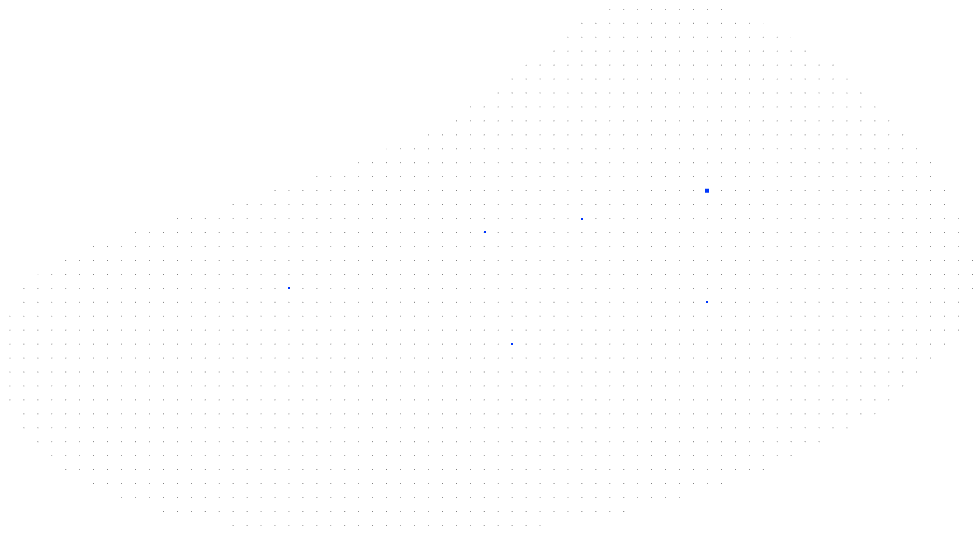
BISTOM
Bayesian Parameter Inference for Stochastic Models
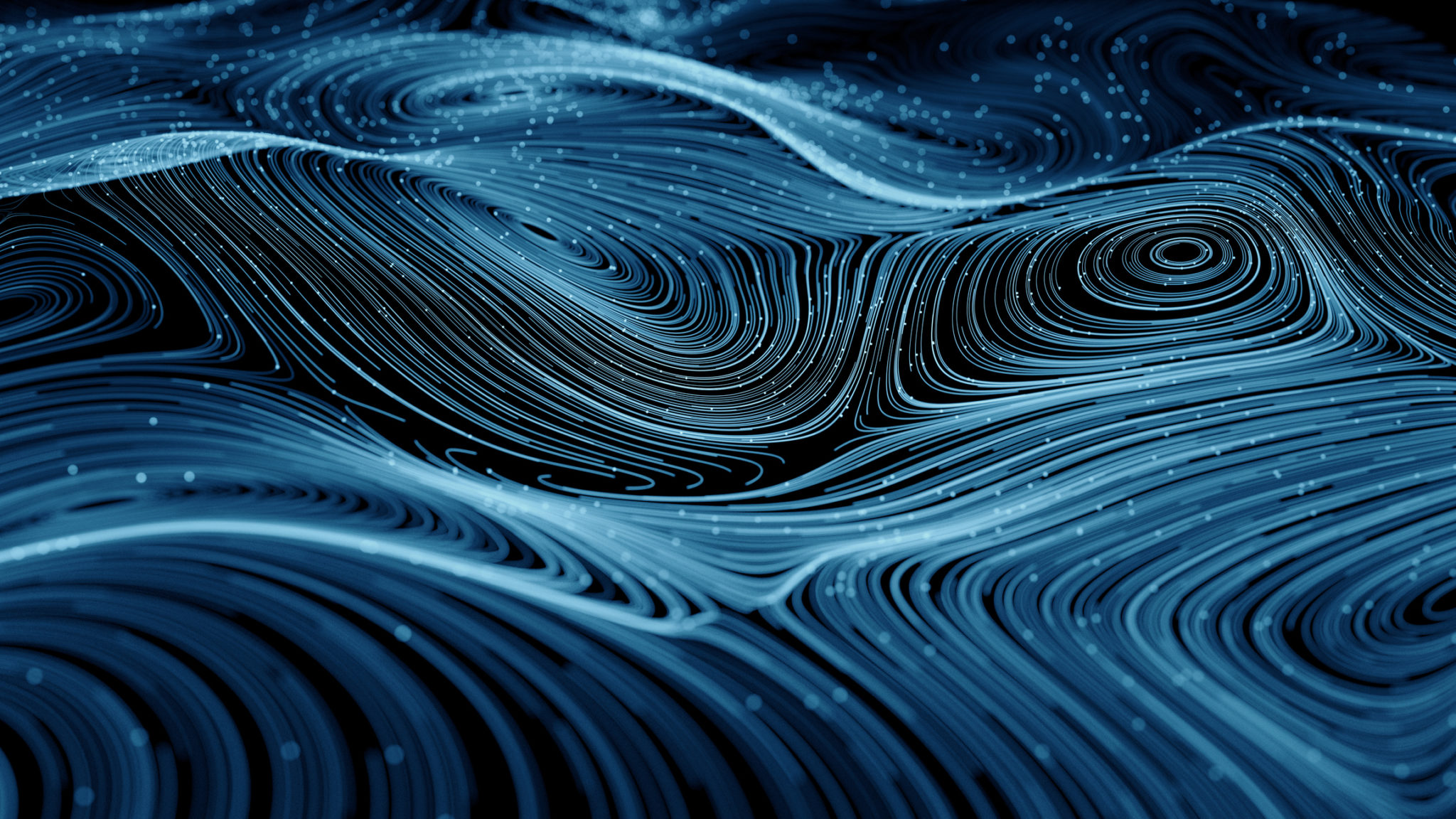
Abstract
For a multitude of different field sciences, determining the underlying mechanistic models is important in order to further our understanding. An accurate estimation of the parameters of such mechanistic models through data can be computationally prohibitive. This project exploits neural networks in order to learn minimal and near sufficient summary statistics as latent embeddings on simulated data for multiple stochastic models. This is corroborated with sharp model parameter posteriors observed through approximate Bayesian computation experiments.
People
Collaborators
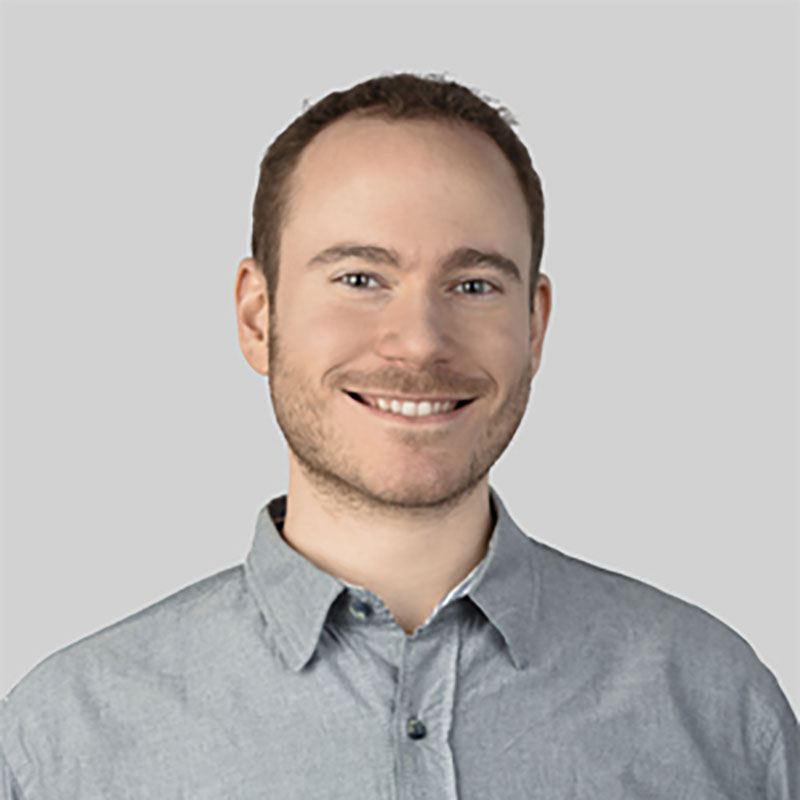
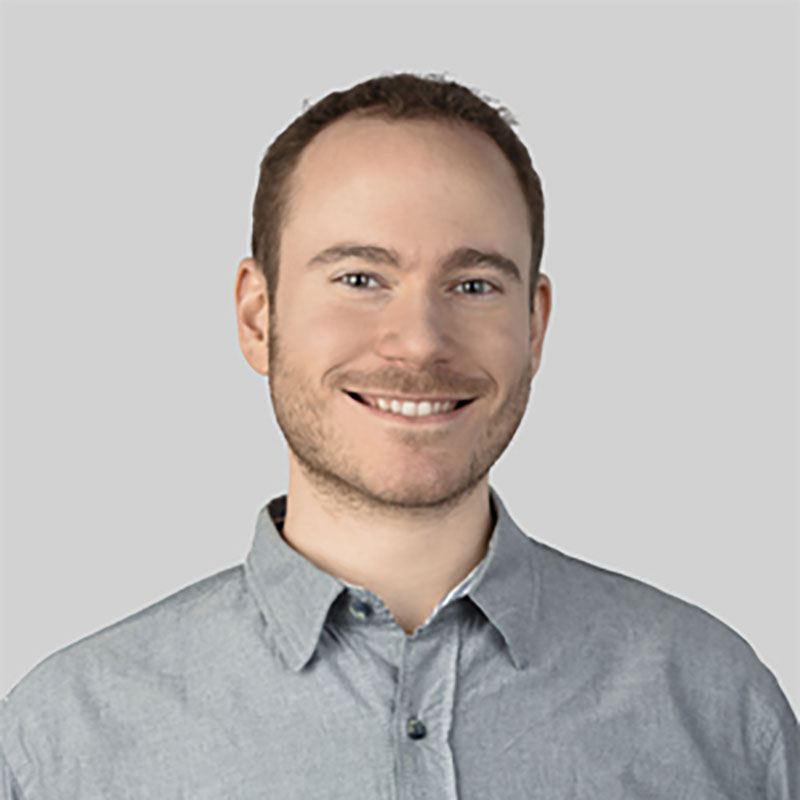
Firat completed his undergraduate studies in Electronics Engineering at Sabanci University. He later received his MSc. in Electrical and Electronics Engineering from EPFL. He conducted his doctoral studies on medical image segmentation in Computer Vision Lab at ETH Zurich. In between, he visited INRIA (Sophia Antipolis, France) and ABB Corporate Research Center (Baden, Switzerland). His research interests revolve around computer vision and machine learning, with a focus on the medical domain. He has been with SDSC since 2019.
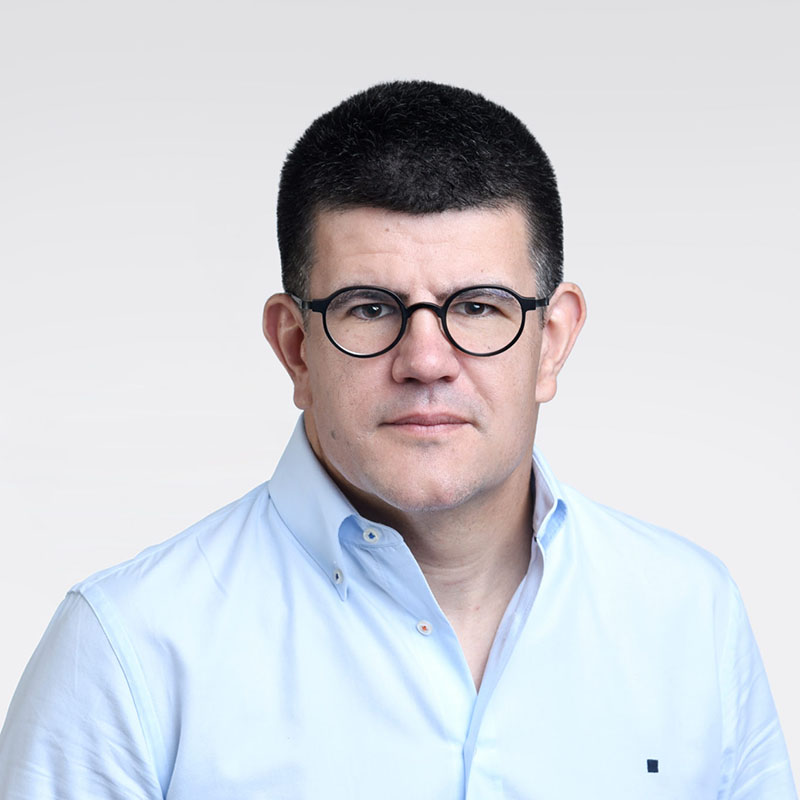
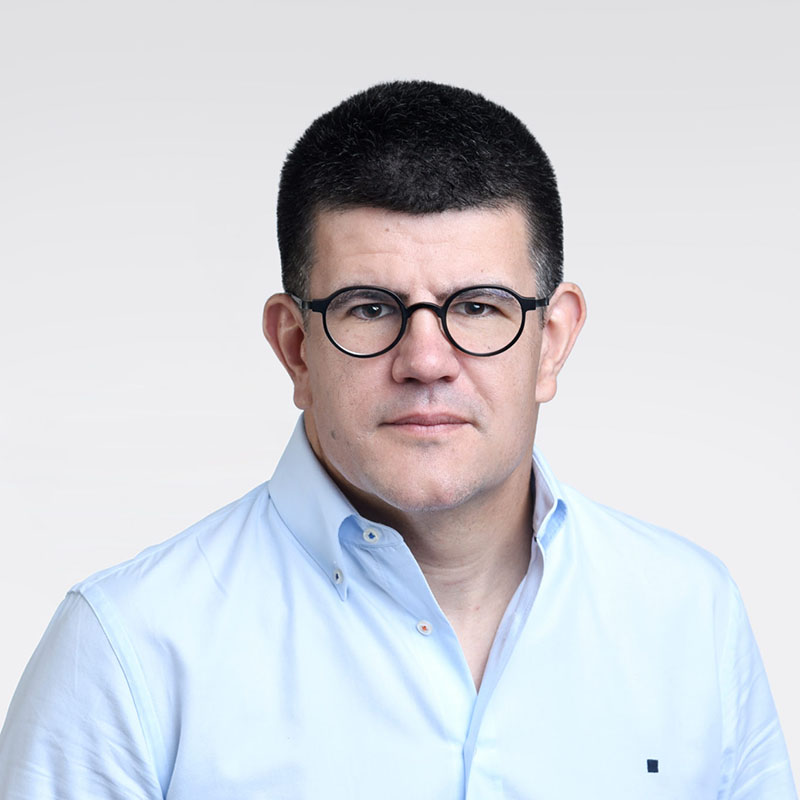
Fernando Perez-Cruz received a PhD. in Electrical Engineering from the Technical University of Madrid. He is Titular Professor in the Computer Science Department at ETH Zurich and Head of Machine Learning Research and AI at Spiden. He has been a member of the technical staff at Bell Labs and a Machine Learning Research Scientist at Amazon. Fernando has been a visiting professor at Princeton University under a Marie Curie Fellowship and an associate professor at University Carlos III in Madrid. He held positions at the Gatsby Unit (London), Max Planck Institute for Biological Cybernetics (Tuebingen), and BioWulf Technologies (New York). Fernando Perez-Cruz has served as Chief Data Scientist at the SDSC from 2018 to 2023, and Deputy Executive Director of the SDSC from 2022 to 2023
description
Goal
Reliably estimating parameters of mechanistic models from data (Bayesian inference) is computationally expensive if (i) the data is big or (ii) the model is stochastic. Stochastic models are needed for reliable predictions.
Impact
The developed methodology can be applied in many fields of science and engineering, wherever system understanding (mechanistic model) needs to be combined with data, for advancing domain knowledge and making more reliable predictions.
Solution
Advances in algorithms as well as parallel computing infrastructure allow for Bayesian inference to be applied to a large class of stochastic models and to be scaled up to big data. We developed neural network based framework which can learn minimal and near sufficient summary statistics as latent embeddings on simulated data for multiple stochastic models. Experiments with approximate Bayesian computation yield sharp model parameter posteriors. For both stochastic models, developed solution finds near sufficient summary statistics.
Presentation
Gallery
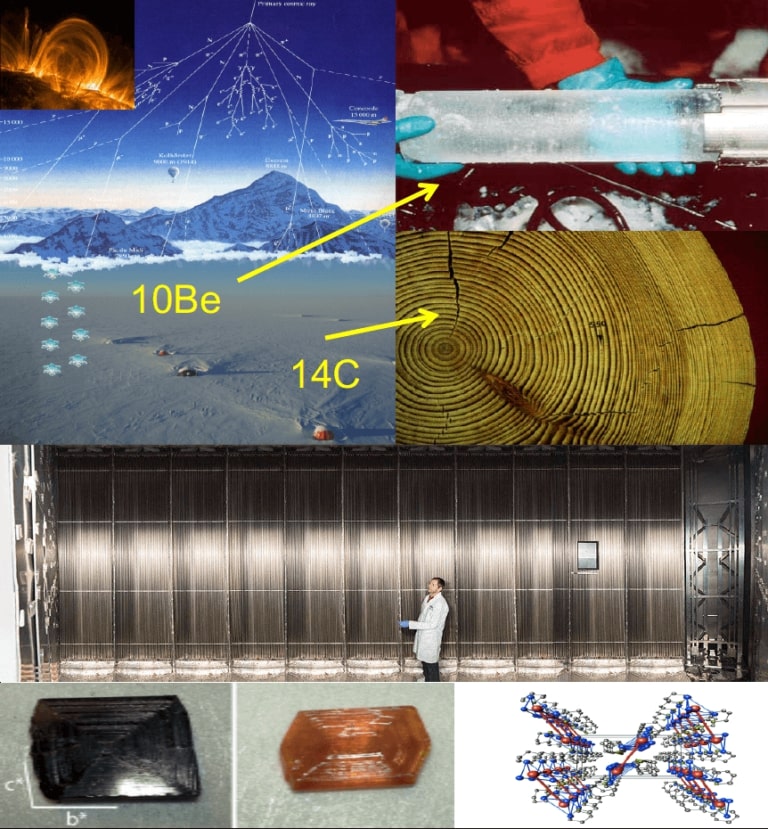
Annexe
Additional resources
Bibliography
Publications
Related Pages
More projects
MAGNIFY
News
Latest news
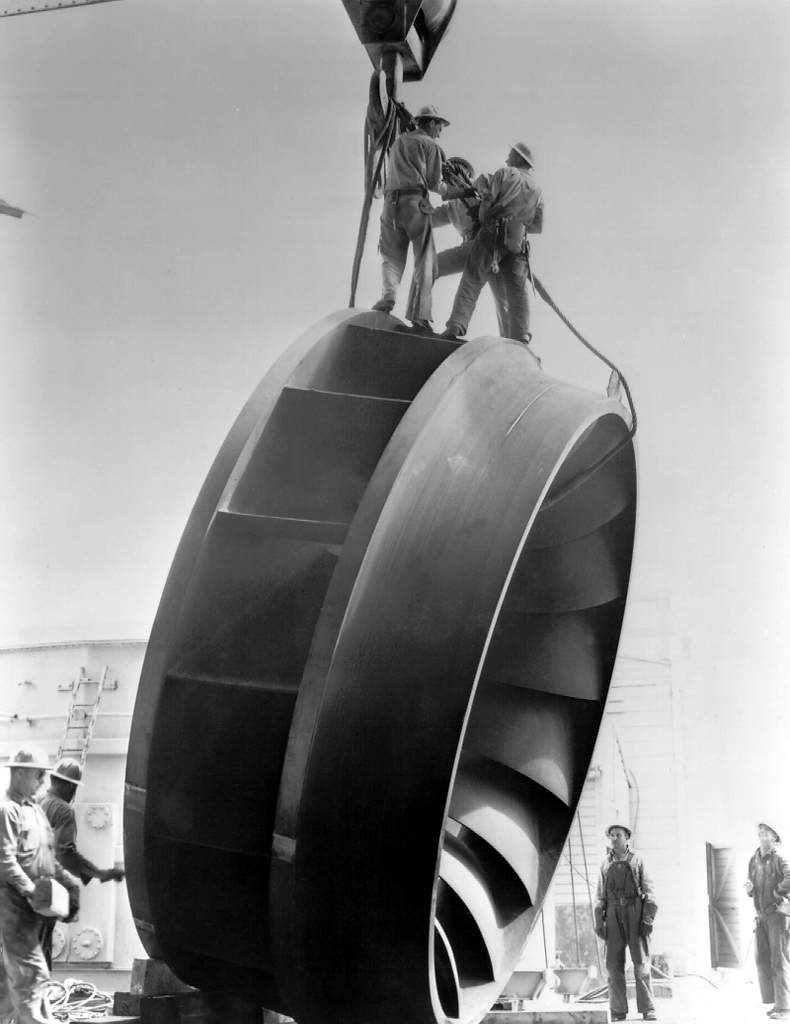
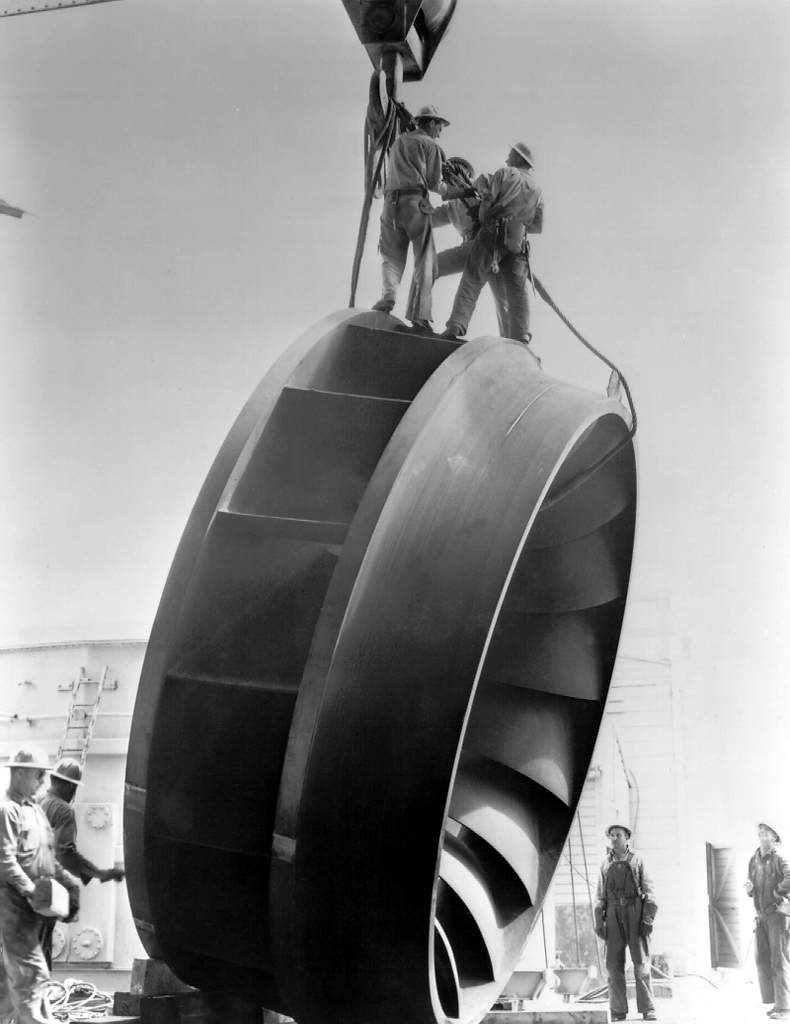
PAIRED-HYDRO | Increasing the Lifespan of Hydropower Turbines with Machine Learning
PAIRED-HYDRO | Increasing the Lifespan of Hydropower Turbines with Machine Learning


First National Calls: 50 selected projects to start in 2025
First National Calls: 50 selected projects to start in 2025
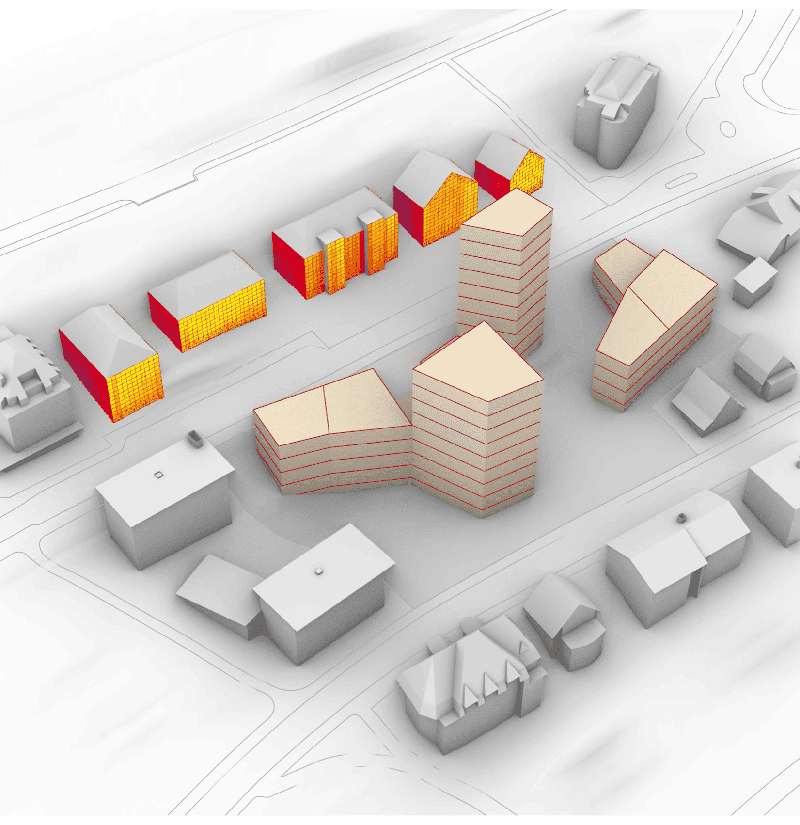
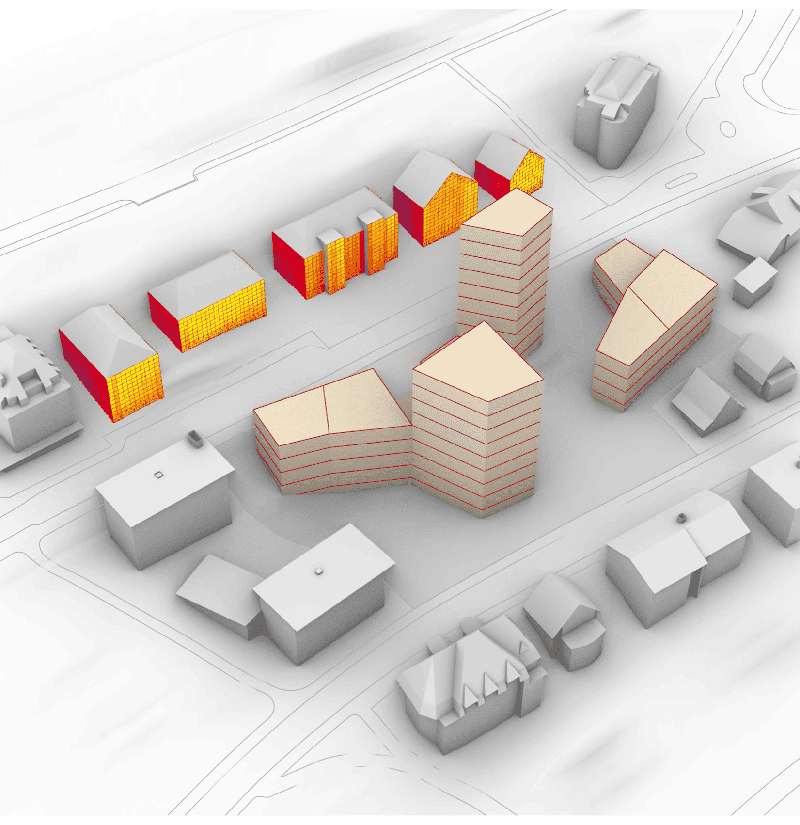
AIXD | Generative AI toolbox for architects and engineers
AIXD | Generative AI toolbox for architects and engineers
Contact us
Let’s talk Data Science
Do you need our services or expertise?
Contact us for your next Data Science project!