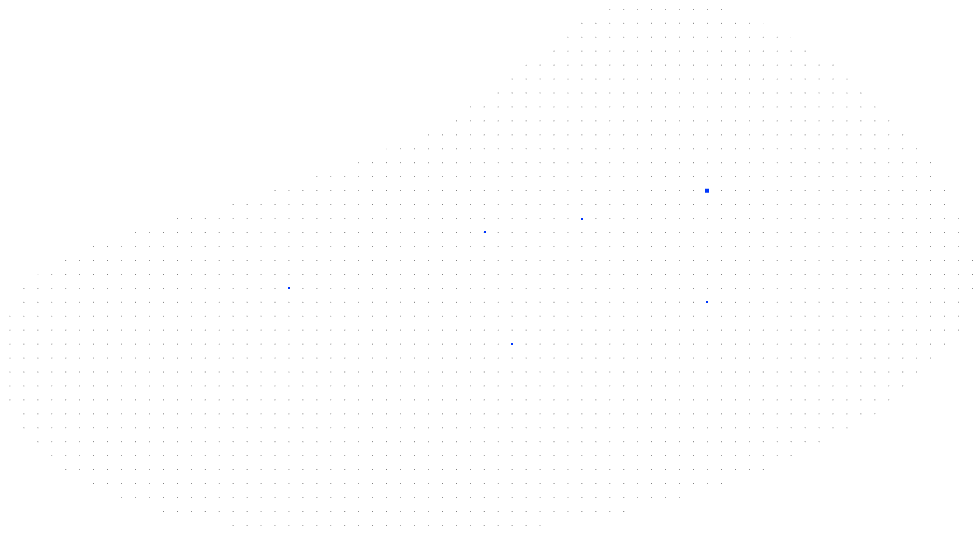
AURORA
From air pollution sources to mortality
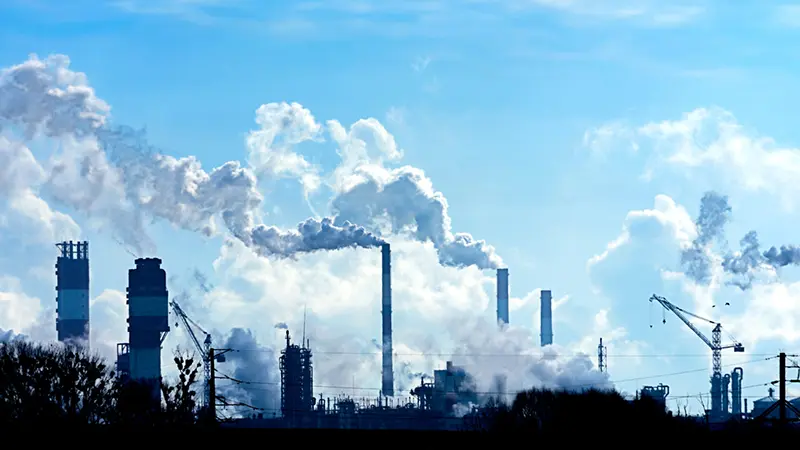
Abstract
Atmospheric aerosols (or particulate matter, PM) are liquid or solid particles suspended in the air with diameters ranging from few nanometers to few tens of micrometers. Poor air quality associated with high levels of PM is a major public health problem, and is one of the five leading causes of premature deaths worldwide, alongside with high blood pressure, smoking, diabetes and obesity. Human exposure to PM caused ~8.9 million deaths, or ~10% of total global burden of mortality in 2015, more than car accidents, HIV and malaria combined. Without any action, these numbers are expected to double by 2050. PM health effects can be both acute and chronic, and have been associated with cardiovascular diseases, respiratory symptoms, different types of cancer, diabetes, sudden infant mortality, and neurodegenerative diseases (upon penetrating the blood-brain barrier). The magnitude of the association between PM exposure and the probability of death, is based on the total PM mass, while PM’s health effects is strongly driven by its chemical composition and size, and hence its origin. PM originates from natural (e.g. volcanoes, pollen) or anthropogenic (e.g. combustion) sources, and can be primary from direct emissions (e.g. metals from vehicular wear) or secondary, formed in the atmosphere through complex oxidation mechanisms of gaseous precursors (e.g. from trees, car/industrial exhaust, residential heating) (Fig. 1). Our ability to identify the major PM sources responsible for health outcomes is a two-fold challenge that requires (1) a fundamental understanding of PM emissions and formation processes and (2) the consideration of the high diversity and spatial heterogeneity of PM emissions, especially in urban settings where most of the population resides. AURORA unifies the expertise from distinct fields of science, including analytical & atmospheric chemistry and numerical modelling, to propose an innovative modelling framework, which integrated data-science, geo-statistics and process-based simulations to achieve a unique combination of source specificity, spatial and temporal coverage and resolution required for human exposure assessments. Model outputs will be combined with invaluable records of Organic Aerosol concentrations on a European scale.
People
Collaborators
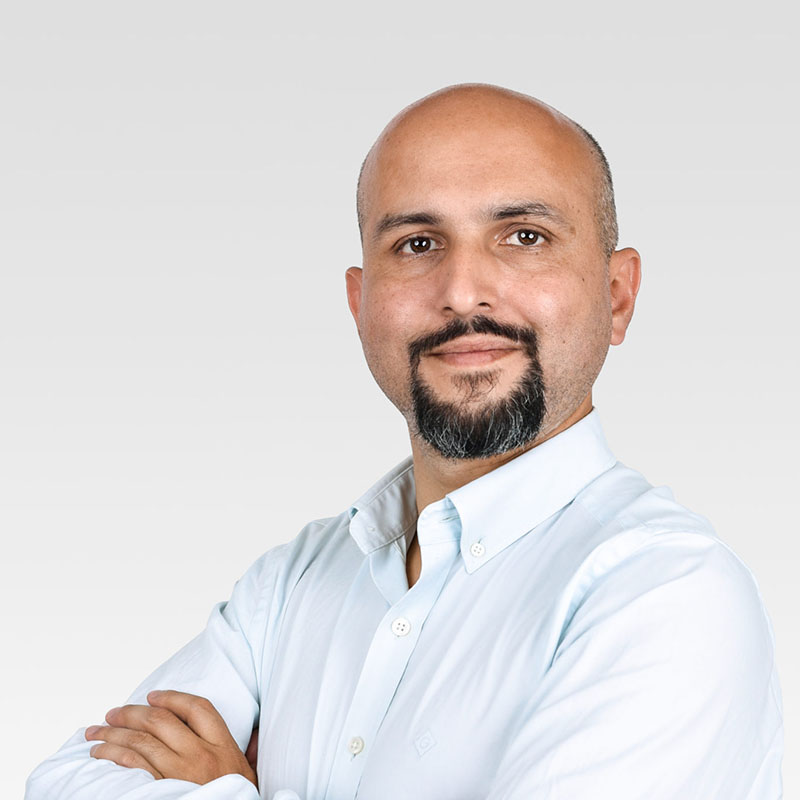
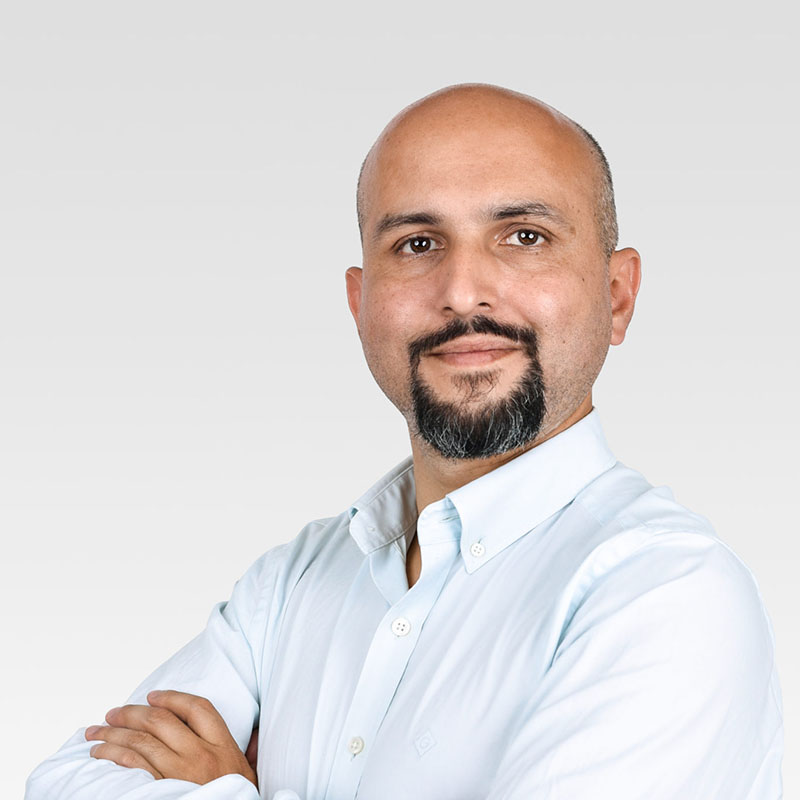
Daniel worked as a postdoctoral researcher on critical event prediction for the University Hospital in Zurich. In addition, Daniel has worked as a postdoctoral researcher in Lausanne, delivering algorithms for Bayesian inference in big panel data. Previously in Paris, he developed models for automated scientific discovery. He obtained a Ph.D. from the University of Edinburgh, funded by a Microsoft Research scholarship. His interest relates primarily to attacking applied biomedicine problems from different angles, frequentist statistics, Bayesian statistics, and Machine Learning.
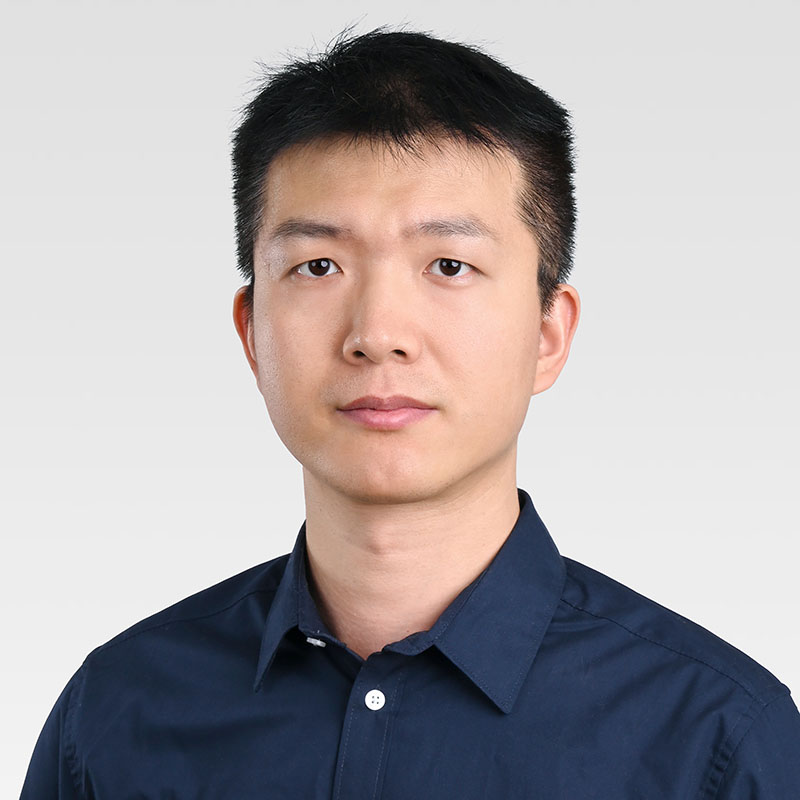
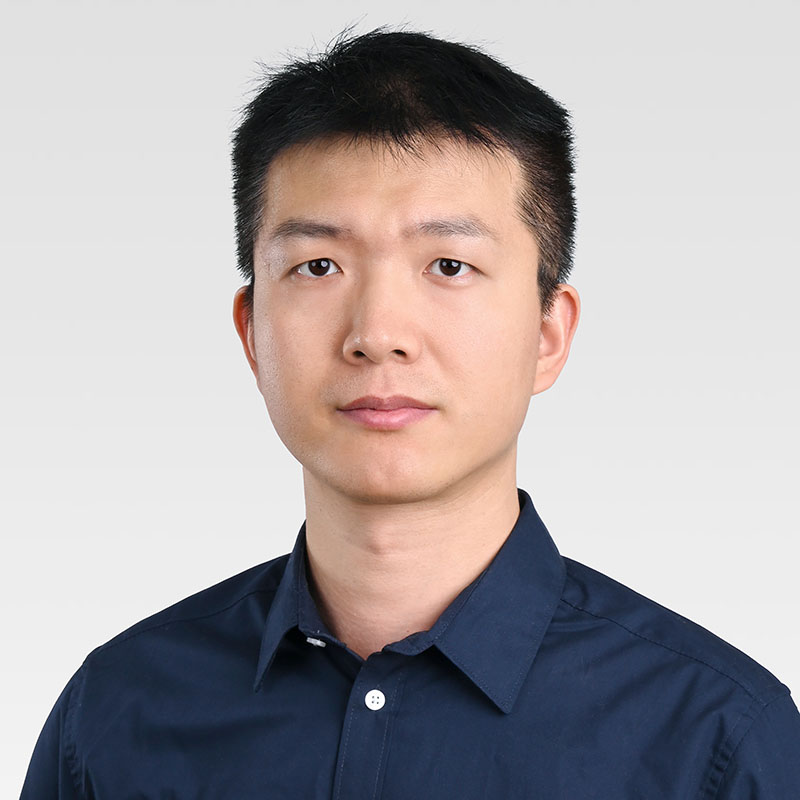
Yun Cheng is a Ph.D. graduate from D-ITET in ETH Zurich, where he was mentored by Prof. Lothar Thiele. His research primarily focuses on IoT, individual and urban services, CPS, and the combination of data-driven and model-driven methods, particularly in their application to real-world challenges. During his Ph.D., he developed various machine intelligence techniques for environmental analysis and control. This work earned him three best paper awards and the Talent Kick Award from the ETH AI Center. Beyond academia, Yun co-founded a startup called Air-Scientific, dedicated to making low-cost sensing and analysis accessible to all.
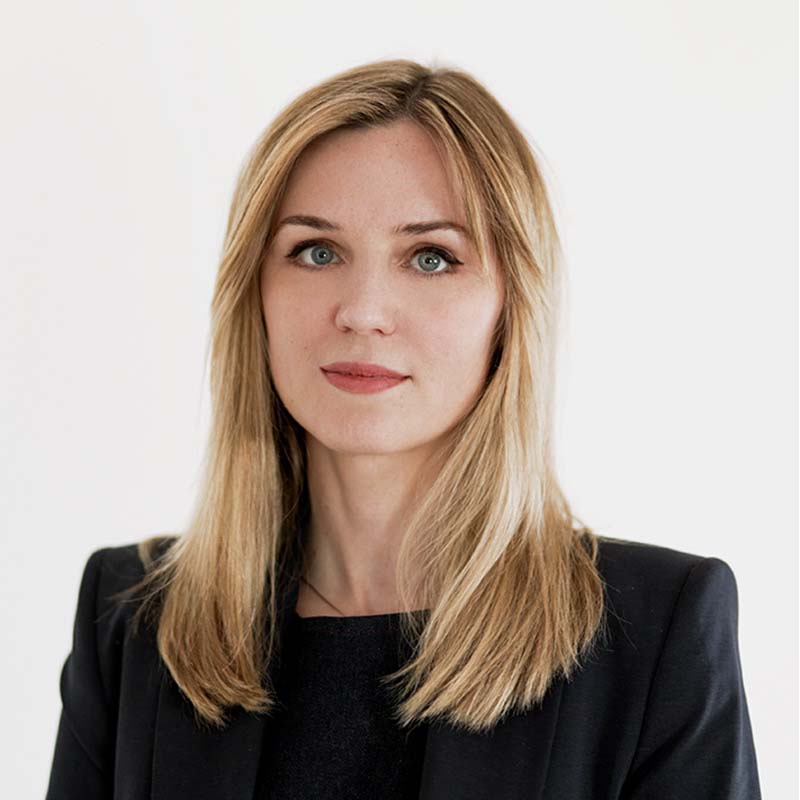
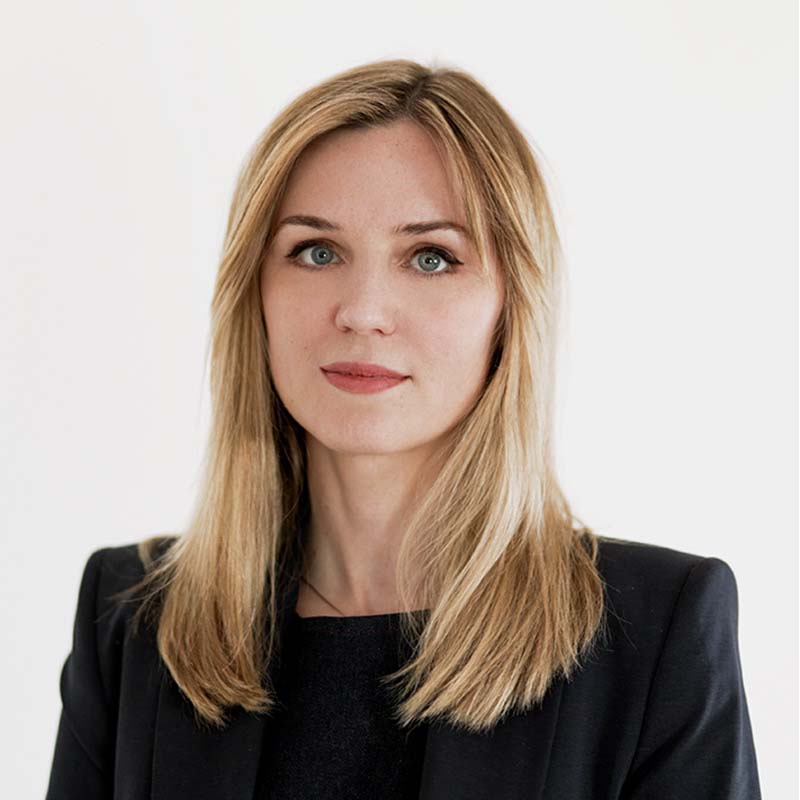
Ekaterina received her PhD in Computer Science from Moscow Institute for Physics and Technology, Russia. Afterwards, she worked as a researcher at the Institute for Information Transmission Problems in Moscow and later as a postdoctoral researcher in the Stochastic Group at the Faculty of Mathematics at University Duisburg-Essen, Germany. She has experience with various applied projects on signal processing, predictive modelling, macroeconomic modelling and forecasting, and social network analysis. She joined the SDSC in November 2019. Her interests include machine learning, non-parametric statistical estimation, structural adaptive inference, and Bayesian modelling.
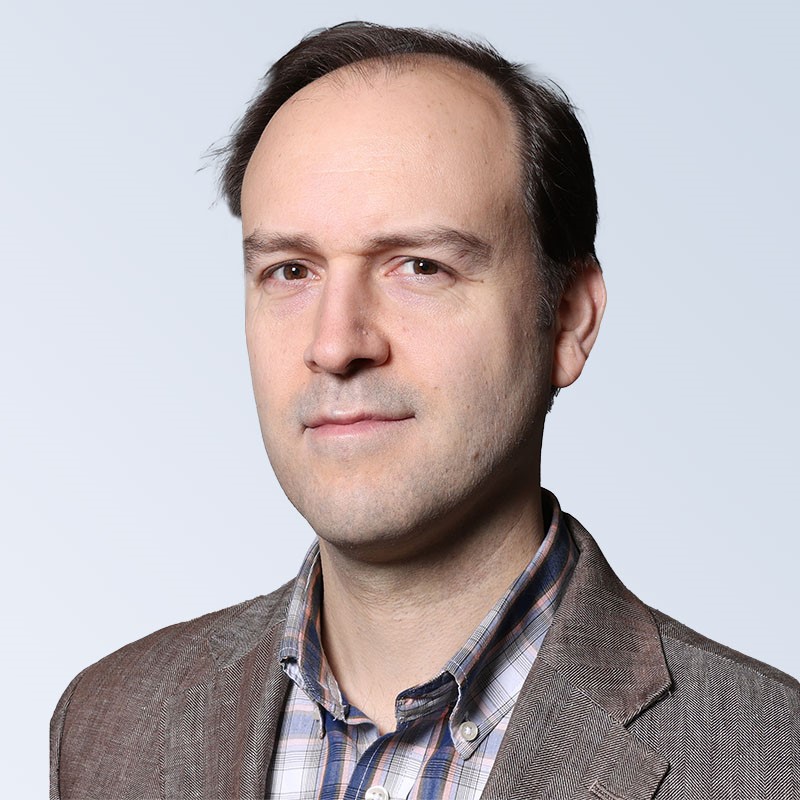
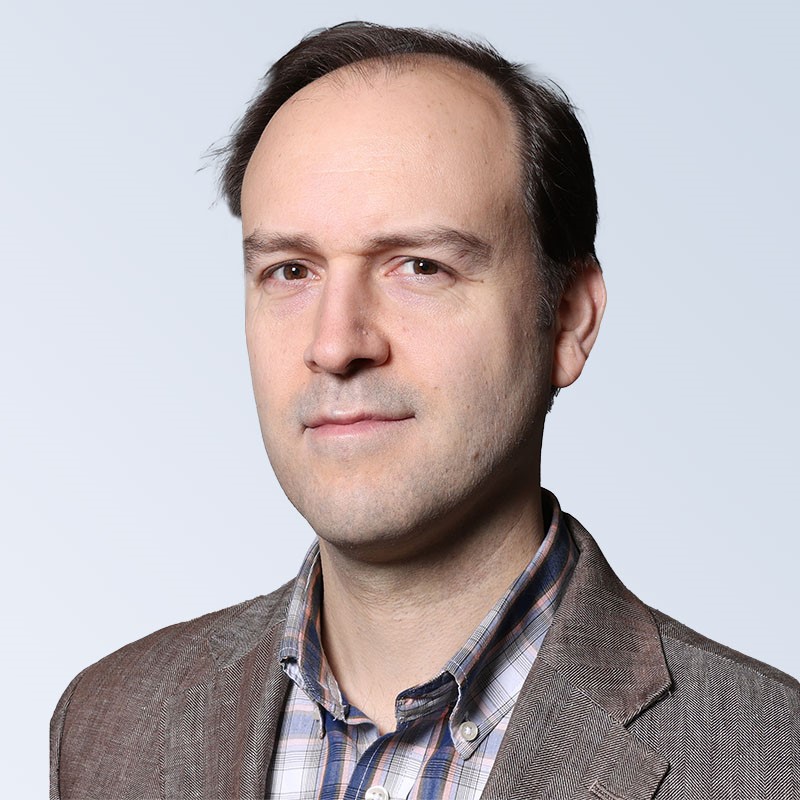
Guillaume Obozinski graduated with a PhD in Statistics from UC Berkeley in 2009. He did his postdoc and held until 2012 a researcher position in the Willow and Sierra teams at INRIA and Ecole Normale Supérieure in Paris. He was then Research Faculty at Ecole des Ponts ParisTech until 2018. Guillaume has broad interests in statistics and machine learning and worked over time on sparse modeling, optimization for large scale learning, graphical models, relational learning and semantic embeddings, with applications in various domains from computational biology to computer vision.
description
Motivation
The project aimed to precisely characterize the distribution over time of air pollution in Europe between 2011 and 2019 and assess the impact of air pollution on health outcomes in Switzerland.
Proposed Approach / Solution
SDSC along with the PSI partners developed methods for integrating PDE-based simulation of pollution transport with observations in over ~400 unique locations in Europe. We used statistics and machine learning to create a high-performing downscaling model that allows us to recover the pollution profiles in all over Europe on a grid composed of cells of 200m.
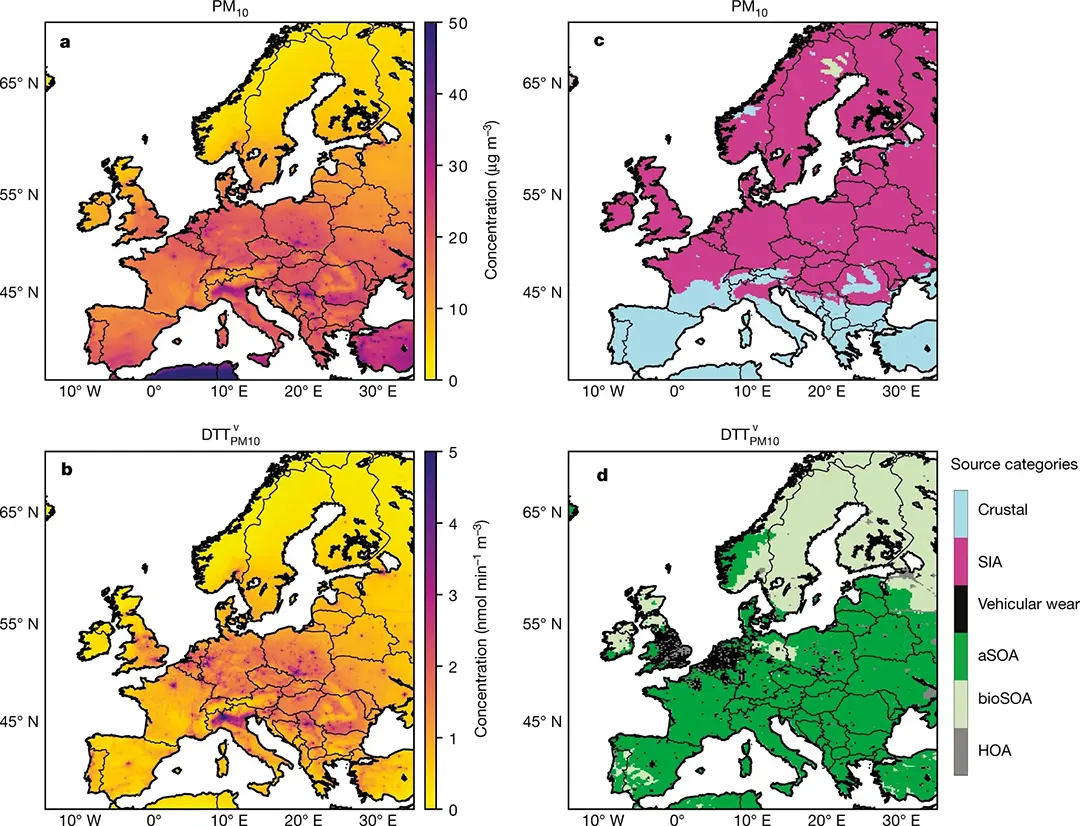
Impact
We estimated and stored the predictions of the machine learning models for the concentrations of Organic Aerosol in Europe. These can be used to examine the exposure of the population to said pollutants and its health consequences, which will also be used in follow-ups of this project.
Presentation
Gallery
Annexe
Additional resources
Bibliography
- Chen, Y. et al. European aerosol phenomenology − 8: Harmonised source apportionment of organic aerosol using 22 Year-long ACSM/AMS datasets. Environ. Int.. 166, 107325 (2022)
- Zhang, X. et al. Ecological Study on Global Health Effects due to Source-Specific Ambient Fine Particulate Matter Exposure. Environ. Sci. Technol. 57, 1278–1291 (2023).
- Chen J. et al. Long-term exposure to fine particle elemental components and natural and cause-specific mortality-a pooled analysis of eight European cohorts within the ELAPSE project. Environ Health Perspect. 129,4 :47009 (2021).
Publications
Related Pages
More projects
MAGNIFY
News
Latest news
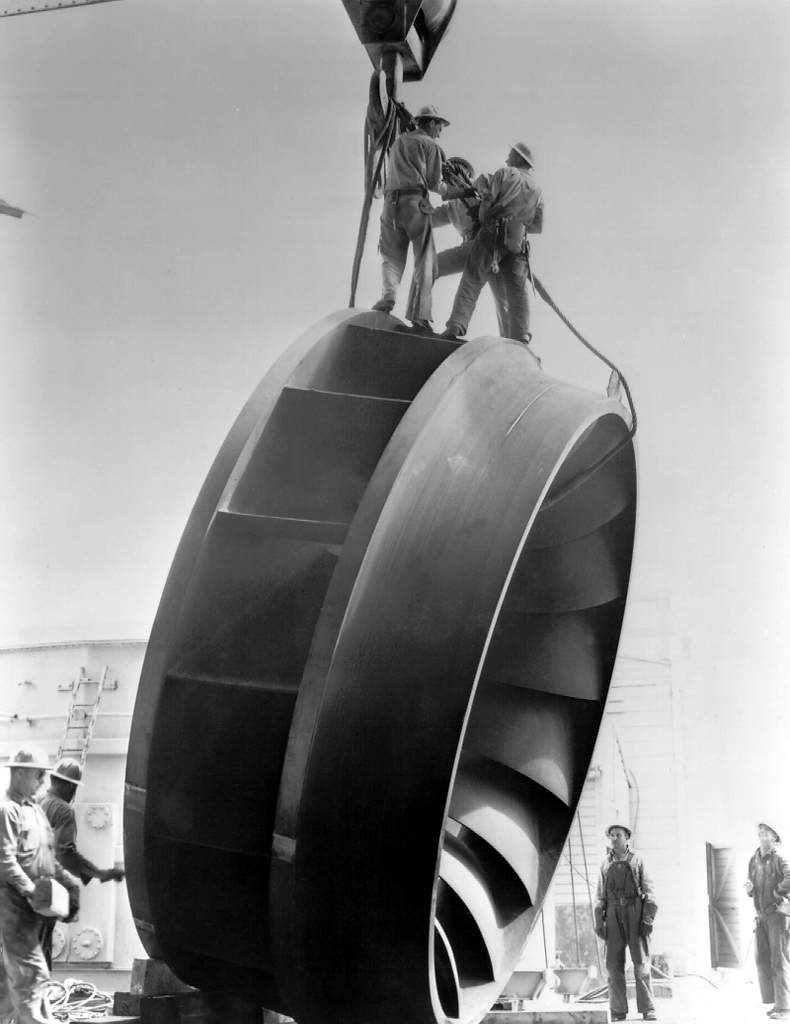
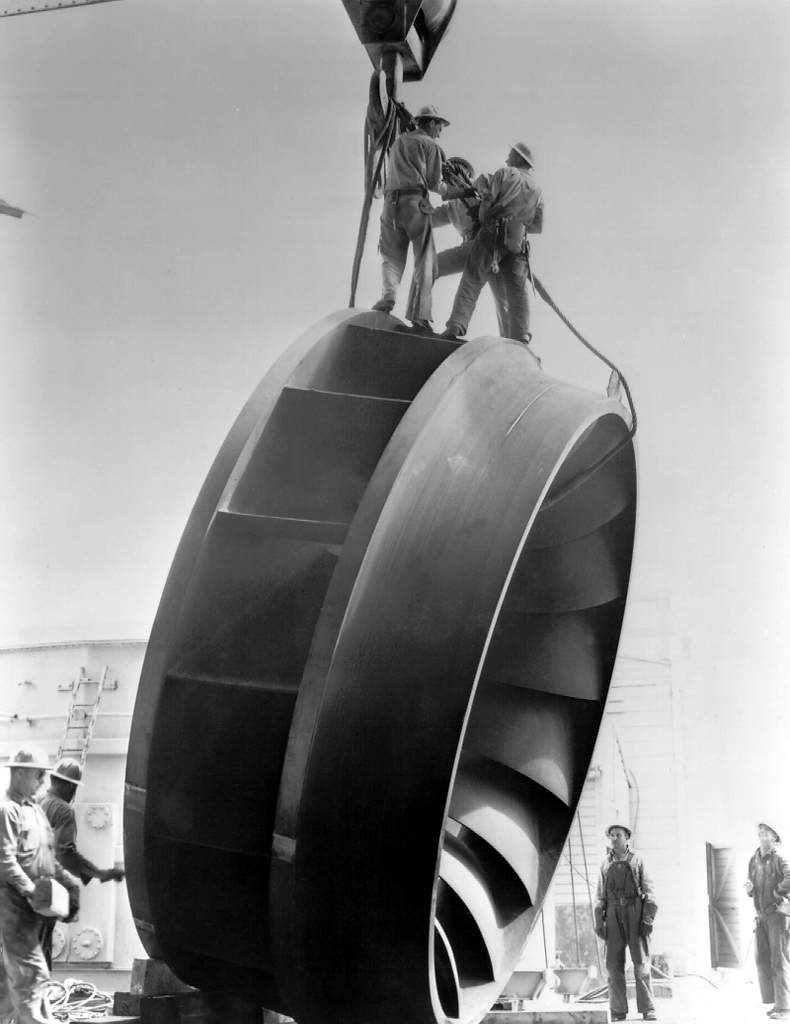
PAIRED-HYDRO | Increasing the Lifespan of Hydropower Turbines with Machine Learning
PAIRED-HYDRO | Increasing the Lifespan of Hydropower Turbines with Machine Learning


First National Calls: 50 selected projects to start in 2025
First National Calls: 50 selected projects to start in 2025
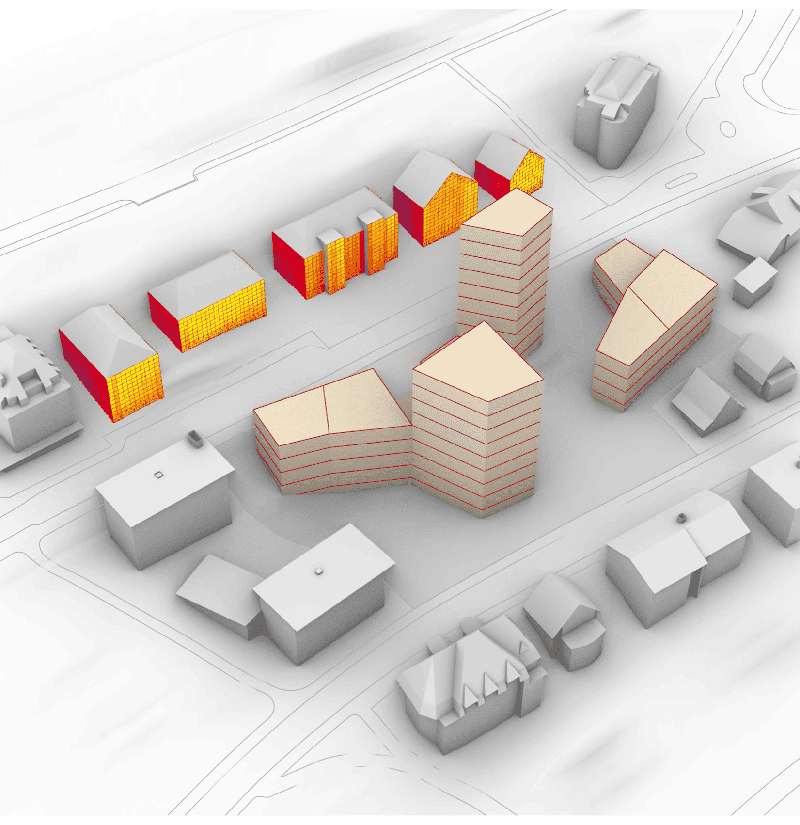
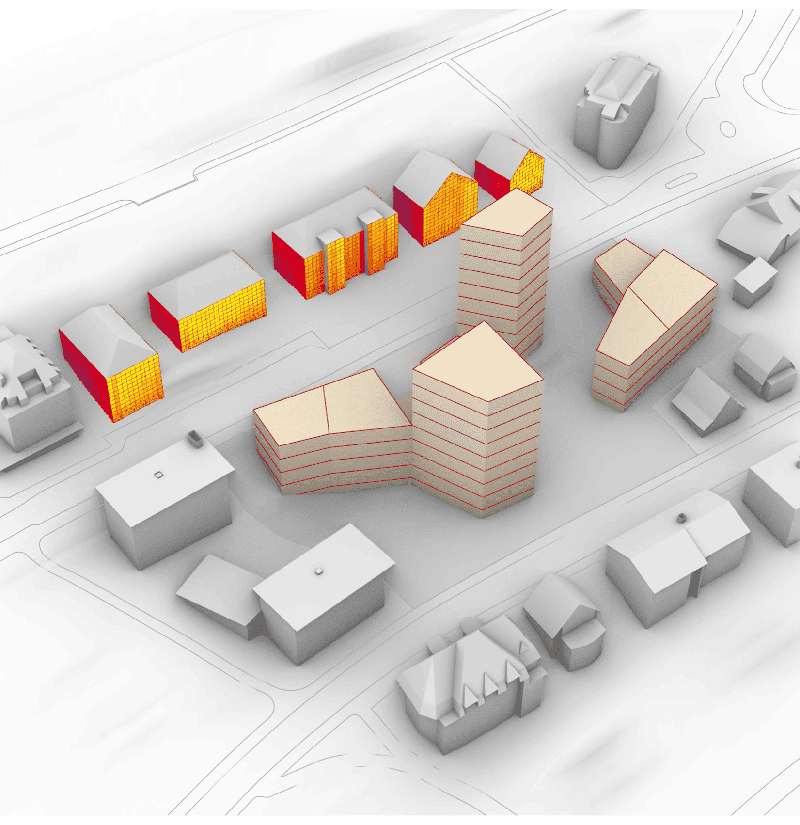
AIXD | Generative AI toolbox for architects and engineers
AIXD | Generative AI toolbox for architects and engineers
Contact us
Let’s talk Data Science
Do you need our services or expertise?
Contact us for your next Data Science project!