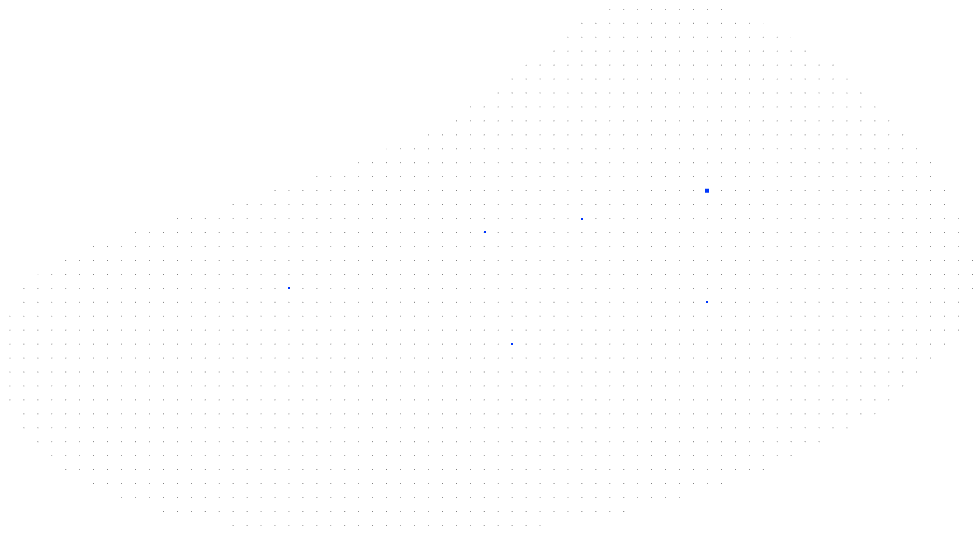
PACMAN HIPA
Particle Accelerators and Machine Learning
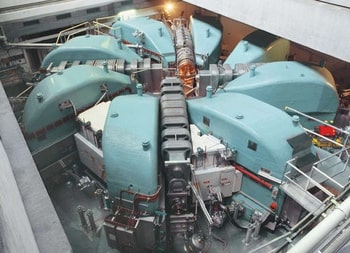
Abstract
The High Intensity Proton Accelerator (HIPA) at Paul Scherrer Institute (PSI) provides the primary beams to PSI’s versatile experimental facilities which in turn provide high intensity beams for research. In an accelerator control room several hundred of continuous sensor data are displayed in order to aid the operators in running the accelerator with maximal performance. We propose to bring Machine Learning (ML) to particle accelerator operation, in order to increase the performance. A more accurate parameter control based on the surrogate modelling will contribute to reliable and safe operation, and increase the accelerator efficiency. The immediate benefits will be: reducing the risks related to the high beam power by reducing the activation and beam losses, an action that will in turn, lead to fewer machine interruptions and possibly higher beam intensities. The project is likely to have a game-changing impact in how we model and operate charged particle accelerators in the near future.
People
Collaborators
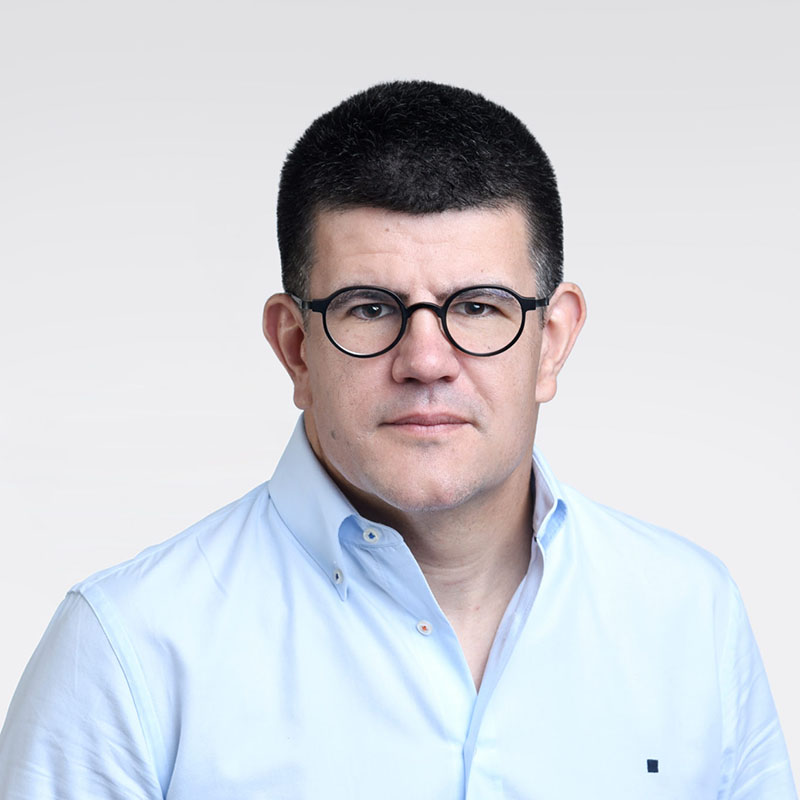
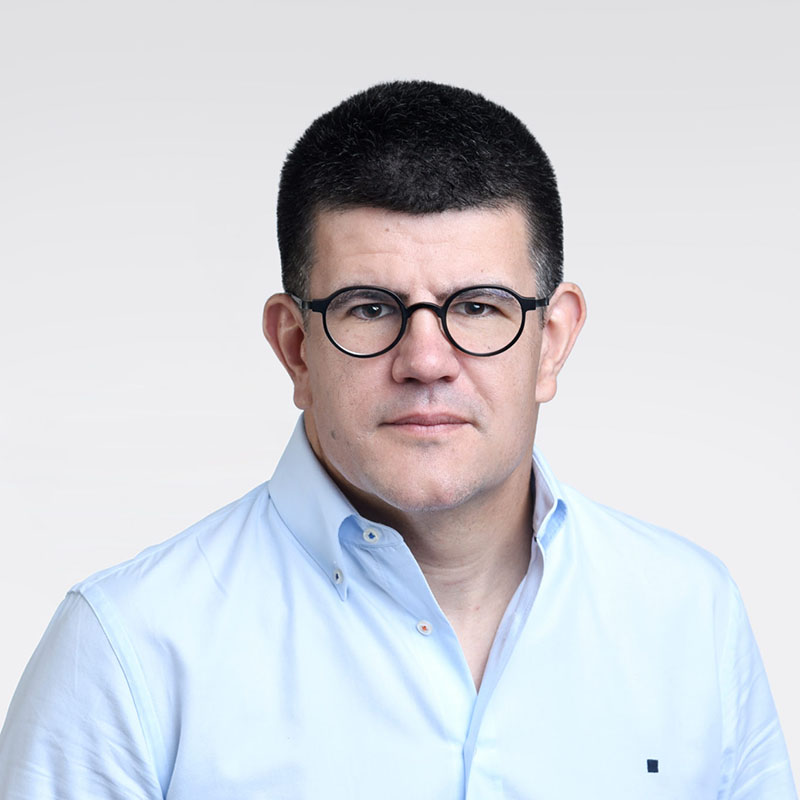
Fernando Perez-Cruz received a PhD. in Electrical Engineering from the Technical University of Madrid. He is Titular Professor in the Computer Science Department at ETH Zurich and Head of Machine Learning Research and AI at Spiden. He has been a member of the technical staff at Bell Labs and a Machine Learning Research Scientist at Amazon. Fernando has been a visiting professor at Princeton University under a Marie Curie Fellowship and an associate professor at University Carlos III in Madrid. He held positions at the Gatsby Unit (London), Max Planck Institute for Biological Cybernetics (Tuebingen), and BioWulf Technologies (New York). Fernando Perez-Cruz has served as Chief Data Scientist at the SDSC from 2018 to 2023, and Deputy Executive Director of the SDSC from 2022 to 2023
PI | Partners:
PSI, Laboratory for Scientific Computing and Modelling:
- Dr. Andreas Adelmann
- Dr. Jaime Coello de Portugal
- Sichen Li
ETH Zurich, Learning & Adaptive Systems Group:
- Prof. Andreas Krause
- Johannes Kirschner
- Mojmir Mutny
description
Motivation
The operation of high-intensity proton accelerators like HIPA at the PSI requires precise control of several hundred machine parameters to ensure efficiency, stability, and safety of the beam line. Traditional approaches rely heavily on manual oversight and classical control strategies, which may not fully exploit the rich, continuous stream of sensor data available. Integrating Machine Learning (ML) offers the potential to improve performance by minimizing beam losses, enhancing parameter control, and preventing unnecessary machine interruptions.
Proposed Approach / Solution
First, we developed a surrogate model based on beam diagnostics data to provide real-time predictions to the machine protection system. The model enables early forecasting of potential interruptions, allowing operators to take preventive actions in advance and maintain stable, uninterrupted accelerator operation. Second, we deployed an automated parameter tuning tool to minimize beam losses. Our approach is based on Safe Bayesian Optimization, a zero-order optimization algorithm that takes safety constraints into account, and in particular prevents interrupts on the accelerator during the optimization.
Impact
This work demonstrated that data-driven prediction and optimization can significantly improve the efficiency and reliability of accelerator operations. By reducing beam losses and unplanned downtimes, the surrogate model contributed to safer, more stable operation at higher beam intensities. As a result, more beam time became available for scientific experiments. The successful integration of predictive modeling marks a step toward smarter, data-driven control of complex accelerator systems.
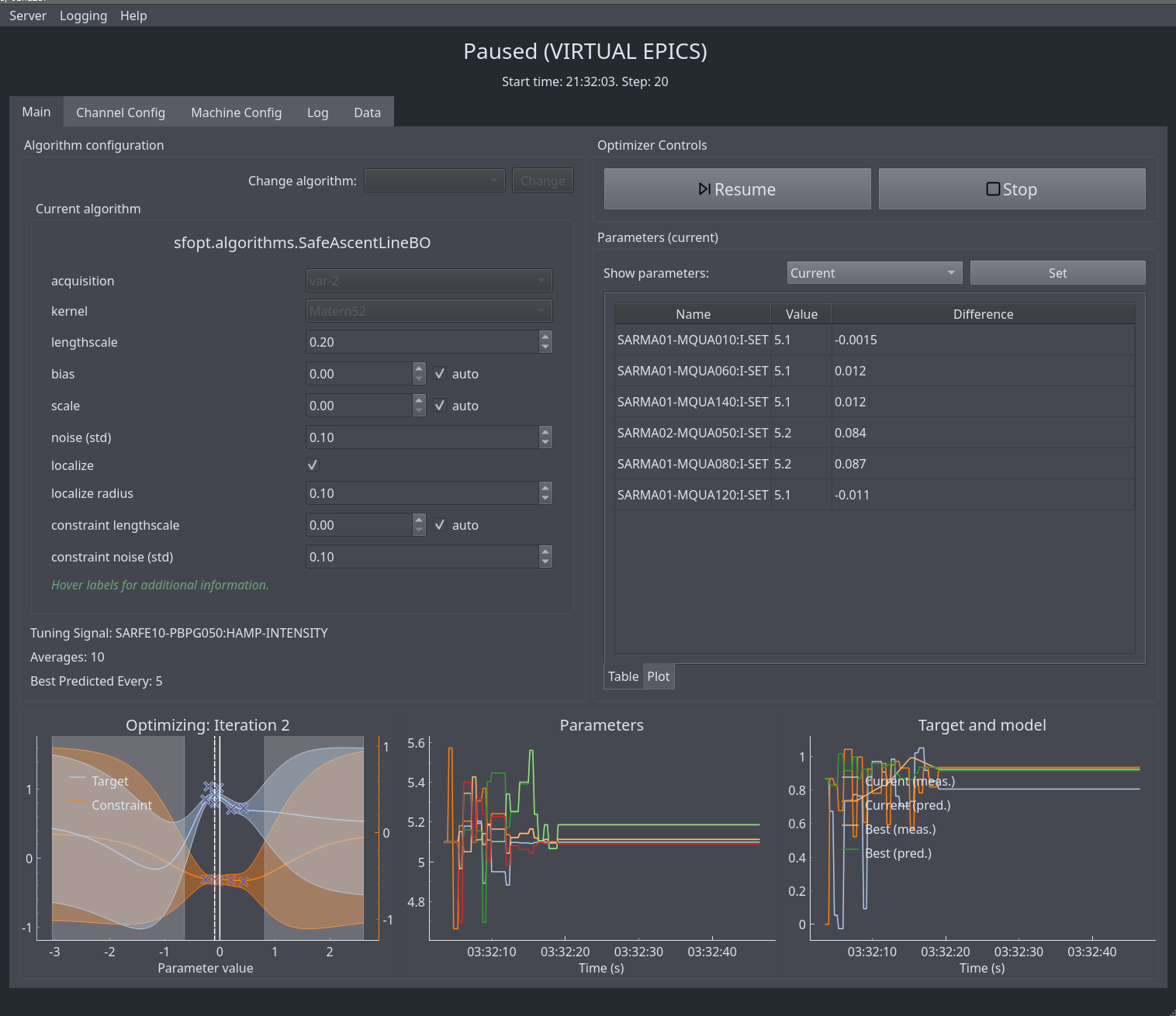
Presentation
Gallery
Annexe
Additional resources
Bibliography
Publications
Related Pages
More projects
MAGNIFY
News
Latest news
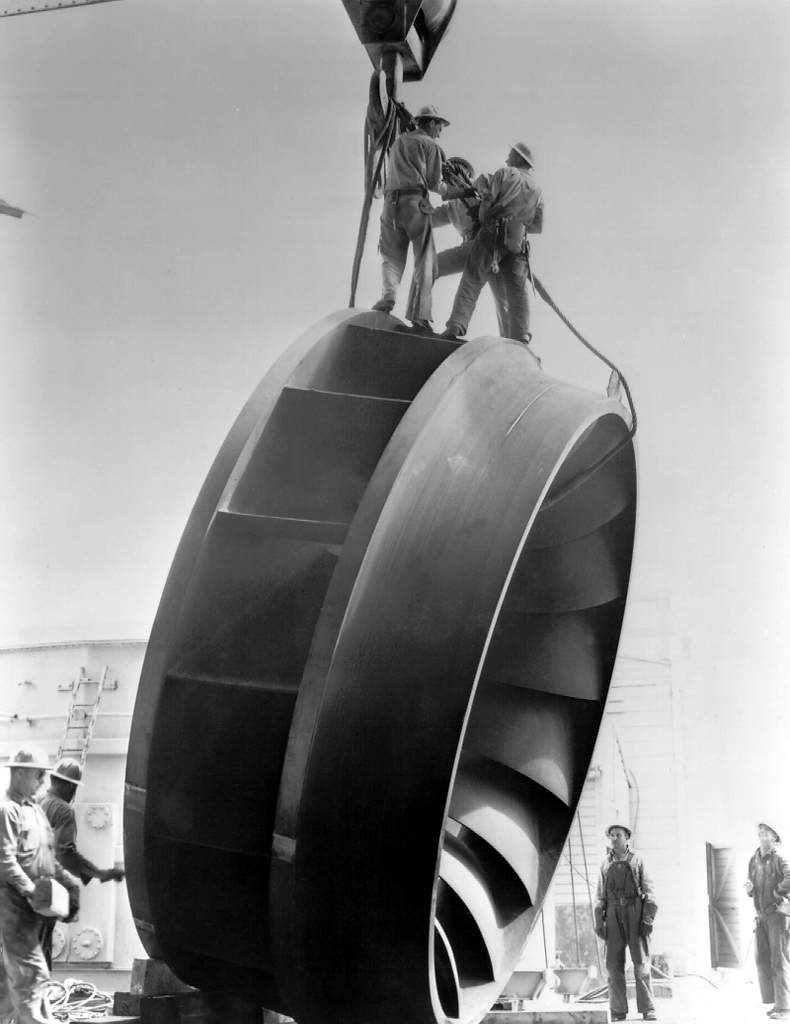
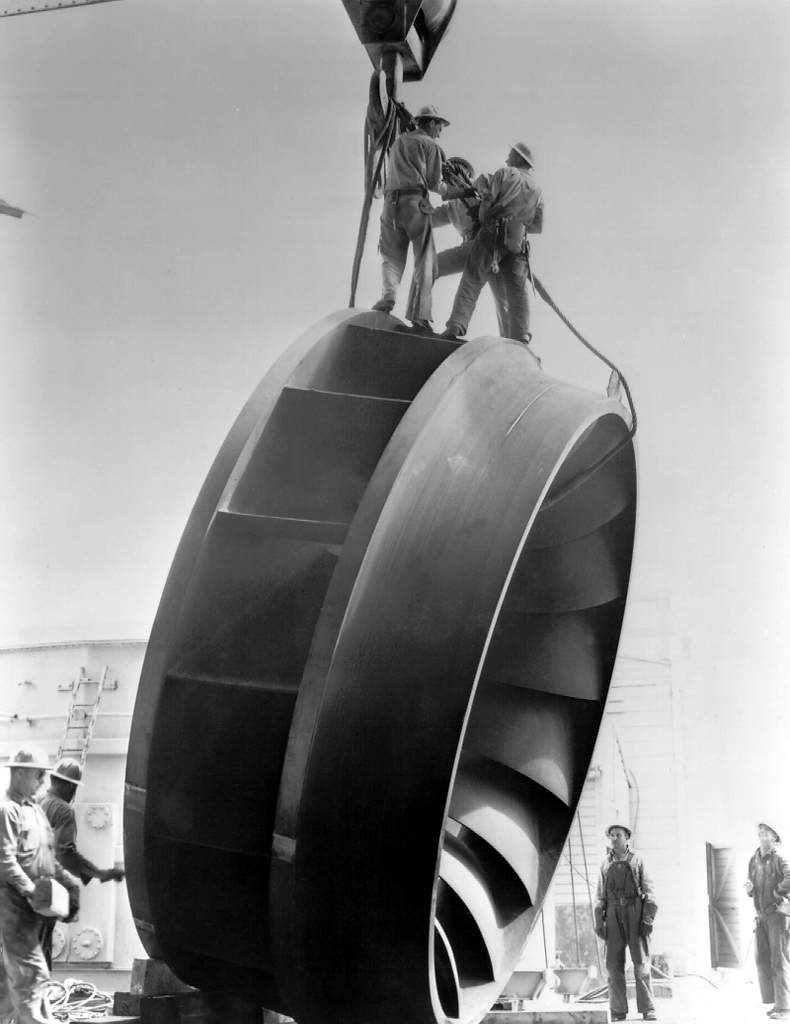
PAIRED-HYDRO | Increasing the Lifespan of Hydropower Turbines with Machine Learning
PAIRED-HYDRO | Increasing the Lifespan of Hydropower Turbines with Machine Learning


First National Calls: 50 selected projects to start in 2025
First National Calls: 50 selected projects to start in 2025
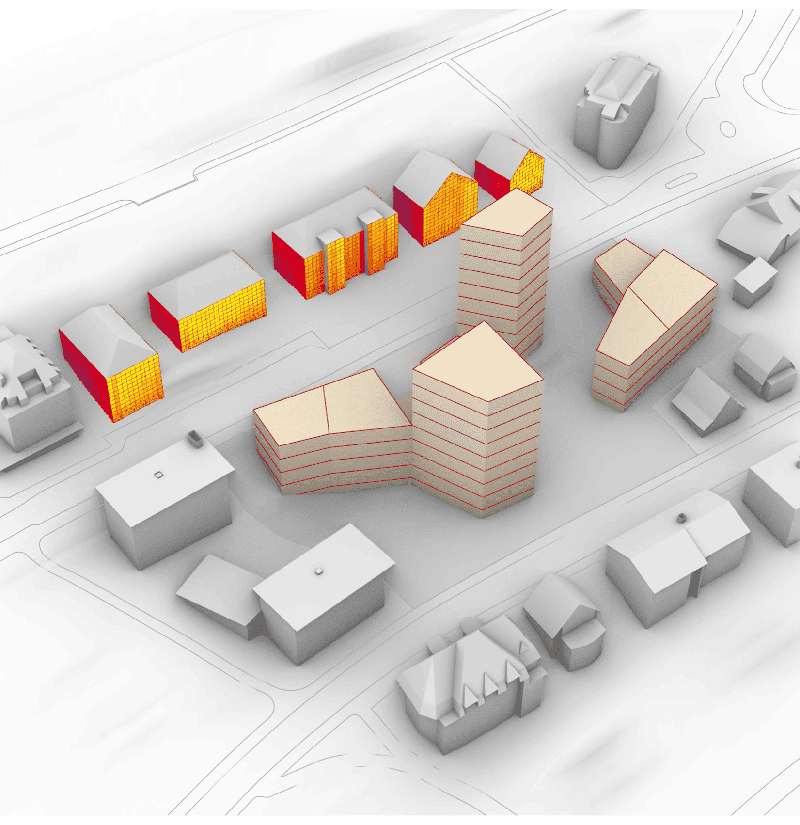
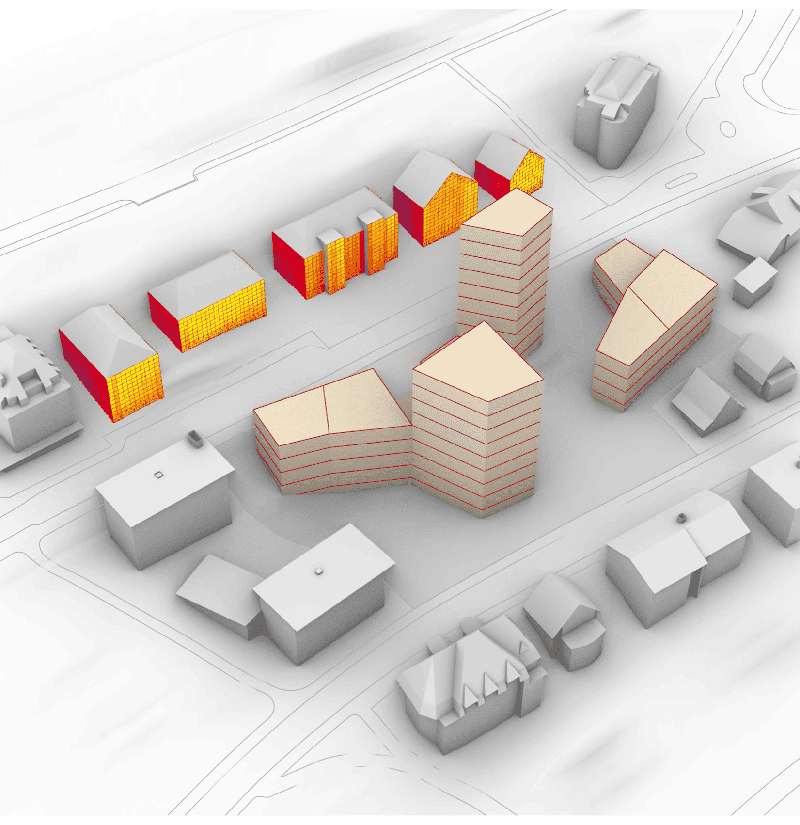
AIXD | Generative AI toolbox for architects and engineers
AIXD | Generative AI toolbox for architects and engineers
Contact us
Let’s talk Data Science
Do you need our services or expertise?
Contact us for your next Data Science project!