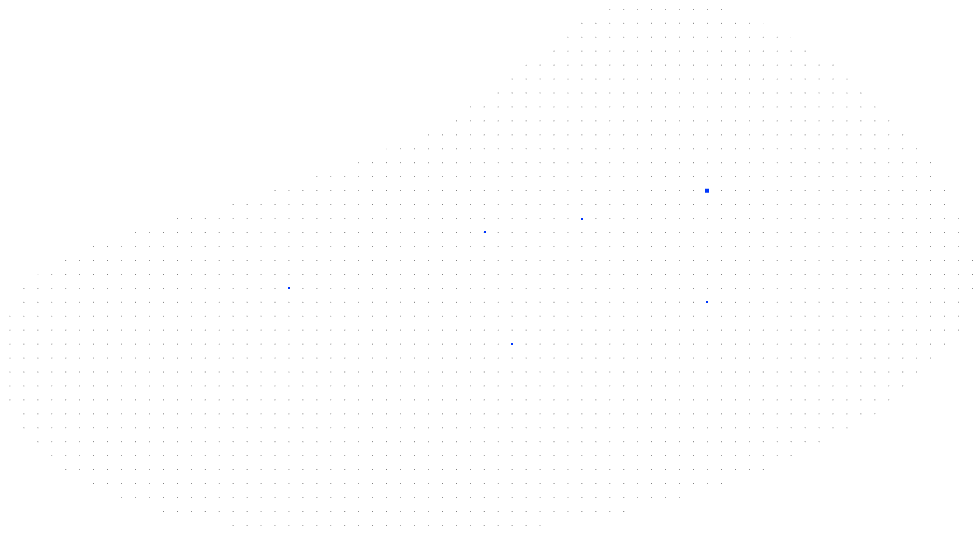
DLBIRHOUI
Deep Learning Based Image Reconstruction for Hybrid Optoacoustic and Ultrasound Imaging

Abstract
Over the last decade, the Razansky lab was instrumental in the development of multi-spectral optoacoustic tomography (MSOT), transforming this novel bio-imaging technology from the initial demonstration of technical feasibility, through establishment of image reconstruction methodologies all the way toward its clinical translation. The method rapidly finds its place as a potent clinical imaging tool due to its high sensitivity and molecular specificity as well as non-invasive, real-time and high-resolution volumetric imaging capabilities deep in living biological tissues. Despite great promise demonstrated in the pilot clinical studies, human imaging with MSOT is afflicted by a limited tomographic access to the region of interest while significant constraints are further imposed on the light deposition in deep tissues. This project aims to develop new artificial intelligence capabilities for improving image quality and diagnostic capacity of MSOT images acquired by sub-optimal scanner configurations resulting from, for example, application-related constraints or low cost design considerations. In particular, we will devise machine learning approaches to enable efficient and robust multimodal combination of MSOT with pulse-echo ultrasonography by training neural networks on high-resolution and high-quality training datasets generated by dedicated optimally designed scanner configurations. The trained models will be used to restore quality of artifactual images produced by various sub-optimal scanner configurations with limited tomographic view or sparsely acquired data in typical clinical imaging scenarios. Those advancements will help reducing inter-clinician variability and enable a more efficient, rapid, and objective analysis of large amounts of image data, thus relaxing requirements for specialized training and facilitating the wider adoption of MSOT apparatus in primary care and other non-hospital settings.
People
Collaborators


Anna joined the SDSC as a Senior Data Scientist in 2020. She is a statistician by training with a Master’s degree with Honors in Mathematical Statistics from Lomonosov Moscow State University. Anna has graduated with a PhD from ETH Zurich in 2018, where she worked on causal structure learning for protein signaling pathways. During her studies she did an internship at Facebook AI Research in New York working on discovery of hierarchies from data using hyperbolic geometry. Later she joined Facebook AI Research in Paris as a postdoctoral researcher, where she worked on a problem of out-of-distribution prediction of unseen drug combinations. Broadly her research interests are in unsupervised and self-supervised learning, domain adaptation and generalisation.
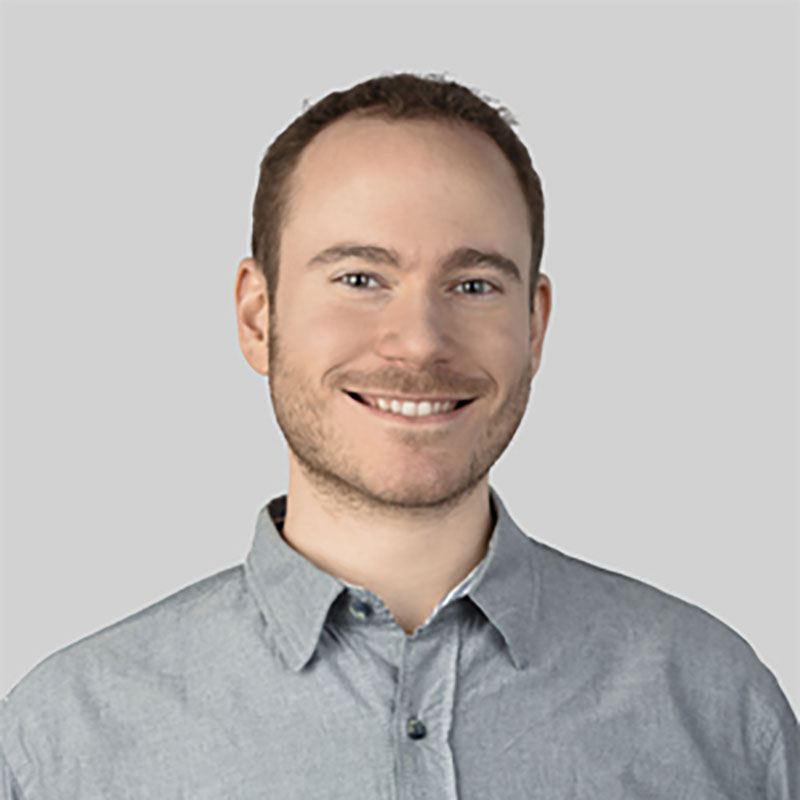
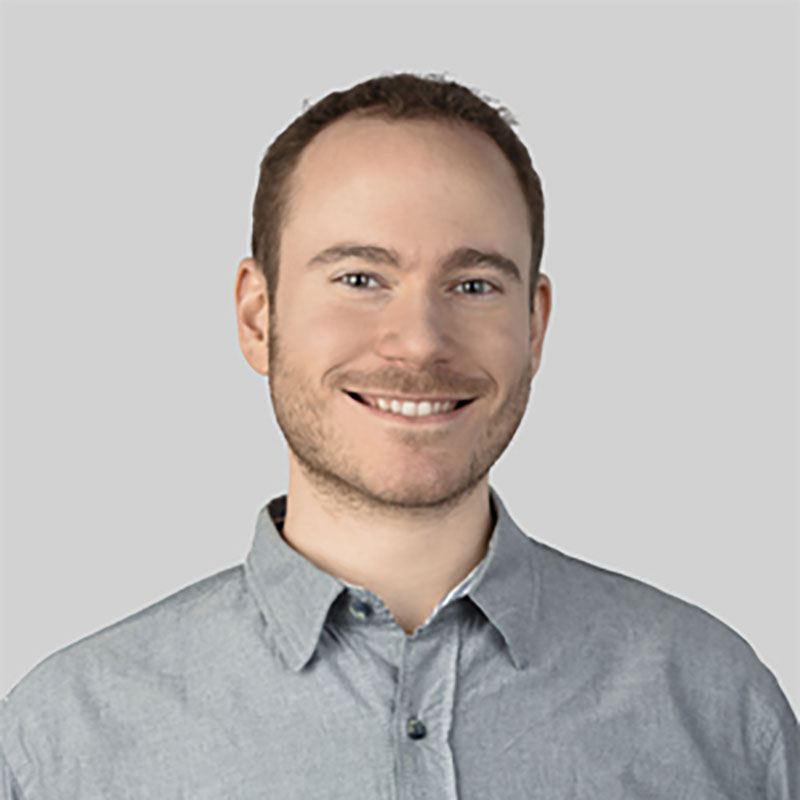
Firat completed his undergraduate studies in Electronics Engineering at Sabanci University. He later received his MSc. in Electrical and Electronics Engineering from EPFL. He conducted his doctoral studies on medical image segmentation in Computer Vision Lab at ETH Zurich. In between, he visited INRIA (Sophia Antipolis, France) and ABB Corporate Research Center (Baden, Switzerland). His research interests revolve around computer vision and machine learning, with a focus on the medical domain. He has been with SDSC since 2019.
PI | Partners:
description
Motivation
As an emerging non-invasive imaging modality optoacoustic (OA) imaging has a lot of potential in clinical and pre-clinical applications (Figure 1). Unleashing this potential relies on overcoming challenges from limited view tomographic reconstruction to various forms of noise. The project tackles these challenges with several data-driven approaches.
Proposed Approach / Solution
A first of its kind, large scale, diverse, user-friendliness oriented, in vivo and simulated optoacoustic data (Experimental and Synthetic Clinical Optoacoustic Data: OADAT) has been publicly released along with a multitude of demos to use OADAT for data science applications (Figures 2 and 3). Additional proposed solutions include neural network-based methodologies for producing high fidelity limited view and sparse view OA image reconstruction as well as exploiting raw OA signals to improve reconstruction of limited view OA images.
Impact
OA imaging requires significant know-how and hardware to acquire data, causing the research to be limited to a few groups. The released dataset, OADAT, not only breaks the entry barrier for the broader community to develop solutions for the OA imaging field, but also serves as a benchmark for a multitude of OA downstream tasks, which the community can build upon and compare their methodologies.
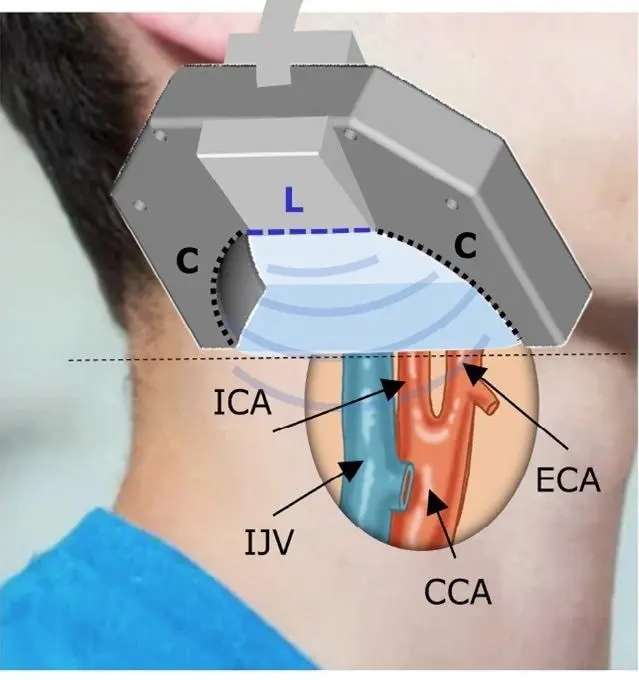


Presentation
Gallery
Annexe
Additional resources
Bibliography
- Steinberg, I., Huland, D. M., Vermesh, O., Frostig, H. E., Tummers, W. S., & Gambhir, S. S. (2019). Photoacoustic clinical imaging. Photoacoustics, 14, 77-98. doi: 10.1016/j.pacs.2019.05.001
- Su, J. L., Wang, B., Wilson, K. E., Bayer, C. L., Chen, Y. S., Kim, S., ... & Emelianov, S. Y. (2010). Advances in clinical and biomedical applications of photoacoustic imaging. Expert opinion on medical diagnostics, 4(6), 497-510. doi: 10.1517/ 17530059.2010.529127
- Lafci, B., Merčep, E., Herraiz, J. L., Dean-Ben, X. L., & Razansky, D. (2020). Noninvasive multiparametric characterization of mammary tumors with transmission-reflection optoacoustic ultrasound. Neoplasia, 22(12), 770-777. doi: https://doi.org/10.1016/j.neo.2020.10.008
- Merčep, E., Burton, N. C., Claussen, J., & Razansky, D. (2015). Whole-body live mouse imaging by hybrid reflection-mode ultrasound and optoacoustic tomography. Optics letters, 40(20), 4643-4646. doi: 10.1364/OL.40.004643.
- Merčep, E., Deán-Ben, X. L., & Razansky, D. (2018). Imaging of blood flow and oxygen state with a multi-segment optoacoustic ultrasound array. Photoacoustics, 10, 48-53. doi: https://doi.org/10.1016/j.pacs.2018.04.002
Publications
Related Pages
Project repositories and pages
More projects
MAGNIFY
News
Latest news
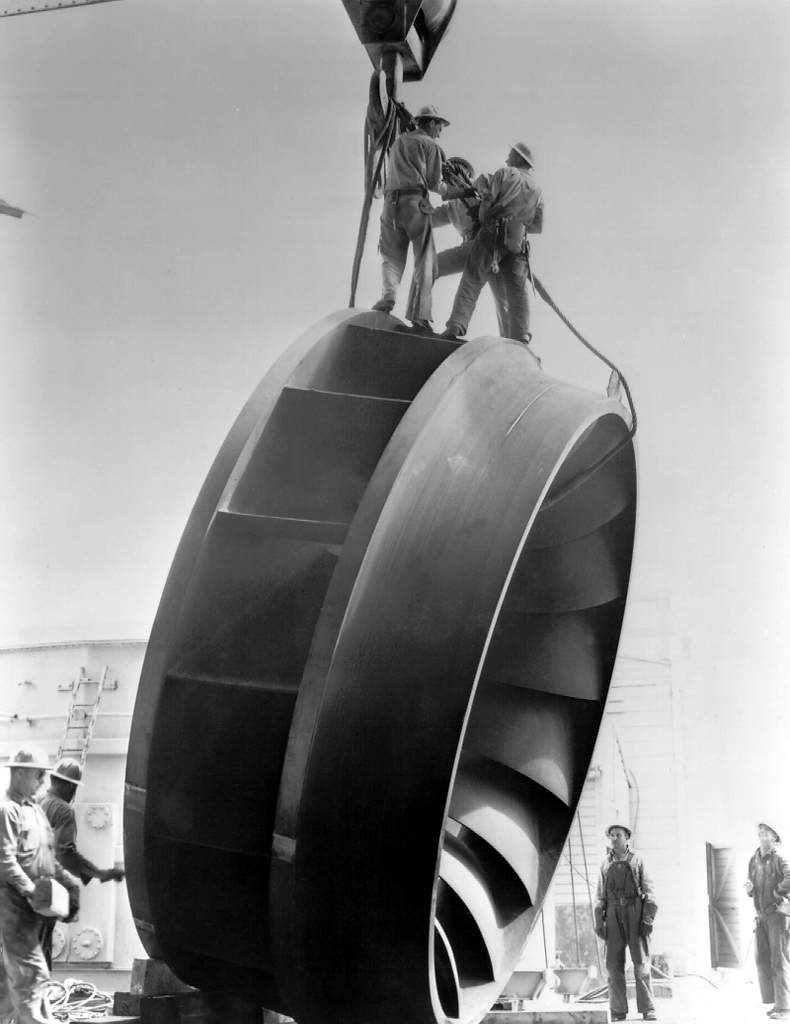
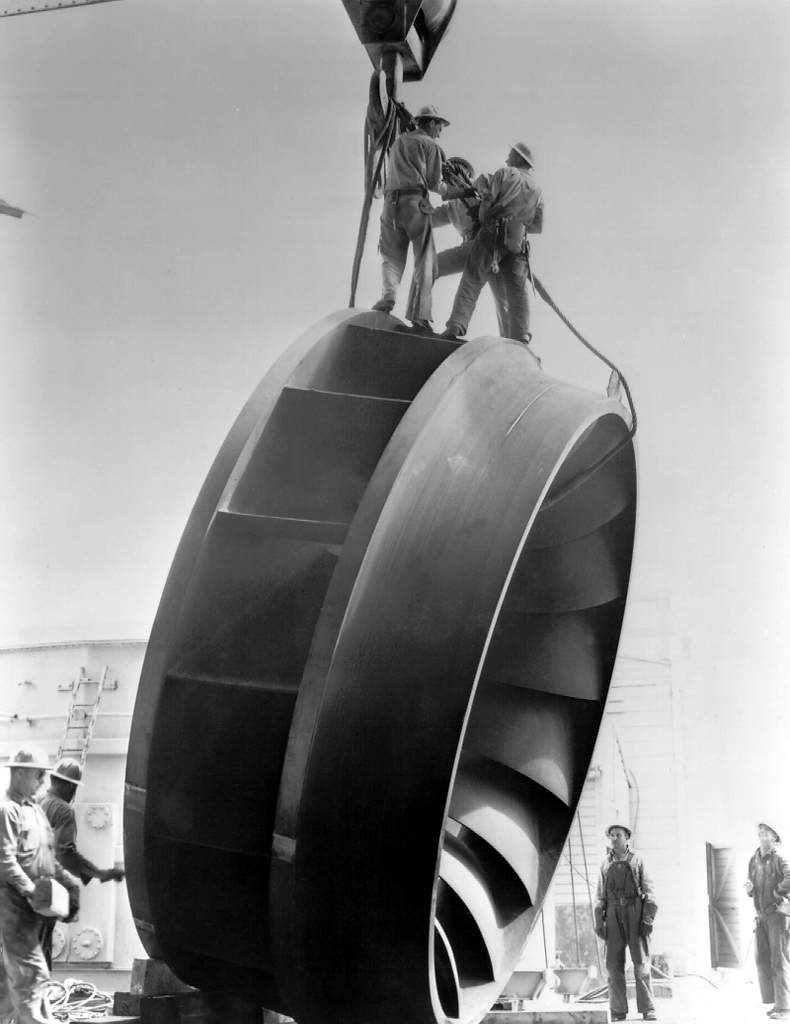
PAIRED-HYDRO | Increasing the Lifespan of Hydropower Turbines with Machine Learning
PAIRED-HYDRO | Increasing the Lifespan of Hydropower Turbines with Machine Learning


First National Calls: 50 selected projects to start in 2025
First National Calls: 50 selected projects to start in 2025
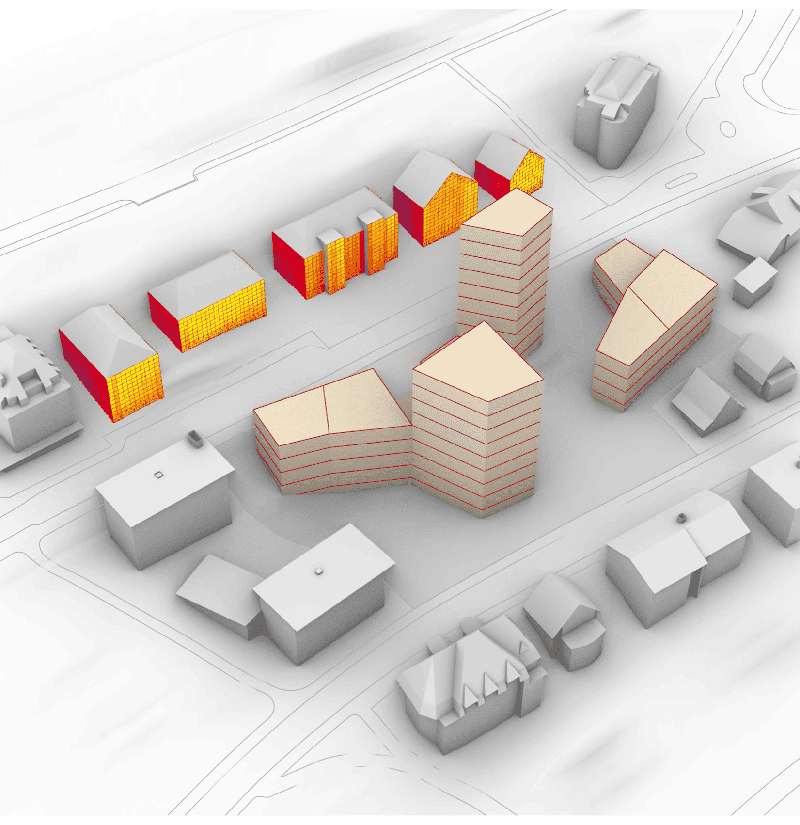
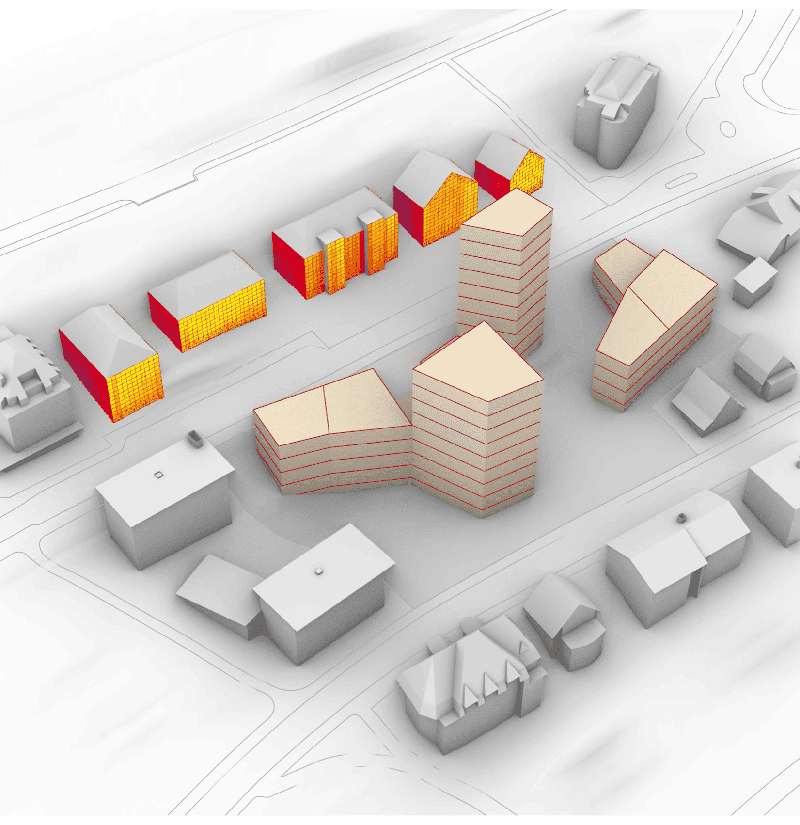
AIXD | Generative AI toolbox for architects and engineers
AIXD | Generative AI toolbox for architects and engineers
Contact us
Let’s talk Data Science
Do you need our services or expertise?
Contact us for your next Data Science project!