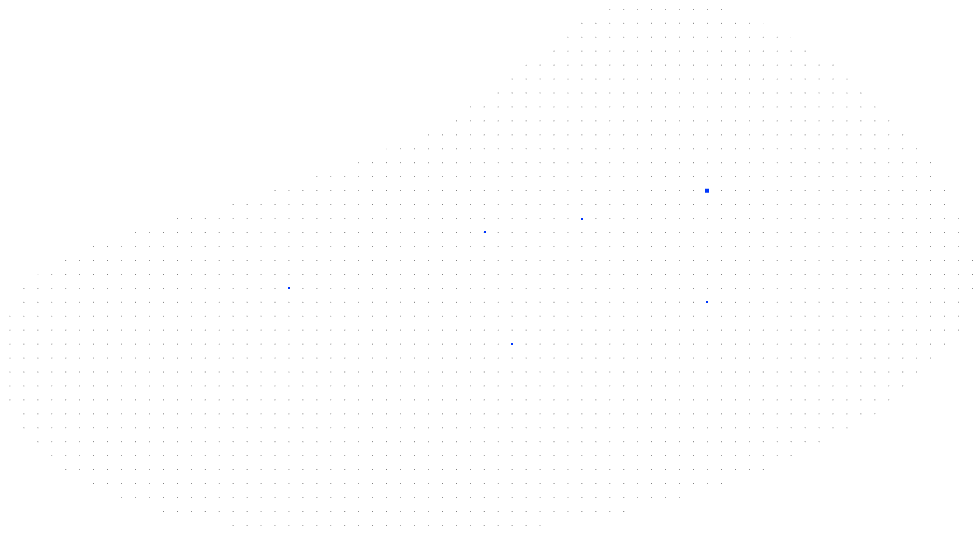
Dataspectrum4CVD
Integrating medical image data and assessments for personalized cardiovascular risk estimation

Abstract
Atherosclerotic cardiovascular disease (CVD) remains the leading global cause of mortality, accounting for 31% of all deaths and 16% of healthcare costs in Switzerland alone. Notably, up to 30% of cardiovascular events can be prevented through effective lipid-lowering therapies and other interventions. Current guidelines for prescribing such treatments rely on risk-based algorithms, yet there is significant room for improving the accuracy and utility of these models.
Recent advancements in understanding the mechanisms underlying cardiovascular risk have highlighted the importance of novel risk factors such as coronary artery calcification, arterial stiffness, elevated lipoprotein(a) levels, physical inactivity, and obesity. Integrating these factors into predictive modeling holds promise for more precise risk stratification. This project aims to improve cardiovascular risk prediction by harnessing multi-modal patient data—including cardiac MRI, ECGs, demographics, biomarkers, and vitals—from the extensive UK Biobank cohort. Through self-supervised learning, we plan to train deep neural networks on this large-scale dataset, paving the way for innovative approaches to early detection and prevention of CVD.
This project is co-funded by PHRT.
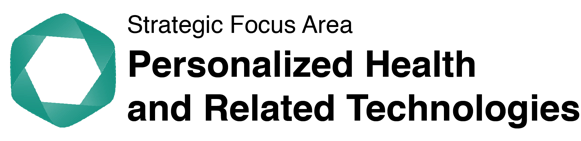
People
Collaborators
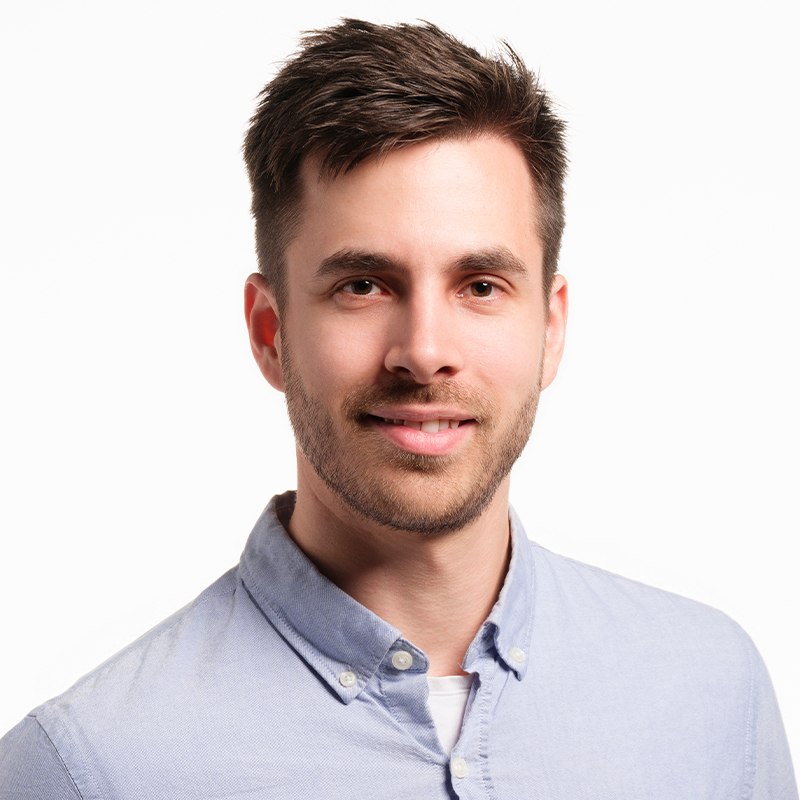
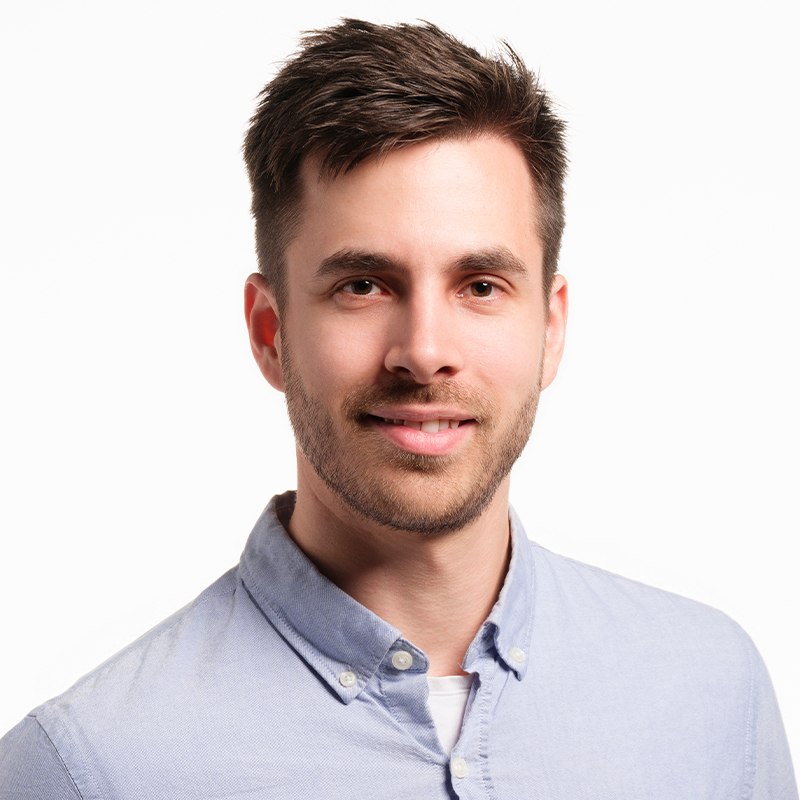
David joined SDSC in February 2024. Before that, he completed his doctorate in computer vision at ETH Zürich. His doctoral thesis focused on multi-task learning and domain adaptation in neural networks for applications in visual recognition. In addition, he gained research experience in transfer learning and multi-modal learning. David’s current work at SDSC ranges from large-scale vegetation modeling based on satellite imaging to analysis of electronic health records with deep learning methods.
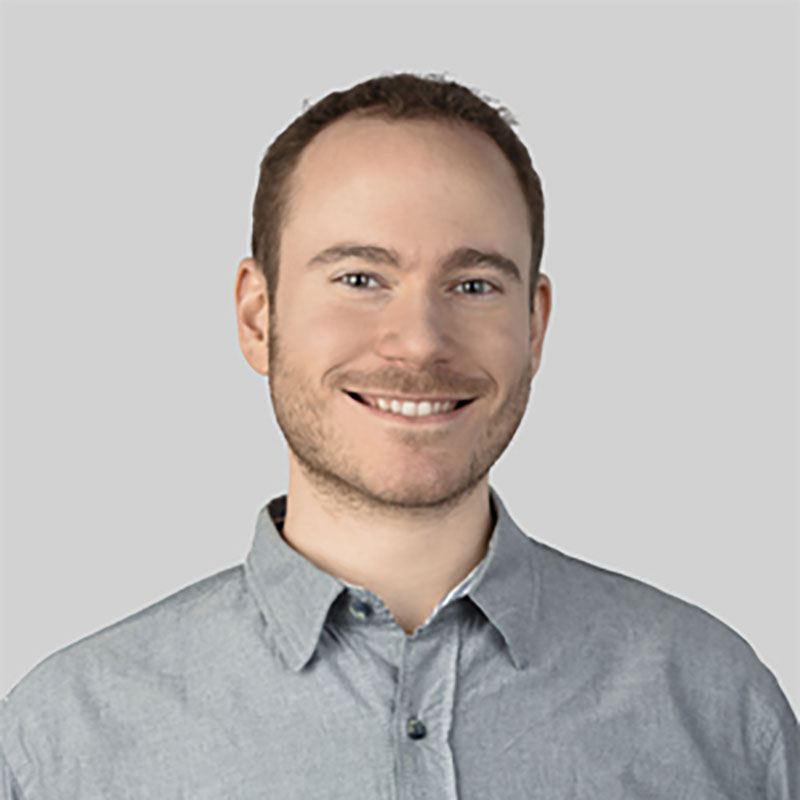
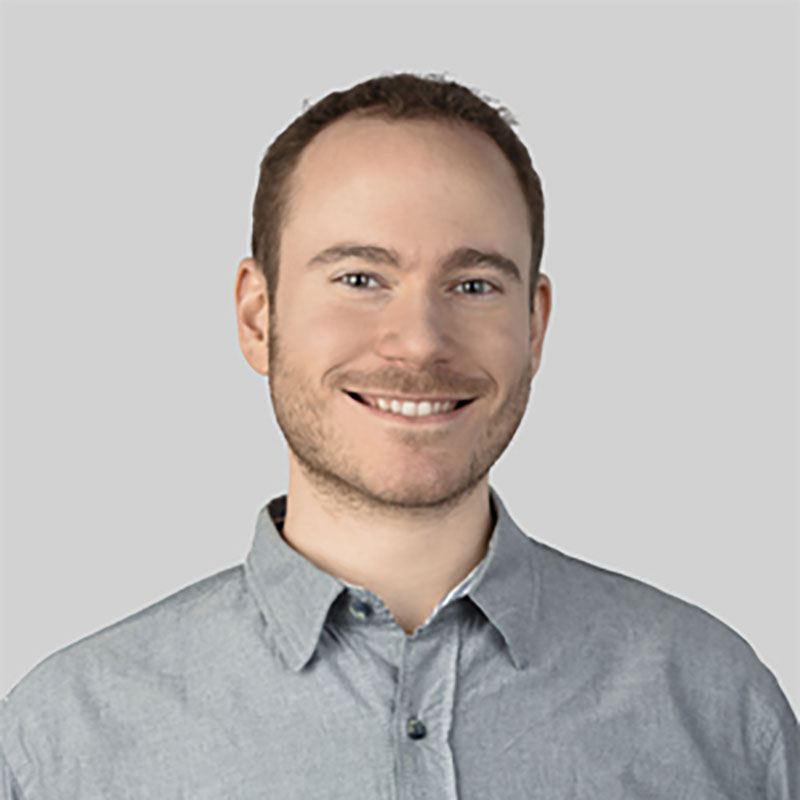
Firat completed his undergraduate studies in Electronics Engineering at Sabanci University. He later received his MSc. in Electrical and Electronics Engineering from EPFL. He conducted his doctoral studies on medical image segmentation in Computer Vision Lab at ETH Zurich. In between, he visited INRIA (Sophia Antipolis, France) and ABB Corporate Research Center (Baden, Switzerland). His research interests revolve around computer vision and machine learning, with a focus on the medical domain. He has been with SDSC since 2019.
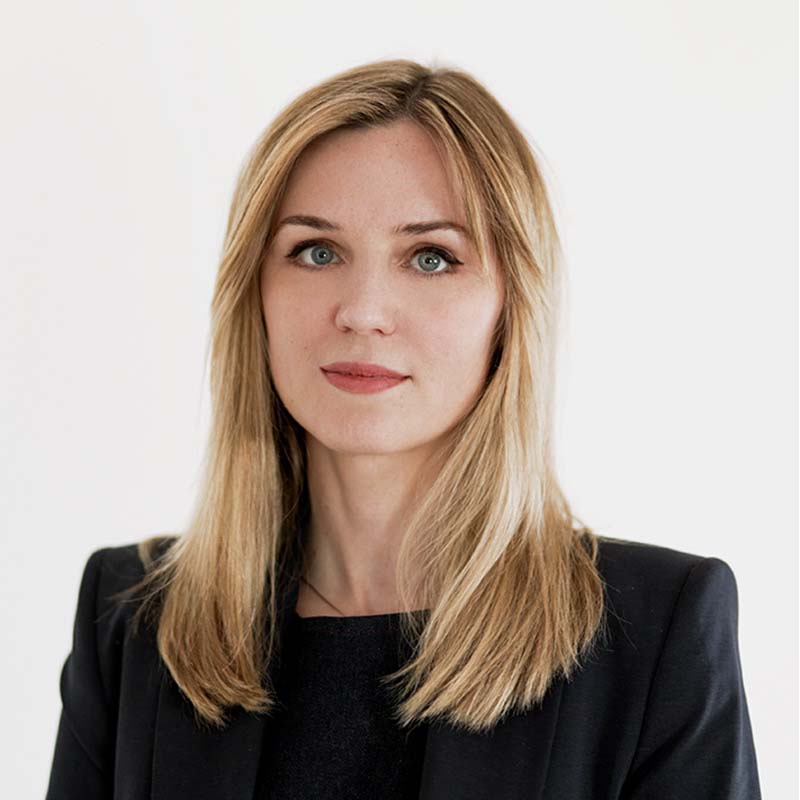
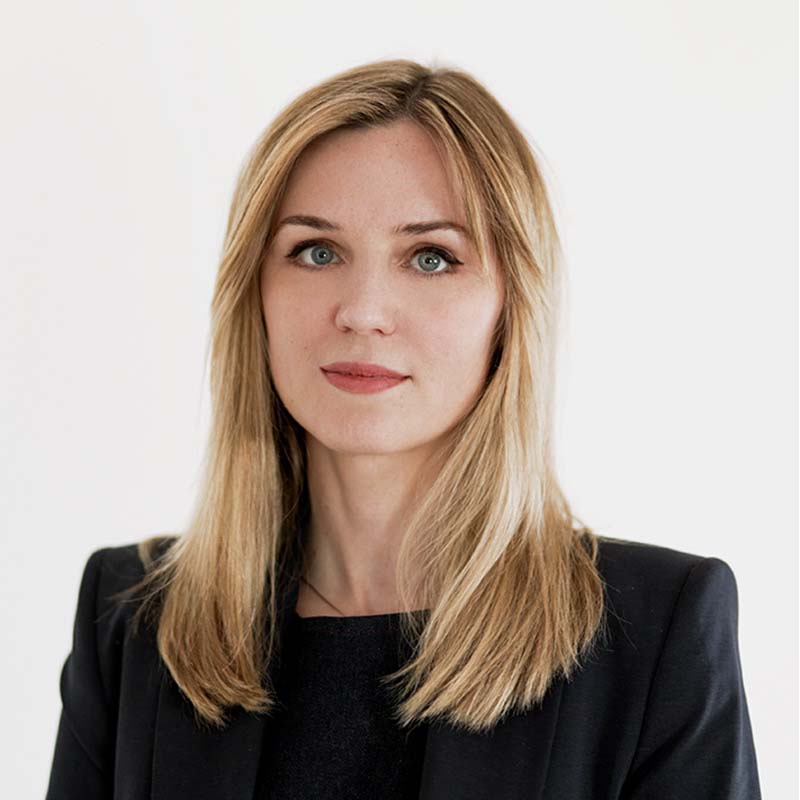
Ekaterina received her PhD in Computer Science from Moscow Institute for Physics and Technology, Russia. Afterwards, she worked as a researcher at the Institute for Information Transmission Problems in Moscow and later as a postdoctoral researcher in the Stochastic Group at the Faculty of Mathematics at University Duisburg-Essen, Germany. She has experience with various applied projects on signal processing, predictive modelling, macroeconomic modelling and forecasting, and social network analysis. She joined the SDSC in November 2019. Her interests include machine learning, non-parametric statistical estimation, structural adaptive inference, and Bayesian modelling.


Mathieu Salzmann is the Deputy Chief Data Scientist of the Swiss Data Science Center and a Senior Scientist and Lecturer at EPFL. He received his PhD from EPFL in 2009 and, between 2009 and 2015, has held post-doctoral and researcher positions at UC Berkeley, TTI-Chicago, and NICTA and the Australian National University. From May 2020 to February 2024, he was also a part-time Senior GNC Engineer at ClearSpace. Mathieu Salzmann has broad interests in machine learning and deep learning, with a particular focus on computer vision. He has published over 100 articles at top-tier peer-reviewed machine learning and computer vision venues and is a strong believer in collaborative work.
PI | Partners:
description
Motivation
Cardiovascular disease (CVD), particularly atherosclerotic forms, remains a critical public health challenge and the leading cause of mortality worldwide. In Switzerland, it accounts for nearly one-third of deaths and a substantial share of healthcare costs. Despite the availability of preventive treatments, current risk-based approaches often fail to capture the complexity of cardiovascular risk mechanisms. This underscores the urgent need for innovative models that integrate broader factors to improve decision-making and reduce the societal and economic toll of CVD.
Proposed Approach
The UK Biobank cohort offers an unparalleled resource with comprehensive medical data for up to 100,000 patients. This dataset includes modalities such as cardiac MRI and ECGs, as well as tabular variables encompassing demographic information, biomarkers, and vitals. To fully leverage this heterogeneous data, we propose developing a unified, patient-specific representation of cardiac health that encapsulates the multifaceted cardiac state of each individual. To achieve this, we will employ self-supervised learning methodologies, including masked autoencoders and joint embedding predictive architectures (JEPA), tailored to train large-scale transformer models. These techniques are capable of extracting meaningful patterns and relationships within multimodal datasets without requiring labeled data, thereby maximizing the utility of the UK Biobank data. Once developed, this unified cardiac representation will be rigorously evaluated for its predictive power in detecting and forecasting various cardiovascular diseases, providing a tool for personalized risk stratification and early intervention.
Impact
By improving cardiovascular risk prediction, this project could enable earlier diagnosis and personalized interventions, reducing the prevalence of CVD and enhancing patient outcomes. Incorporating novel risk factors into predictive models also offers deeper insights into disease mechanisms and better resource allocation in healthcare. These advancements hold the potential for widespread clinical, societal, and economic benefits globally.
Presentation
Gallery
Annexe
Cover image source: Adobe Stock
Additional resources
Bibliography
- Greenland, P., LaBree, L., Azen, S. P., Doherty, T. M., & Detrano, R. C. (2004). Coronary artery calcium score combined with Framingham score for risk prediction in asymptomatic individuals. Jama, 291(2), 210-215.
- Mitchell, G. F., Hwang, S. J., Vasan, R. S., Larson, M. G., Pencina, M. J., Hamburg, N. M., ... & Benjamin, E. J. (2010). Arterial stiffness and cardiovascular events: the Framingham Heart Study. Circulation, 121(4), 505-511.
- Antonopoulos, A. S., Sanna, F., Sabharwal, N., Thomas, S., Oikonomou, E. K., Herdman, L., ... & Antoniades, C. (2017). Detecting human coronary inflammation by imaging perivascular fat. Science translational medicine, 9(398), eaal2658.
- Littlejohns, T. J., Holliday, J., Gibson, L. M., Garratt, S., Oesingmann, N., Alfaro-Almagro, F., ... & Allen, N. E. (2020). The UK Biobank imaging enhancement of 100,000 participants: rationale, data collection, management and future directions. Nature communications, 11(1), 2624.
Publications
Related Pages
More projects
MAGNIFY
News
Latest news
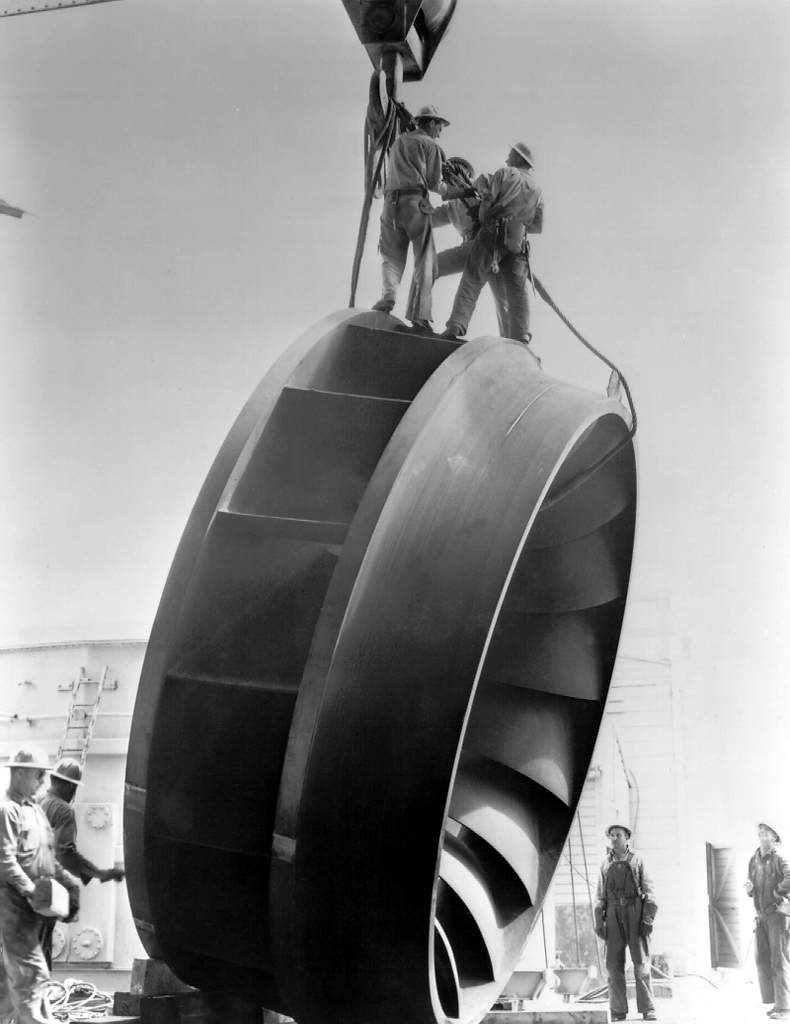
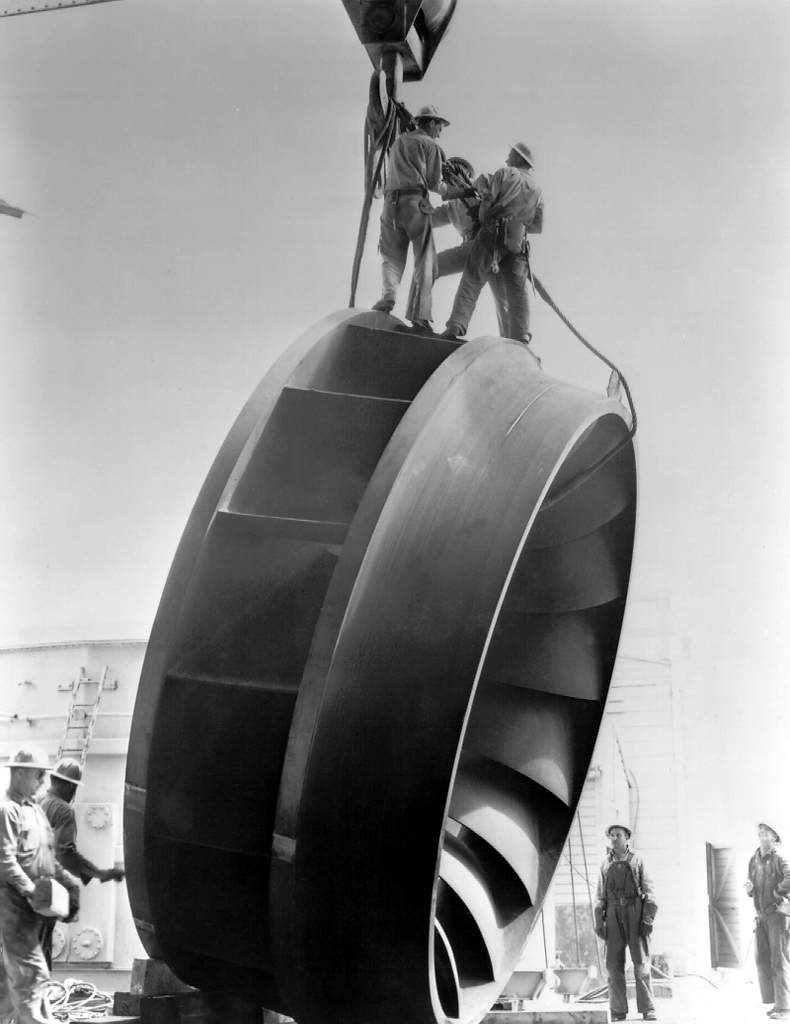
PAIRED-HYDRO | Increasing the Lifespan of Hydropower Turbines with Machine Learning
PAIRED-HYDRO | Increasing the Lifespan of Hydropower Turbines with Machine Learning


First National Calls: 50 selected projects to start in 2025
First National Calls: 50 selected projects to start in 2025
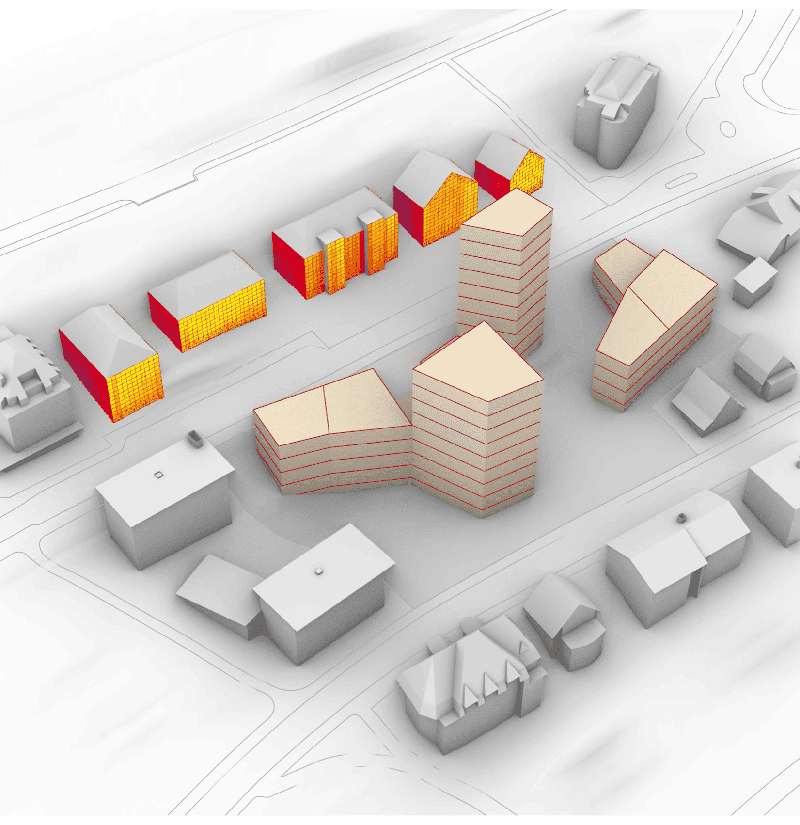
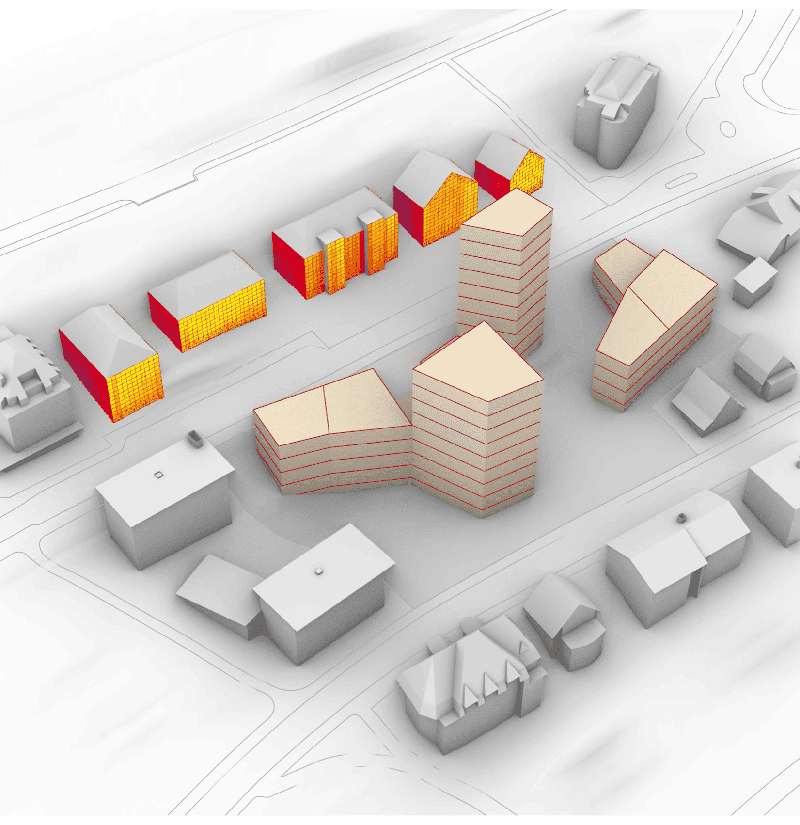
AIXD | Generative AI toolbox for architects and engineers
AIXD | Generative AI toolbox for architects and engineers
Contact us
Let’s talk Data Science
Do you need our services or expertise?
Contact us for your next Data Science project!