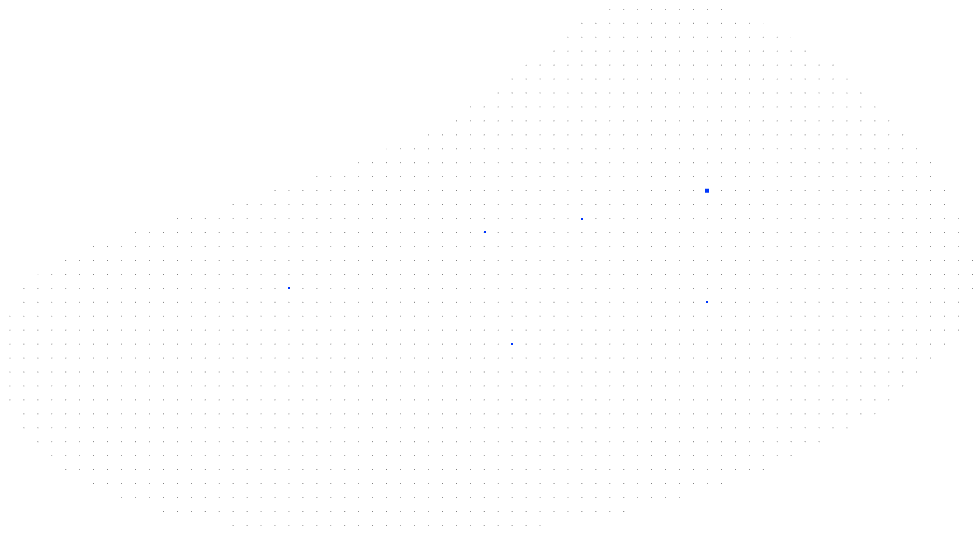
MLATEM
Machine Learning tools for Analytical Transmission Electron Microscopy

Abstract
Modern transmission electron microscopes are equipped with aberration correctors and high-resolution spectrometers, which enable the spatially-resolved chemical analysis of an unlimited variety of materials on the nanometric or even atomic length scales. The ultimate ambition of a researcher is to segment and accurately quantify the analytical data recorded from such the sample, in order to precisely characterize the spatial distribution and nature of each of its phases. However, currently, such an aim can only be realized in a limited fraction of cases, owing to factors including signal convolutions and non-linear backgrounds, detector and signal noise characteristics, and segmentation challenges from the projection effects.
The aim of this project is to address and resolve these deficits, by innovating a radically new approach to the data analysis.
The aim of our project is to develop an integrated approach where all of these elements are synergistically coupled together: prior information; a directed “dictionary learning” approach using the optimal data science tools; and physical model-based quantification. With interactive feedback between these elements, it will be possible to achieve a step change in the quality of data analysis. A successful project will therefore significantly increase the ability of the researcher to leverage their data for obtaining new scientific insights and technological advances across a wide range of fields.
People
Collaborators
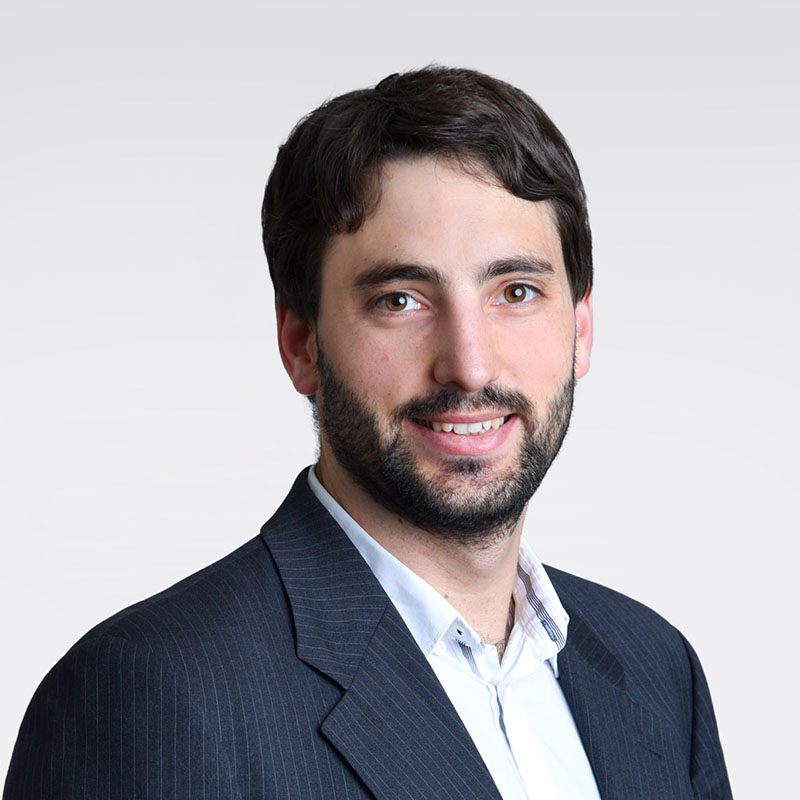
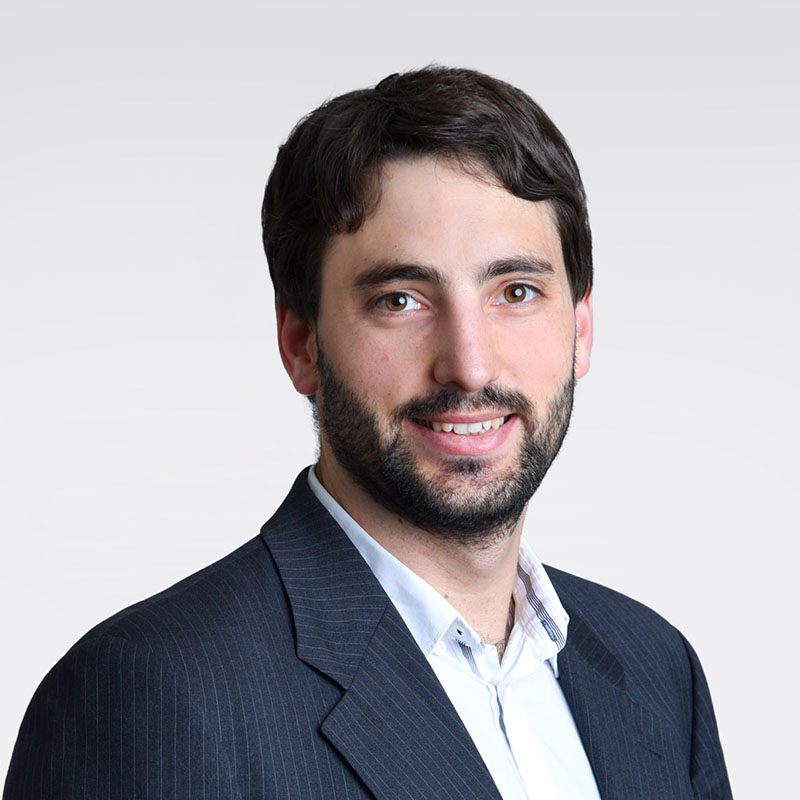
After finishing his Master in electrical engineering at the Ecole Fédérale de Lausanne (EPFL), Nathanaël worked as a researcher in the Acoustic Research Institute (ARI) in Vienna. In 2013, he returned to EPFL for a PhD, where he specialized himself in different fields of data science: signal processing, machine learning, graph theory and optimization. Furthermore, he created two open source libraries for optimization (UNLocBoX) and graph signal processing (GSPBOX). Since 2017, Nathanaël Perraudin is a Research Data Scientist at the Swiss Data Science Center in the ETH Zurich. He focuses on different aspects of deep learning in the area of generative models (VAE and GAN), recursive architectures and convolutional neural network for irregular domains. Outside office hours, he is passionate by tango dancing, tandem bike touring, skiing and rock climbing.
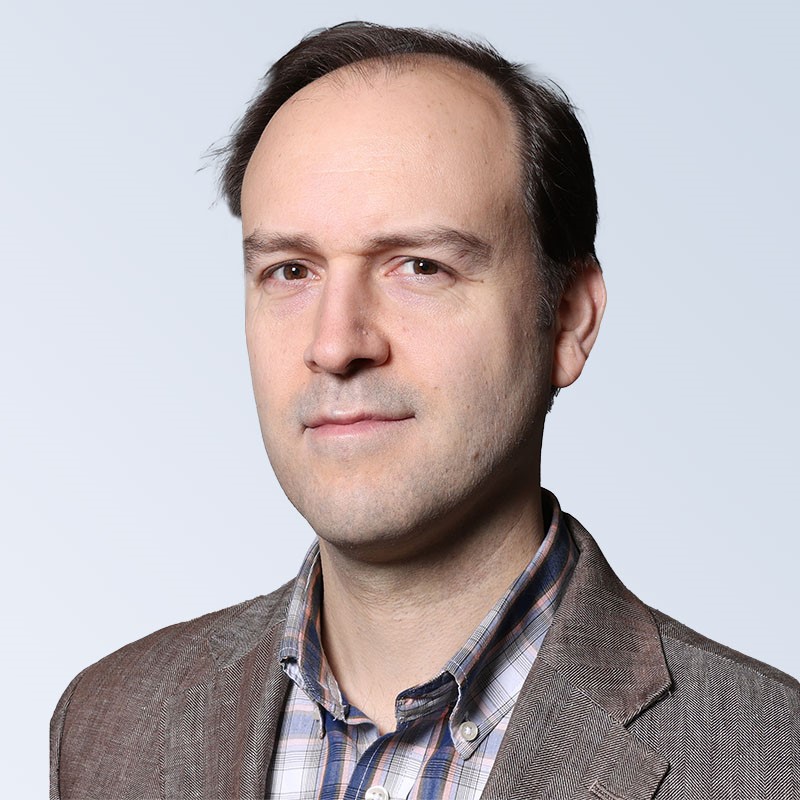
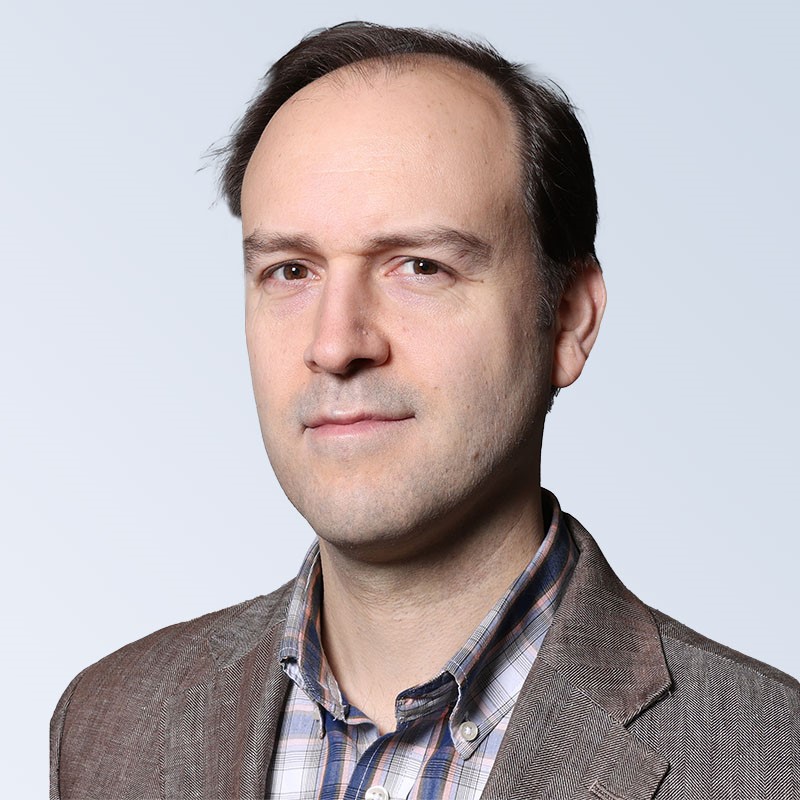
Guillaume Obozinski graduated with a PhD in Statistics from UC Berkeley in 2009. He did his postdoc and held until 2012 a researcher position in the Willow and Sierra teams at INRIA and Ecole Normale Supérieure in Paris. He was then Research Faculty at Ecole des Ponts ParisTech until 2018. Guillaume has broad interests in statistics and machine learning and worked over time on sparse modeling, optimization for large scale learning, graphical models, relational learning and semantic embeddings, with applications in various domains from computational biology to computer vision.
PI | Partners:
EPFL, Electron Spectrometry and Microscopy Laboratory:
- Prof. Cécile Hébert
- Dr. Adrien Teurtrie
- Dr. Duncan Alexander
- Hui Chen
description
Problem:
The spectroscopic data obtain in scanning transmission electron microscopes (STEM) are in most cases not straightforward to analyse because of noise and mixed features (both spectrally and spatially). The current state-of-the-art in this community in terms of data analysis is limited to basic ML algorithms such as PCA or NMF which, in general, do not retrieve the original physical features of the observed sample. In the MLATEM project we aim at designing a physics-guided ML algorithm which will retrieve the physically-correct features of the observed sample. In that perspective, the SDSC will provide an expertise on cutting-edge ML techniques to develop this algorithm while the collaborating team will provide domain knowledge.
Goal:
Our goal is to develop a new standard algorithm for the electromicroscopy community.
Presentation
Gallery
Annexe
Additional resources
Bibliography
- D.D. Lee, H.S. Seung, Learning the parts of objects by non-negative matrix factorization, Nature. 401 (1999) 788.
- Trebbia, P., Bonnet, N., 1990. Ultramicroscopy 34, 165–178 & Bonnet, N., Brun, N., Colliex, C., 1999.
- K.J. Dudeck, M. Couillard, S. Lazar, C. Dwyer, G.A. Botton, Quantitative statistical analysis, optimization and noise reduction of atomic resolved electron energy loss spectrum images, Micron. 43 (2012) 57–67.
- A.B. Yankovich, C. Zhang, A. Oh, T.J.A. Slater, F. Azough, R. Freer, S.J. Haigh,
- R. Willett, P.M. Voyles, Non-rigid registration and non-local principle component analysis to improve electron microscopy spectrum images, Nanotechnology. 27 (2016) 364001.
- B.H. Martineau, D.N. Johnstone, A.T.J. van Helvoort, P.A. Midgley, A.S. Eggeman, Unsupervised machine learning applied to scanning precession electron diffraction data, Adv. Struct. Chem. Imaging. 5 (2019) 3.
- M. Shiga, K. Tatsumi, S. Muto, K. Tsuda, Y. Yamamoto, T. Mori, T. Tanji, Sparse modeling of EELS and EDX spectral imaging data by nonnegative matrix factorization, Ultramicroscopy. 170 (2016) 43–59.
- Verbeeck, J. and S. Van Aert (2004), 'Model based quantification of EELS spectra', Ultramicroscopy, 101 (2-4), 207-224.
Publications
Related Pages
More projects
MAGNIFY
News
Latest news


First National Calls: 50 selected projects to start in 2025
First National Calls: 50 selected projects to start in 2025
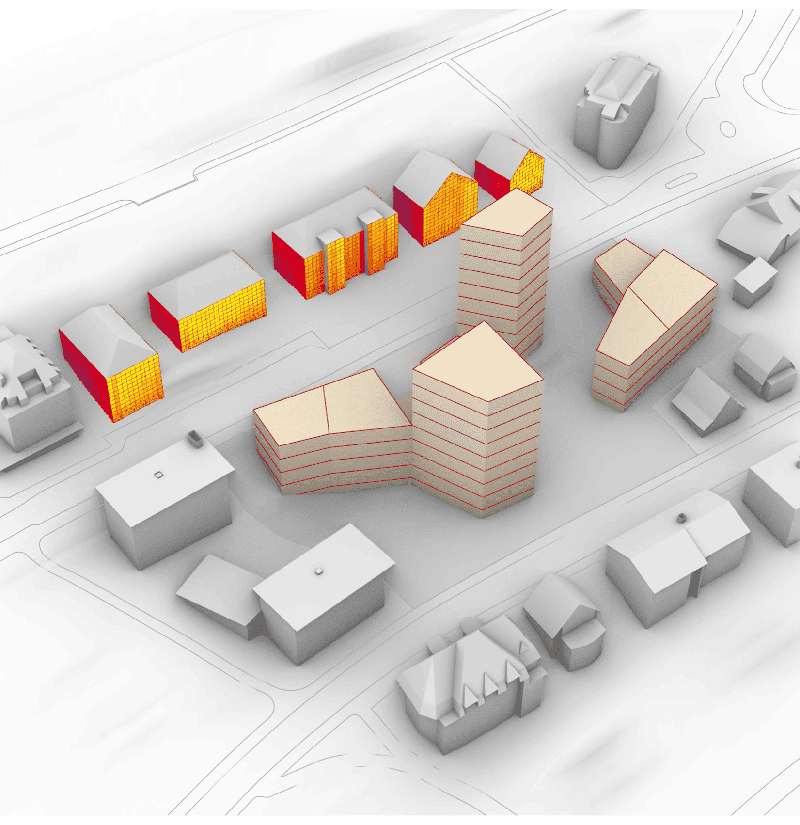
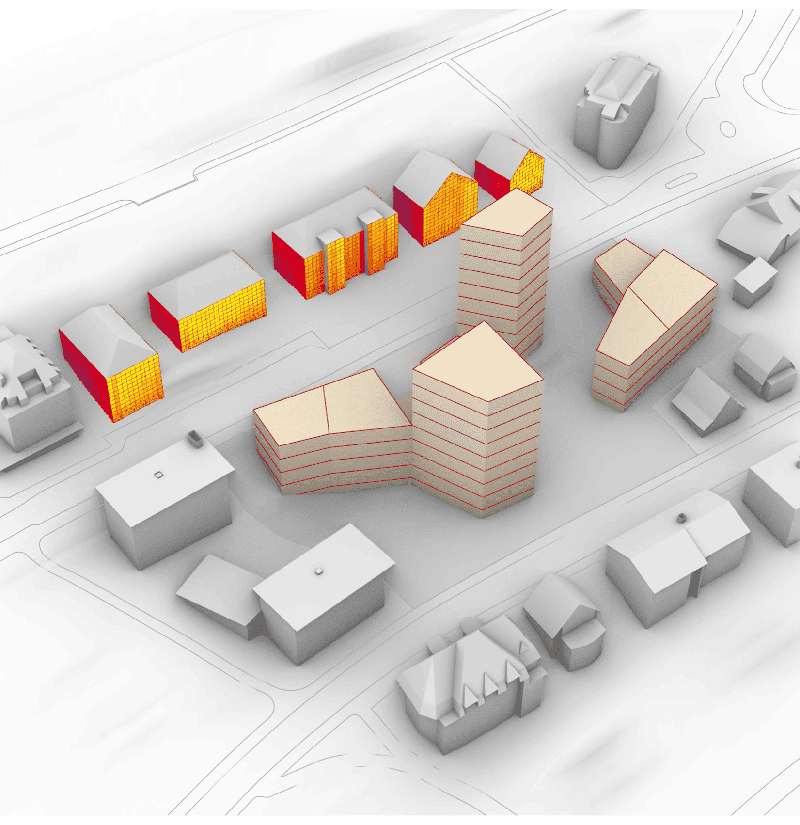
AIXD | Generative AI toolbox for architects and engineers
AIXD | Generative AI toolbox for architects and engineers


Smartair | An active learning algorithm for real-time acquisition and regression of flow field data
Smartair | An active learning algorithm for real-time acquisition and regression of flow field data
Contact us
Let’s talk Data Science
Do you need our services or expertise?
Contact us for your next Data Science project!