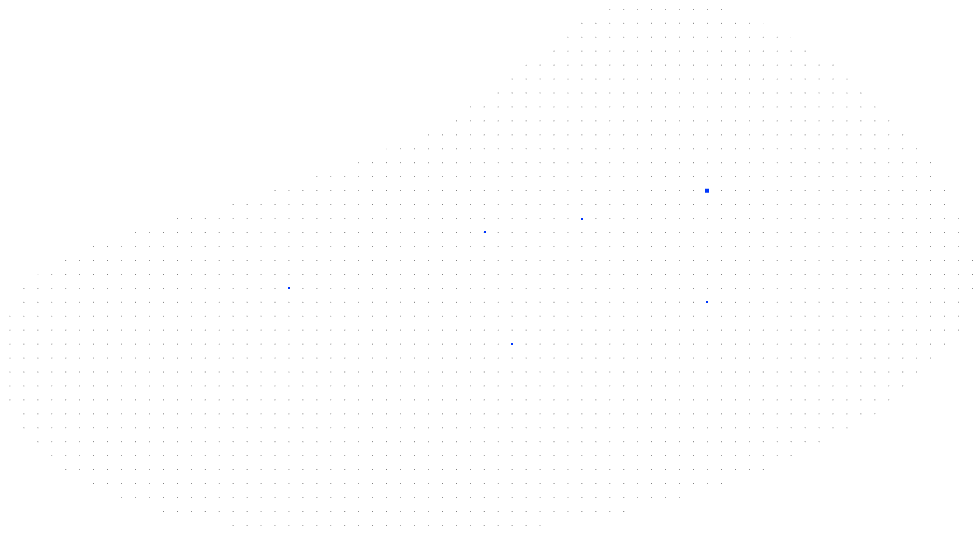
DIMPEO
Detecting drought impacts on forests in earth observation data

Abstract
Extremely dry and hot summers in recent years have caused significant damage to forests in Switzerland and neighboring regions. Tree mortality and premature defoliation can be detected by satellite-based remote sensing as an anomalous “browning” of the vegetated land surface. To better detect and understand the drivers of these impacts, this project will develop a multivariate anomaly detection method for identifying and characterizing forest browning events in high-resolution Earth Observation (EO) data. We will use Sentinel-2 satellite imagery, climate reanalysis data, vegetation height and composition to detect spatio-temporal anomalies in vegetation greenness and relate them to different drivers, including droughts, late frost, deforestation, or storm damage. The project will address the challenges of identifying anomalies in large and noisy EO data, accounting for the heterogeneity of the land surface, phenology, vegetation types, and different browning drivers, including drought. A better understanding of the timing, location, magnitude, and drivers of forest browning will pave the way for future research, developing predictive methods in a near-range (weeks-months lead time) drought impact and/or forest fire forecasting context.
People
Collaborators
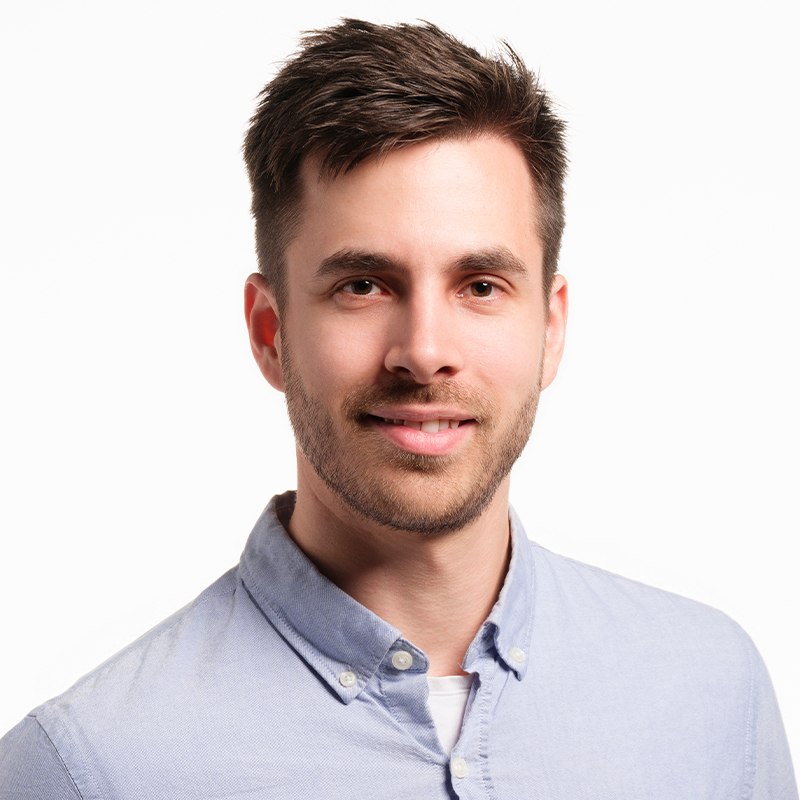
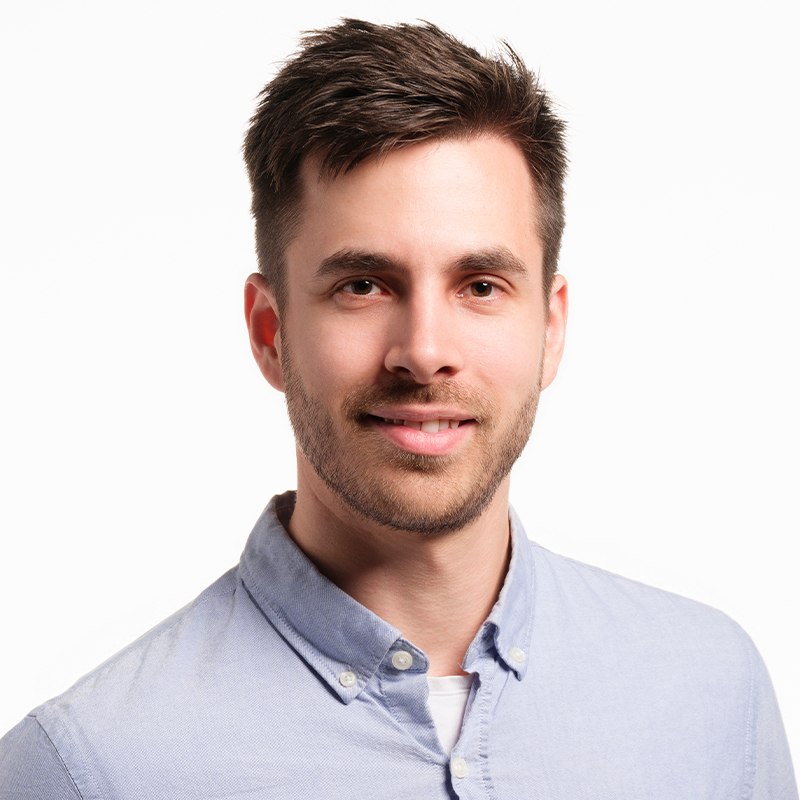
David joined SDSC in February 2024. Before that, he completed his doctorate in computer vision at ETH Zürich. His doctoral thesis focused on multi-task learning and domain adaptation in neural networks for applications in visual recognition. In addition, he gained research experience in transfer learning and multi-modal learning. David’s current work at SDSC ranges from large-scale vegetation modeling based on satellite imaging to analysis of electronic health records with deep learning methods.
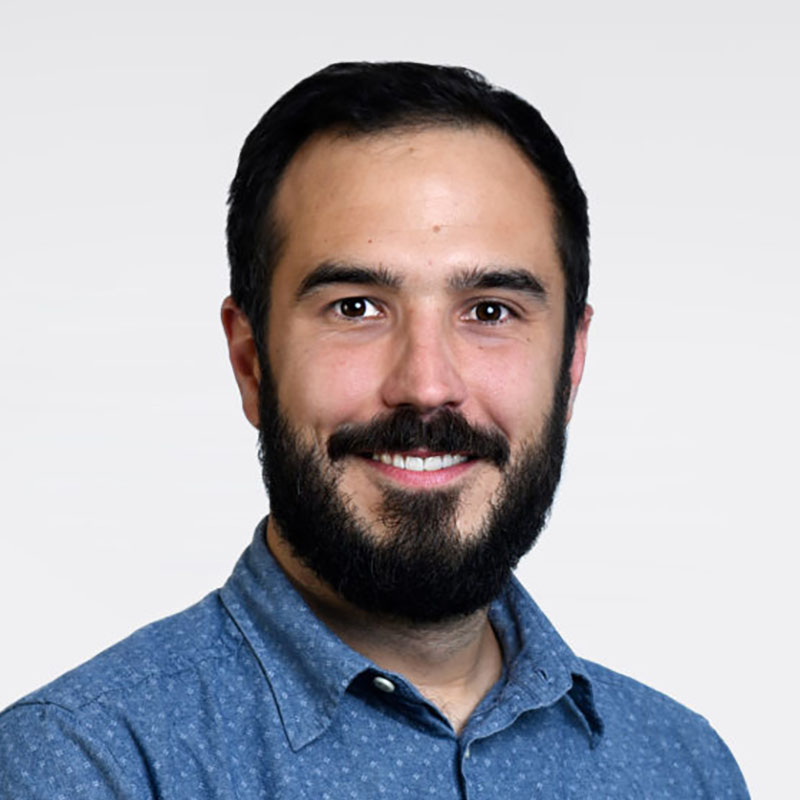
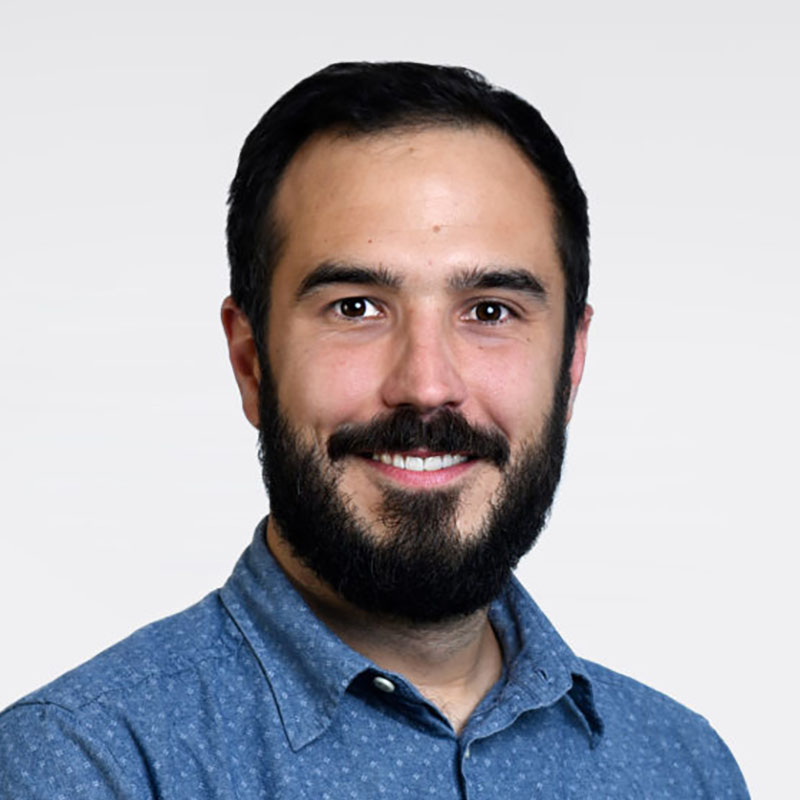
Michele received a Ph.D. in Environmental Sciences from the University of Lausanne (Switzerland) in 2013. He was then a visiting postdoc in the CALVIN group, Institute of Perception, Action and Behaviour of the School of Informatics at the University of Edinburgh, Scotland (2014-2016). He then joined the Multimodal Remote Sensing and the Geocomputation groups at the Geography department of the University of Zurich, Switzerland (2016-2017). His main research activities were at the interface of computer vision, machine and deep learning for the extraction of information from aerial photos, satellite optical images and geospatial data in general.
PI | Partners:
University of Bern, Geocomputation and Earth Observation Group:
- Prof. Benjamin Stocker
- Samantha Biegel
- Nils Tinner
description
Motivation
In recent years, the forest damage caused by dry and hot summers has become a pressing concern for multiple stakeholders in Switzerland and neighboring regions. Understanding where and when climate extremes affect forest health and vitality is a crucial step for developing forecasting and early warning systems. Satellite-based remote sensing provides a powerful tool for monitoring forests, enabling the detection of anomalous “browning”, i.e. an unexpected decrease in plant growth or sudden reduction in plant productivity, of the vegetated land surface. However, working with large and noisy Earth Observation (EO) data presents challenges, so how can we accurately identify these anomalies? This project aims to implement a robust pipeline for detecting land surface browning anomalies using high-resolution EO time series data. By doing so, we can map and quantify the impacts of summer droughts on Swiss forests and identify drivers of browning events.
Proposed Approach / Solution
In the first step, suitable algorithms for greenness anomaly detection are identified and implemented. Surface greenness depends on the seasons, elevation, exposition, location, and the terrain, so these factors will have to be accounted for. The resulting anomaly detection model should scale to the high-resolution EO data covering the entirety of the Swiss forests. In the second step, the detected anomalies will be attributed to ecological drivers by relating them with respect to additional covariates, such as summer droughts, late frost, deforestation, and storm damage.
Impact
Developing capacity for drought impact monitoring and near-term forecasting (“early warning”, weeks-months lead time) has been declared a high priority by the Swiss Federal Council and a national program for its implementation has recently been launched as part of the National Centre for Climate Services (NCCS). Methodological developments achieved by this project will enable an open-access, robust, transparent, and updatable drought impact detection and quantification workflow and will serve to improve methods in drought impact and forest fire forecasting, achieved in future work.
Presentation
Gallery
Annexe
Additional resources
Bibliography
- Brun, P., Psomas, A., Ginzler, C., Thuiller, W., Zappa, M., & Zimmermann, N. E. (2020). Large‐scale early‐wilting response of Central European forests to the 2018 extreme drought. Global change biology, 26(12), 7021-7035.
- Sturm, J., Santos, M. J., Schmid, B., & Damm, A. (2022). Satellite data reveal differential responses of Swiss forests to unprecedented 2018 drought. Global Change Biology, 28(9), 2956-2978.
Publications
Related Pages
More projects
ML-L3DNDT
BioDetect
News
Latest news


Smartair | An active learning algorithm for real-time acquisition and regression of flow field data
Smartair | An active learning algorithm for real-time acquisition and regression of flow field data
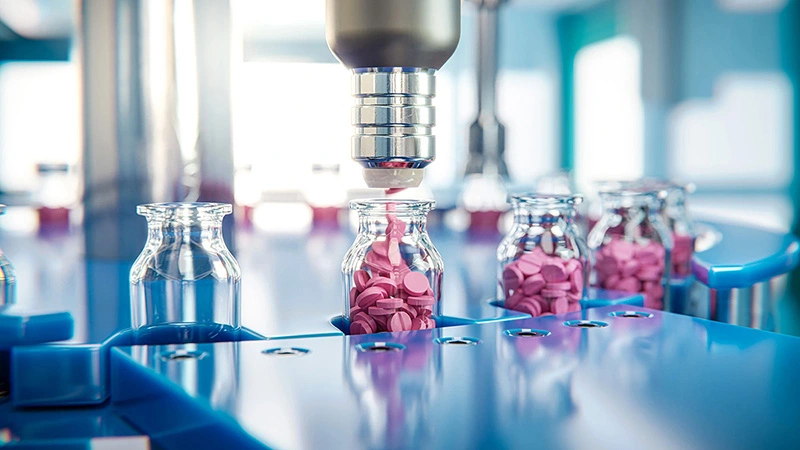
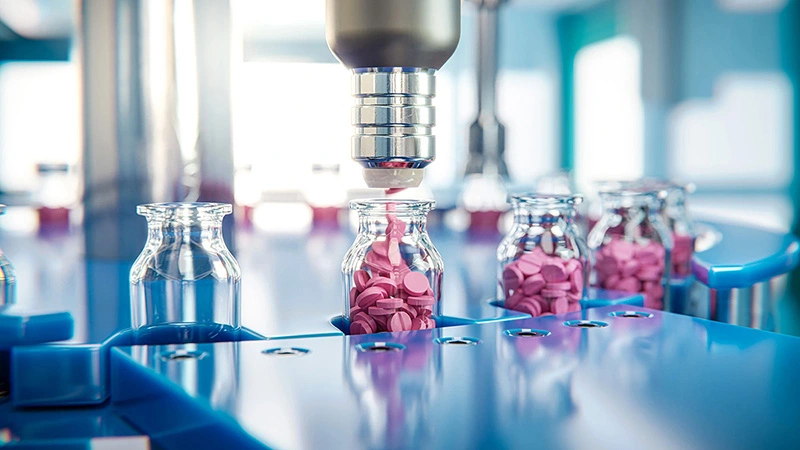
The Promise of AI in Pharmaceutical Manufacturing
The Promise of AI in Pharmaceutical Manufacturing


Efficient and scalable graph generation through iterative local expansion
Efficient and scalable graph generation through iterative local expansion
Contact us
Let’s talk Data Science
Do you need our services or expertise?
Contact us for your next Data Science project!