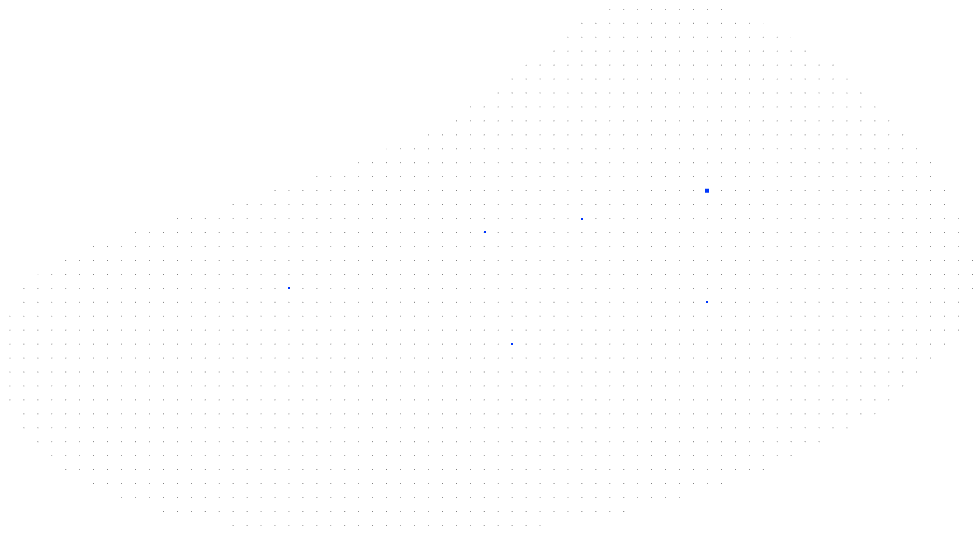
CHEMSPEC
Cost-effective chemical speciation monitoring of particulate matter air pollution
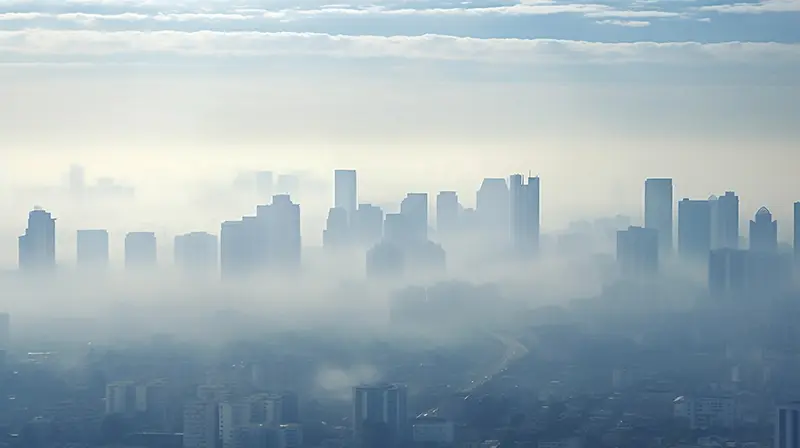
Abstract
Ambient particulate matter is one of the greatest environmental health risks to society, but cost-effective methods for chemical characterization and source apportionment necessary to inform robust regulatory strategies are lacking. Our team has demonstrated the possibility to use infrared spectroscopy as an inexpensive optical probe to obtain chemically informative spectra of particulate matter, and this analytical technique is increasingly being used in air quality monitoring networks and research campaigns worldwide. To obtain reliable predictions at such a prolific scale, advanced data-driven methods and an open platform where the global community can access them are necessary to fully take advantage of the rich but complex infrared spectra acquired from these samples.
CHEMSPEC brings together an unprecedented data set from multiple monitoring networks and laboratory-generated particles to learn the relations between chemical composition and spectroscopic signatures that can be used in quantitative modeling of chemical species concentrations and source profiles associated with fossil fuel combustion, biomass burning, dust, and other major contributors to particulate matter. The results of this project will advance the characterization of particulate
matter not only in existing monitoring networks, but in critical areas of the world where the particulate matter composition is virtually unknown.
People
Collaborators
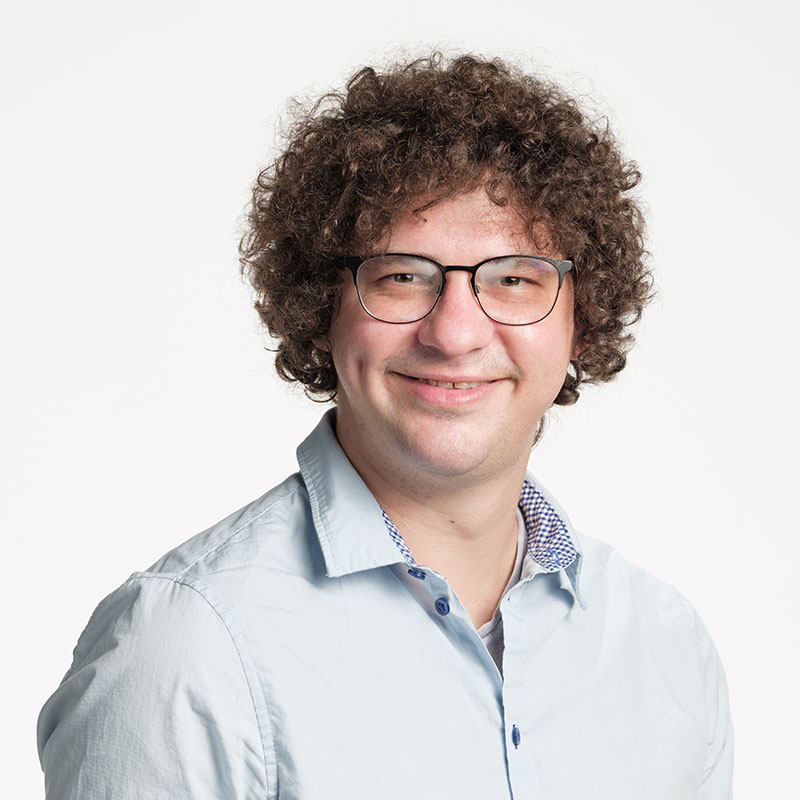
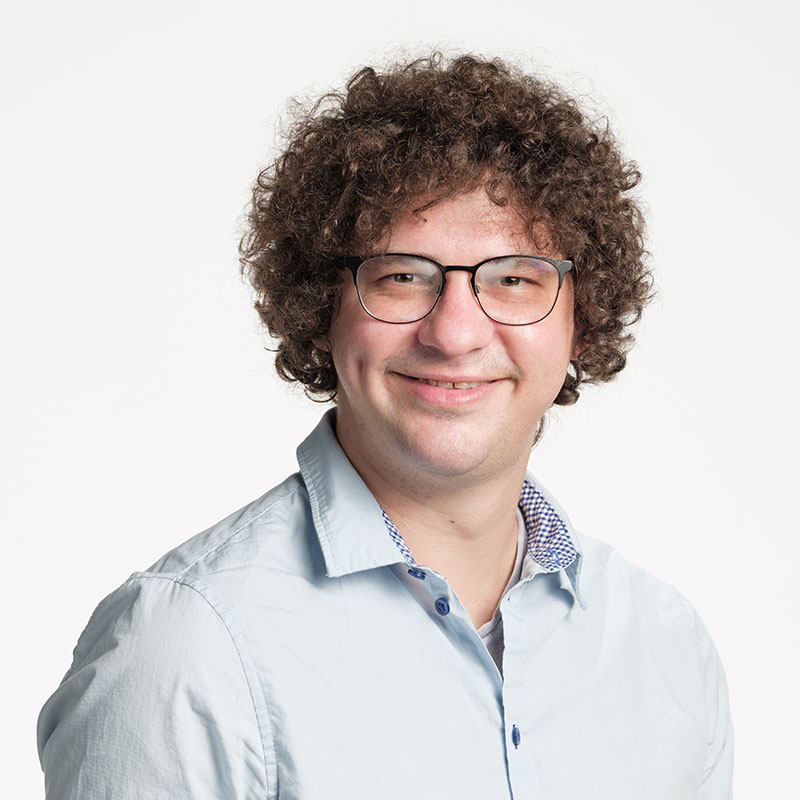
Francois received his PhD in December 2018 from Stellenbosch University (SU) in South Africa where he focused on improving inference algorithms for probabilistic graphical models. Before joining the SDSC, Francois worked as a senior lecturer at the Department of Statistics and Actuarial Science at SU. His research interests include probabilistic graphical models, time series forecasting, causal inference and discovery, extreme value theory, and machine learning in general.
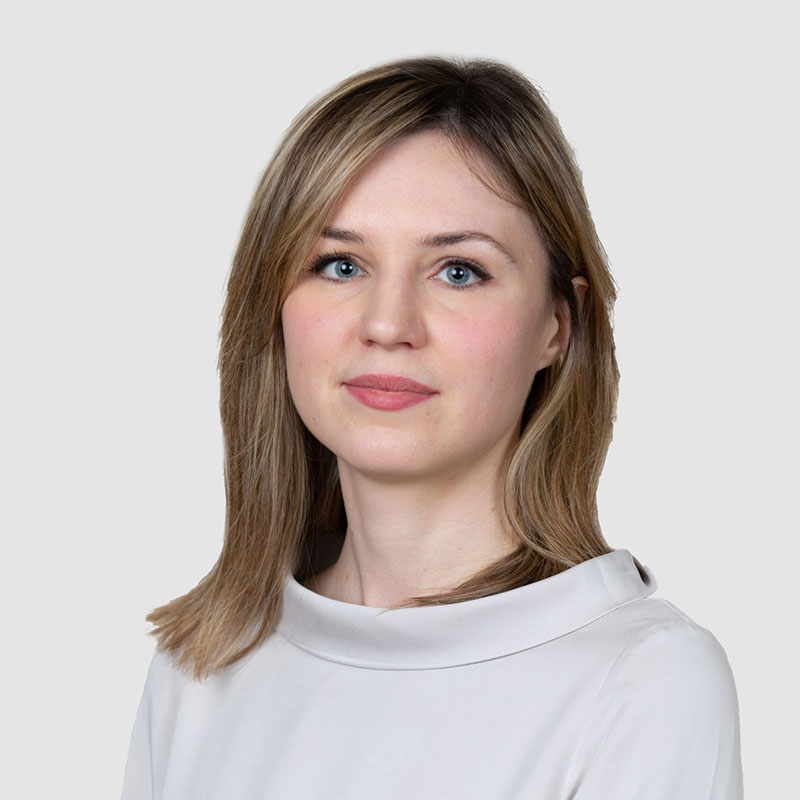
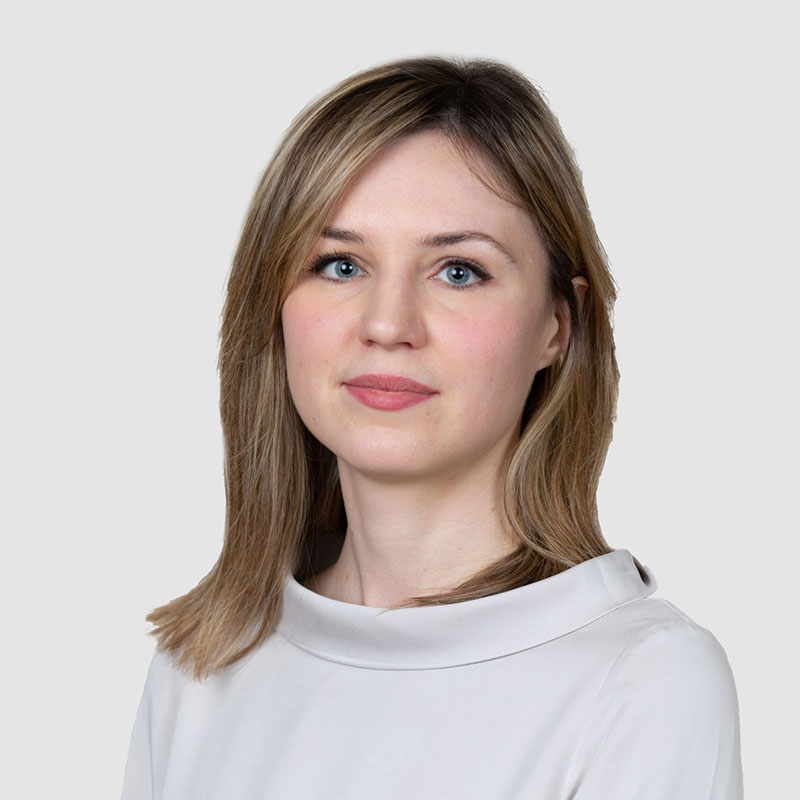
Ekaterina received her PhD in Computer Science from Moscow Institute for Physics and Technology, Russia. Afterwards, she worked as a researcher at the Institute for Information Transmission Problems in Moscow and later as a postdoctoral researcher in the Stochastic Group at the Faculty of Mathematics at University Duisburg-Essen, Germany. She has experience with various applied projects on signal processing, predictive modelling, macroeconomic modelling and forecasting, and social network analysis. She joined the SDSC in November 2019. Her interests include machine learning, non-parametric statistical estimation, structural adaptive inference, and Bayesian modelling.
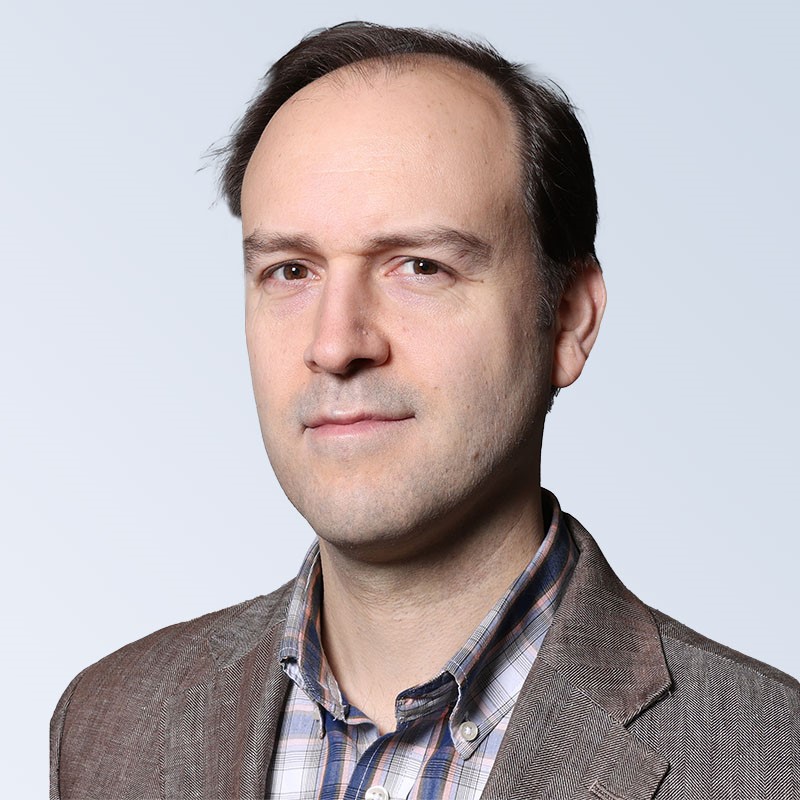
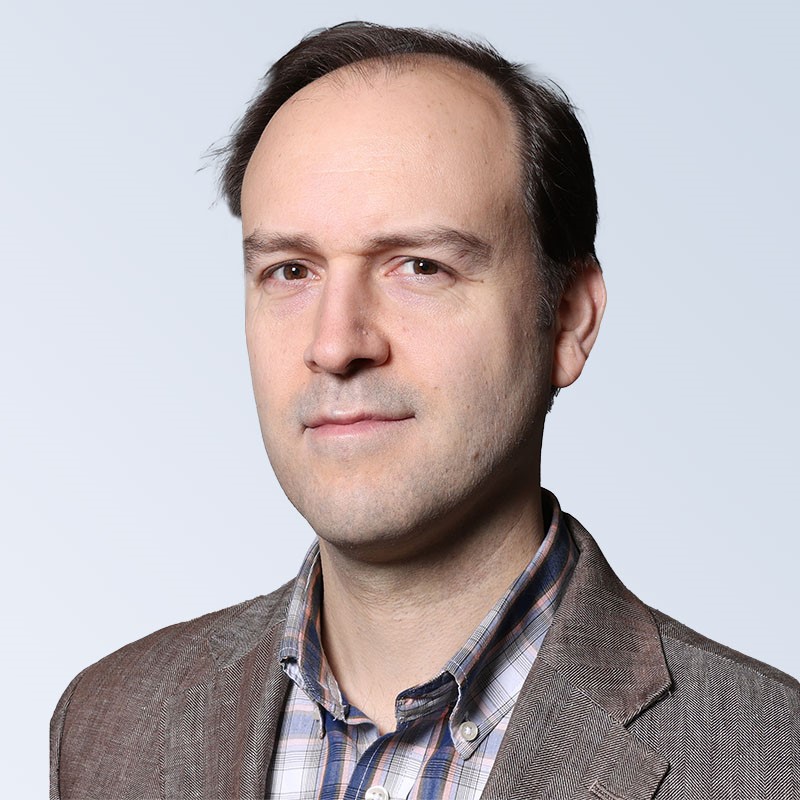
Guillaume Obozinski graduated with a PhD in Statistics from UC Berkeley in 2009. He did his postdoc and held until 2012 a researcher position in the Willow and Sierra teams at INRIA and Ecole Normale Supérieure in Paris. He was then Research Faculty at Ecole des Ponts ParisTech until 2018. Guillaume has broad interests in statistics and machine learning and worked over time on sparse modeling, optimization for large scale learning, graphical models, relational learning and semantic embeddings, with applications in various domains from computational biology to computer vision.
description
Motivation
We aim to develop infrared spectroscopy as a method to monitor ambient particulate matter in the air as an inexpensive alternative to current approaches. In this way we aim to improve the monitoring of air quality in established networks, as well as in other critical areas of the world, to aid with the formulation of emissions control policy and improve healthcare in general.
Proposed Approach
We propose removing background interference from field spectra through a prior obtained by fitting a distribution to blank spectra, i.e. spectra free from any ambient particulate matter. Once the background interference has been removed, we aim to learn profiles of chemical species using, for example, non-negative matrix factorization approaches. These profiles can then be used to obtain predictions of the concentrations of ambient particulate matter in the field, generalizable to a variety of locations.
Impact
The anticipated impact of this project is the expansion in capabilities of monitoring networks worldwide – especially in areas where particle composition is virtually unknown – to cost - effectively provide chemical composition information required to identify the key sources and constituents contributing to PM2.5 at every location critical for shaping necessary emissions control policies, evaluating air quality action plans, supporting air quality modeling, and remote sensing efforts.
Note. Here PM2.5 is the mass concentration of ambient particulate matter less than 2.5 micrometers in diameter.
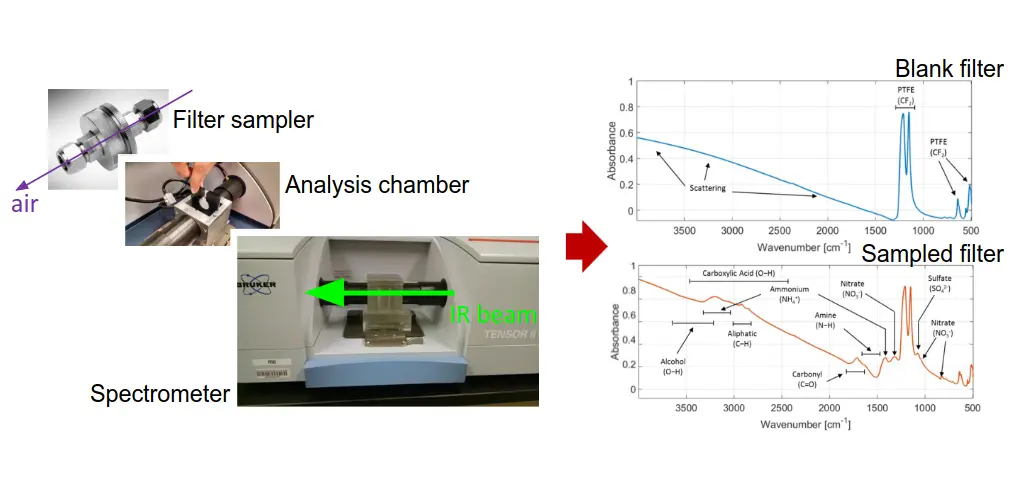
Presentation
Gallery
Annexe
Additional resources
Bibliography
- Solomon, P. A. et al. U.S. National PM2.5 Chemical Speciation Monitoring Networks—CSN and
IMPROVE: Description of networks. Journal of the Air & Waste Management Association 64,
1410–1438. eprint: https://doi.org/10.1080/10962247.2014.956904. https://doi.org/10.
1080/10962247.2014.956904 (2014). - Reggente, M., Dillner, A. M. & Takahama, S. Analysis of functional groups in atmospheric aerosols
by infrared spectroscopy: systematic intercomparison of calibration methods for US measurement
network samples. Atmospheric Measurement Techniques 12, 2287–2312. https : / / www . atmos -
meas-tech.net/12/2287/2019/ (2019). - Bürki, C. et al. Analysis of functional groups in atmospheric aerosols by infrared spectroscopy:
method development for probabilistic modeling of organic carbon and organic matter concentrations.
Atmospheric Measurement Techniques 13, 1517–1538. AMT – Volume 13, 2020 1517/2020/ (2020). - Boris, A. J. et al. Quantifying organic matter and functional groups in particulate matter filter
samples from the southeastern United States – Part 2: Spatiotemporal trends. English. Atmospheric
Measurement Techniques 14. Publisher: Copernicus GmbH, 4355–4374. issn: 1867-1381. https :
//amt.copernicus.org/articles/14/4355/2021/ (2021) (June 2021).
Publications
Related Pages
More projects
ML-L3DNDT
BioDetect
News
Latest news


Smartair | An active learning algorithm for real-time acquisition and regression of flow field data
Smartair | An active learning algorithm for real-time acquisition and regression of flow field data
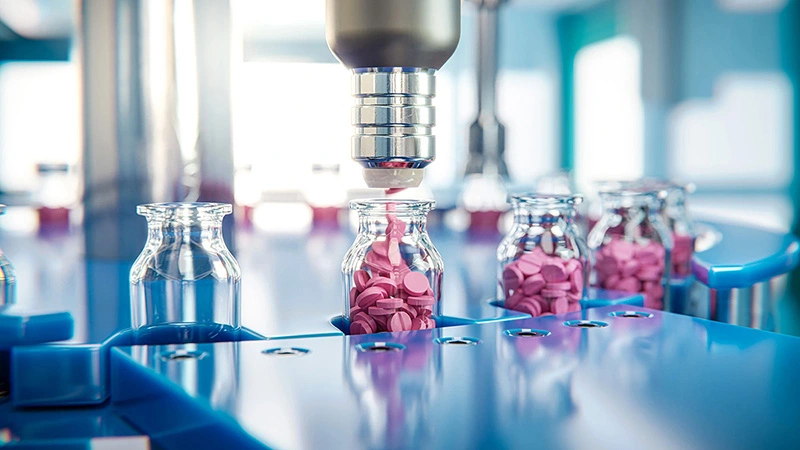
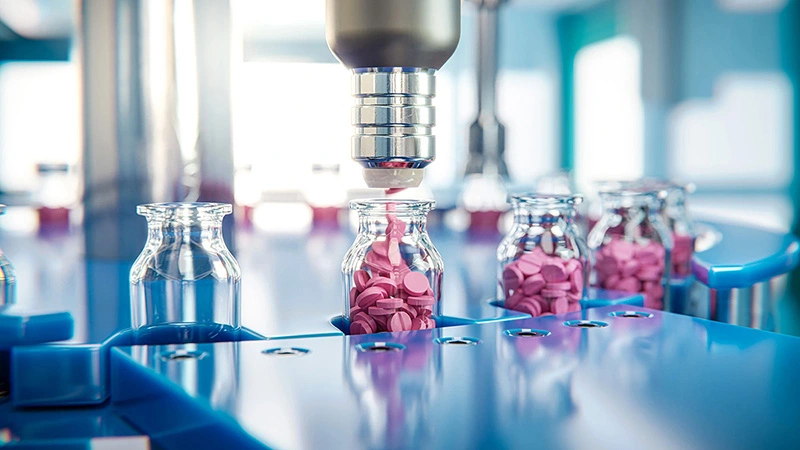
The Promise of AI in Pharmaceutical Manufacturing
The Promise of AI in Pharmaceutical Manufacturing


Efficient and scalable graph generation through iterative local expansion
Efficient and scalable graph generation through iterative local expansion
Contact us
Let’s talk Data Science
Do you need our services or expertise?
Contact us for your next Data Science project!