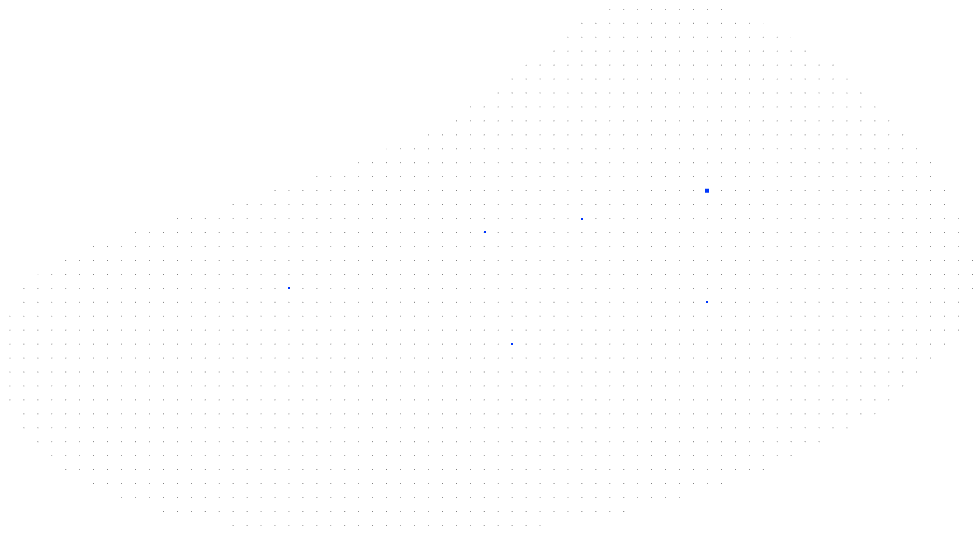
EUTHERMO
Scalable deep reinforcement learning algorithms for building climate control and energy management

Abstract
Controlling indoor comfort and optimizing energy flows in modern buildings has become an increasingly complex, integrating photovoltaics, batteries or electric vehicle chargers. Yet, effective building energy management remains a critical challenge, as buildings contribute to 32% of global primary energy use and a significant share of greenhouse gas emissions.
While traditional rule-based controllers are limited by their manual tuning and performance, advanced methods like Model Predictive Control (MPC) require costly and complex modeling, limiting their widespread adoption. Reinforcement learning (RL), particularly deep RL, has emerged as a promising alternative for building control due to its ability to handle system complexity and adapt to dynamic environments. However, RL applications remain largely confined to simulations, with limited real-world deployments.
To bridge this gap, Euthermo project focuses on developing scalable and transferable deep RL algorithms for building energy management. These methods are validated on the NEST demonstration building at the Empa campus. Additionally, the integration of linked data to enhance transfer learning and scalability is explored.
People
Collaborators
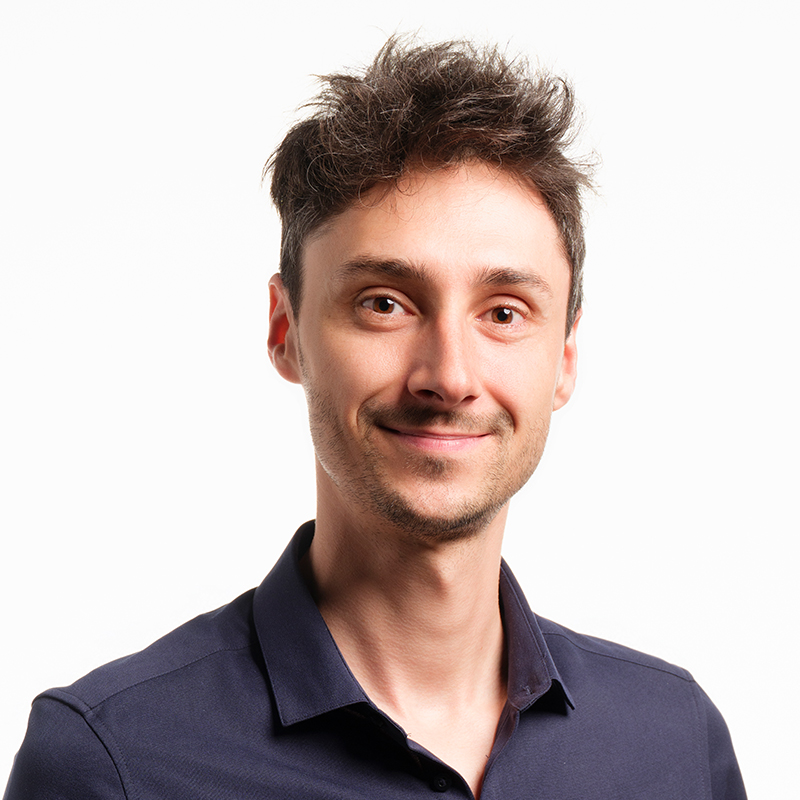
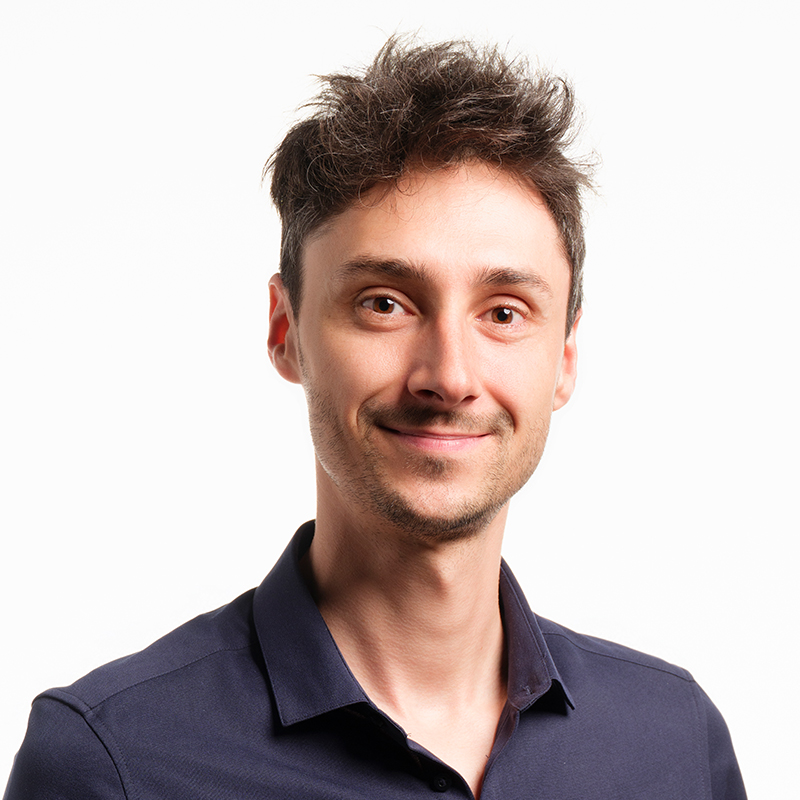
Carl holds a Ph.D in Mathematics from École des Ponts ParisTech and Université Gustave Eiffel in Paris. He has broad interests in statistics and stochastic control, and works on reinforcement learning, generative methods and time series forecasting, with applications in various domains such as energy, finance and physics. He worked with EDF R&D and Finance des Marchés de l’Energie (FiME) laboratory on applications of machine learning to risk management, including time series generation and deep hedging. He joined the SDSC in 2022 as a senior data scientist in the academic team at École Polytechnique Fédérale de Lausanne (EPFL).
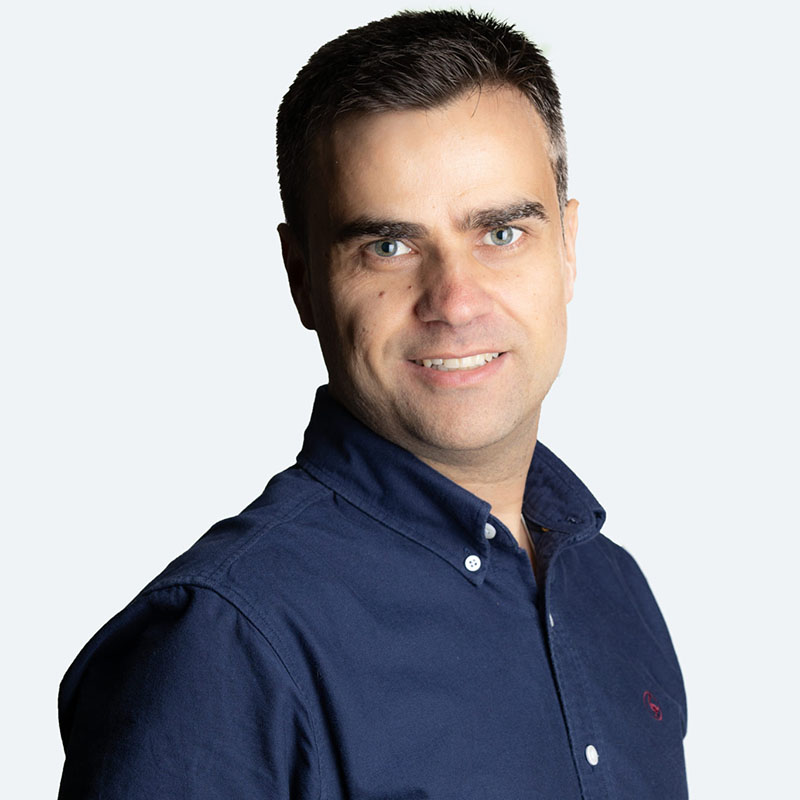
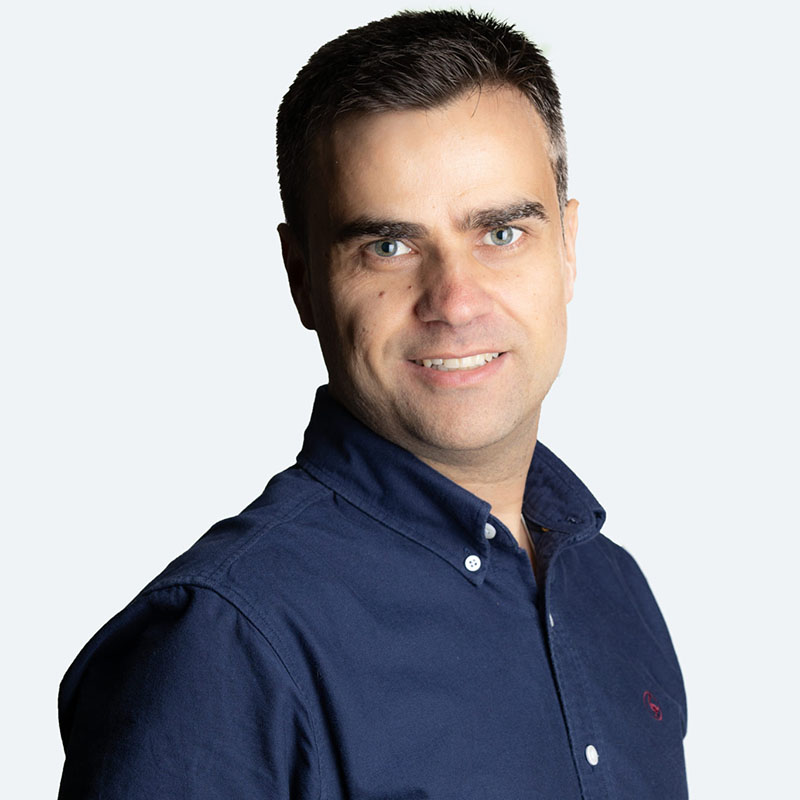
Benjamín Béjar received a PhD in Electrical Engineering from Universidad Politécnica de Madrid in 2012. He served as a postdoctoral fellow at École Polytechnique Fédérale de Lausanne until 2017, and then he moved to Johns Hopkins University where he held a Research Faculty position until Dec. 2019. His research interests lie at the intersection of signal processing and machine learning methods, and he has worked on topics such as sparse signal recovery, time-series analysis, and computer vision methods with special emphasis on biomedical applications. Since 2021, Benjamin leads the SDSC office at the Paul Scherrer Institute in Villigen.
PI | Partners:
Empa, Urban Energy Systems Laboratory:
- Dr. Bratislav Svetozarevic
- Dr. James Allan
- Philipp Heer
description
Motivation
This project addresses the inadequacy of existing control strategies, both conventional (i.e. industrial) ones and advanced ones based on physical models, to provide optimal control performance of modern buildings with integrated energy generation, storage, and EV charging capacities. The project aspires to deliver practical solutions with high technology readiness while contributing to the broader fields of machine learning and automatic control.
- Objective 1: performance of the learning control algorithms.
- Objective 2: scalability and transferability of learning control algorithms.
Proposed Approach / Solution
The proposed solution involves a modular reinforcement learning (RL) agent designed to handle the complexity of modern building energy systems and ensure easy transferability across buildings. To train the agent effectively, a physically consistent neural network is employed to model the building environment and dynamics. This environment model serves as a training simulator, allowing the RL agent to safely explore and learn optimal control strategies. Ultimately, the approach is validated under real-world conditions on the NEST demonstration building at Empa (see Figure 1). An illustrative example over a two-day episode showing room temperature, with heating control signals and weather conditions is provided Figure 2.
Impact
The outcomes of this project can accelerate the adoption of technologies that enhance occupant comfort while reducing energy consumption in buildings. They also facilitate the integration and management of renewable energy sources and electric vehicles within building systems.

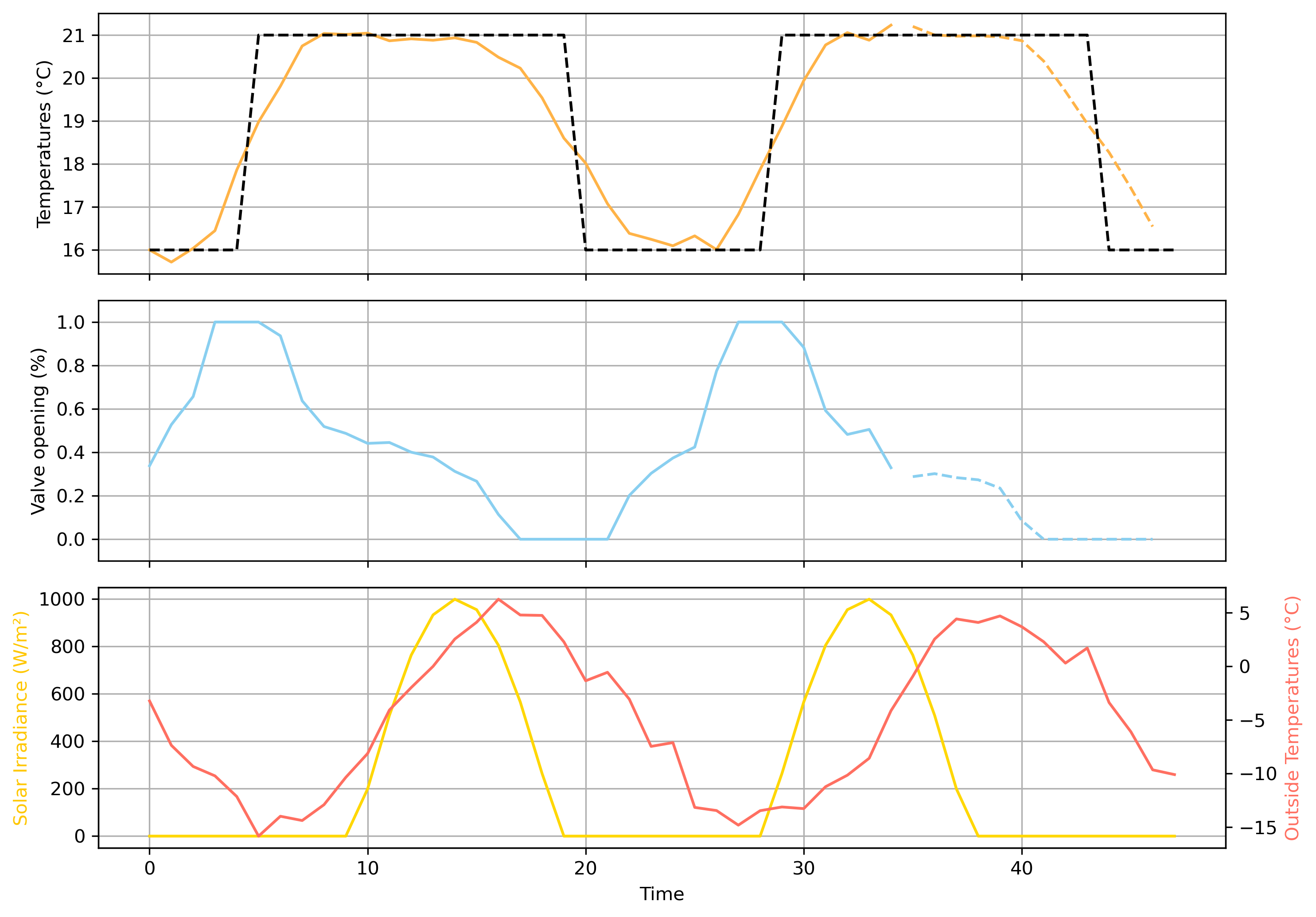
Presentation
Gallery
Annexe
Cover image source: Empa.ch
Additional resources
Bibliography
- Svetozarevic, B., Baumann, C., Muntwiler, S., Di Natale, L., Zeilinger, M. N., & Heer, P. (2022). Data-driven control of room temperature and bidirectional EV charging using deep reinforcement learning: Simulations and experiments. Applied Energy, 307, 118127.
- Di Natale, L., Svetozarevic, B., Heer, P., & Jones, C. N. (2021, November). Deep Reinforcement Learning for room temperature control: a black-box pipeline from data to policies. In Journal of Physics: Conference Series (Vol. 2042, No. 1, p. 012004). IOP Publishing.
- Di Natale, L., Svetozarevic, B., Heer, P., & Jones, C. N. (2022). Physically consistent neural networks for building thermal modeling: theory and analysis. Applied Energy, 325, 119806.
- Di Natale, L., Svetozarevic, B., Heer, P., & Jones, C. N. (2022, June). Near-optimal deep reinforcement learning policies from data for zone temperature control. In 2022 IEEE 17th International Conference on Control & Automation (ICCA) (pp. 698-703). IEEE.
Publications
Related Pages
More projects
MAGNIFY
News
Latest news
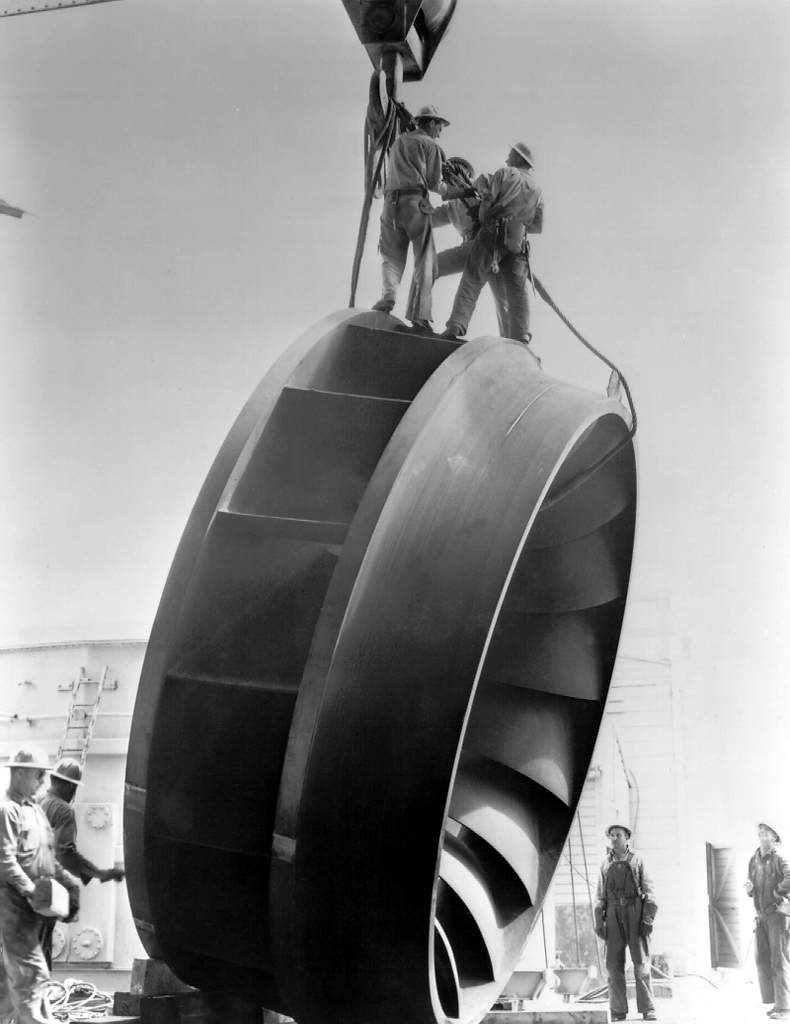
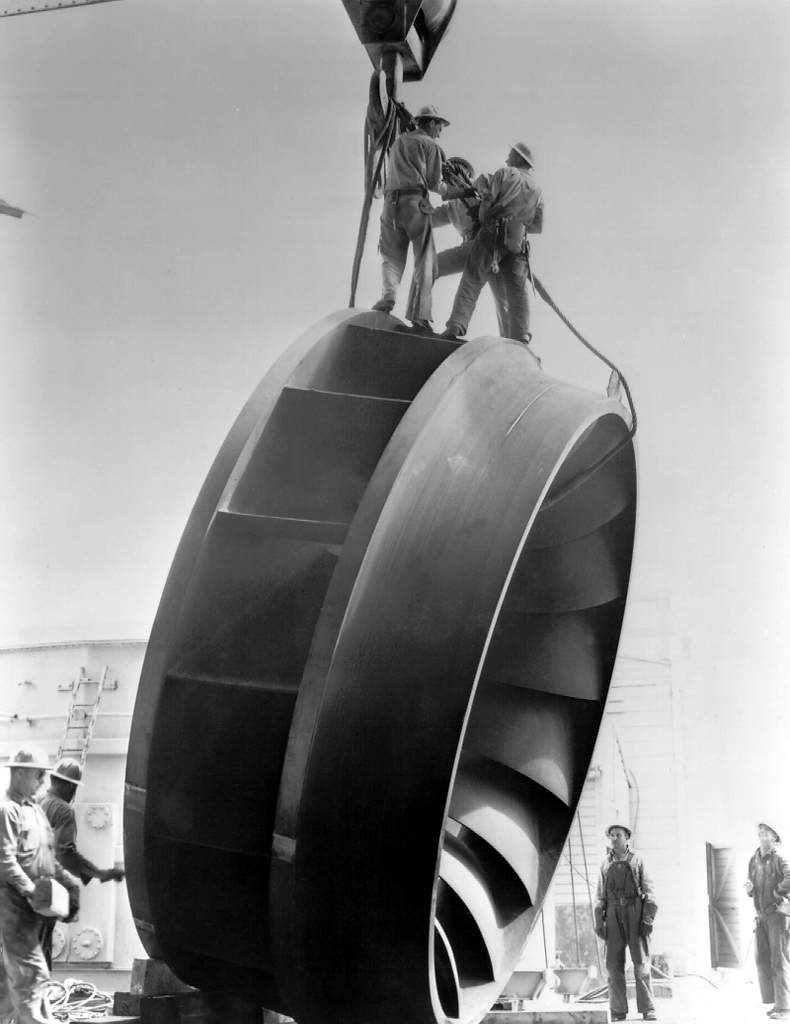
PAIRED-HYDRO | Increasing the Lifespan of Hydropower Turbines with Machine Learning
PAIRED-HYDRO | Increasing the Lifespan of Hydropower Turbines with Machine Learning


First National Calls: 50 selected projects to start in 2025
First National Calls: 50 selected projects to start in 2025
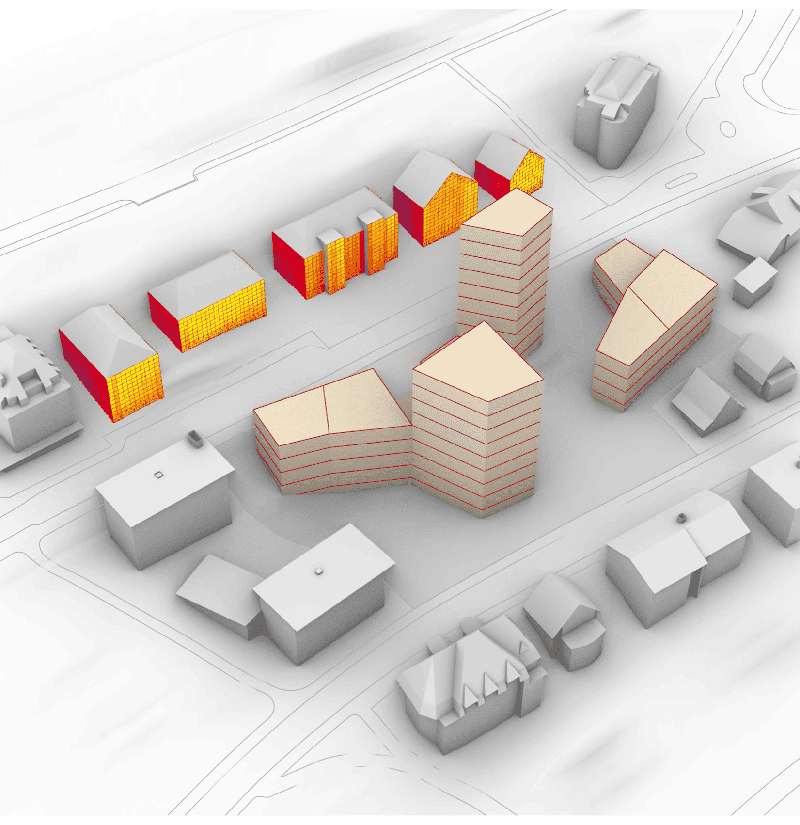
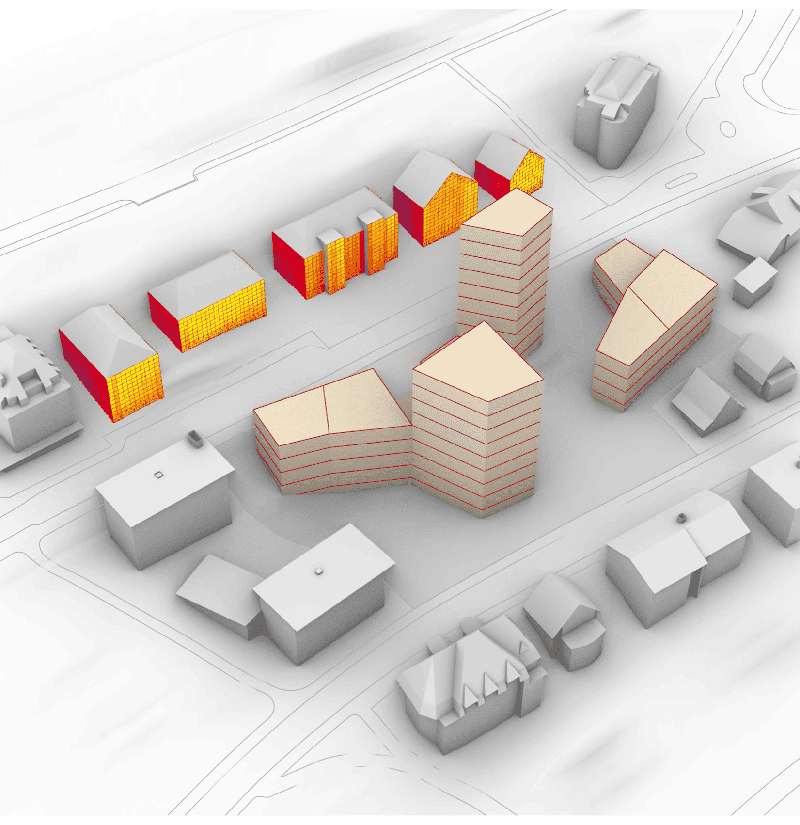
AIXD | Generative AI toolbox for architects and engineers
AIXD | Generative AI toolbox for architects and engineers
Contact us
Let’s talk Data Science
Do you need our services or expertise?
Contact us for your next Data Science project!