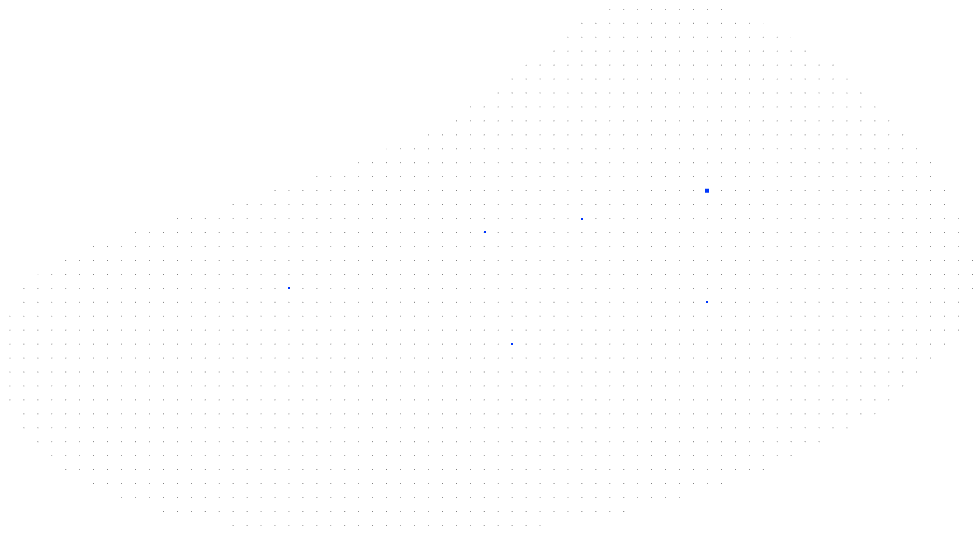
PACMAN LHC
Particle Accelerators and Machine Learning

Abstract
Particle accelerator facilities have a wide range of operational needs when it comes to tuning, optimization, and control. At the Large Hadron Collider (LHC) at CERN reducing the risks related to the high beam power by reducing the beam losses will lead to an increase in particle collision rates and a deeper understanding of the physics mechanisms. In order to meet these demands, particle accelerators rely on interactions with control systems, on fine-tuning of machine settings by operators, on online optimization routines, and on databases of previous settings that were known to be optimal for some desired operating condition. We aim to bring Machine Learning (ML) to particle accelerator operation to increase their performance. Each of the mentioned operational needs have corresponding ML-based approaches that could be used to supplement the existing workflows. In addition, new High-Luminosity LHC (HL-LHC) and the Future Circular Collider (FCC) designs will be proposed based on the LHC findings and prepared for more effective novel FCC operation.
People
Collaborators
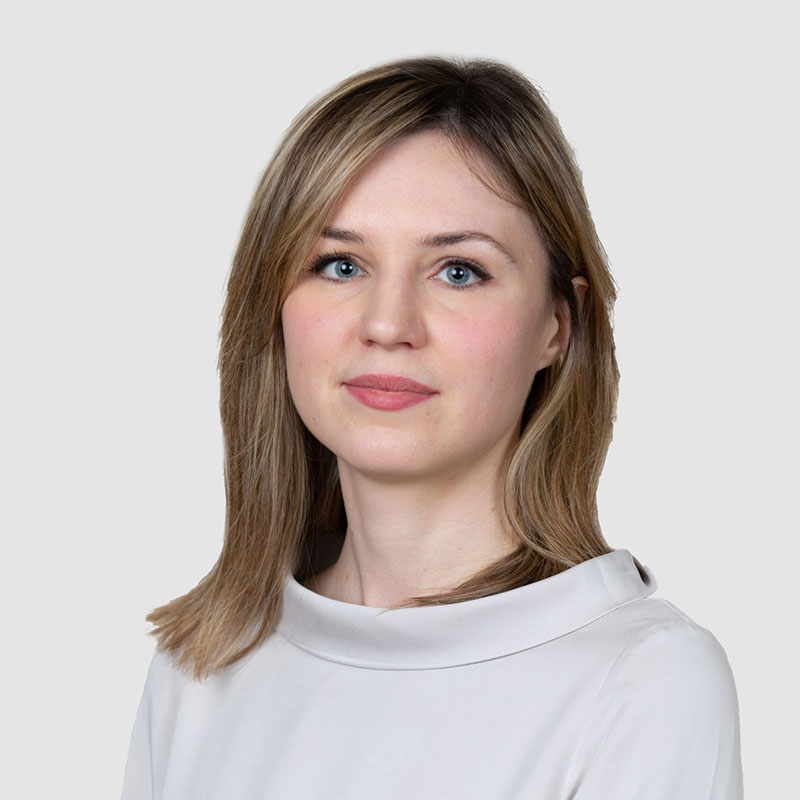
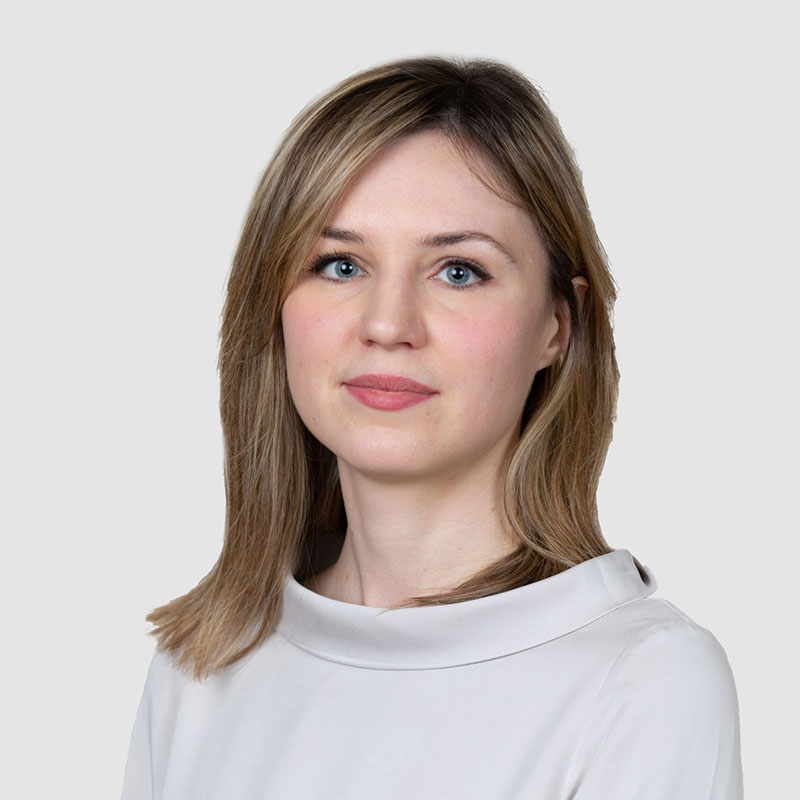
Ekaterina received her PhD in Computer Science from Moscow Institute for Physics and Technology, Russia. Afterwards, she worked as a researcher at the Institute for Information Transmission Problems in Moscow and later as a postdoctoral researcher in the Stochastic Group at the Faculty of Mathematics at University Duisburg-Essen, Germany. She has experience with various applied projects on signal processing, predictive modelling, macroeconomic modelling and forecasting, and social network analysis. She joined the SDSC in November 2019. Her interests include machine learning, non-parametric statistical estimation, structural adaptive inference, and Bayesian modelling.
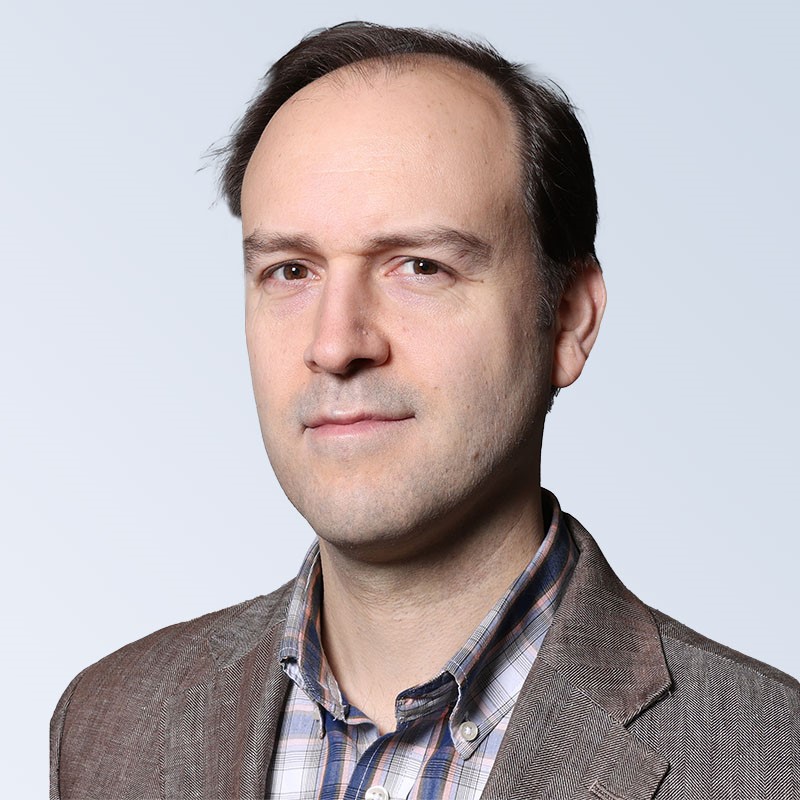
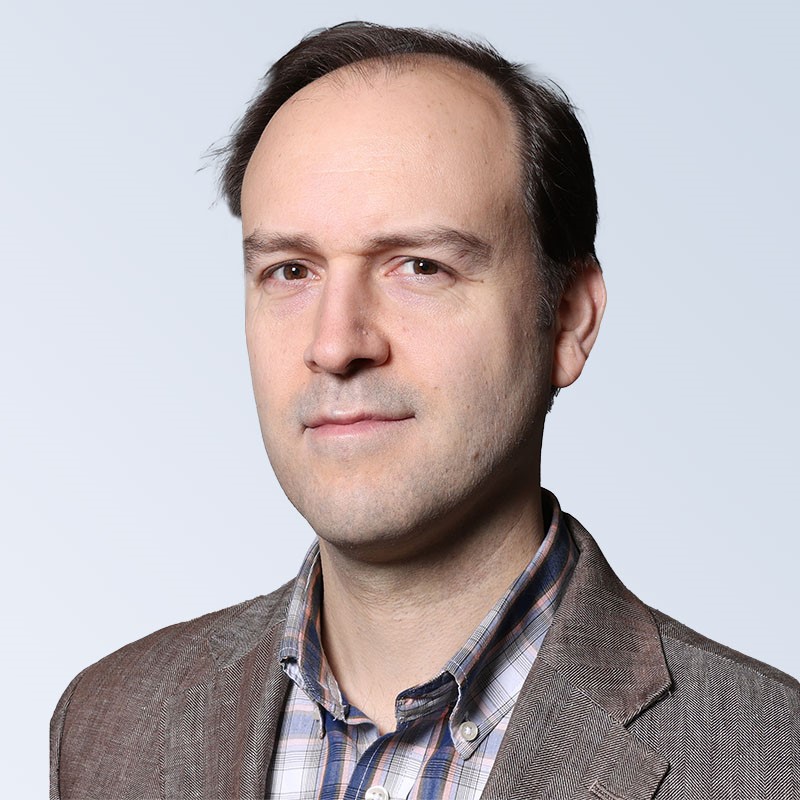
Guillaume Obozinski graduated with a PhD in Statistics from UC Berkeley in 2009. He did his postdoc and held until 2012 a researcher position in the Willow and Sierra teams at INRIA and Ecole Normale Supérieure in Paris. He was then Research Faculty at Ecole des Ponts ParisTech until 2018. Guillaume has broad interests in statistics and machine learning and worked over time on sparse modeling, optimization for large scale learning, graphical models, relational learning and semantic embeddings, with applications in various domains from computational biology to computer vision.
PI | Partners:
EPFL, Particle Accelerator Physics Laboratory:
- Dr. Tatiana Pieloni
- Dr. Michael Schenk
- Loic Coyle
description
Motivation
The goal of the project is to apply ML techniques to increase the performance of the accelerators. In collaboration with the LHC Operation groups, we aim to evaluate automatic and semi-automatic ways to model and optimize the overall collider set-up and define the strategy for the operational aspects of future projects (i.e., HL-LHC and FCC). The main goal is to get a deeper understanding of the physics mechanisms by modeling the losses in the LHC and further optimizing the beam losses in the LHC to increase the particle collision rates. This requires developing the model of the losses and dynamic aperture (DA) in the LHC depending on the control parameters.
Proposed Approach / Solution
The models of the particle losses in the LHC which depend only on the instantaneous values of control parameters do not generalize well to unseen data. We propose to model the losses time series depending on previously observed control parameters. Using a standard reparametrization, we reformulate the model as a Kalman Filter (KF) which allows for a flexible and efficient estimation procedure (Figure 1). For modeling the particle stability related to DA based on the simulated LHC data, it was proposed to use convolutional generative adversarial networks (GAN). The loss prediction was obtained from the estimated number of survived particles predicted by the network (Figure 2).
Impact
Understanding the influence of control parameters on the losses is extremely important to improve the operation, performance, and future design of accelerators. Our models based on machine data are a valuable addition to numerical models of particle losses, which can boost and improve the understanding of particle losses and help in the design of future colliders.


Presentation
Gallery
Annexe
Publications
- Schenk, M., Coyle, L., Pieloni, T., Obozinski, G., Giovannozzi, M., Mereghetti, A., & Krymova, E. (2021). JACoW: Modeling Particle Stability Plots for Accelerator Optimization Using Adaptive Sampling. JACoW IPAC, 2021, 1923-1926. https://doi.org/10.18429/JACoW-IPAC2021-TUPAB216
- Coyle, L., Pieloni, T., Solfaroli Camillocci, M., Obozinski, G., Schenk, M., Buffat, X., Krymova, E., Wenninger, J. and Blanc, F. (2021). JACoW: Detection and Classification of Collective Beam Behaviour in the LHC. JACoW IPAC, 2021, 4318-4321. https://doi.org/10.18429/JACoW-IPAC2021-THPAB260
- Krymova E., Obozinski, G., Schenk, M., Coyle, L., Pieloni, T. (2023). Data-driven modeling of beam loss in the LHC. Frontiers in Physics, 2022, 10. https://doi.org/10.3389/fphy.2022.960963
- Krymova E., Obozinski, G., Schenk, M., Coyle, L., Pieloni, T. (2022). Data-driven modeling of beam loss in the LHC [Data set]. Zenodo. https://doi.org/10.5281/zenodo.7305102
Additional resources
Bibliography
- G. Apollinari et al. (including T. Pieloni) “High-Luminosity Large Hadron Collider (HL- LHC): Preliminary Design Report - Chapter 2: Machine Layout and Performances” https://cds.cern.ch/record/2116337
Publications
Related Pages
More projects
STIMO
News
Latest news


Smartair | An active learning algorithm for real-time acquisition and regression of flow field data
Smartair | An active learning algorithm for real-time acquisition and regression of flow field data
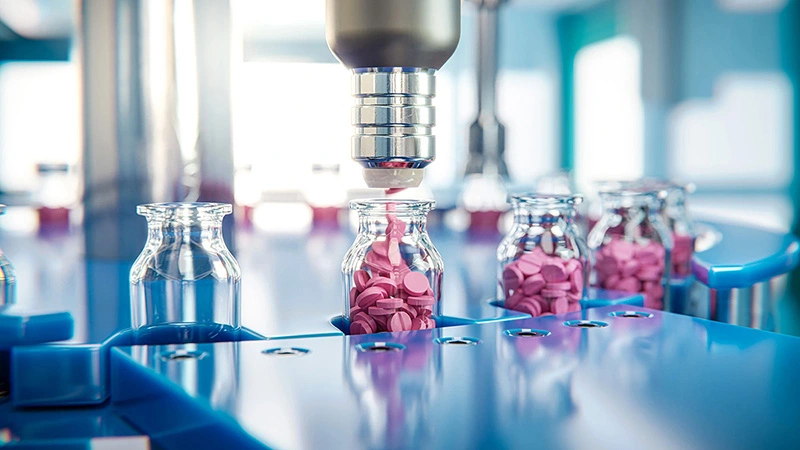
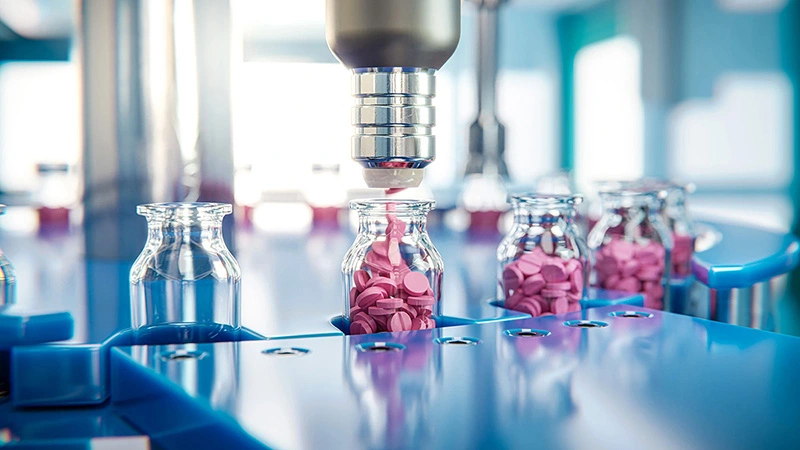
The Promise of AI in Pharmaceutical Manufacturing
The Promise of AI in Pharmaceutical Manufacturing


Efficient and scalable graph generation through iterative local expansion
Efficient and scalable graph generation through iterative local expansion
Contact us
Let’s talk Data Science
Do you need our services or expertise?
Contact us for your next Data Science project!