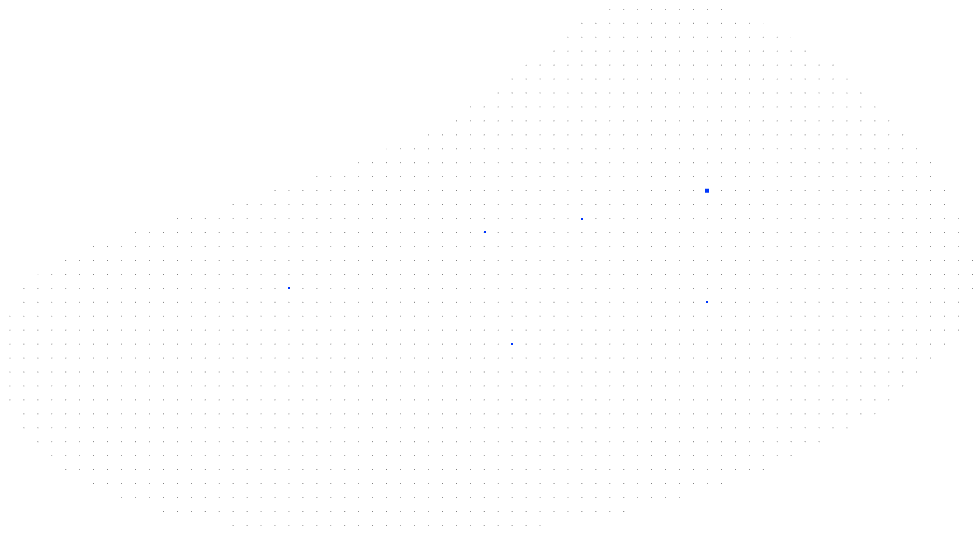
MUTIGER
MUTations, Interactions and GEne Regulation

Abstract
Non-coding mutations constitute more than 95% of all mutations; however, they remain understudied in the context of diseases such as cancer. Several studies recently documented the consequences of non-coding mutations in cancer on the activity of regulatory elements linked to changes in the expression of cancer-related genes. Nevertheless, a comprehensive study that would evaluate the effects of non-coding mutations on the 3D structure of enhancer- promoter looping across various types of cancer is currently missing. Moreover, there is no available computational approach to predict and evaluate the effects of large structural variants (e.g., translocations or large genomic duplications and deletions) on enhancer-promoter looping, and consecutively gene expression, when the gene itself is not affected by the rearrangement.
This project's primary goal is to build a computational approach to reliably predict the effect of each non-coding genomic variant in a tumor genome in a cell-type-specific manner via explicitly modeling changes in the activity of regulatory elements and 3D chromatin structure.
Overall, this work will further investigate the role of non-coding variation including structural rearrangements in cancer development making a specific emphasis on variants affecting DNA 3D structure and activity of regulatory elements. We are confident that the application of our method will allow extracting a very small number of truly functional non-coding variants that affect the expression of neighboring genes. Using our analysis in hundreds of available cancer whole-genome sequence samples, we aim to improve our understanding of cancer drivers, further elucidating oncogenic mechanisms in human cancers.
People
Collaborators


Lin Zhang joined the SDSC as a senior data scientist. She completed her PhD at ETH Zurich in 2023, with a focus on simulation in medical imaging with deep learning. Before that, she obtained a bachelor degree in electrical engineering from the Technical University Munich, and a master degree in biomedical engineering from ETH Zurich. Her research interests include deep generative models, domain adaptation and applications of machine learning in healthcare.


Till obtained his Bachelor's and his Master's degrees in Physics at ETH Zurich in 2020 and 2022 respectively. Over the course of his studies and by applying computational methods to problems in physics, he developed a fascination with data science and machine learning. Having joined the SDSC in July 2022, Till seeks to gain an impression of academia outside the physics domain, as well as a deeper understanding of data-driven problem-solving. In his work, Till is using Deep Learning methods to lengthen the lifespan of hydropower turbines and to better understand the effect of mutations in non-coding regions of the DNA.
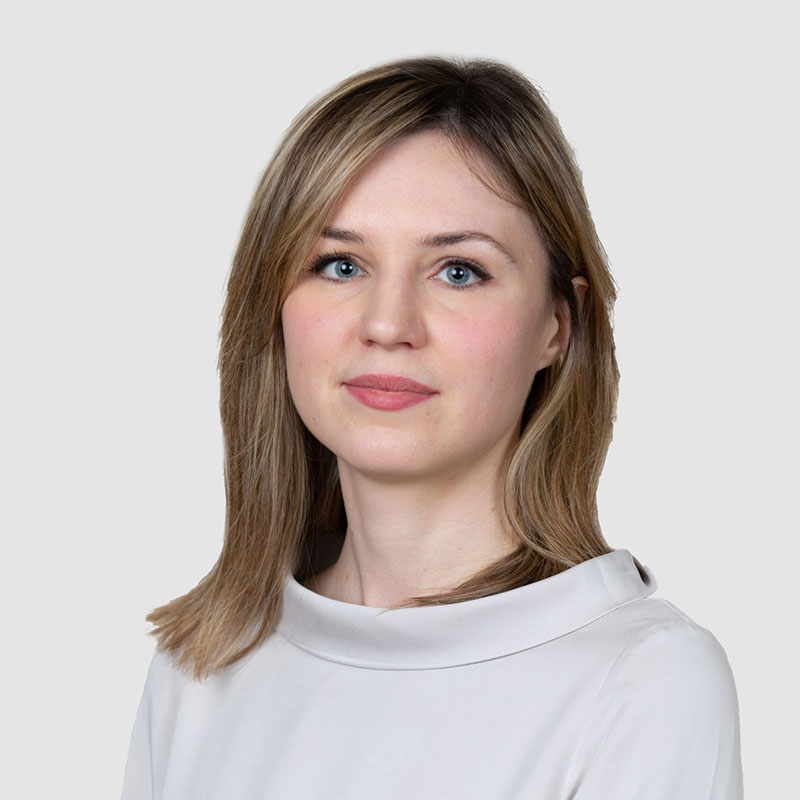
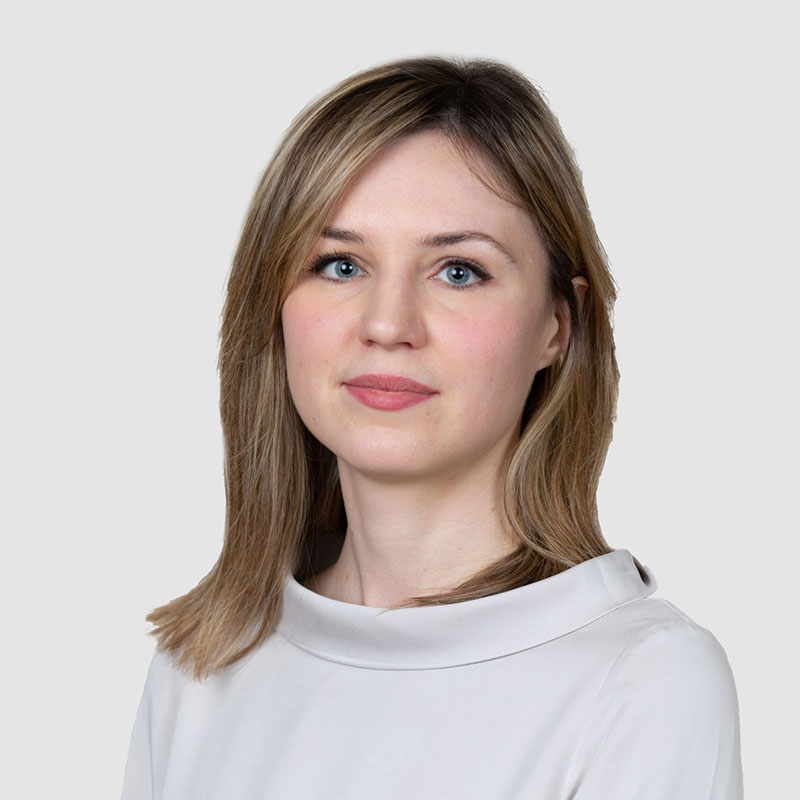
Ekaterina received her PhD in Computer Science from Moscow Institute for Physics and Technology, Russia. Afterwards, she worked as a researcher at the Institute for Information Transmission Problems in Moscow and later as a postdoctoral researcher in the Stochastic Group at the Faculty of Mathematics at University Duisburg-Essen, Germany. She has experience with various applied projects on signal processing, predictive modelling, macroeconomic modelling and forecasting, and social network analysis. She joined the SDSC in November 2019. Her interests include machine learning, non-parametric statistical estimation, structural adaptive inference, and Bayesian modelling.
PI | Partners:
description
Motivation
Non-coding mutations remain under-explored in cancer research. There is a lack of comprehensive study on how these mutations affect the 3D structure of enhancer-promoter looping across different cancer types. While experimental methods are available, they are expensive and constrained by technical limitations, making them impractical for high-throughput analysis. This project aims to develop computational approaches to assess the effects of non-coding variants and large structural variants in a cell-type-specific manner by explicitly modelling alterations in regulatory element activity and 3D chromatin structure caused by genomic variations.
Proposed Approach / Solution
SDSC is engaged in the development of computational methods for prediction of cell-type-specific effects of non-coding variants based on unmatched open chromatin data and DNA sequence. The objective is to create a user-friendly tool capable of predicting the influence of non-coding variants on regulatory element activity, thereby how it affects the expression of target genes.
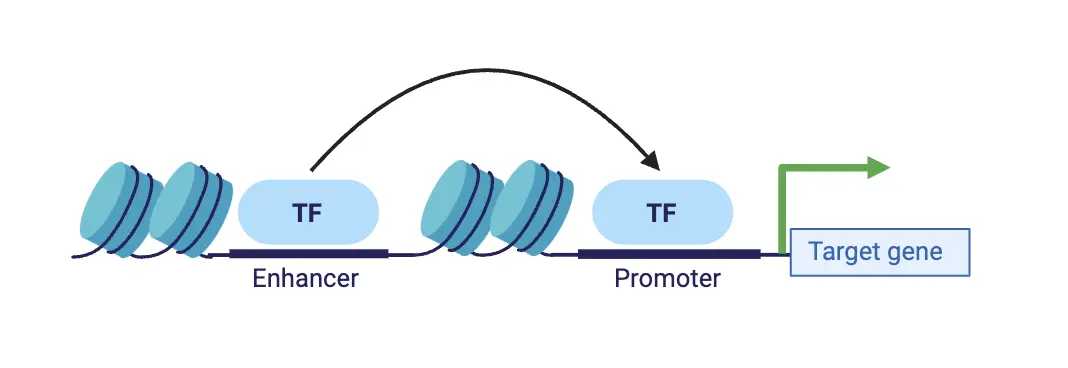
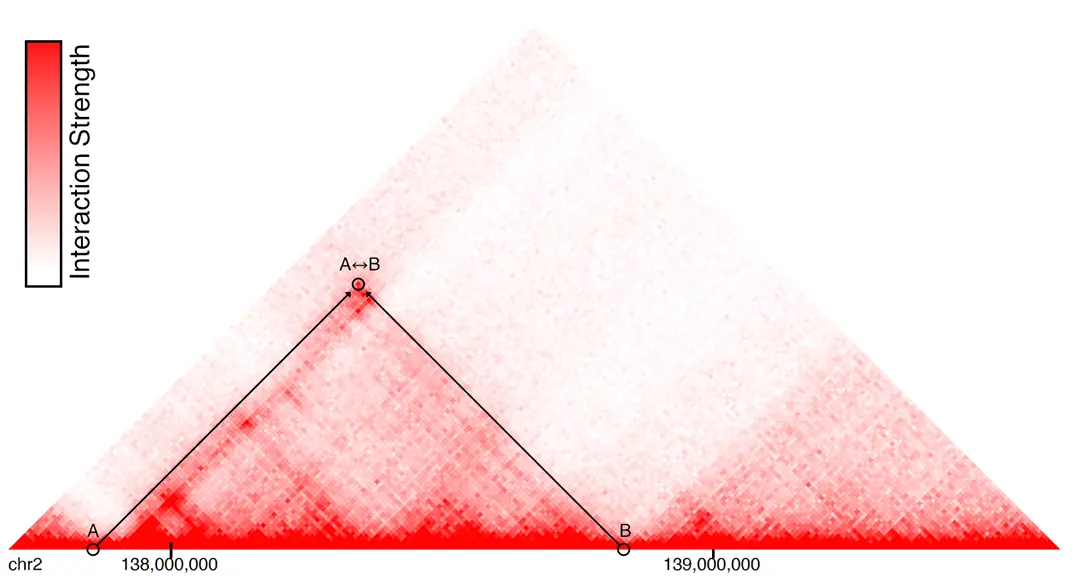
Impact
This project aims to delve deeper into the role of non-coding mutations and structural variants in cancer development. The methodology devised in this project offers a valuable tool for identifying truly functional non-coding mutations and structural variants that influence the expression of cancer-related genes. This advancement can significantly deepen our understanding of oncogenic mechanisms in human cancers.
Presentation
Gallery
Annexe
Additional resources
Bibliography
- Tan, J., Shenker-Tauris, N., Rodriguez-Hernaez, J. et al. Cell-type-specific prediction of 3D chromatin organization enables high-throughput in silico genetic screening. Nat Biotechnol 41, 1140–1150 (2023). https://doi.org/10.1038/s41587-022-01612-8
- Fudenberg, G., Kelley, D.R. & Pollard, K.S. Predicting 3D genome folding from DNA sequence with Akita. Nat Methods 17, 1111–1117 (2020). https://doi.org/10.1038/s41592-020-0958-x
- Kelley DR (2020) Cross-species regulatory sequence activity prediction. PLOS Computational Biology 16(7): e1008050. Cross-species regulatory sequence activity prediction
- Avsec, Ž., Agarwal, V., Visentin, D. et al. Effective gene expression prediction from sequence by integrating long-range interactions. Nat Methods 18, 1196–1203 (2021). https://doi.org/10.1038/s41592-021-01252-x
Publications
Related Pages
More projects
ML-L3DNDT
BioDetect
News
Latest news


Smartair | An active learning algorithm for real-time acquisition and regression of flow field data
Smartair | An active learning algorithm for real-time acquisition and regression of flow field data
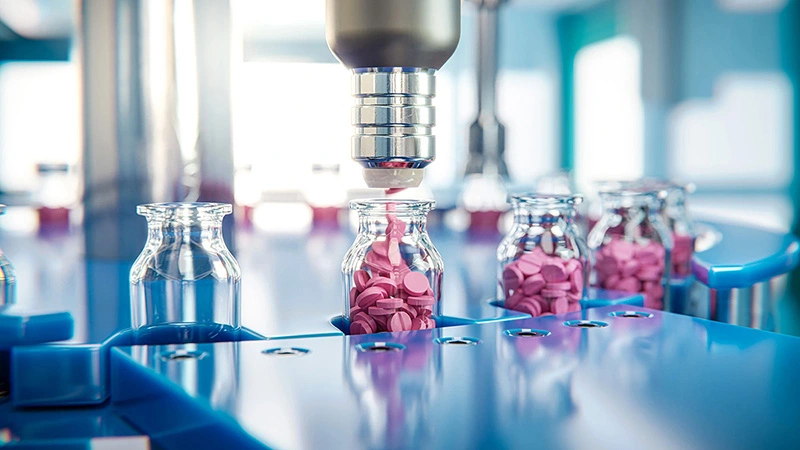
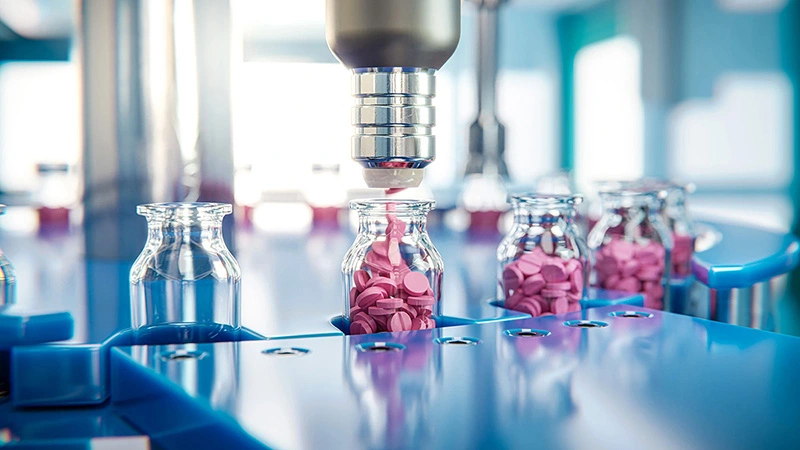
The Promise of AI in Pharmaceutical Manufacturing
The Promise of AI in Pharmaceutical Manufacturing


Efficient and scalable graph generation through iterative local expansion
Efficient and scalable graph generation through iterative local expansion
Contact us
Let’s talk Data Science
Do you need our services or expertise?
Contact us for your next Data Science project!