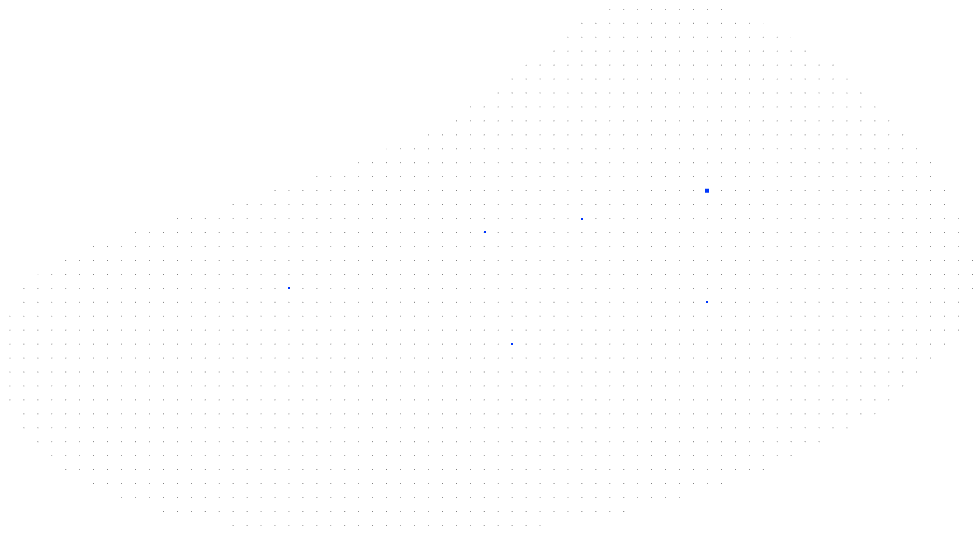
SDATE
Smart Data Acquisition for Tomoscopy Experiments

Abstract
Imaging experiments with synchrotron radiation are generating data at ever-increasing rates. In order to increase the efficiency for experiments at synchrotron light sources as well as the corresponding data transmission, processing, analysis and storage, a breakthrough step would come from the so-called smart experiment which is able to perform experiments and collect data intelligently.
In this project, we plan to demonstrate this concept using data from various types of time-resolved X-ray tomography (tomoscopy) experiments at the TOMCAT beamline of the Swiss Light Source. We are aiming to develop novel data science approaches (e.g., machine learning algorithms) to extract features from tomographically reconstructed data and/or raw projection images, and use these features to detect different phases and the changepoints between them in near real time in a dynamic process.
This information can not only help guide human experts during a fast-paced experiment but also be used as feedback to the data acquisition system. As a result, the data acquisition and readout can be performed at a speed which is fast enough to guarantee adequate temporal sampling of the fastest event during the entire dynamic process, without worrying about excessive oversampling of the slow events.
Although the reconstructed data are more interpretable and provide more insight into the dynamic process compared to the raw data, the state-of-the-art live reconstruction pipeline is only capable of generating a small number of arbitrarily oriented reconstructed slices in real time. It poses one of the major challenges in this project and one possible solution would be to directly extract features from the raw data.
A successful completion of the project will pave the way for implementing a smart data acquisition system for tomoscopy experiments, and is foreseen to significantly reduce the amount of data written to disks and accelerate scientific discovery.
People
Collaborators
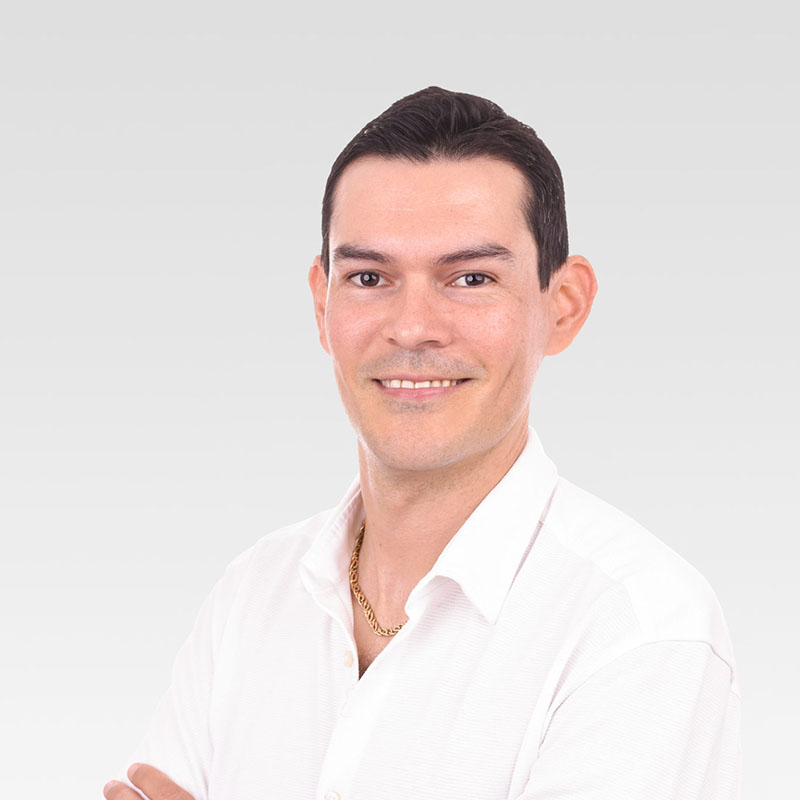
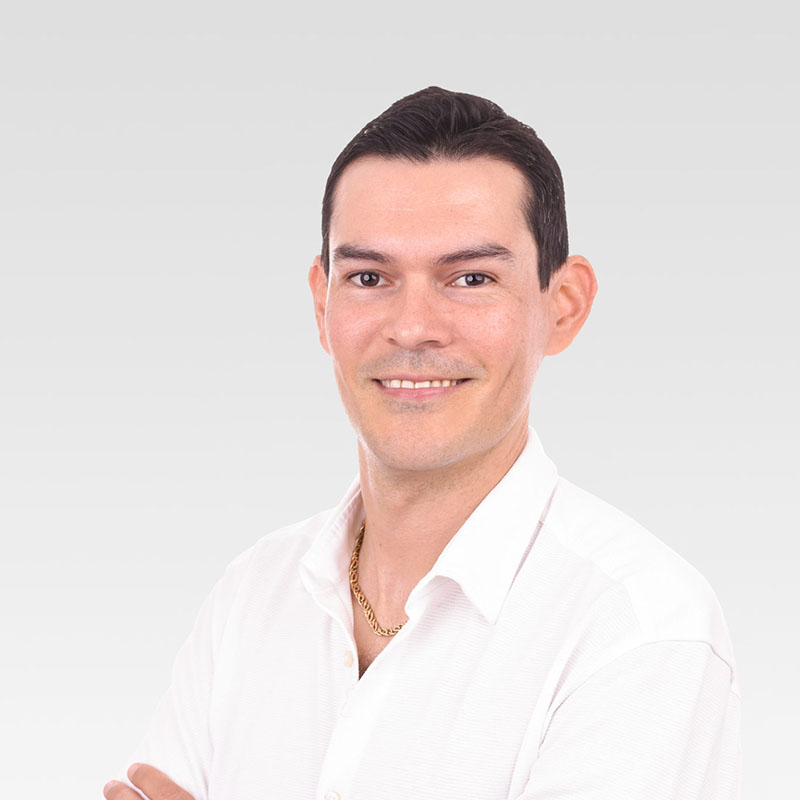
Luis Barba Flores joined the SDSC in 2022 as Senior Data Scientist. He received a joined PhD in Computers Science in 2016 from the Université Libre de Bruxelles and Carleton University. He served as a postdoctoral researcher at ETH Zurich from 2016 to 2019, and then moved to EPFL Lausanne to work in the Machine Learning and Optimization Group until 2022. His research interests include distributed optimization algorithms, first-order optimization methods and their applications to Deep Learning models.
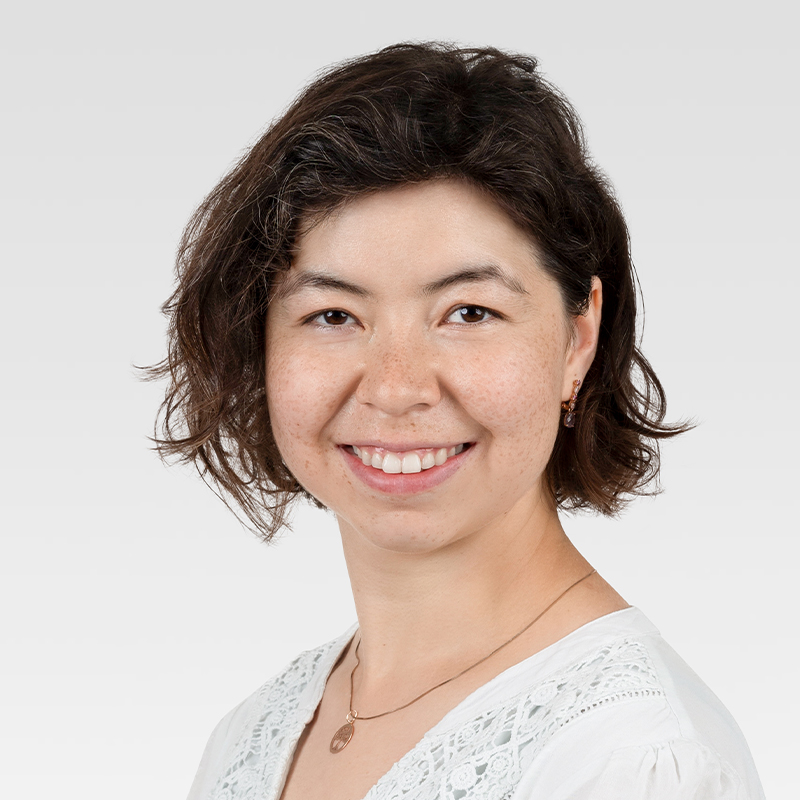
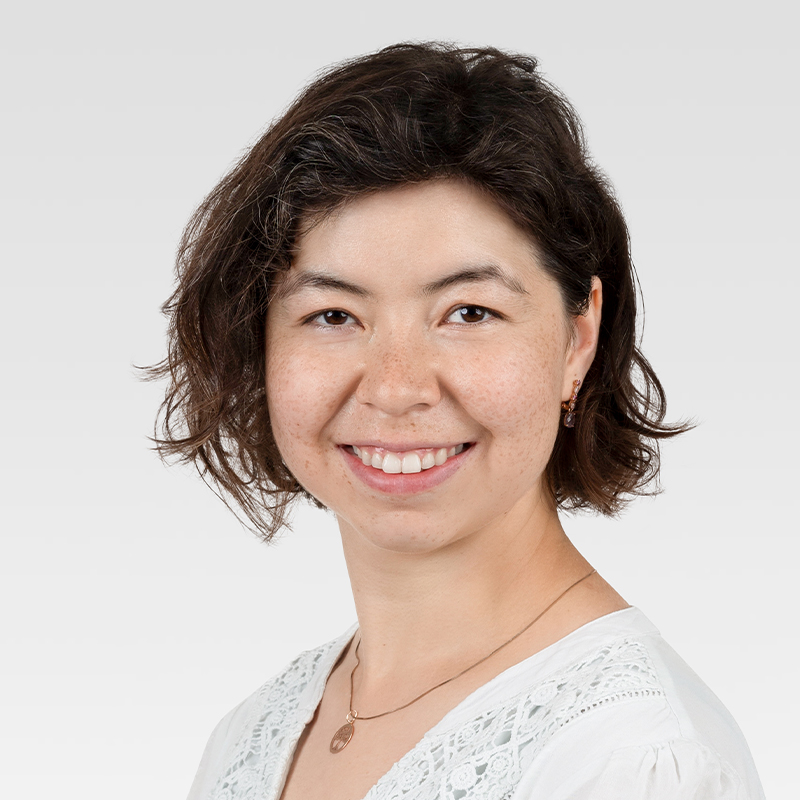
During 2023, Ilnura was doing a postdoc at the Technion, Israel, working with Prof. Dr. Kfir Levy. She received her PhD in September 2022 from the Automatic Control Laboratory (IfA) at ETH, Zürich. She joined IfA in 2017 to the Prof. Dr. Maryam Kamgarpour group and was co-supervised by Prof. Dr. Andreas Krause, working on safe learning methods. She obtained her Master's Degree jointly in Applied Mathematics at Institut Polytechnique de Grenoble (France) and Moscow Institute of Physics and Technology (Russia) in 2017. Her Master's thesis research was on stochastic optimization theory under the supervision of Prof. Dr. Anatoli Juditsky. Her Bachelor of Physics and Applied Math was obtained from the Moscow Institute of Physics and Technology (Russia) in 2015. Ilnura was born and finished high school in Almaty, Kazakhstan.
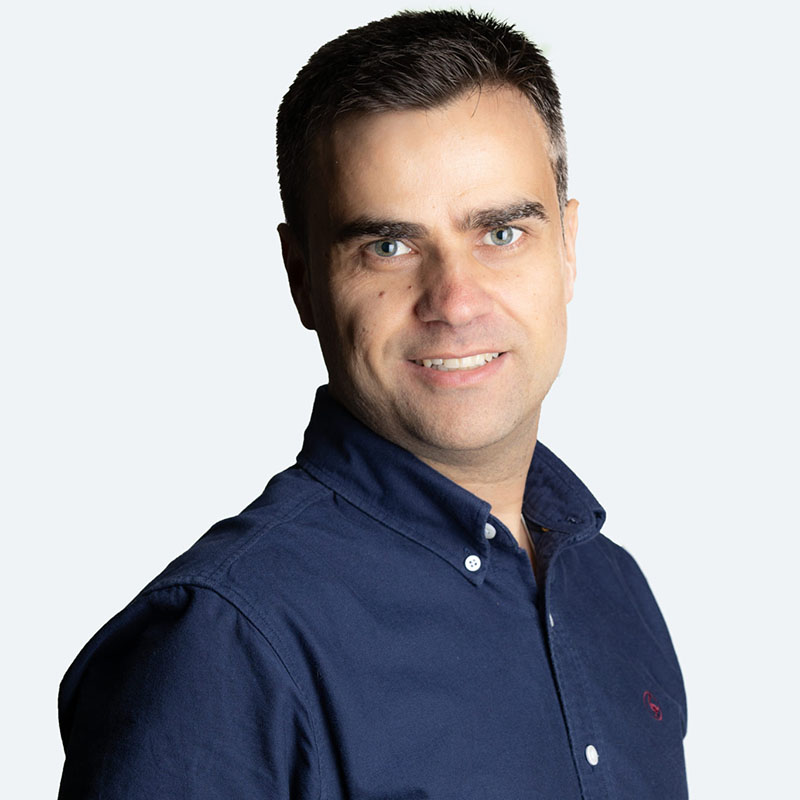
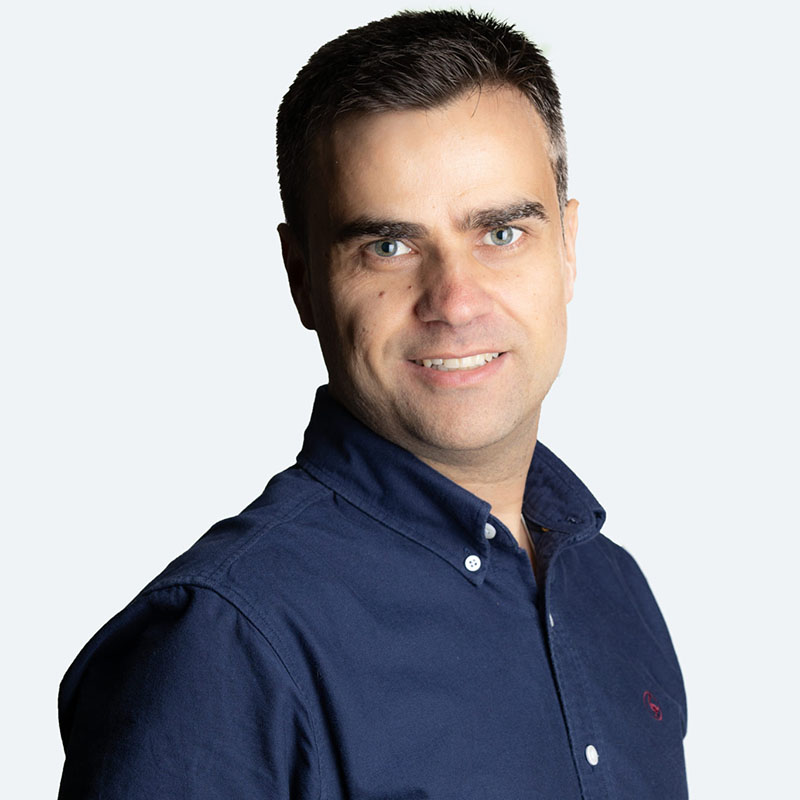
Benjamín Béjar received a PhD in Electrical Engineering from Universidad Politécnica de Madrid in 2012. He served as a postdoctoral fellow at École Polytechnique Fédérale de Lausanne until 2017, and then he moved to Johns Hopkins University where he held a Research Faculty position until Dec. 2019. His research interests lie at the intersection of signal processing and machine learning methods, and he has worked on topics such as sparse signal recovery, time-series analysis, and computer vision methods with special emphasis on biomedical applications. Since 2021, Benjamin leads the SDSC office at the Paul Scherrer Institute in Villigen.
description
Motivation
Time-resolved X-ray tomography experiments (tomoscopy) at synchrotron radiation facilities generate data at unprecedented rates, posing significant challenges in data management, transmission, storage, and analysis. With the upcoming upgrade of the Swiss Light Source (SLS 2.0), data generation is expected to exceed tens of terabytes per day, overwhelming current data-handling capabilities. Therefore, there is an urgent need to develop intelligent methods for real-time data acquisition, aiming at capturing only scientifically relevant data and drastically reducing data storage and processing requirements.

Proposed Approach
The project proposes to implement a smart data acquisition system leveraging advanced data science and machine learning techniques. Specifically, it will develop algorithms capable of extracting critical features directly from tomographic images and reconstructing data streams in near-real-time. By integrating these algorithms into the data acquisition pipeline at the TOMCAT beamline, the system will intelligently identify significant changepoints during dynamic experiments, selectively writing only the relevant data to disk. This smart system will be tested through simulated experiments using real-world datasets, ensuring robust performance prior to full integration. An example of important changes are highlighted in Figures 1 and 2 for two different tomoscopy experiments.
Impact
Successful implementation of this smart data acquisition framework is anticipated to reduce the amount of data written to disk by factors between 4 and 40, significantly surpassing conventional compression methods. This dramatic reduction in data storage will accelerate data processing, facilitate real-time decision-making during experiments, and enhance the scientific throughput at the TOMCAT beamline. Furthermore, the open-source algorithms developed will be generalizable to other synchrotron beamlines worldwide, benefiting a broader scientific community and solidifying TOMCAT’s leading position in tomoscopy research.

Presentation
Gallery
Annexe
Cover image source: Adobe Stock
Additional resources
Bibliography
- García-Moreno, F. et al. Tomoscopy: Time-Resolved Tomography for Dynamic Processes in Materials. Advanced Materials, 33 (2021).
- Marone, F., Studer, A., Billich, H., Sala, L. & Stampanoni, M. Towards on-the-fly data post-processing for real-time tomographic imaging at TOMCAT. Advanced Structural and Chemical Imaging, 3 (2017).
- Buurlage, J.-W. et al. Real-time reconstruction and visualisation towards dynamic feedback control during time-resolved tomography experiments at TOMCAT. Scientific Reports, 9 (2019).
- Novák, V., Blažek, M., Schlepütz, C. M., Kočí, P. & Stampanoni, M. Drying of water from porous structures investigated by time-resolved X-ray tomography. Drying Technology, 1–19 (2022).
- Fischer, R. et al. Wicking dynamics in yarns. Journal of Colloid and Interface Science, 625, 1–11 (2022).
Publications
Related Pages
More projects
MAGNIFY
News
Latest news


First National Calls: 50 selected projects to start in 2025
First National Calls: 50 selected projects to start in 2025
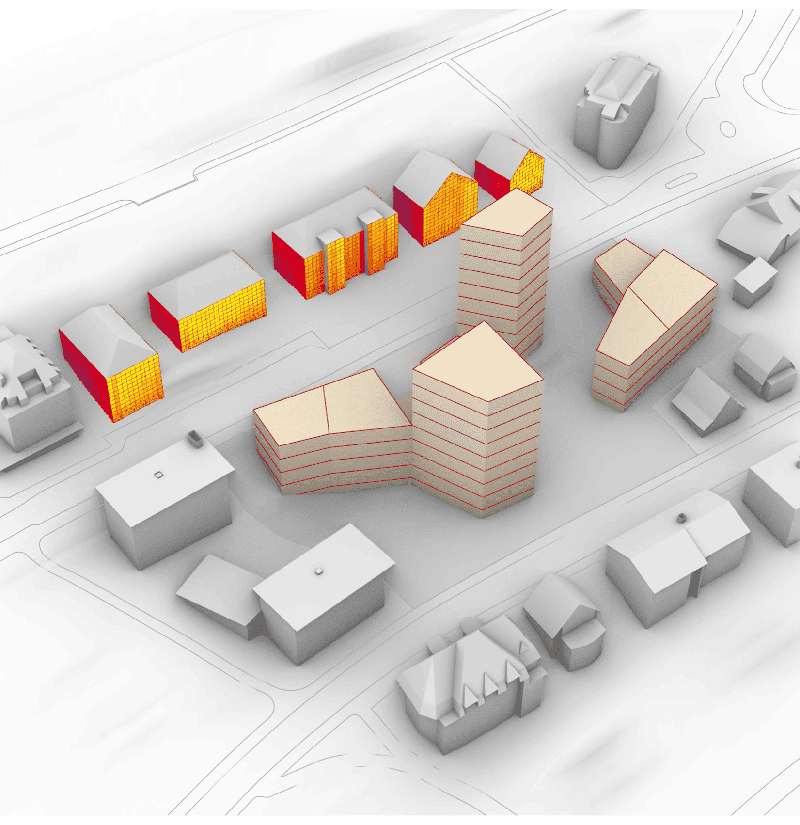
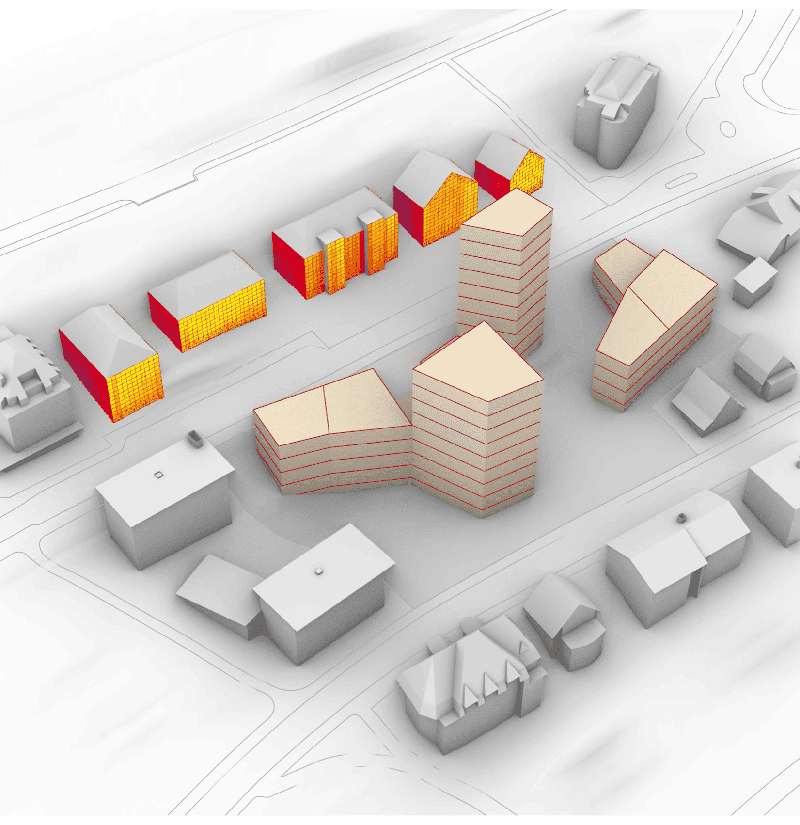
AIXD | Generative AI toolbox for architects and engineers
AIXD | Generative AI toolbox for architects and engineers


Smartair | An active learning algorithm for real-time acquisition and regression of flow field data
Smartair | An active learning algorithm for real-time acquisition and regression of flow field data
Contact us
Let’s talk Data Science
Do you need our services or expertise?
Contact us for your next Data Science project!