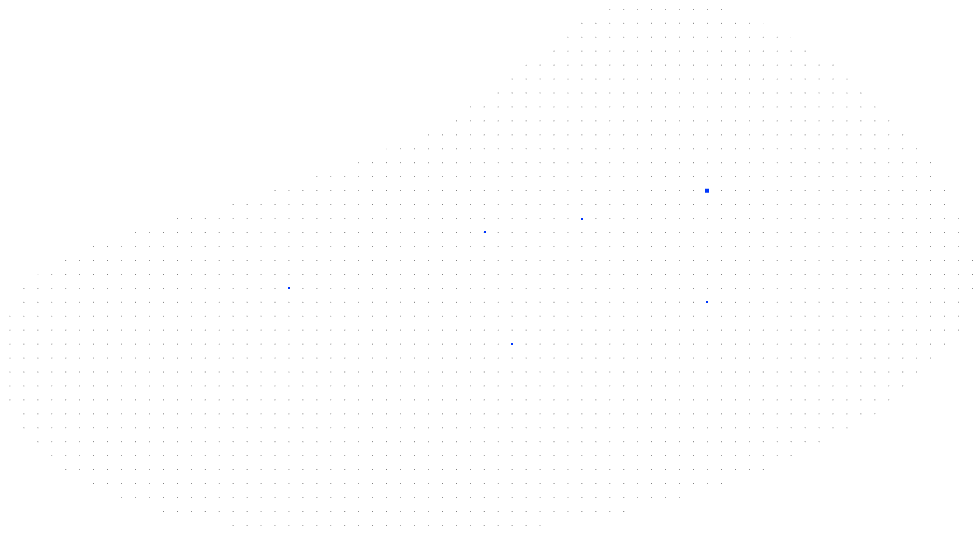
ML4FCC
Machine Learning for the Future Circular Collider Design

Abstract
The design and operation of the Future Circular Collider at the Council of the European Organization for Nuclear Research (CERN) as a precision instrument for particle physics is an exciting Big Data and Machine Learning opportunity. Accelerator performance is characterized by the dynamic aperture (DA), which represents the size of the area in phase space where the beam particles feature stable behavior under long-term tracking. Particles located in the chaotic and unstable areas will be lost from the beam and will reduce its lifetime, so measuring the DA is vital. So far, this was done with manual adjustment of hundreds of control parameters and particle tracking simulations, which are computationally slow and expensive. We automated the optimization of the DA by developing a data-driven algorithm for its prediction and accurate quantification of the uncertainty.
People
Collaborators
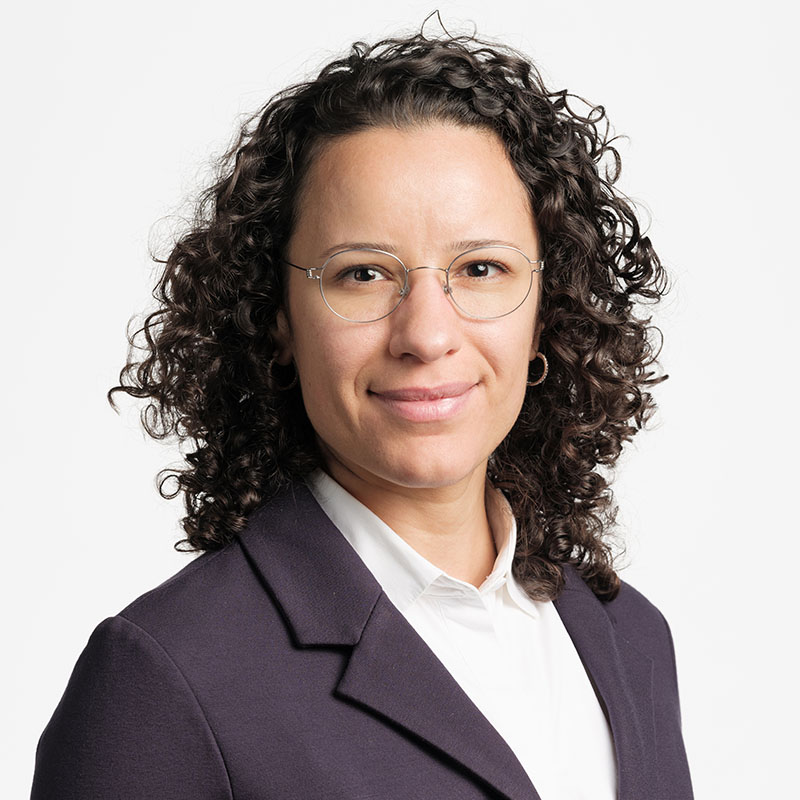
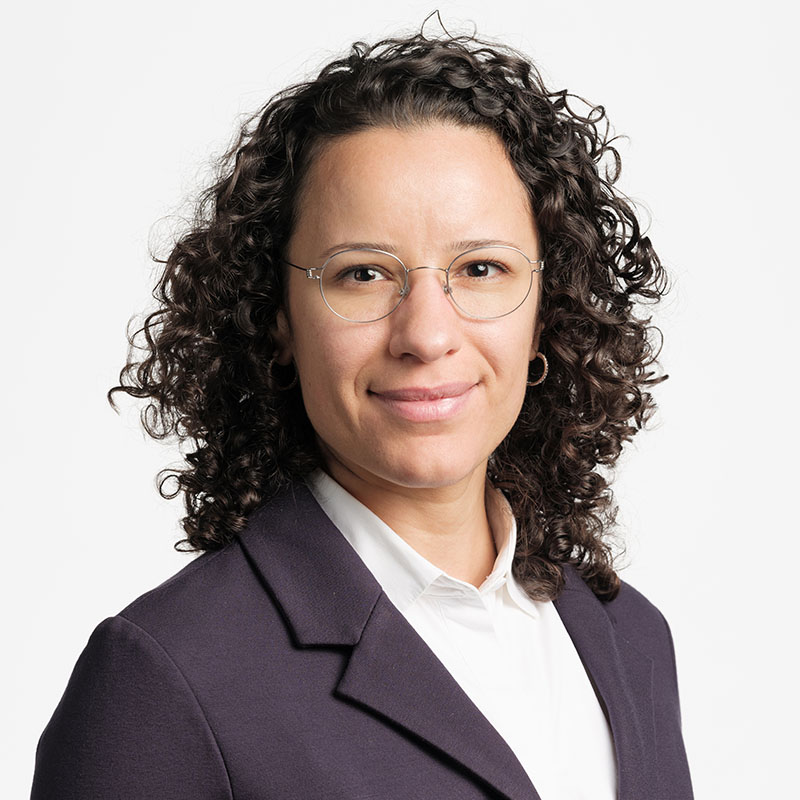
Yousra studied Mathematics and Computational Statistics. She did a PhD in Statistical Learning followed by a post-doc both at EPFL, where she developed empirical Bayes methods for automatic L2 regularization problems in smooth regression for big data. Before joining SDSC, she worked at SIB/UNIL, where she developed a statistical optimization solution to the parent-of-origin identification problem in human genetics. Her technical expertise includes supervised learning, numerical optimization for machine learning, statistical modeling and methodology, high-performance and distributed computing for big data, Bayesian computation and time series analysis. She worked on applied problems in quantitative finance and environmental sciences.
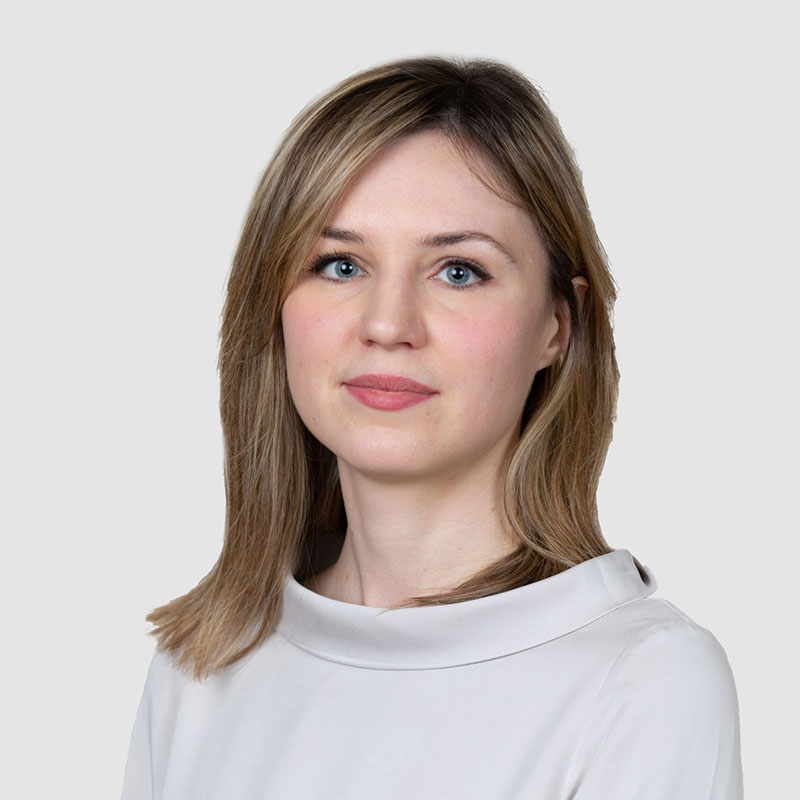
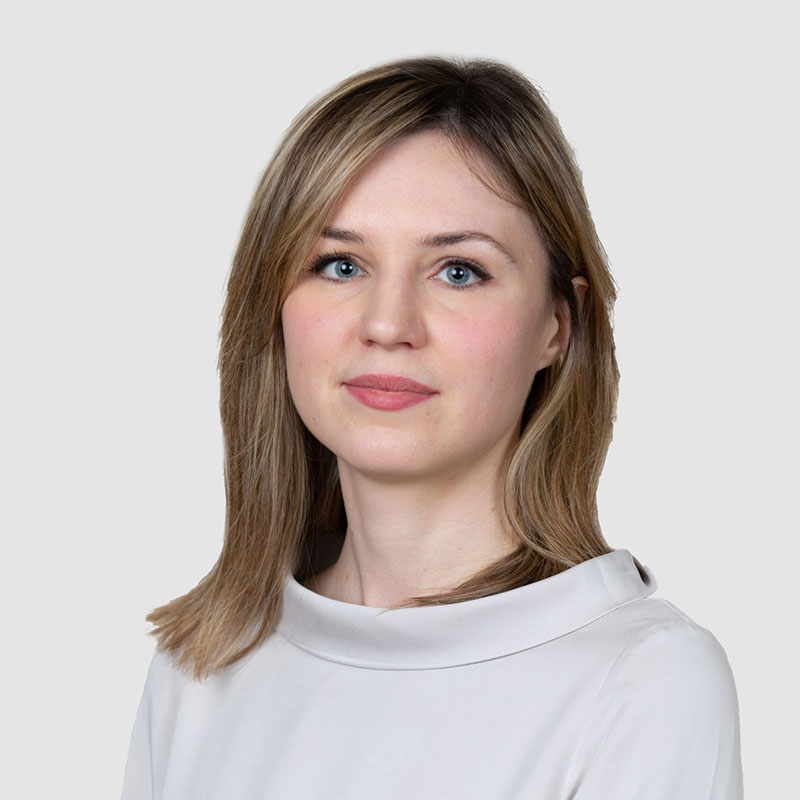
Ekaterina received her PhD in Computer Science from Moscow Institute for Physics and Technology, Russia. Afterwards, she worked as a researcher at the Institute for Information Transmission Problems in Moscow and later as a postdoctoral researcher in the Stochastic Group at the Faculty of Mathematics at University Duisburg-Essen, Germany. She has experience with various applied projects on signal processing, predictive modelling, macroeconomic modelling and forecasting, and social network analysis. She joined the SDSC in November 2019. Her interests include machine learning, non-parametric statistical estimation, structural adaptive inference, and Bayesian modelling.
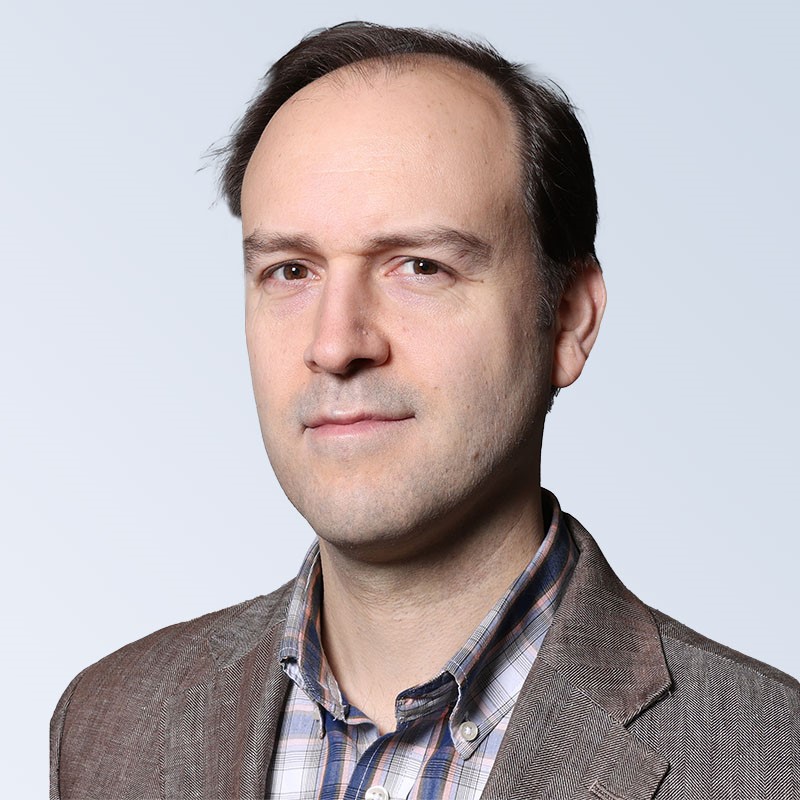
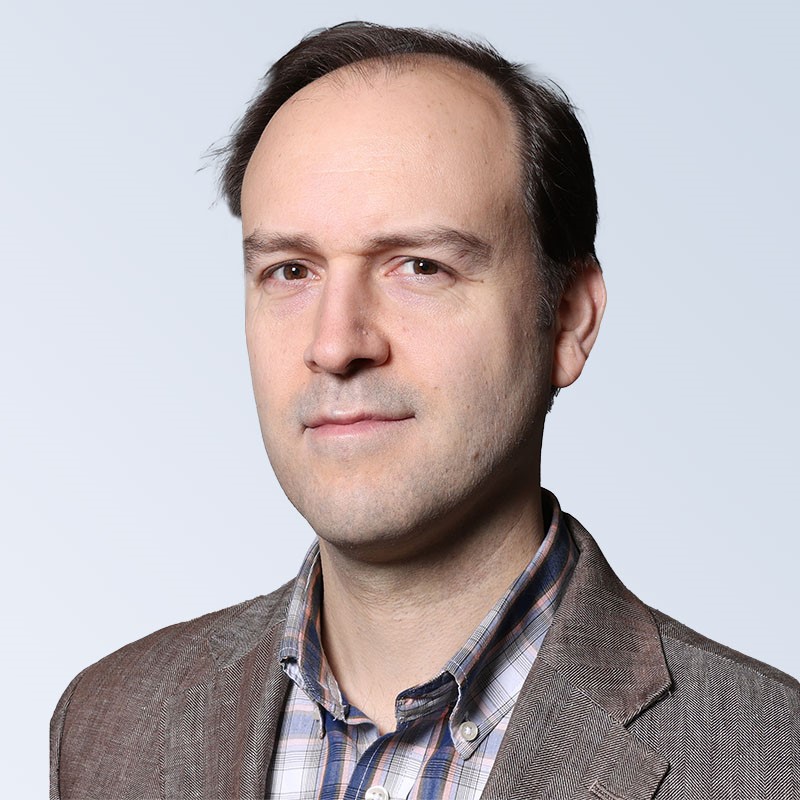
Guillaume Obozinski graduated with a PhD in Statistics from UC Berkeley in 2009. He did his postdoc and held until 2012 a researcher position in the Willow and Sierra teams at INRIA and Ecole Normale Supérieure in Paris. He was then Research Faculty at Ecole des Ponts ParisTech until 2018. Guillaume has broad interests in statistics and machine learning and worked over time on sparse modeling, optimization for large scale learning, graphical models, relational learning and semantic embeddings, with applications in various domains from computational biology to computer vision.
PI | Partners:
description
Motivation
Particle tracking simulations enable the identification of three regions in CERN’s accelerators. Particles that reach the highest possibly observable number of turns in the accelerator contribute to defining the stable region, whereas those that are lost at the early stages of the experiment form the unstable region. Between these stable and unstable regions exists a chaotic state, where particles make a significant number of turns but fewer than the maximum achievable. Different sets of control parameter values of the accelerator lead to different unique machine configurations, and Figure 1 illustrates three examples in which the DA is the stable region. The chaotic region depends upon the subjective threshold at which the number of turns is deemed significant and distinct from the one that formed the unstable region, leading thus to a highly noisy class that is difficult to predict.
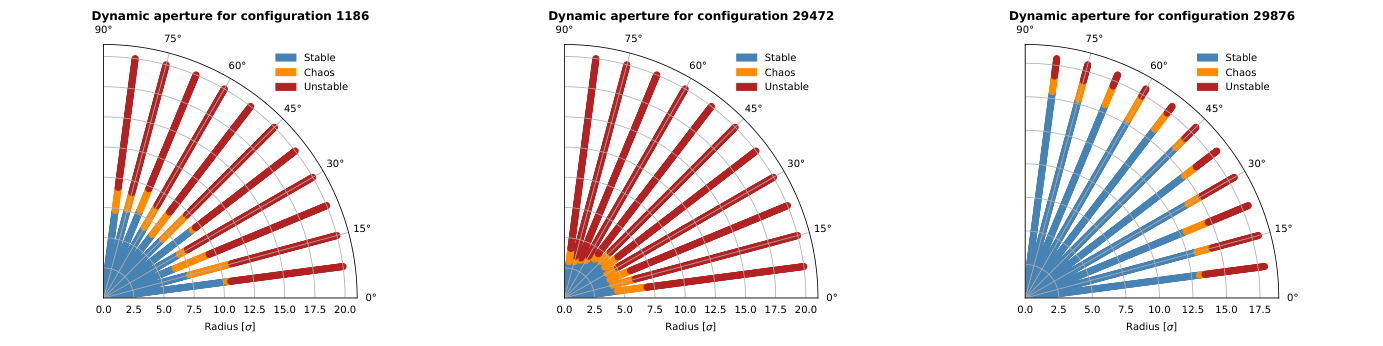
The dataset we analyzed is big, the chaotic region is highly noisy, and parametric representations of the DA are difficult to define, raising thus two challenging objectives: i) to develop a fully automated and computationally efficient machine learning model of the stable region as a function of the accelerator control parameters; and ii) to accurately quantify uncertainty of the predictions to improve the search for optimal machine configurations that lead to the largest DA.
Proposed Approach / Solution
We developed a big, flexible, distributed and Heteroscedastic Spectral-Normalized Neural Process which integrates uncertainty representation in its architecture to predict the stable and (chaotic, unstable) regions now merged into the label “unstable”. We used an empirical Bayes approach to automatically and simultaneously tune all the hyperparameters during the training phase. Figure 2 shows good performance of the best model on the training, the validation and the testing sets.
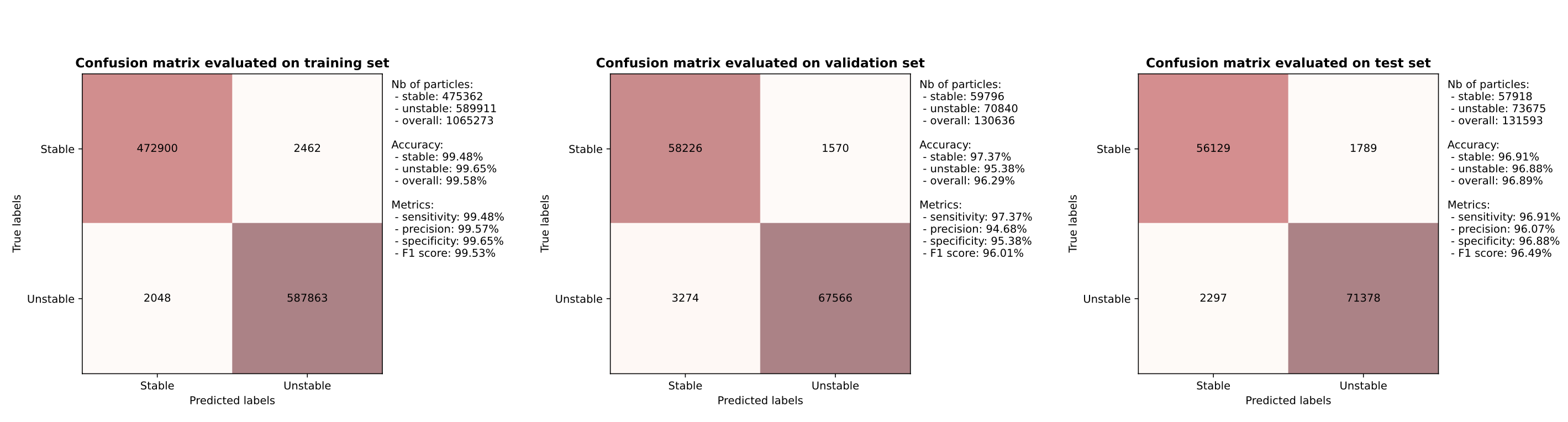
Figure 3 illustrates good accuracy for three test configurations and plausible uncertainty based on the predictive variance. As expected, the uncertainty is high when the predictions are wrong, here at the boundary between the stable and unstable regions, and the uncertainty is low when the predictions look correct.
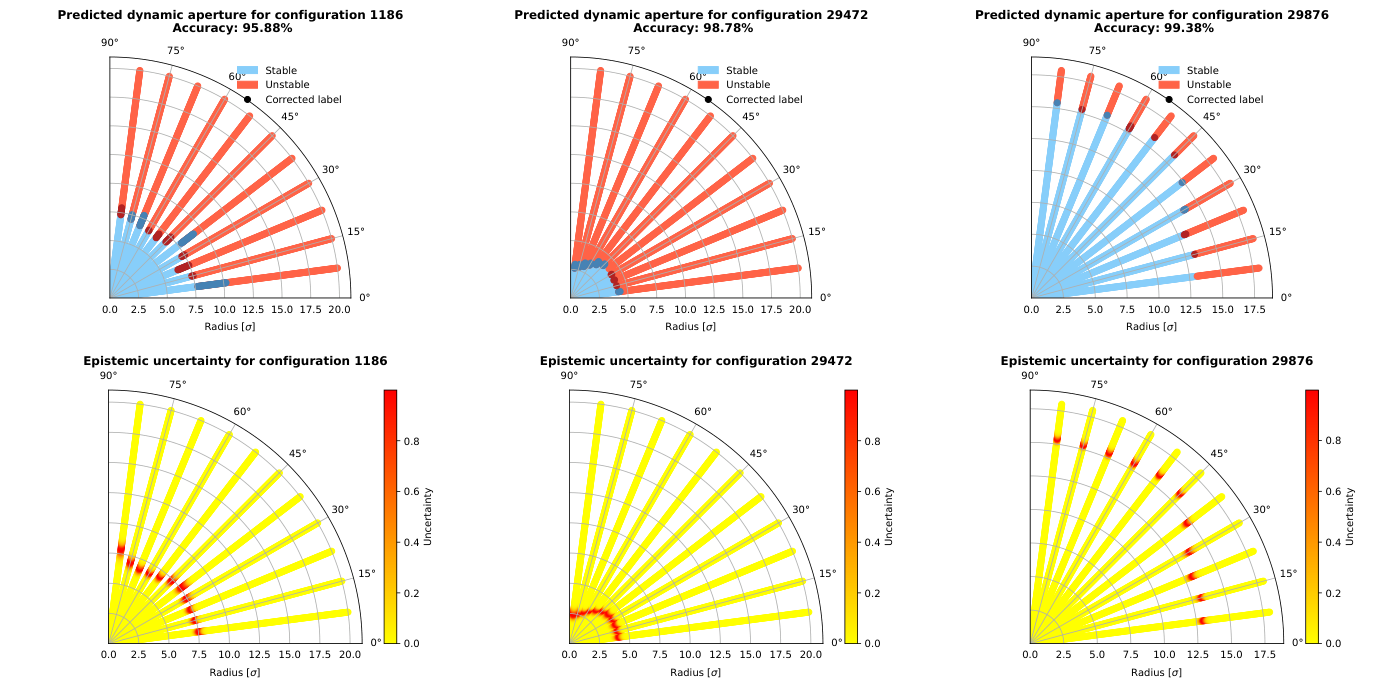
Impact
The results obtained in the project have the potential to revolutionize accelerator design by focusing the automated tracking of particles only on stable areas of the phase space since particles in the unstable areas will anyway be lost. This will optimize the performance of an expensive research infrastructure, and will enable faster scientific discoveries.
Presentation
Gallery
Annexe
Additional resources
Bibliography
- Fortuin, V., Collier, M. P., Wenzel, F., Allingham, J. U., Liu, J., Tran, D., Lakshminarayanan, B., Jerent, J., Jenatton, R. and Kokiopoulou, E. (2022). Deep Classifiers with Label Noise Modeling and Distance Awareness. Transactions on Machine Learning Research, 2835-8856.
- Liu, J., Padhy, S. , Ren, J., Lin, Z., Wen, Y., Jerfel, G., Nado, Z., Snoek, J., Tran, D., and Lakshminarayanan, B. (2023). A Simple Approach to Improve Single-Model Deep Uncertainty via Distance-Awareness. Journal of Machine Learning Research, 24(42):1-63.
Publications
Related Pages
More projects
MAGNIFY
News
Latest news


First National Calls: 50 selected projects to start in 2025
First National Calls: 50 selected projects to start in 2025
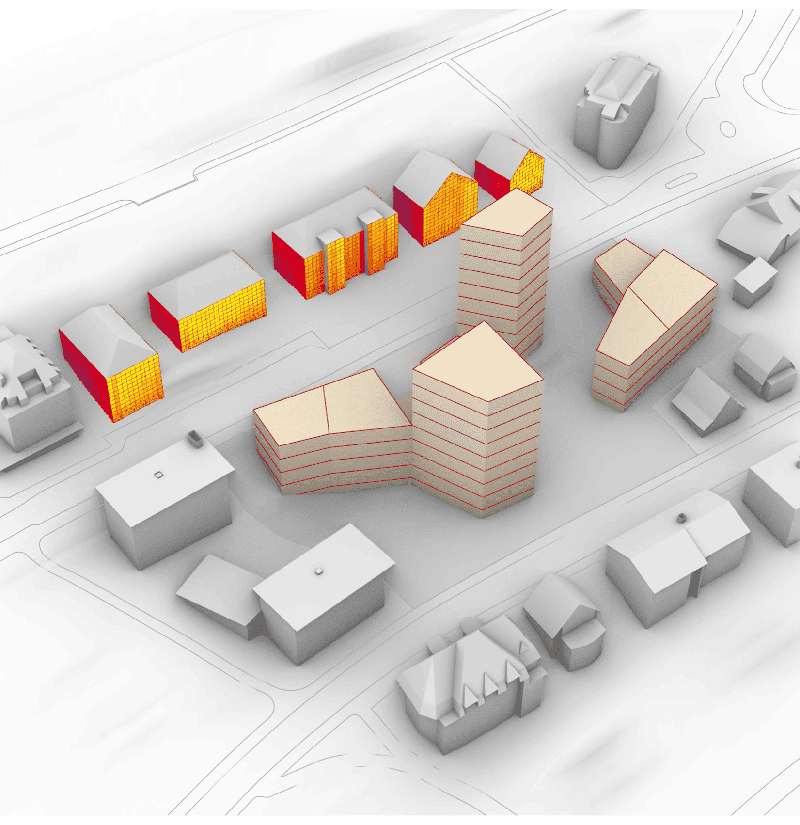
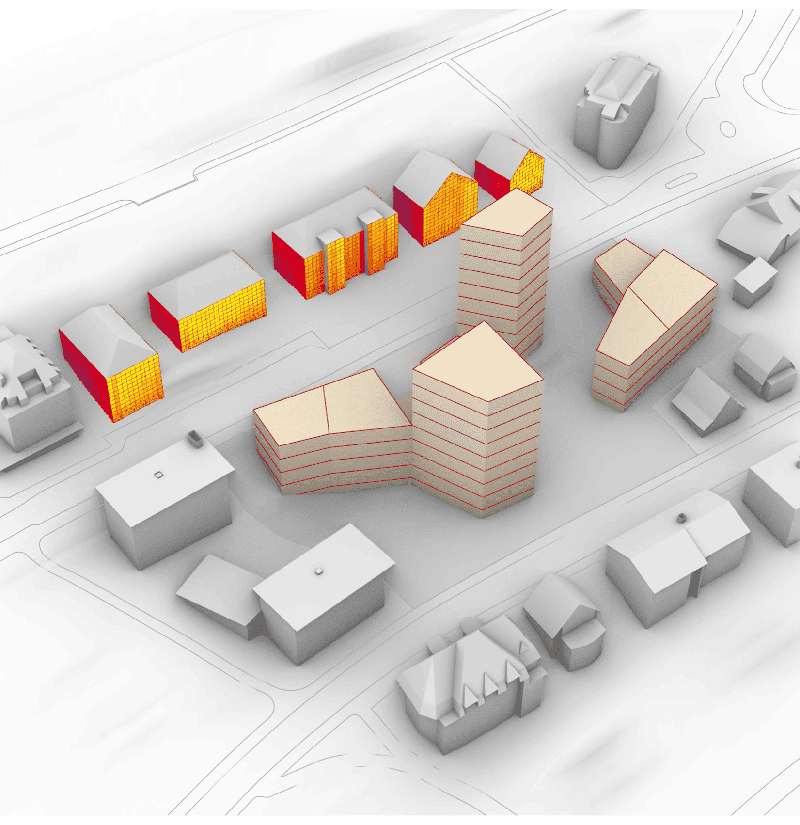
AIXD | Generative AI toolbox for architects and engineers
AIXD | Generative AI toolbox for architects and engineers


Smartair | An active learning algorithm for real-time acquisition and regression of flow field data
Smartair | An active learning algorithm for real-time acquisition and regression of flow field data
Contact us
Let’s talk Data Science
Do you need our services or expertise?
Contact us for your next Data Science project!