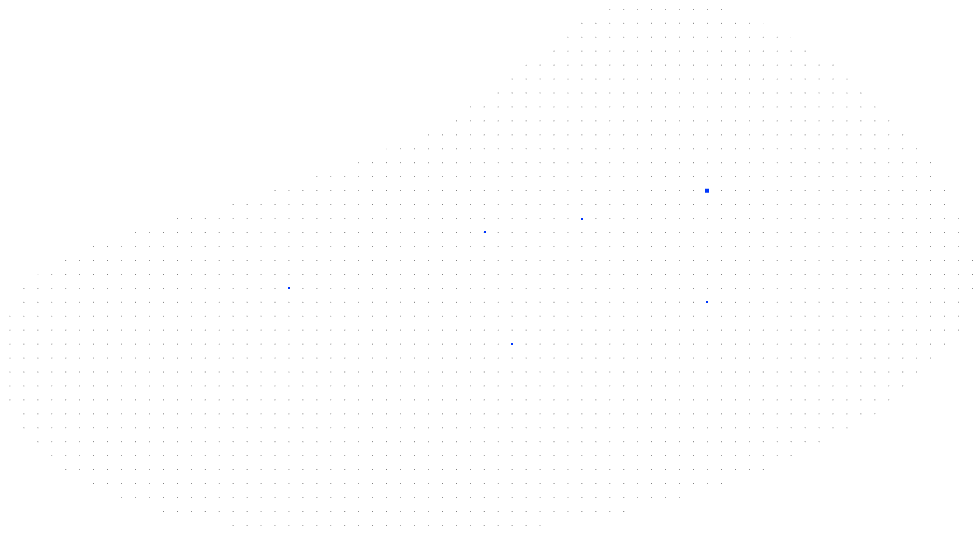
MedCare
Detecting novel drug combinations associated with adverse events

Abstract
Drug-drug interactions (DDIs) are a leading cause of adverse drug events (ADEs), which are a major cause of preventable harm and mortality. While a small number of DDIs are known at the time of market approval, based on the pharmacokinetics of drug metabolism, the potential for additive effects from multi-drug combinations (MDCs) in patients with high medication use (polypharmacy) remains largely unknown. Moreover, as the number of possible drug combinations is immense in patients with high medication use (>1 billion), it is not possible to identify new harmful DDIs using traditional methods.
Thus, advances in machine learning (ML) that can identify harmful MDCs while addressing causal inference (i.e., time relationships) are needed. The MedCare project aims to develop and apply new approaches to identify and evaluate DDIs in large healthcare data.
This project is co-funded by PHRT.
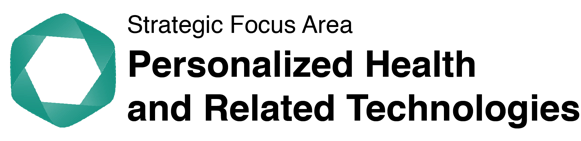
People
Collaborators
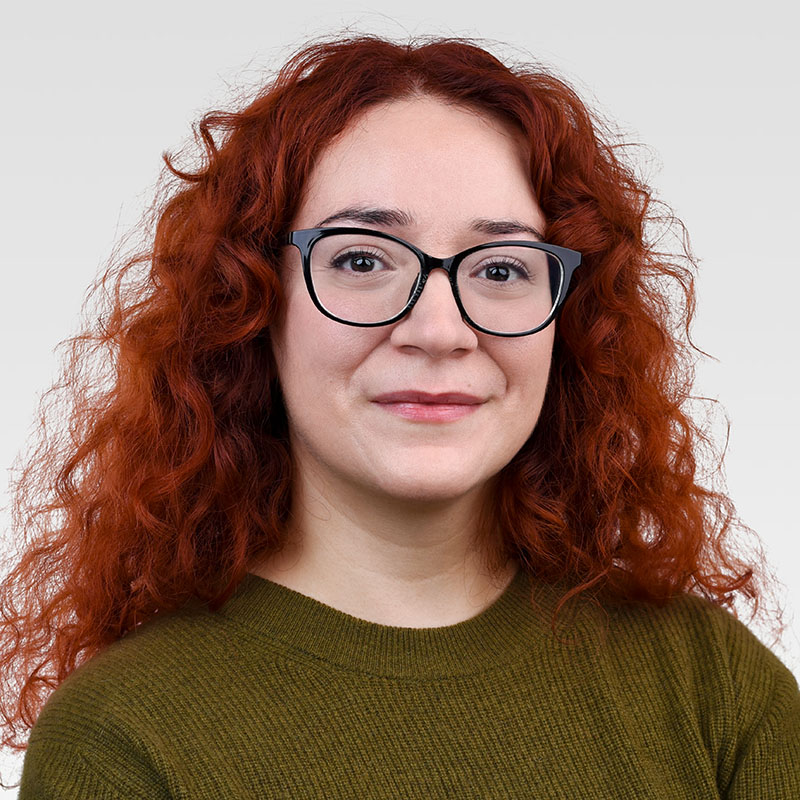
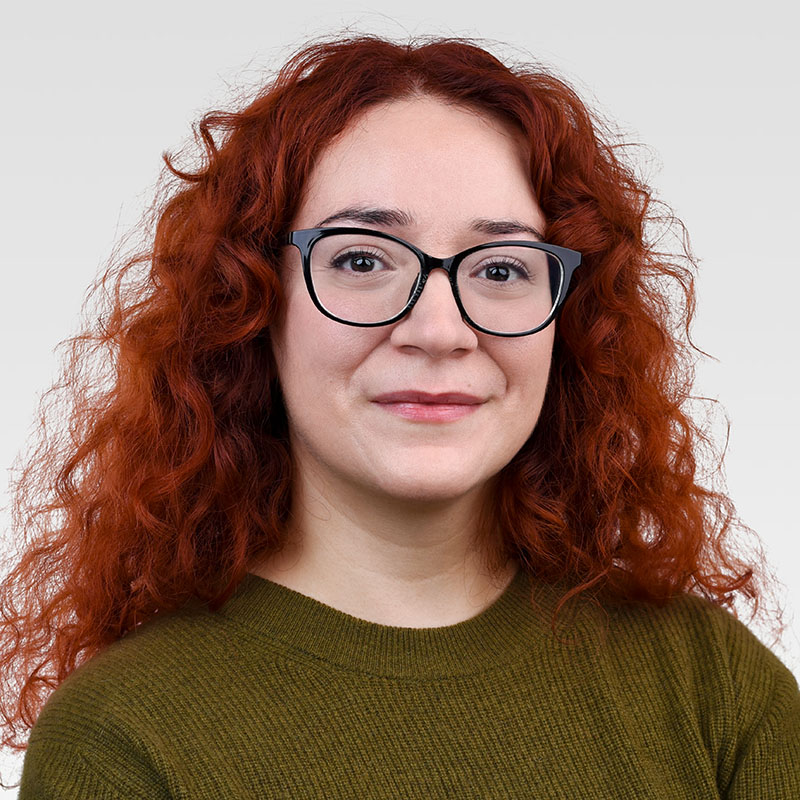
Paraskevi holds a Bachelor's degree and a PhD in Computer Science from Aristotle University of Thessaloniki, obtained in 2014 and 2021 respectively. Her thesis was focused on supervised and unsupervised Deep Learning methodologies with applications in computer and robotic vision as well as timeseries analysis. Her research interests include deep learning for computer vision, robotics, timeseries forecasting and gravitational waves analysis.


Maxim joined the SDSC as a Senior Data Scientist in September 2023. He is interested in developing practical Machine Learning tools and deriving actionable insights for complex real-world challenges. He has worked on a variety of topics in generative AI, computer vision, and deep learning theory, with practical applications in different areas like object detection in environmental science or data-driven discovery of novel molecules in chemistry. Maxim holds a Ph.D. in computer science from the University of Basel and a B.Sc. as well as an M.Sc. in physics from ETH Zurich.


Leonid joined SDSC in 2022 to design data-driven solutions for academic research projects. His research interests are in the areas of uncertainty quantification, ensemble learning, and probabilistic modeling. Leonid graduated from Moscow State University and defended his PhD at HSE University in 2021. Leonid has experience working in financial services, where he was engaged in projects related to pricing, product bundling, and uplift modeling. His recent cross-disciplinary collaborations involve applications in medicine and physics.
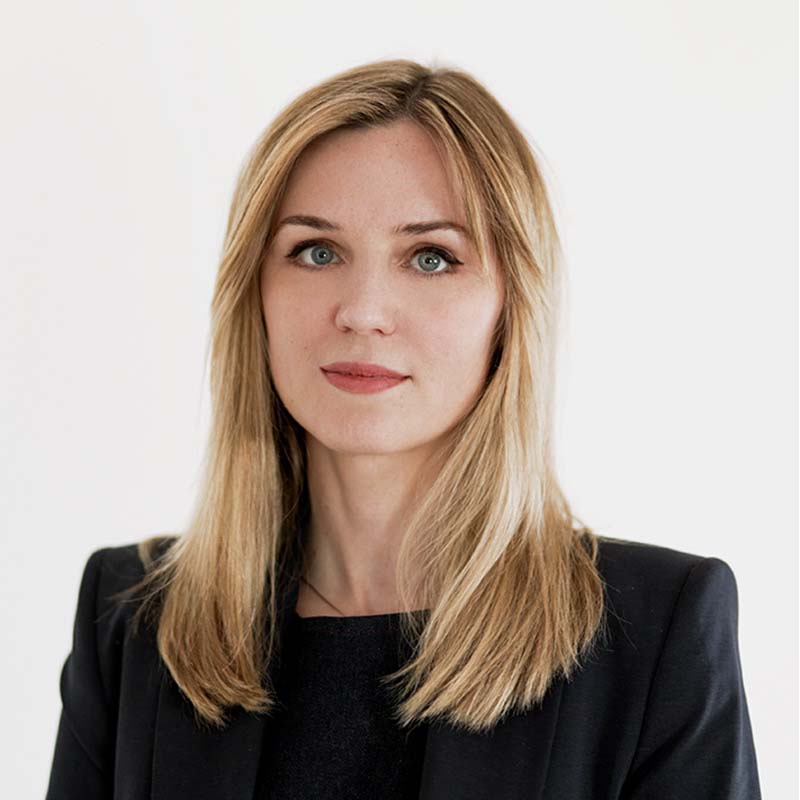
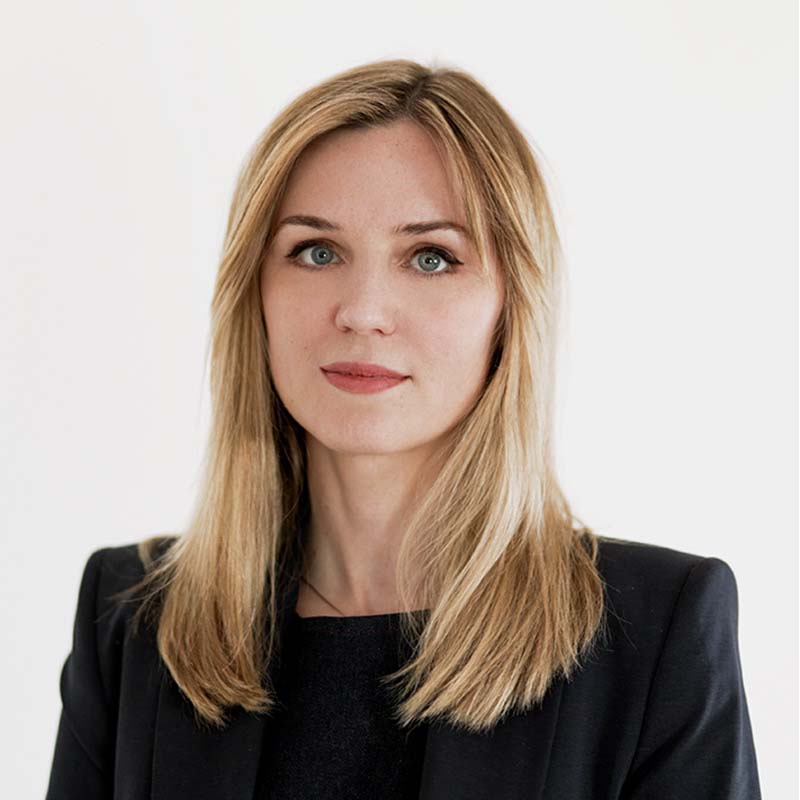
Ekaterina received her PhD in Computer Science from Moscow Institute for Physics and Technology, Russia. Afterwards, she worked as a researcher at the Institute for Information Transmission Problems in Moscow and later as a postdoctoral researcher in the Stochastic Group at the Faculty of Mathematics at University Duisburg-Essen, Germany. She has experience with various applied projects on signal processing, predictive modelling, macroeconomic modelling and forecasting, and social network analysis. She joined the SDSC in November 2019. Her interests include machine learning, non-parametric statistical estimation, structural adaptive inference, and Bayesian modelling.
PI | Partners:
ETH Zurich, Department of Chemistry and Applied Biosciences, Institute of Pharmaceutical Sciences, Pharmacoepidemiology:
- Prof. Dr. Andrea Burden
- Dr. Adrian Martinez de la Torre
- Franziska Ulrich
description
Motivation
The risk of an interaction between two or more drugs leading to a DDI is elevated in patients taking multiple medications (i.e., polypharmacy). The objective of MedCare is to develop new approaches to identify new DDIs or MDCs associated with ADEs in real-world post-market healthcare data. With these approaches, we expect to improve the detection and evaluation of previously undetected adverse drug events associated with MDCs. Importantly, as the results of MedCare will have direct relevance to the medical care of patients, an emphasis on transparency and interpretability is a primary focus.
Proposed Approach/Solution
MedCare aims to overcome the limitations of current approaches by combining the strengths of machine learning (ML) developed with the methodological knowledge of pharmacoepidemiology and electronic pharmacovigilance as well as medical record data. The project will have two main components: (1) to develop and refine ML models that can detect drug interactions in pharmacovigilance data, and (2) adapt ML for pharmacovigilance data to electronic healthcare data that includes longitudinal (i.e., time-series) patient data.
Impact
MedCare will advance our scientific knowledge of how to best identify new harmful DDIs using ML methods. If successful, the approaches used in MedCare will be an important step forward for developing ML models that can detect harmful drug combinations and extend beyond a two-drug interaction approach. These results will advance the knowledge of harmful DDIs and will ideally be used to improve patient safety.
Presentation
Gallery
Annexe
Cover image source: Adobe Stock
Additional resources
Bibliography
- Basile, A. O., Yahi, A., & Tatonetti, N. P. (2019). Artificial intelligence for drug toxicity and safety. Trends in Pharmacological Sciences, 40(9), 624–635. https://doi.org/10.1016/j.tips.2019.07.005
- Blozik, E., Rapold, R., von Overbeck, J., & Reich, O. (2013). Polypharmacy and potentially inappropriate medication in the adult, community-dwelling population in Switzerland. Drugs & Aging, 30, 561–568. https://doi.org/10.1007/s40266-013-0073-0
- Dumbreck, S., Flynn, A., Nairn, M., Wilson, M., Treweek, S., Mercer, S. W., Alderson, P., Thompson, A., Payne, K., & Guthrie, B. (2015). Drug-disease and drug-drug interactions: systematic examination of recommendations in 12 UK national clinical guidelines. BMJ (Online), 350, 1–8. https://doi.org/10.1136/bmj.h949
- Marengoni, A., & Onder, G. (2015). Guidelines, polypharmacy, and drug-drug interactions in patients with multimorbidity. BMJ (Online), 350, 1059. https://doi.org/10.1136/bmj.h1059
- Tatonetti, N. P., Fernald, G. H., & Altman, R. B. (2012). A novel signal detection algorithm for identifying hidden drug-drug interactions in adverse event reports. Journal of the American Medical Informatics Association, 19(1), 79–85. https://doi.org/10.1136/amiajnl-2011-000214
- Tatonetti, N. P., Ye, P. P., Daneshjou, R., & Altman, R. B. (2012). Data-driven prediction of drug effects and interactions. Science Translational Medicine, 4(125), 125ra31. https://doi.org/10.1126%2Fscitranslmed.3003377
Publications
Related Pages
More projects
MAGNIFY
News
Latest news
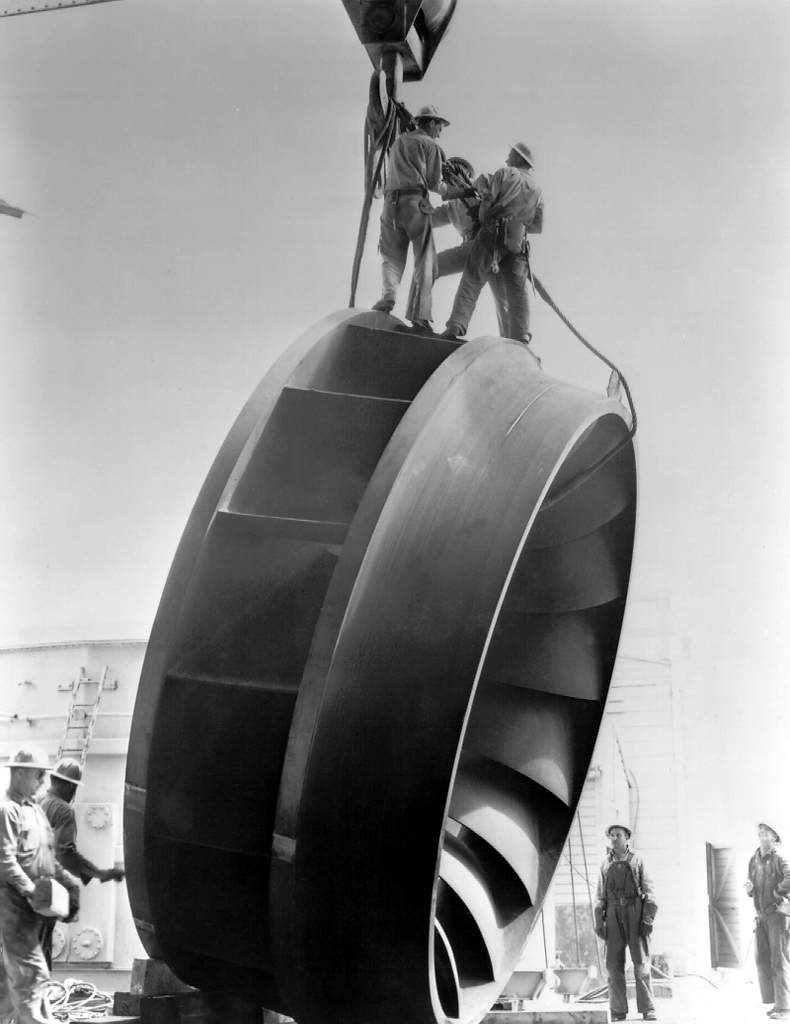
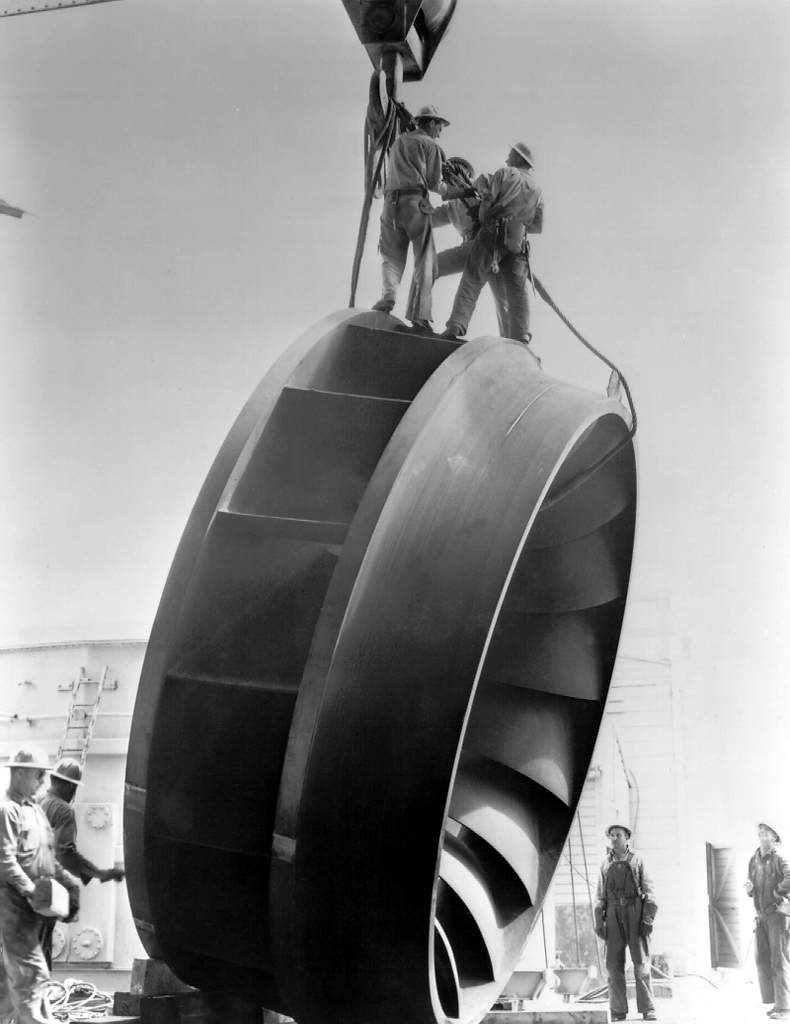
PAIRED-HYDRO | Increasing the Lifespan of Hydropower Turbines with Machine Learning
PAIRED-HYDRO | Increasing the Lifespan of Hydropower Turbines with Machine Learning


First National Calls: 50 selected projects to start in 2025
First National Calls: 50 selected projects to start in 2025
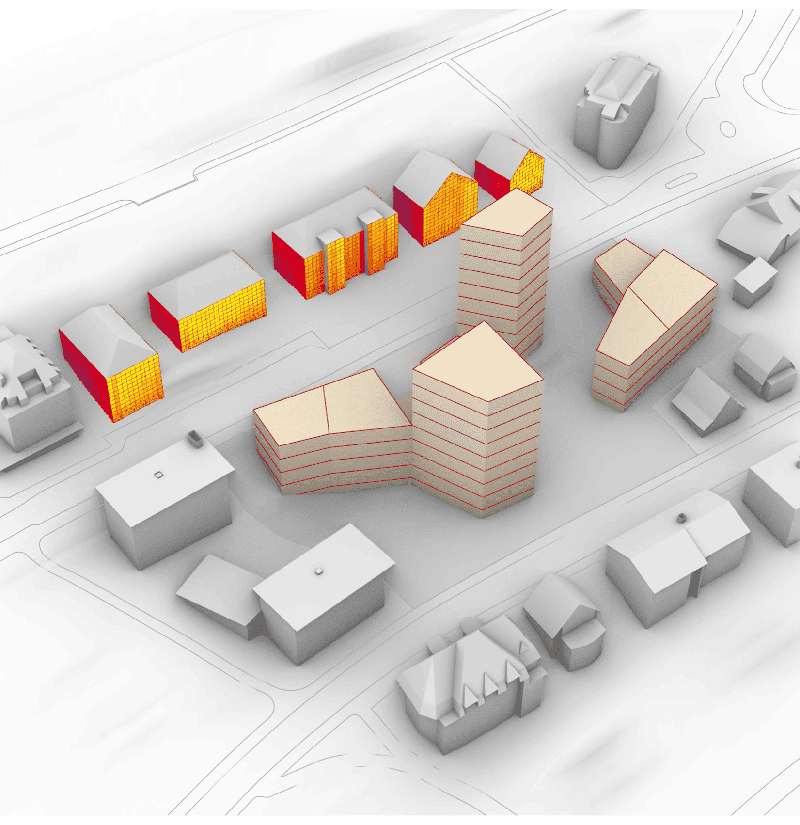
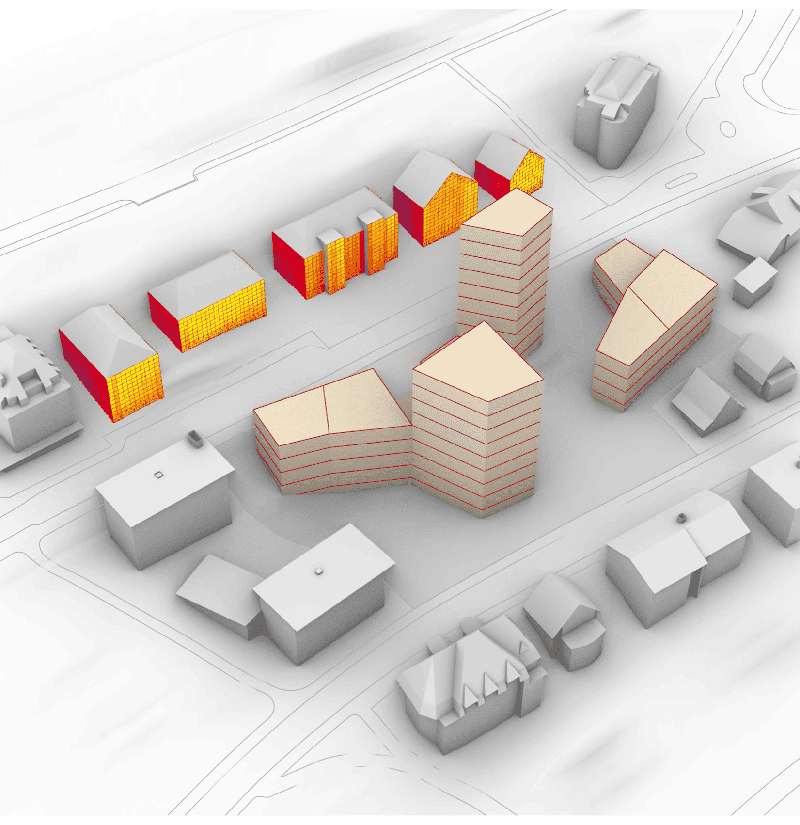
AIXD | Generative AI toolbox for architects and engineers
AIXD | Generative AI toolbox for architects and engineers
Contact us
Let’s talk Data Science
Do you need our services or expertise?
Contact us for your next Data Science project!