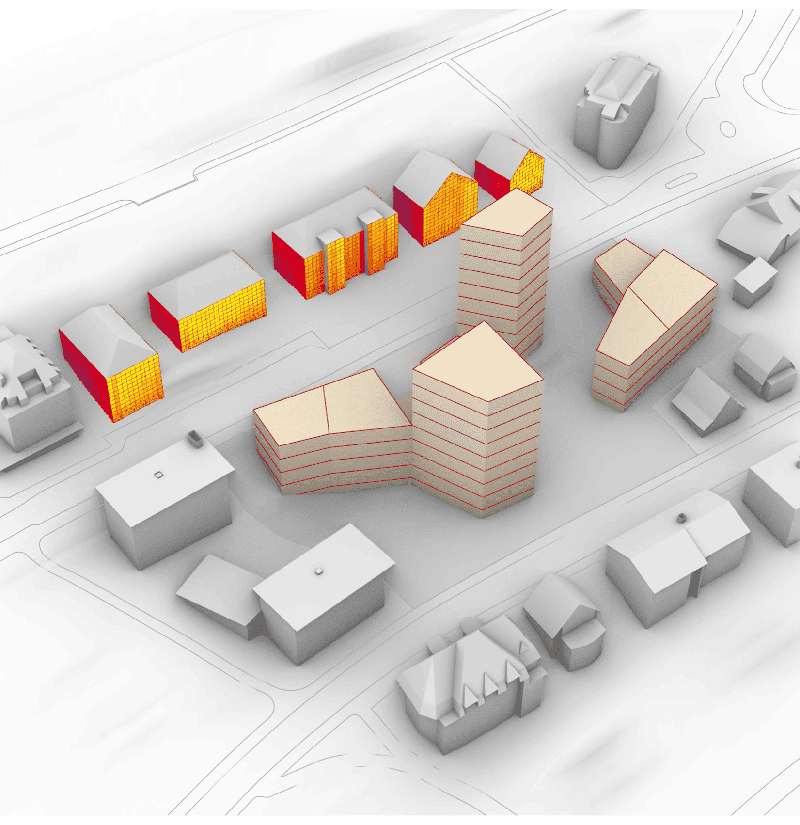
AIXD | Generative AI toolbox for architects and engineers
AIXD | Generative AI toolbox for architects and engineers
Contact us
Let’s talk Data Science
Do you need our services or expertise?
Contact us for your next Data Science project!