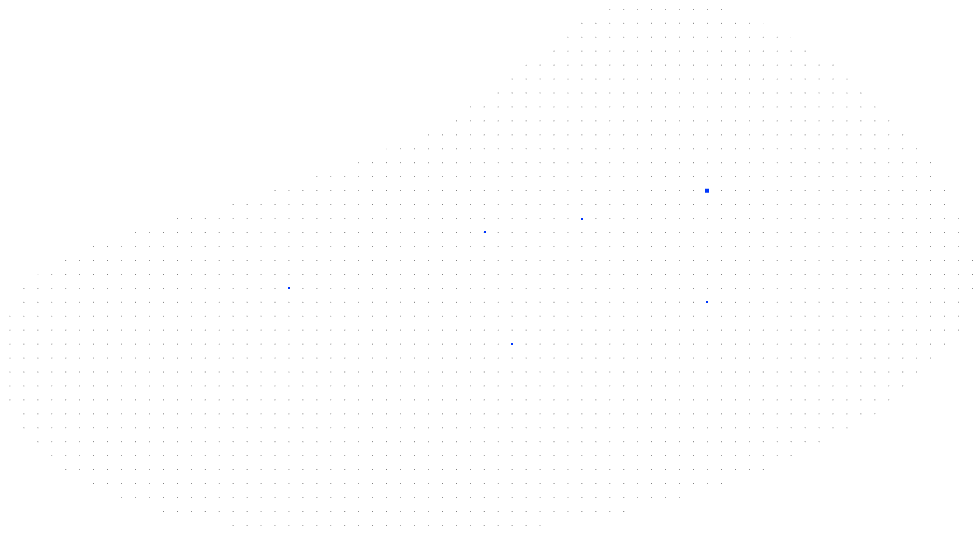
CHIP
MaCHIne-Learning-assisted Ptychographic nanotomography

Abstract
The high penetration and small wavelength of X-ray photons provides unsurpassed capabilities to probe matter down to the atomic scale. Prominent examples are given in the wide-spread impact of X-ray tomography, X-ray crystallography, and X-ray spectroscopy. Nanoscale, non-destructive imaging with X-rays can probe representative volumes and has numerous applications in energy research, materials science, biology, and medicine.
However, current measurement strategies largely follow the Nyquist-Shannon theorem (1949), which requires dense sampling in all dimensions and therewith ensures that no signal is missed in the measurement. For many scientific cases, there are patterns, anisotropy, heterogeneity, and sparsity that can be leveraged to optimize sampling for 3D imaging. Reducing and optimizing the number of measurements for X-ray nanoimaging is crucial because access is scarce at large-scale facilities, but more fundamentally, the ultimate limit for X-ray tomography resolution is given by the tolerance of the sample to radiation-induced damage. Viewed like this the total dose that the sample can tolerate can be seen as a limited budget, which, if optimized, can lead to resolution and quality that would be otherwise inaccessible.
The project has two main goals. The first is to develop, test, and implement data-driven measurement techniques that optimize sampling during the experiment. From a quick low-resolution measurement an optimal high-resolution sampling strategy is determined and fed back to the experiment. The tools developed here are of general applicability to samples with anisotropic information distribution. The second goal is the leveraging of prior information about the sample in the measurement and reconstruction. For this we will focus on imaging of integrated circuits (IC) with (<20 nm) FinFET technology. For such samples we have detailed global distribution system (GDS) layout, which can be used to further reduce the number of measurements needed.
People
Collaborators
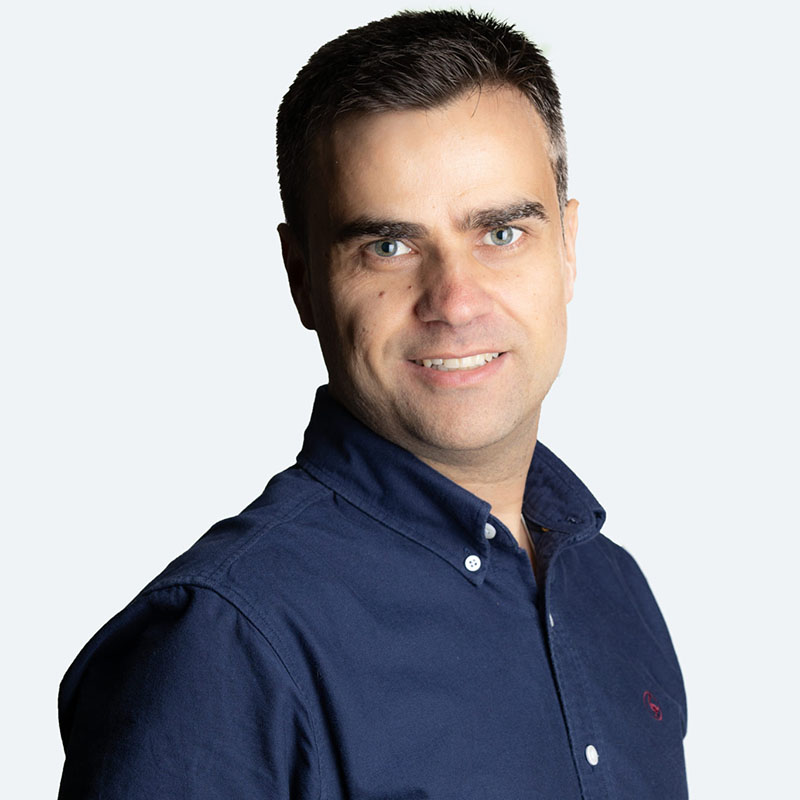
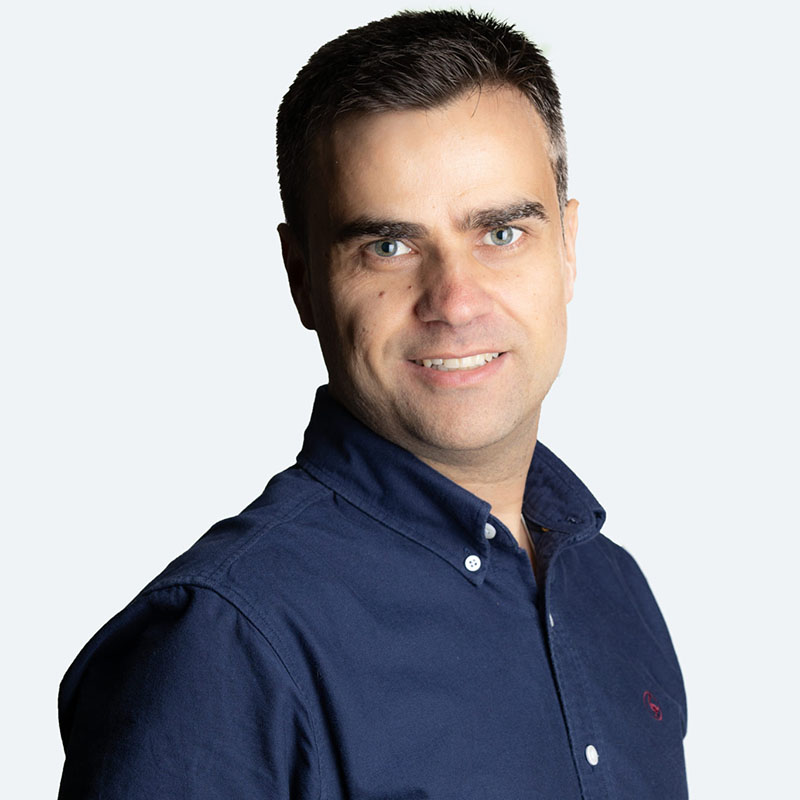
Benjamín Béjar received a PhD in Electrical Engineering from Universidad Politécnica de Madrid in 2012. He served as a postdoctoral fellow at École Polytechnique Fédérale de Lausanne until 2017, and then he moved to Johns Hopkins University where he held a Research Faculty position until Dec. 2019. His research interests lie at the intersection of signal processing and machine learning methods, and he has worked on topics such as sparse signal recovery, time-series analysis, and computer vision methods with special emphasis on biomedical applications. Since 2021, Benjamin leads the SDSC office at the Paul Scherrer Institute in Villigen.
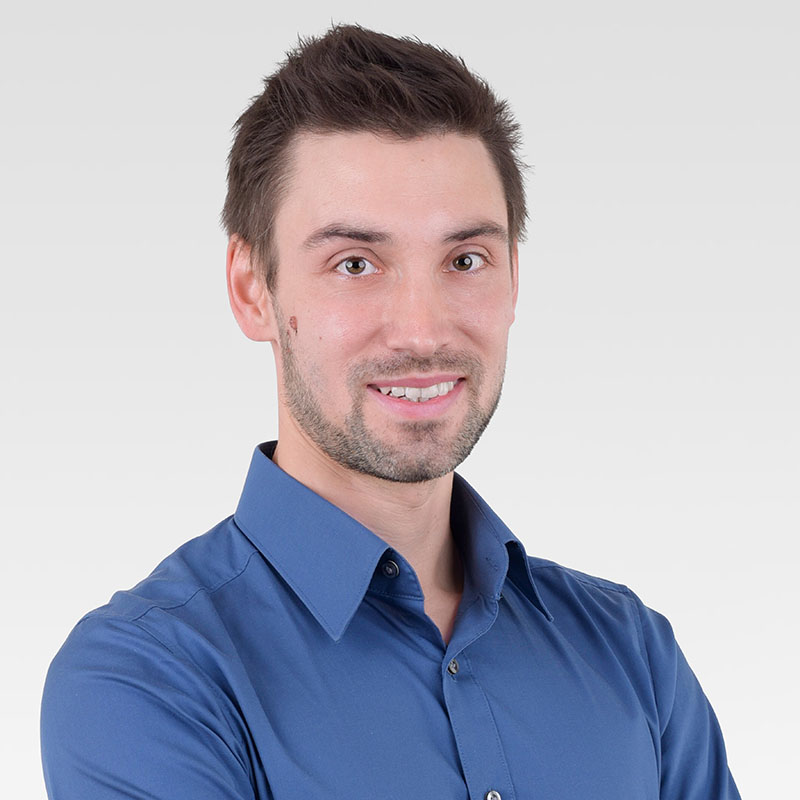
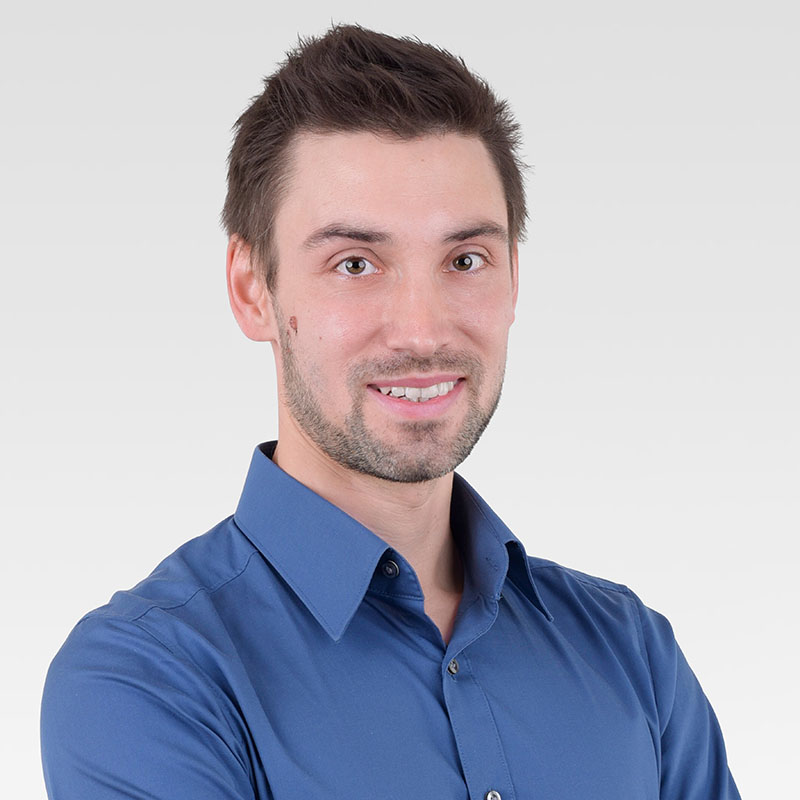
Johannes’ research focuses on developing practical andprincipled algorithms for sequential decision-making. His expertise spans awide range of topics from reinforcement learning theory and control, Bayesian optimization, safety and robustness to modern deep learning. He worked on challenging application domains, including deploying state-of-the-art data-driven optimization algorithms on two particle accelerators at the Paul Scherrer Institute. Before joining the SDSC in August 2023, Johannes was a postdoctoral researcher at the University of Alberta and completed an internship at Google DeepMind. Johannes earned his PhD in Computer Science in 2016 at ETH Zurich with Prof. Andreas Krause, and he holds a Master in Mathematics from ETH Zurich.
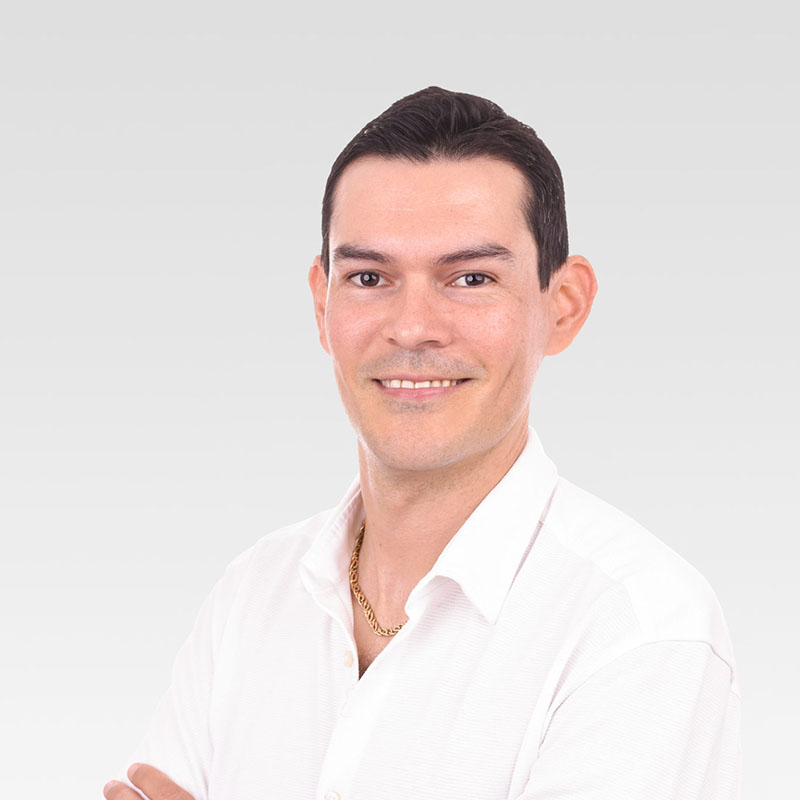
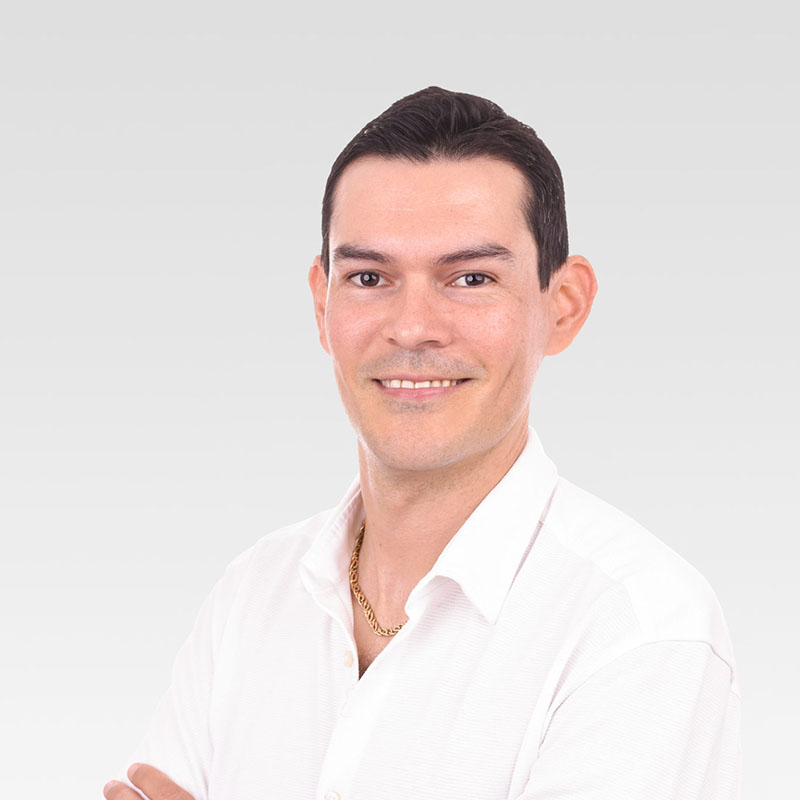
Luis Barba Flores joined the SDSC in 2022 as Senior Data Scientist. He received a joined PhD in Computers Science in 2016 from the Université Libre de Bruxelles and Carleton University. He served as a postdoctoral researcher at ETH Zurich from 2016 to 2019, and then moved to EPFL Lausanne to work in the Machine Learning and Optimization Group until 2022. His research interests include distributed optimization algorithms, first-order optimization methods and their applications to Deep Learning models.
PI | Partners:
PSI, Computational X-ray Imaging Group and EPFL, Institute of Physics, Computational X-ray Imaging Laboratory:
- Prof. Dr. Manuel Guizar-Sicairos
- Dr. Mirko Holler
- Dr. Tomas Aidukas
University of Southern California, Viterbi School of Engineering:
- Prof. Dr. Tony Levi
- M.Sc. Walter Unglaub
description
Motivation
Computed tomography aims to create a 3D reconstruction from 2D projection images that are obtained from multiple X-ray scans of the object of interest (Figure 1). The X-ray scans are typically performed according to a fixed (uniform) design with limited adaptation to the scanned object. Adaptive experimental design and learning methods are a promising approach to improve the data efficiency and limiting the total amount of X-rays required for the reconstruction. In particular, learning methods can be trained on prior data, thereby leveraging structure of the data distribution in a more efficient way than traditional reconstruction methods.
Proposed Approach / Solution
The SDSC is leading the development of active learning and adaptive experimental design algorithms for X-ray tomography and ptychography as well as developing novel reconstruction algorithms using computer vision and generative modelling. The goal is to scale the approach to high-resolution 3D imaging of integrated circuits (Figure 2) and deploy it on PSI’s state-of-the-art X-ray imaging facilities.
Impact
Increasing data efficiency of computed tomography saves valuable measurement time and limits the X-ray exposure to the scanned object. Faster reconstruction methods facilitate online evaluation of the experiment, thereby providing valuable feedback to the practitioners. Beyond the scope of the project, we expect the techniques to be relevant for a wider range of scientific questions, including medical applications.


Presentation
Gallery
Annexe
Additional resources
Bibliography
- Guizar-Sicairos, Manuel, and Pierre Thibault. "Ptychography: A solution to the phase problem." Physics Today 74.9 (2021): 42-48. https://doi.org/10.1063/pt.3.4835
- Dierolf, Martin, Andreas Menzel, Pierre Thibault, Philipp Schneider, Cameron M. Kewish, Roger Wepf, Oliver Bunk, and Franz Pfeiffer. "Ptychographic X-ray computed tomography at the nanoscale." Nature 467, no. 7314 (2010): 436-439. https://doi.org/10.1038/nature09419
- Barbano, R., Leuschner, J., Antorán, J., Jin, B., & Hernández-Lobato, J. M. (2022). “Bayesian experimental design for computed tomography with the linearised deep image prior”. arXiv preprint arXiv:2207.05714. https://doi.org/10.48550/arXiv.2207.05714
- Holler, M., Odstrcil, M., Guizar-Sicairos, M., Lebugle, M., Müller, E., Finizio, S., Tinti, G., David, C., Zusman, J., Unglaub, W. and Bunk, O., 2019. Three-dimensional imaging of integrated circuits with macro-to nanoscale zoom. Nature Electronics, 2(10), pp.464-470. https://doi.org/10.1038/s41928-019-0309-z
- Donnelly, Claire, Manuel Guizar-Sicairos, Valerio Scagnoli, Sebastian Gliga, Mirko Holler, Jörg Raabe, and Laura J. Heyderman. "Three-dimensional magnetization structures revealed with X-ray vector nanotomography." Nature 547, no. 7663 (2017): 328-331. https://doi.org/10.1038/nature23006
Publications
Related Pages
More projects
STIMO
News
Latest news


Smartair | An active learning algorithm for real-time acquisition and regression of flow field data
Smartair | An active learning algorithm for real-time acquisition and regression of flow field data
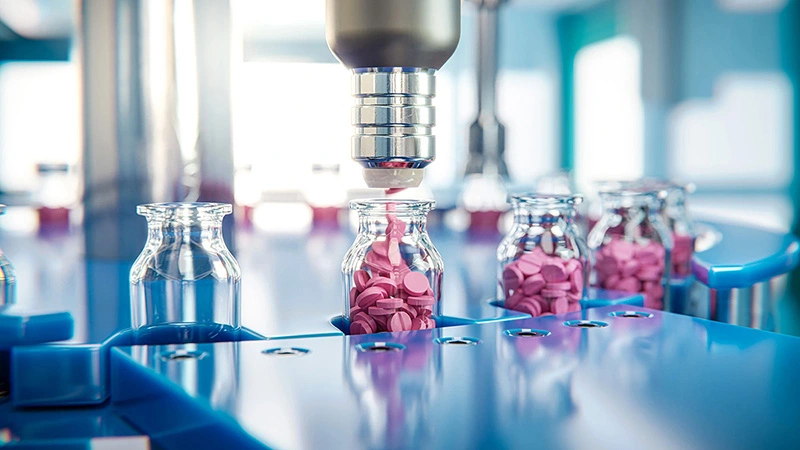
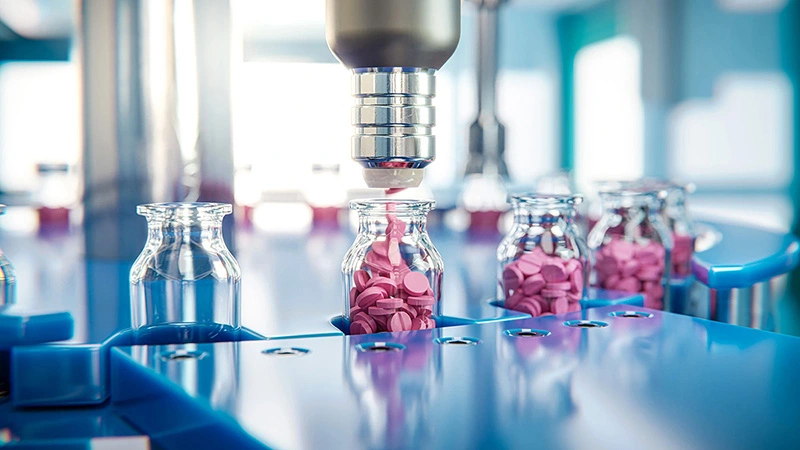
The Promise of AI in Pharmaceutical Manufacturing
The Promise of AI in Pharmaceutical Manufacturing


Efficient and scalable graph generation through iterative local expansion
Efficient and scalable graph generation through iterative local expansion
Contact us
Let’s talk Data Science
Do you need our services or expertise?
Contact us for your next Data Science project!