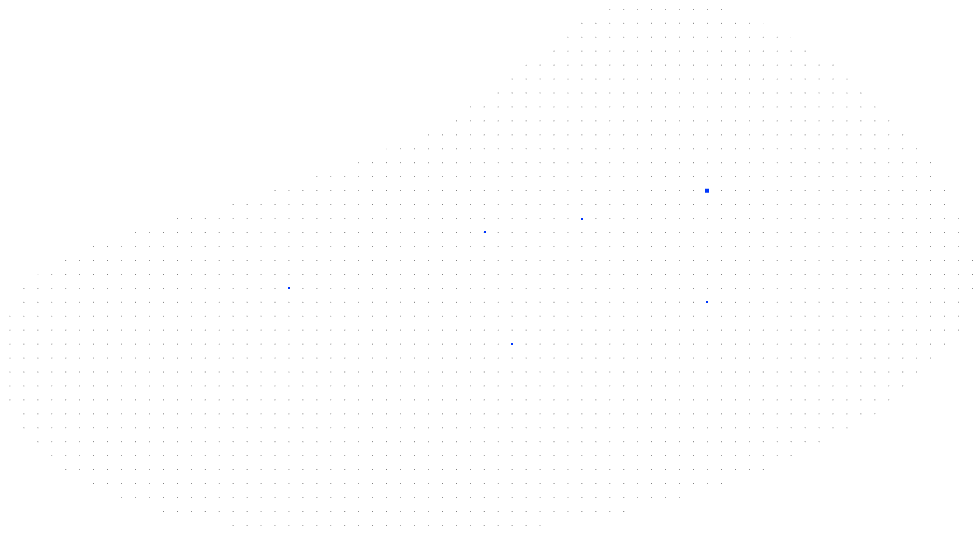
EXPECTmine
Mining Toxicity and High Resolution Mass Spectrometry Data for Linking Exposures to Effects
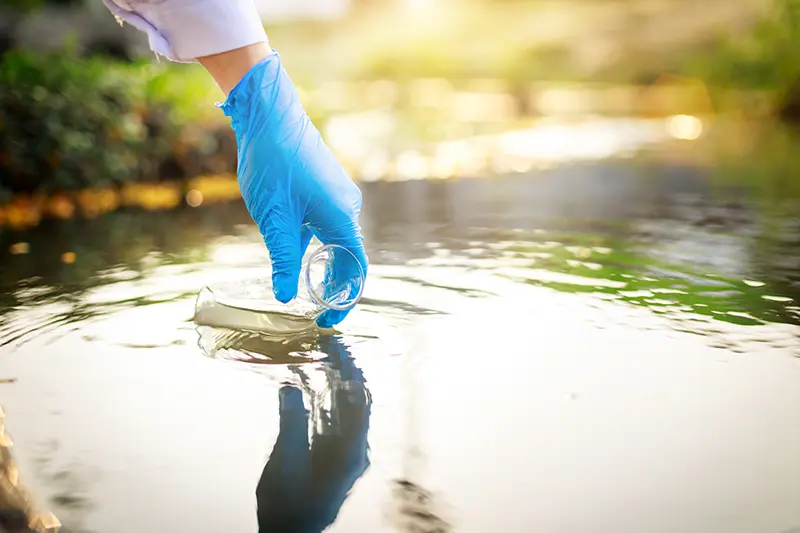
Abstract
In the EXPECTmine project, we propose to solve the challenge of attaching toxicological relevance to environmental analysis by developing MLinvitroTox, a machine learning tool for predicting toxicity fingerprints from high-resolution mass spectrometry (HRMS/MS) data and consolidating it with the existing computational methods into a novel hazard-driven data processing pipeline. The pipeline aims to assess risks (risk = exposure x effect) associated with anthropogenic pollutants and their mixtures directly from HRMS/MS by mapping their exposures (measured concentrations) to the effects (predicted toxicity), thus focusing the analysis from tens of thousands of signals detected via HRMS to a fraction of chemical structures with a high potential to cause harm in the environment and to human health. The biggest impact of EXPECTmine will be realized by mapping toxicologically relevant pollution in aquatic environments, helping to protect humans and natural habitats from particularly harmful anthropogenic pollutants. The highly interdisciplinary EXPECTmine project, which combines elements of analytical chemistry, environmental sciences, toxicology, and data science, gathers collaborators and experts uniquely positioned to solve the challenges in the field from across the whole of Europe. We aim to employ state-of-the-art data science techniques to perform advanced data cleanup, train supervised classification machine learning models to predict toxicity, compile the trained models into the open-source tool MLinvitroTox, as well as to build a pipeline tailored to the complex and interdisciplinary problem of establishing toxicological relevance to HRMS results.
People
Collaborators
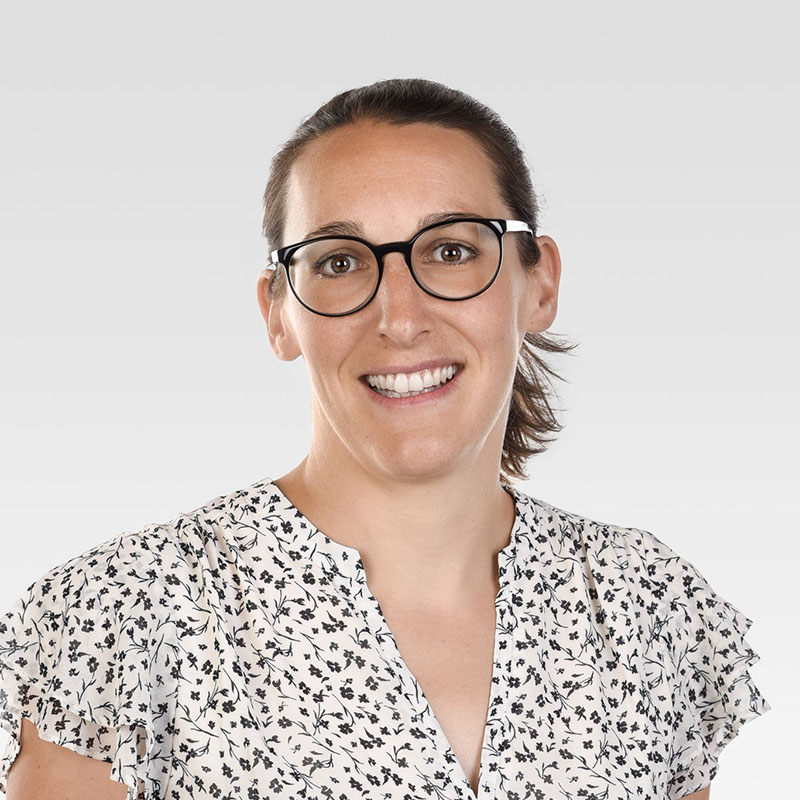
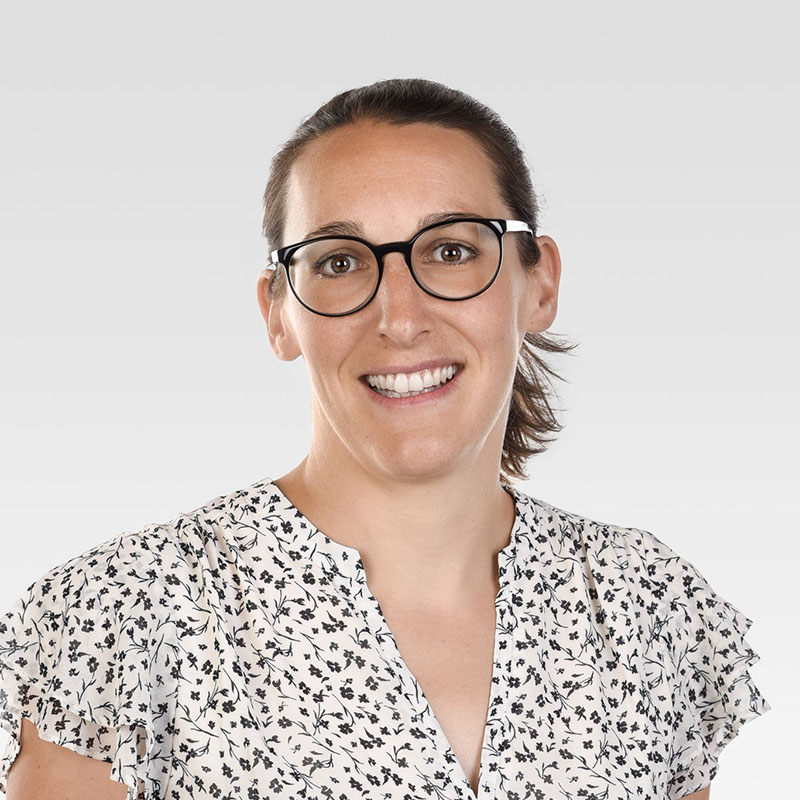
Lili obtained the MSc in Statistics from ETH in 2018. She wrote her Master thesis at the Swiss Data Science Center applying topic modelling to political data. She rejoined the center in May 2020 after a year as a statistical consultant at the Seminar for Statistics at ETH. With her MSc in Chemical Engineering, she worked as a process engineer in the glass industry for several years. She is interested in interdisciplinary projects where data science can help uncover new insights.
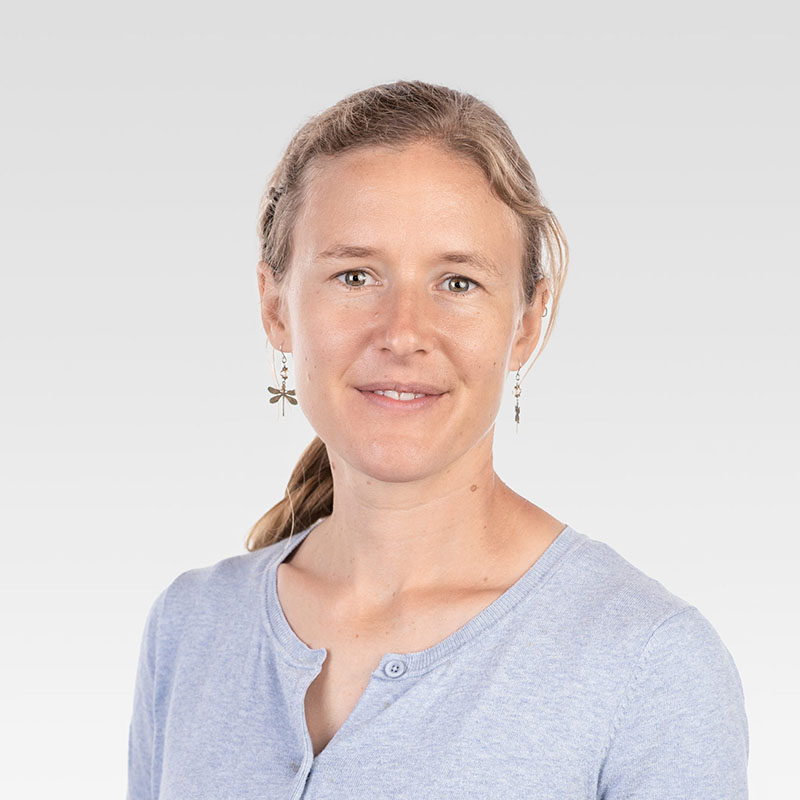
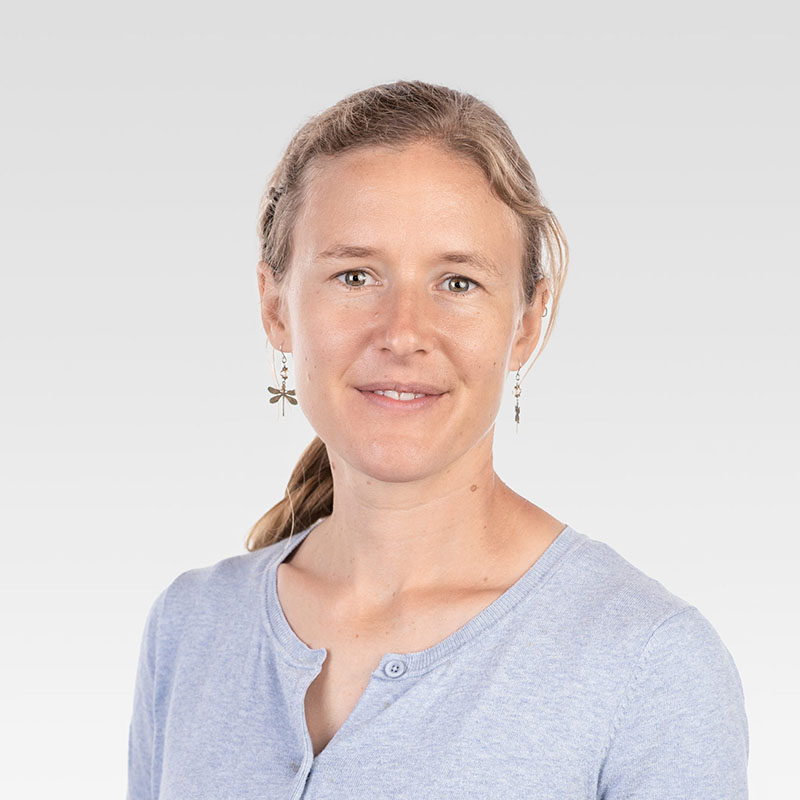
Eliza started at SDSC in March 2021, working as a Senior Scientist as part of the SDSC's academic team. She had previously worked as a postdoctoral researcher at the Massachusetts Institute of Technology (2012-2013), Empa (2013-2017), and the University of Innsbruck (2017-2020). Eliza had received her PhD in Atmospheric Science from the Max Planck Institute for Chemistry in 2012, and her Bachelor’s degree with Honours in Antarctic Science from the University of Tasmania in 2008. Her previous research had centered around the use of novel isotopic measurements and modeling approaches in atmospheric and biogeosciences, particularly the nitrogen cycle. Her research at SDSC was to focus on data analytics and machine learning approaches in environmental and natural sciences. Eliza's mission with SDSC ended in September 2024.
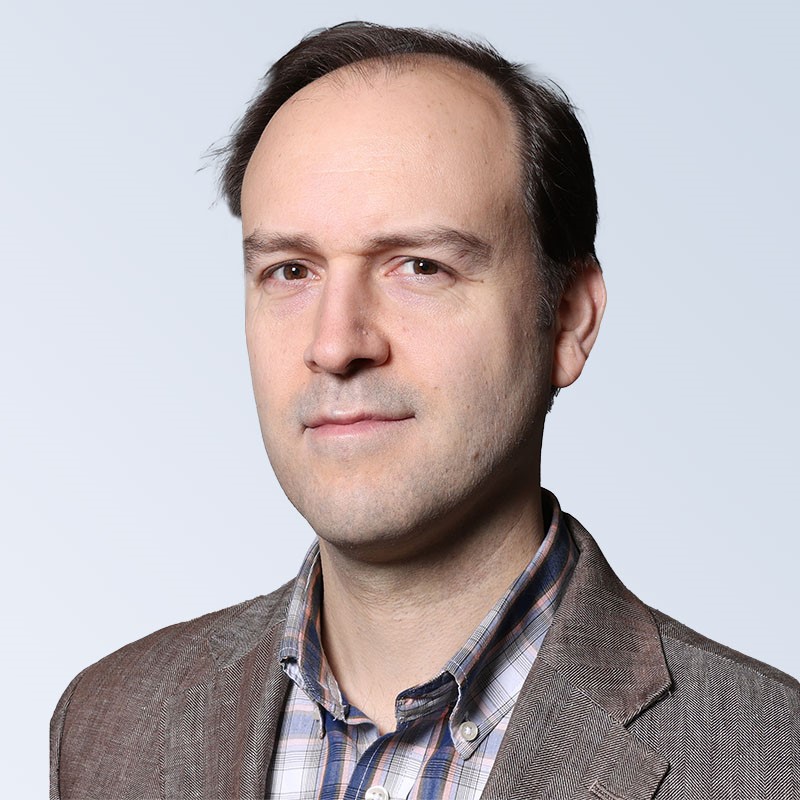
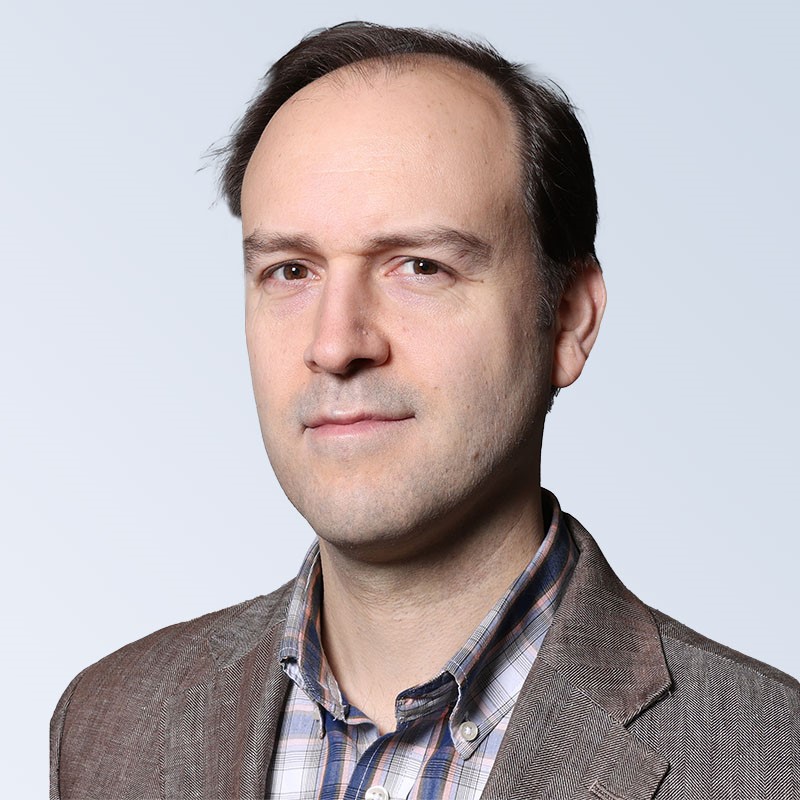
Guillaume Obozinski graduated with a PhD in Statistics from UC Berkeley in 2009. He did his postdoc and held until 2012 a researcher position in the Willow and Sierra teams at INRIA and Ecole Normale Supérieure in Paris. He was then Research Faculty at Ecole des Ponts ParisTech until 2018. Guillaume has broad interests in statistics and machine learning and worked over time on sparse modeling, optimization for large scale learning, graphical models, relational learning and semantic embeddings, with applications in various domains from computational biology to computer vision.
PI | Partners:
EAWAG, Contaminant fate processes group:
- Prof. Dr. Juliane Hollender
- Dr. Kasia Arturi
Stockholm University, Department of Materials and Environmental Chemistry:
- Prof. Dr. Anneli Kruve
- Dr. Pilleriin Peets
Helmholtz Center for Environmental Research, Cell Toxicology department:
- Prof. Dr. Beate Escher
- Dr. Rita Schlichting
- Georg Braun
Friedrich-Schiller Universität Jena, Bioinformatik:
- Prof. Dr. Sebastian Böcker
- Dr. Kai Dührkop
description
Motivation
Environmental pollution is leading to the destruction of biodiversity, contamination of food chains, and lack of potable water. While more than 183 million chemical compounds have been registered, and an estimated 30’000 to 70’000 chemicals are used in households alone, only a few hundred are monitored worldwide. Modern analytical methods such as high-resolution mass spectrometry (HRMS/MS) reveal the presence of thousands of unknown compounds in aquatic environments. Non-targeted screening (NTS) data processing workflows have been developed to convert the detected HRMS/MS signals into quantified chemical structures. But these are based on signal’s abundance and lack the toxicological relevance essential to understand the impact of pollution.
Proposed Approach / Solution
SDSC takes part in the development of MLinvitroTox, a machine learning tool to predict toxicity fingerprints from HRMS/MS data. State-of-the-art classification models are applied to structural fingerprint descriptors to predict the toxicity (either toxic or non-toxic) of all relevant assay endpoints, which are then combined in a toxicity fingerprint. The MLinvitroTox tool is the crucial element of the EXPECTmine pipeline (Figure 1).

Impact
Narrowing the analytical focus from tens of thousands of signals detected via HRMS/MS to a fraction of chemical structures with a high potential to cause harm, will have tangible impacts on the mapping of toxicologically relevant pollution in aquatic environments, helping to protect humans and natural habitats from particularly harmful anthropogenic pollutants, as outlined in the objectives of the Chemicals Strategy for Sustainability in the European Green Deal.
Presentation
Gallery
Annexe
Additional resources
Bibliography
- Hollender, J., Schymanski, E. L., Singer, H. P., & Ferguson, P. L. (2017). Nontarget screening with high resolution mass spectrometry in the environment: ready to go?. Nontarget Screening with High Resolution Mass Spectrometry in the Environment: Ready to Go?
- Dührkop, K., Fleischauer, M., Ludwig, M., Aksenov, A. A., Melnik, A. V., Meusel, M., ... & Böcker, S. (2019). SIRIUS 4: a rapid tool for turning tandem mass spectra into metabolite structure information. Nature methods, 16(4), 299-302. https://www.nature.com/articles/s41592-019-0344-8
- Neale, P. A., Munz, N. A., Aїt-Aїssa, S., Altenburger, R., Brion, F., Busch, W., ... & Hollender, J. (2017). Integrating chemical analysis and bioanalysis to evaluate the contribution of wastewater effluent on the micropollutant burden in small streams. Science of the Total Environment, 576, 785-795. https://doi.org/10.1016/j.scitotenv.2016.10.141
Publications
Related Pages
- Official project pages on institutional webpage: Eawag - Swiss Federal Institute of Aquatic Science and Technology - Eawag
More projects
ML-L3DNDT
BioDetect
News
Latest news


First National Calls: 50 selected projects to start in 2025
First National Calls: 50 selected projects to start in 2025
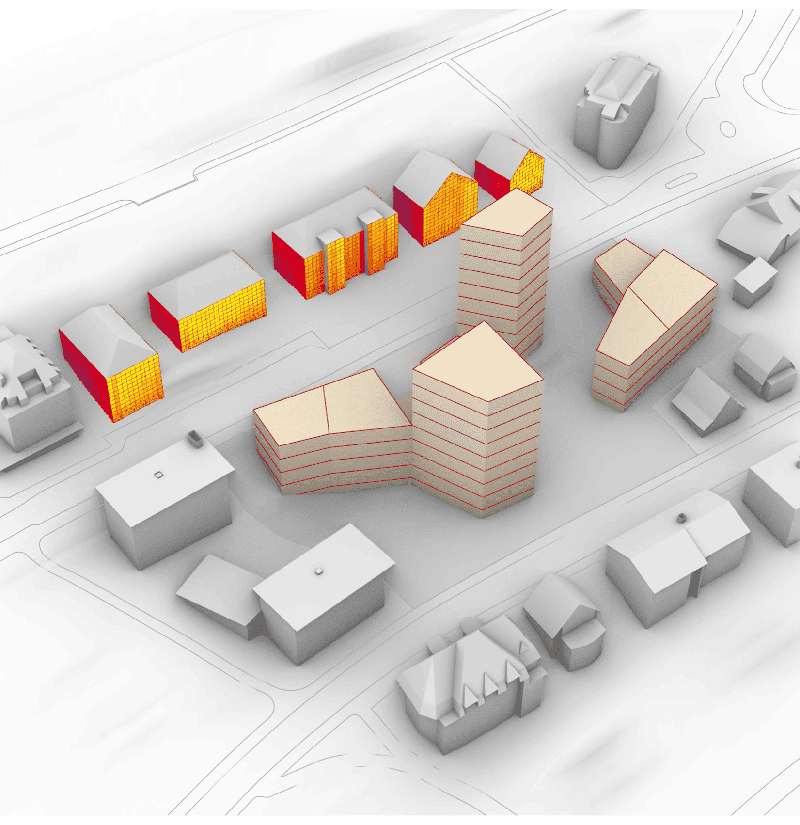
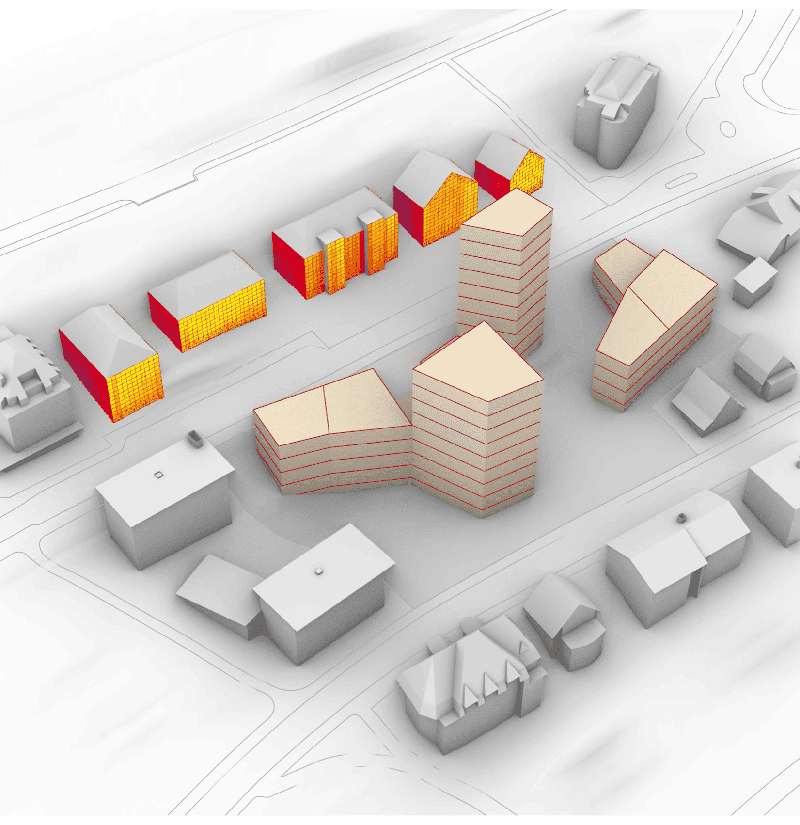
AIXD | Generative AI toolbox for architects and engineers
AIXD | Generative AI toolbox for architects and engineers


Smartair | An active learning algorithm for real-time acquisition and regression of flow field data
Smartair | An active learning algorithm for real-time acquisition and regression of flow field data
Contact us
Let’s talk Data Science
Do you need our services or expertise?
Contact us for your next Data Science project!