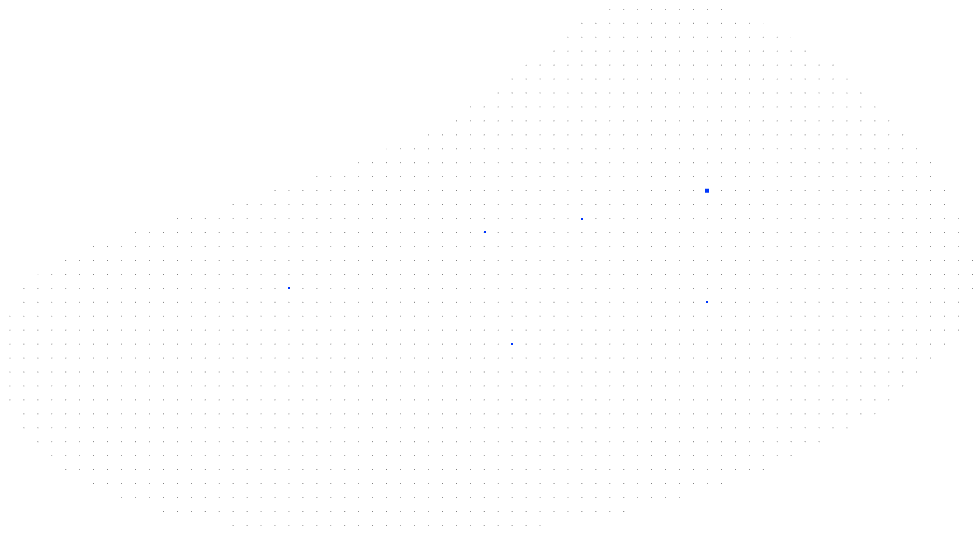
LETITIA
Learning tumor dynamics and early markers of immunotherapy response from PET/CT imaging

Abstract
The recent advances in oncology, particularly in immunotherapy with immune checkpoint inhibitors (ICIs), have delivered astounding clinical successes in metastatic cancers. However, the response rate remains partial (~50%), with some patients experiencing no benefit and strong adverse effects. This calls for the development of personalized medicine approaches to cancer therapy, and in particular, the identification of predictive biomarkers that can identify responders before or at an early stage of ICI treatment.
The primary goal of this project is to build innovative machine learning solutions for predicting patients’ response to ICI therapy. We explore the rich information provided by full-body PET/CT imaging obtained at different points in time during treatment. Our main assumption is that, if handled appropriately, these data can reveal tumor dynamics in a non-invasive fashion.
Using an unprecedented dataset of full-body PET/CT scans acquired from cohorts of metastatic melanoma and lung cancer patients treated with ICIs at the University Hospital Zurich (USZ) and the University Hospital Lausanne (CHUV), we design a novel data-driven framework that identifies tumor lesions at different stages of the disease (before, during, and after treatment), and exploits the combined trajectories of individual metastatic lesions to predict ICI response.
This project offers strong potential for enabling interdisciplinary synergy between EPFL, HES-SO, the Lausanne University Hospital, the University Hospital Zurich, and the Swiss Data Science Center, in order to foster a scientific breakthrough in the highly impactful domain of personalized oncology. We believe that the proposed line of research paves the way for improved patient selection and treatment recommendations in the era of personalized medicine.
This project is co-funded by PHRT.
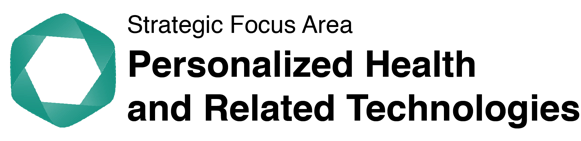
People
Collaborators


Isinsu joined the SDSC in May 2022. She obtained a Bachelor’s degree in Computer Science from Middle East Technical University, Turkey, and later received a Master’s degree in Computer Science from EPFL. She conducted her doctoral studies in the Computer Vision Lab at EPFL with a focus on human body pose estimation and motion prediction using deep learning-based methods. Her research interests include self- and semi-supervised learning for image-based analysis of the human body and motion, with applications ranging from athletic training to healthcare.


Mathieu Salzmann is the Deputy Chief Data Scientist of the Swiss Data Science Center and a Senior Scientist and Lecturer at EPFL. He received his PhD from EPFL in 2009 and, between 2009 and 2015, has held post-doctoral and researcher positions at UC Berkeley, TTI-Chicago, and NICTA and the Australian National University. From May 2020 to February 2024, he was also a part-time Senior GNC Engineer at ClearSpace. Mathieu Salzmann has broad interests in machine learning and deep learning, with a particular focus on computer vision. He has published over 100 articles at top-tier peer-reviewed machine learning and computer vision venues and is a strong believer in collaborative work.
PI | Partners:
description
Motivation
Despite the transformative success of immune checkpoint inhibitors (ICIs) in oncology, treatment outcomes remain highly variable and difficult to predict, with a critical lack of clinically validated biomarkers to guide patient stratification. Understanding distinct response patterns, such as dissociated response or pseudoprogression, remains a pressing clinical challenge, especially in cancers like melanoma where current imaging studies are limited and methodologically inconsistent. This project aims to address these gaps by developing advanced, data-driven tools that leverage longitudinal PET/CT imaging and radiomics to capture the spatial and temporal evolution of individual tumor lesions. By automating lesion tracking and integrating imaging biomarkers with clinical data, the goal is to build robust models that predict ICI response at an early stage, ultimately enabling more personalized and effective treatment strategies.
Proposed Approach / Solution
We propose to address these objectives by casting the problem of predicting ICI response in a spatiotemporal context, through two main stages. In the first stage, we will develop a longitudinal PET/CT segmentation and tracking algorithm that captures the temporal evolution of individual lesions and uses this information to improve segmentation accuracy and lesion detection. This will be achieved by building on recent advances in medical foundation models trained across diverse imaging modalities. In the second stage, we will design a dynamic graph representation learning framework that models both intra- and inter-lesion dependencies. The lesion trajectories extracted in the first stage will serve to combine PET/CT radiomics features from a patient’s metastatic lesions, clinical data such as blood biomarkers, and informative representations from foundation model embeddings, to predict ICI treatment outcomes for melanoma and lung cancer patients. These methods will first be developed and validated on the USZ-Melanoma dataset, which includes ground-truth lesion detection and tracking. The CHUV-Melanoma and CHUV-Lung cancer datasets, which contain noisier annotations, will then be used to evaluate the generalizability of both frameworks.

Impact
The proposed research will demonstrate the power of data science in personalized oncology by developing an automated algorithm for longitudinal segmentation and lesion tracking from routine clinical PET/CT scans. This technology will enable large-scale, automated curation of real-world imaging data, supporting research into tumor dynamics and offering potential clinical applications in monitoring treatment response. By identifying biomarkers of ICI response from non-invasive imaging, the project aims to improve patient-specific treatment stratification, optimizing outcomes while minimizing adverse effects.
Presentation
Gallery
Annexe
Cover image source: Adobe Stock
Additional resources
Bibliography
- Cai, J., Tang, Y., Yan, K., Harrison, A. P., Xiao, J., Lin, G., & Lu, L. Deep Lesion Tracker: Monitoring Lesions in 4D Longitudinal Imaging Studies. IEEE/CVF Conference on Computer Vision and Pattern Recognition, 15154-15164 (2021).
- Garutti M., Bonin S., Buriolla S., Bertoli E., Pizzichetta M. A., Zalaudek I., & Puglisi F. Find the Flame: Predictive Biomarkers for Immunotherapy in Melanoma. Cancers (Basel), 13(8) (2021).
- Skarding, J., Gabrys, B., & Musial, K. Foundations and Modeling of Dynamic Networks Using Dynamic Graph Neural Networks: A Survey. IEEE Access, 9 (2021).
- Gabryś H. S., Basler L., Burgermeister S., Hogan S., Ahmadsei M., Pavic M., Bogowicz M., Vuong D., Tanadini-Lang S., Förster R., Kudura K., Huellner M., Dummer R., Levesque M. P., Guckenberger M. PET/CT radiomics for prediction of hyperprogression in metastatic melanoma patients treated with immune checkpoint inhibitors. Frontiers in Oncology, 12 (2022).
- Abler D., Courlet P., Dietz M., Gatta R., Girard P., Munafo A., Wicky A., Jreige M., Guidi M., Latifyan S., De Micheli R., Csajka C., Prior J. O., Michielin O., Terranova N., & Cuendet M. A. Semiautomated Pipeline to Quantify Tumor Evolution From Real-World Positron Emission Tomography/Computed Tomography Imaging. JCO Clin Cancer Inform., 7, e2200126(2023).
Publications
Related Pages
More projects
MAGNIFY
News
Latest news


First National Calls: 50 selected projects to start in 2025
First National Calls: 50 selected projects to start in 2025
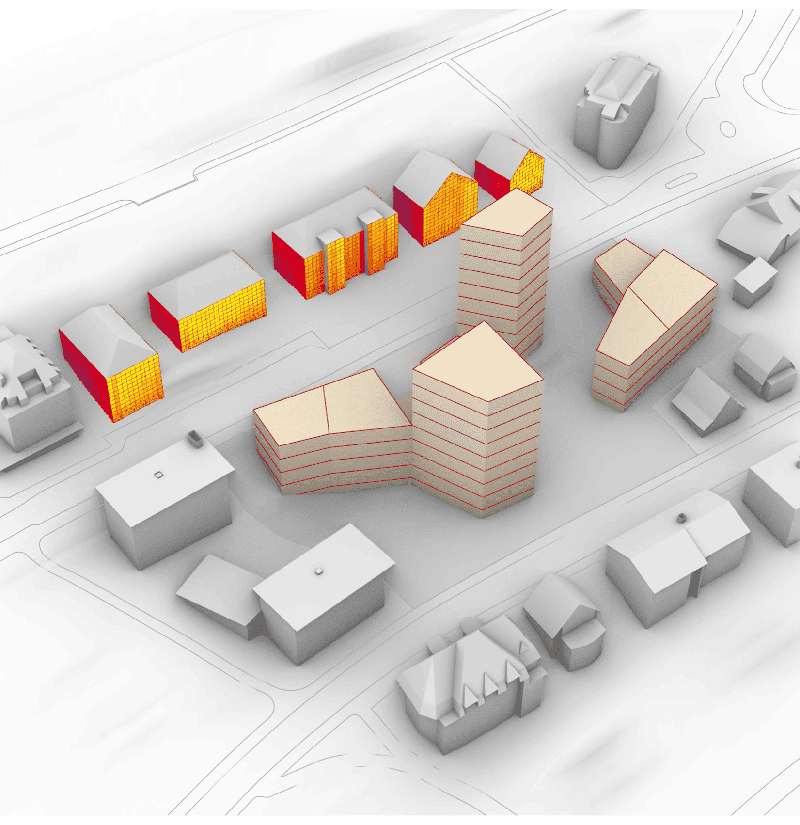
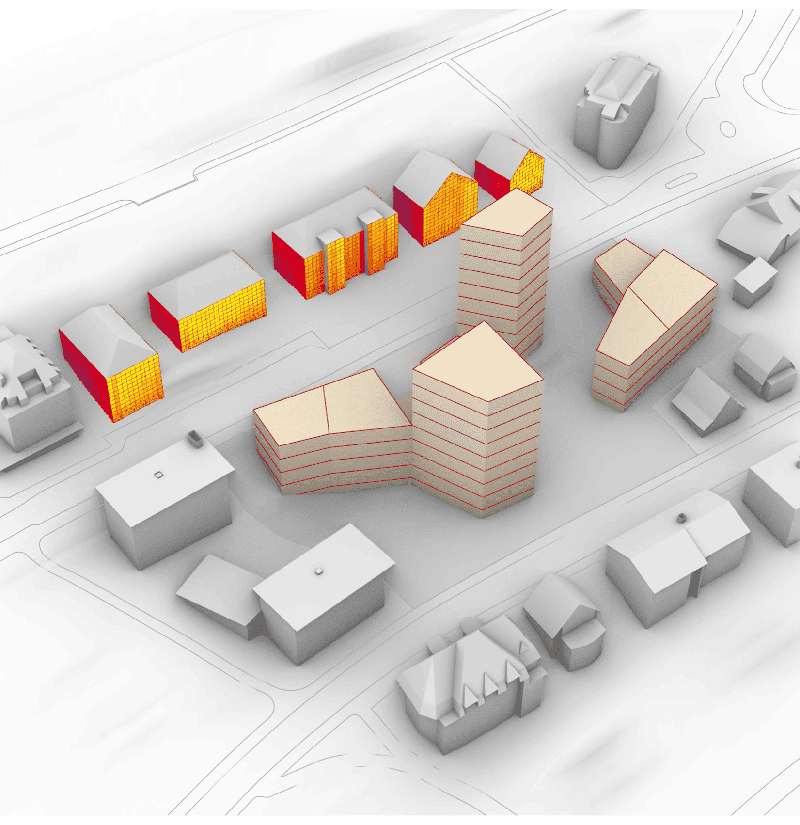
AIXD | Generative AI toolbox for architects and engineers
AIXD | Generative AI toolbox for architects and engineers


Smartair | An active learning algorithm for real-time acquisition and regression of flow field data
Smartair | An active learning algorithm for real-time acquisition and regression of flow field data
Contact us
Let’s talk Data Science
Do you need our services or expertise?
Contact us for your next Data Science project!