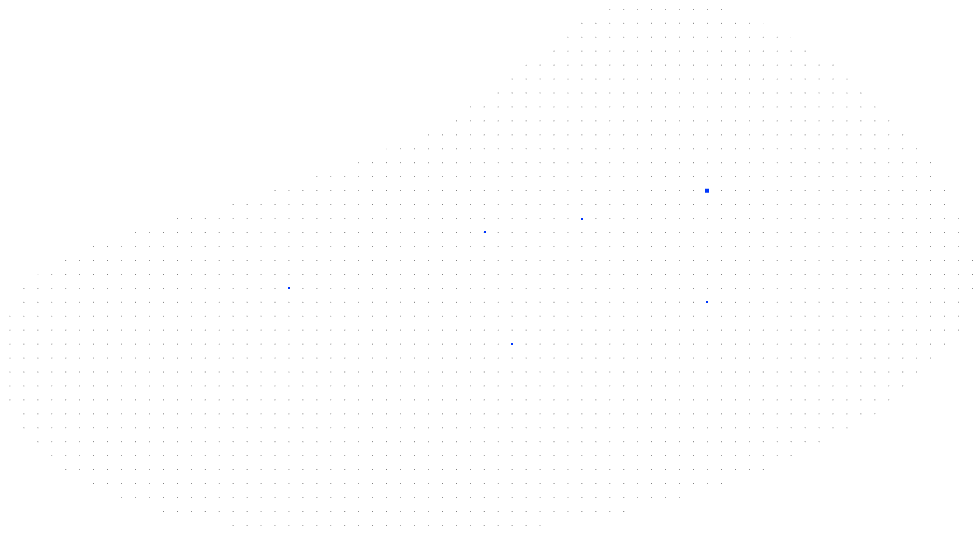
AADS
Data Science Enabled Acoustic Design
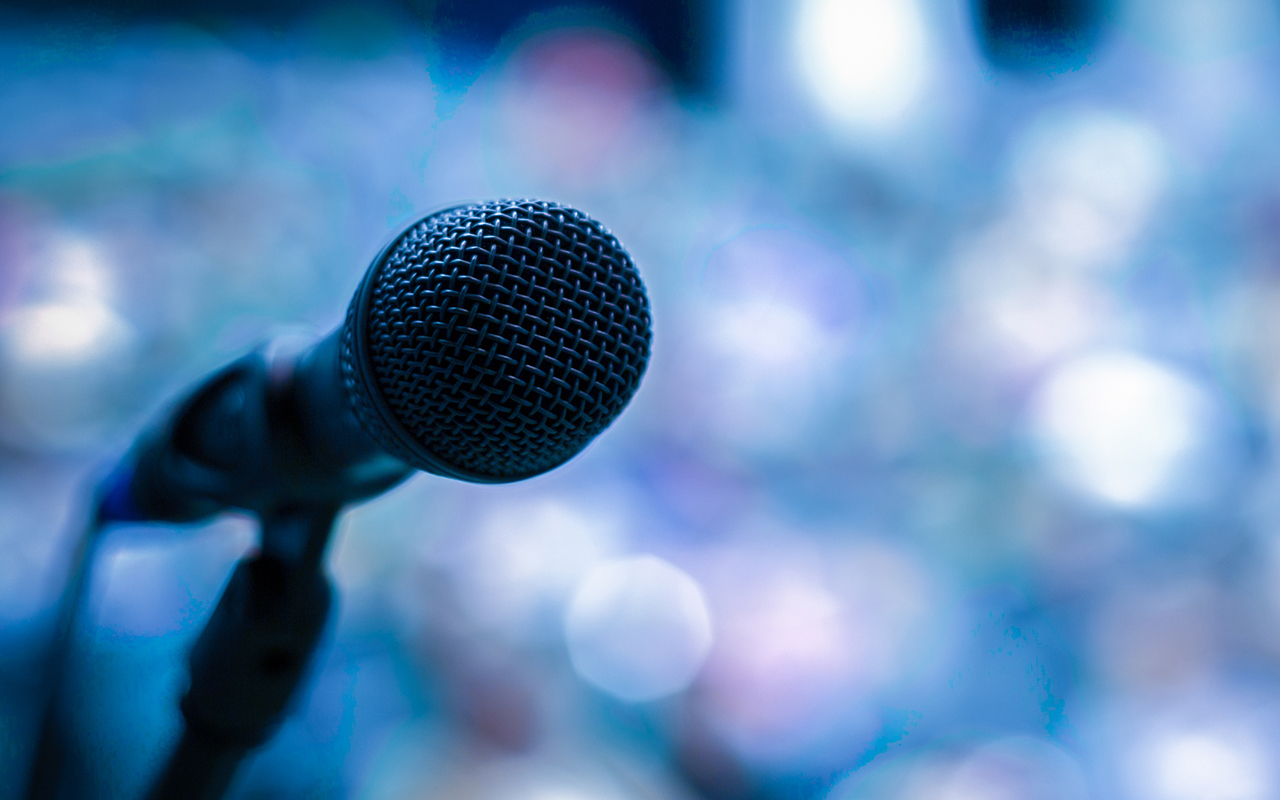
Abstract
Today, 54 percent of the world’s population lives in urban areas, a proportion that is expected to increase to 66 percent by 2050 [1]. Increasing urbanization demands for sustainable design and planning of dense urban spaces to provide healthy environments and support the well-being of the population [2, 3, 4, 5]. However, today architectural acoustic design is often limited to noise reduction or to typologies that demand high-end acoustics like concert halls or auditoriums. It is every so often neglected in the consideration for the design of the vast majority of the built environment, leading to negative health effects from acoustic pollution, cost for noise abatement measures and unaesthetic retrofitting of built structures both indoors and outdoors.
People
Collaborators
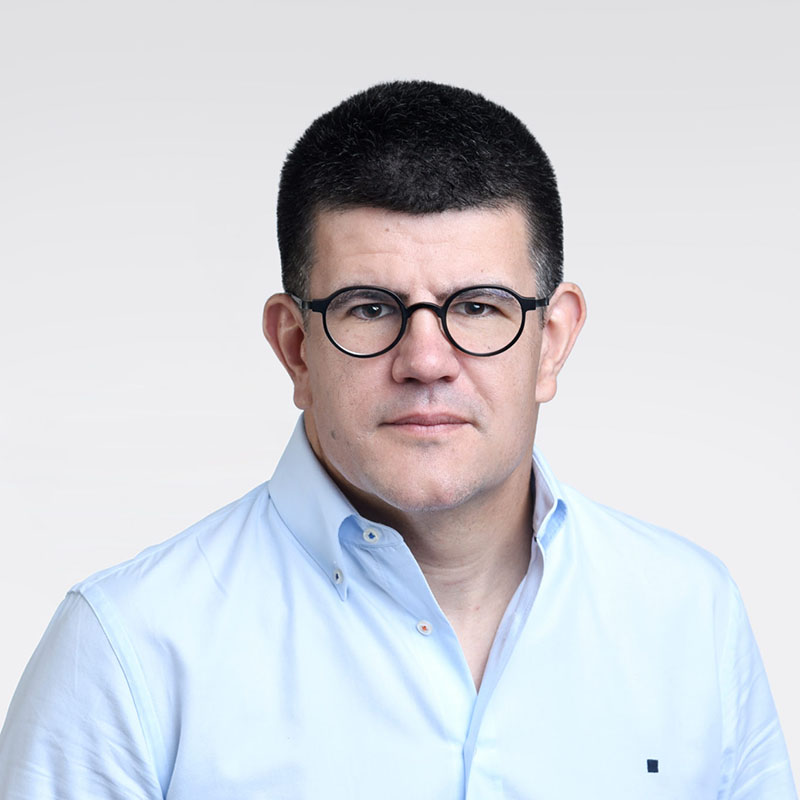
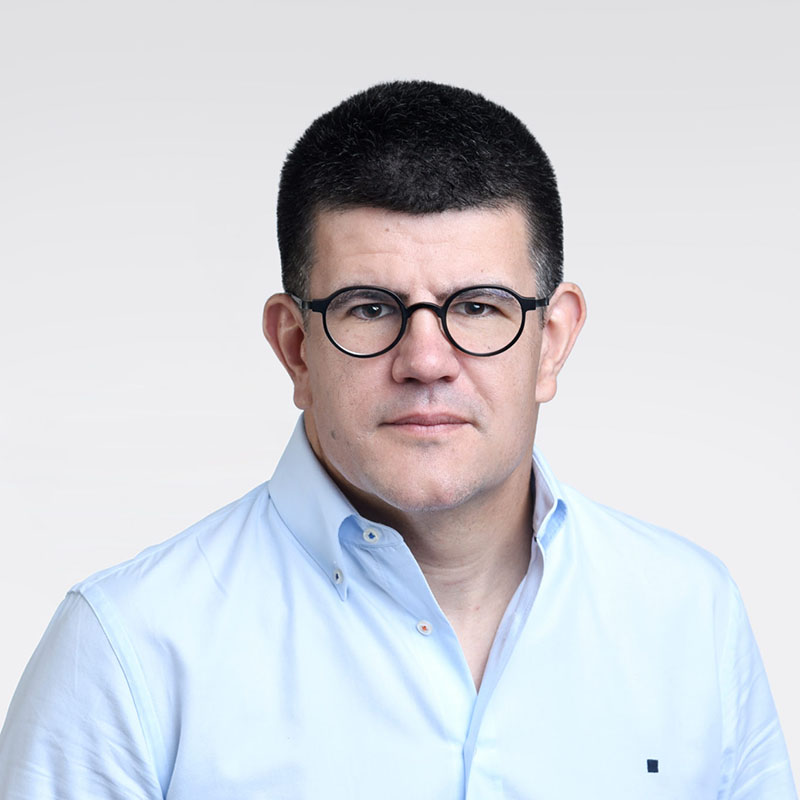
Fernando Perez-Cruz received a PhD. in Electrical Engineering from the Technical University of Madrid. He is Titular Professor in the Computer Science Department at ETH Zurich and Head of Machine Learning Research and AI at Spiden. He has been a member of the technical staff at Bell Labs and a Machine Learning Research Scientist at Amazon. Fernando has been a visiting professor at Princeton University under a Marie Curie Fellowship and an associate professor at University Carlos III in Madrid. He held positions at the Gatsby Unit (London), Max Planck Institute for Biological Cybernetics (Tuebingen), and BioWulf Technologies (New York). Fernando Perez-Cruz has served as Chief Data Scientist at the SDSC from 2018 to 2023, and Deputy Executive Director of the SDSC from 2022 to 2023
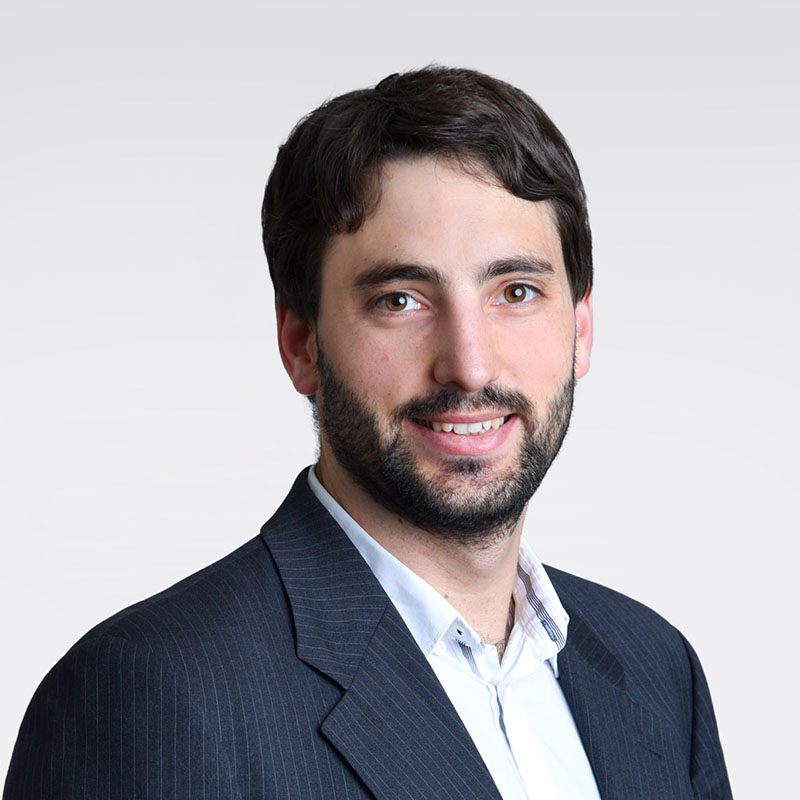
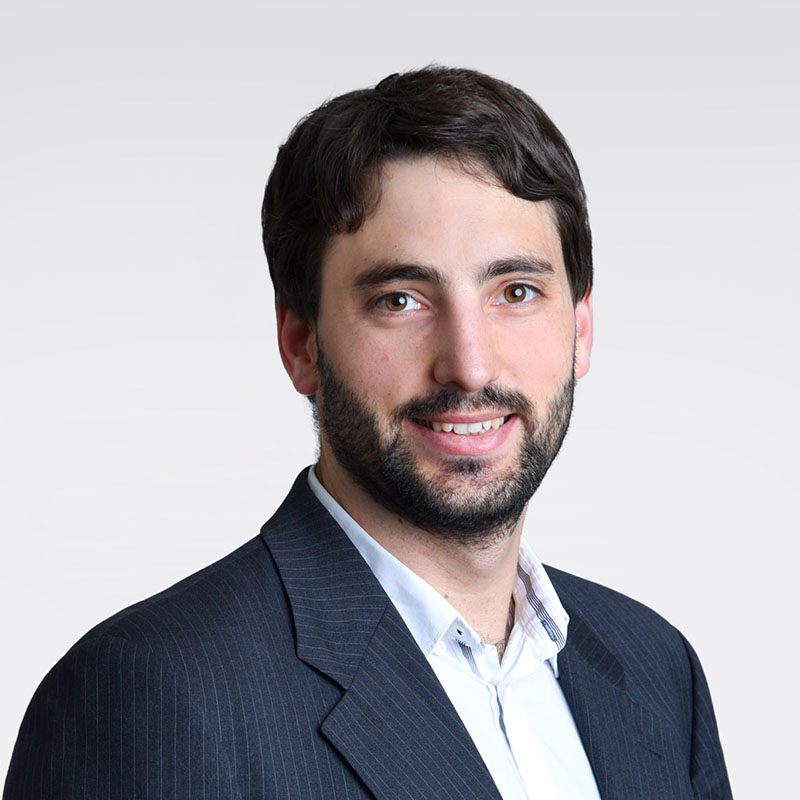
After finishing his Master in electrical engineering at the Ecole Fédérale de Lausanne (EPFL), Nathanaël worked as a researcher in the Acoustic Research Institute (ARI) in Vienna. In 2013, he returned to EPFL for a PhD, where he specialized in different fields of data science: signal processing, machine learning, graph theory and optimization. Furthermore, he created two open source libraries for optimization (UNLocBoX) and graph signal processing (GSPBOX). In 2017, Nathanaël Perraudin joined the Swiss Data Science Center in the ETH Zurich team as a Research Data Scientist. He focused on different aspects of deep learning in the area of generative models (VAE and GAN), recursive architectures and convolutional neural network for irregular domains. Outside office hours, he is passionate in tango dancing, tandem bike touring, skiing and rock climbing.
description
In acoustic design, there is a multitude of interacting parameters and nonlinear relationships, resulting in complex acoustic wave phenomena [6, 7]. While certain acoustic effects such as pure geometric reflections can be digitally modeled, the accurate three-dimensional wave simulation of complex architectural build-ups integrating acoustic diffusion remains a challenge, because computational costs at the interesting frequency range are usually too high [7]. A proven method used to analyze and validate acoustic phenomena of buildings are physical scale models [6]. Here, sound sources are installed at required positions, emitting sound in a scaled frequency range and the corresponding audio signals are recorded at different positions of the model. The resulting measurements can be applied to analyze the acoustic performance [7] and improve the design [8]. However, this method is extremely time- and resource-inefficient, as the amount of variations on the design are limited to the number of built models.
Recent advances in digital fabrication technology allow the efficient production of non-standard architectural structures with complex geometries and textures. Contrary to traditional flat building components, digitally fabricated structures can adapt to different acoustic requirements. By controlling the variation of depth and cavities at multiple resolutions, a required acoustic performance can be achieved. The proposed research takes advantage of an automated robotic setup to test a high number of digitally fabricated articulated walls and a set of reference walls for their sound performance: By automatically deploying audio sources and receivers on varying positions, a consistent data set of measurements of diverse spatiotemporal-acoustic scenarios can be collected. As such, the proposed work targets the acquisition of a multi-variable data collection that contains geometric as well as annotated acoustic information. The physically derived data set serves as a foundation to analyze relations between geometrical and acoustical configurations, to analyze performance clusters in relation to acoustic reference parameters, in order to enable novel designs that are optimized for specific acoustic criteria, while maintaining fabrication feasibility.
This research presents a significant step towards understanding the mutual relationship between build and acoustic spaces. Here, data science will help to a) understand the patterns that are implicit in the data, b) to discover particular sonic signatures, c) to enable design exploration from the learned data and d) – in the future – to synthesize new geometries that create particular sonic configurations significantly impacting our built environment.
Problem
As of today, acoustic design is often neglected in the majority of the built environment because it is too complex or too expensive. One major issue is that the simulation of sound diffusion at different frequencies is computationally expensive and often does not always represent well the reality.
Solution
In this project, we 3D printed and measured more than 300 surfaces including complex geometries. Using an automatic setup and an anechoic chamber, we then recorded the acoustic response for multiple location.
As a next step, we created a dataset of geometries and impulse response and applied Machine Learning algorithms to predict the acoustic response of non-measured surfaces.
Impact
Our goal is to bridge real-world models and data models in order to have a high impact in architectural acoustics.
Presentation
Gallery
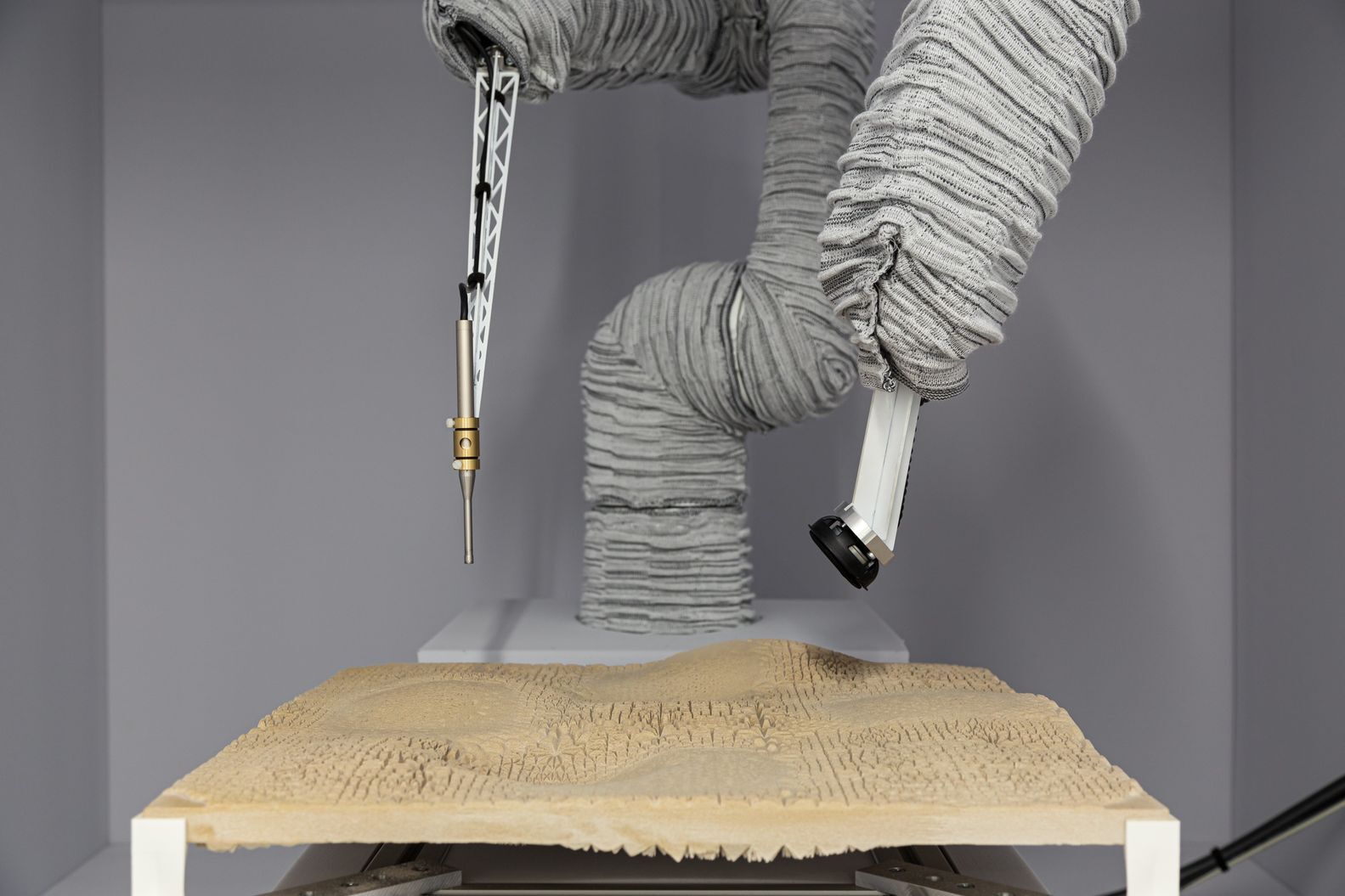
Annexe
Additional resources
Bibliography
- United Nations, 2014. Revision of World Urbanization Prospects. Accessed online 19.04.2017. URL:
World Urbanization Prospects - Population Division - United Nations - W. Kropp, J. Forssén, & L. Estévez Mauriz (Eds.), Urban sound planning – the SONORUS project (pp. 37- 51). DanagårdLiTHO.
- T. J. Cox, P. D'Antonio. „Acoustic absorbers and diffusers“. 3rd ed. Taylor and Francis, 2017
- I. Medebach, T. Gutenkunst, A. Hannemann, A. Lykartsis, and S. Weinzierl. ”Classification of Geometric Room Shapes Through Room Acoustic Parameters By Using Machine Learning Algorithms”. In: Proceedings DAGA 2017, 2017, pp. 253-256.
- M. Vomhof, L. Vasey, S. Bräuer, K. Eggenschwiler, J. Strauss, F. Gramazio, and M. Kohler. "Robotic Fabrication of Acoustic Brick Walls". In: Proceedings of the 34th Annual Conference of the Association for Computer Aided Design in Architecture (ACADIA). Ed. by D. Gerber, Los Angeles, USA: Roundhouse Publishing Group, 2014, pp. 555–564. URL: http://www.gramaziokohler.com/data/publikationen/1090.pdf
- A. Sanavi, B. Schäffer, K. Heutschi, K. Eggenschwiler “On the effect of acoustic diffusers in comparison to absorbers on the subjectively perceived quality of speech in ordinary rooms”, Submitted to Acta Acustica united with Acustica, in review since July 2017
- Heutschi, K. „Incoherence factor as descriptor for the diffusivity of building facades“. Acta Acustica United with Acustica, 97(6), 933-939. 2011
Publications
Related Pages
More projects
MAGNIFY
News
Latest news
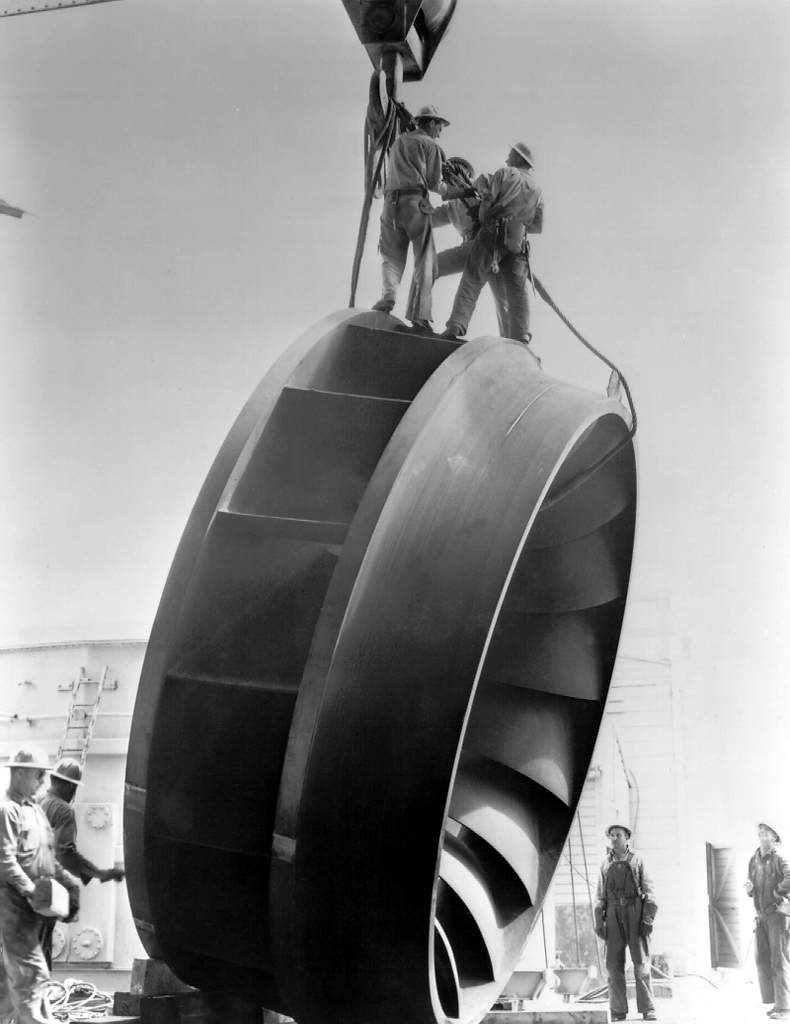
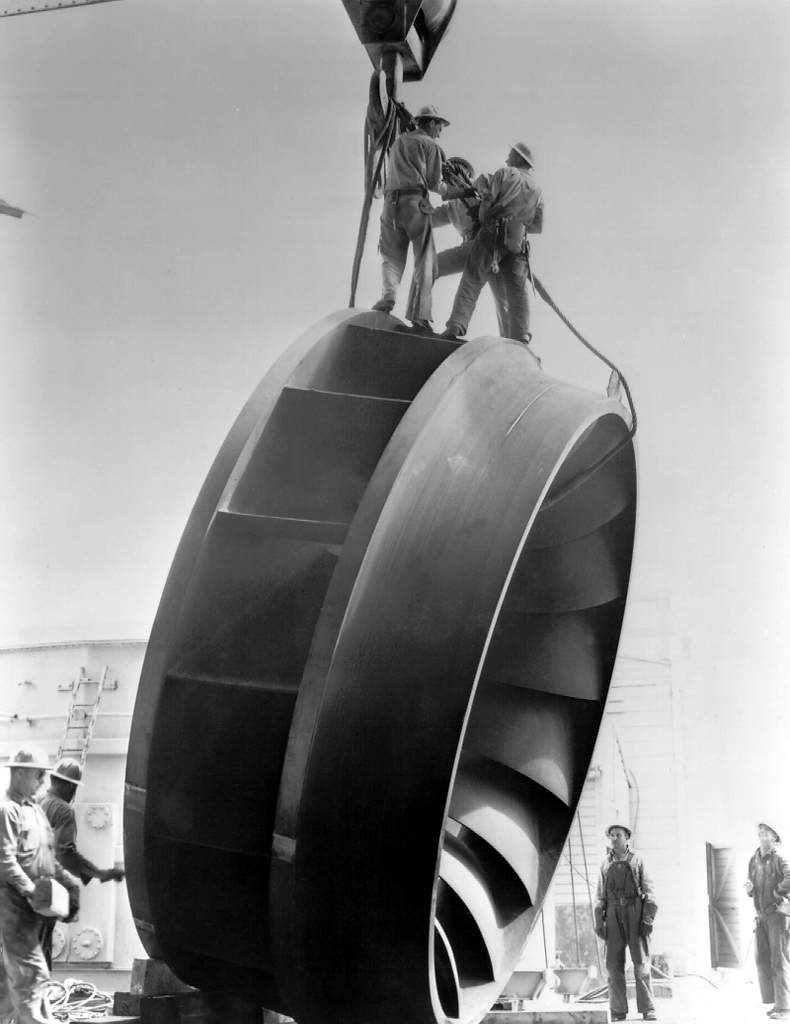
PAIRED-HYDRO | Increasing the Lifespan of Hydropower Turbines with Machine Learning
PAIRED-HYDRO | Increasing the Lifespan of Hydropower Turbines with Machine Learning


First National Calls: 50 selected projects to start in 2025
First National Calls: 50 selected projects to start in 2025
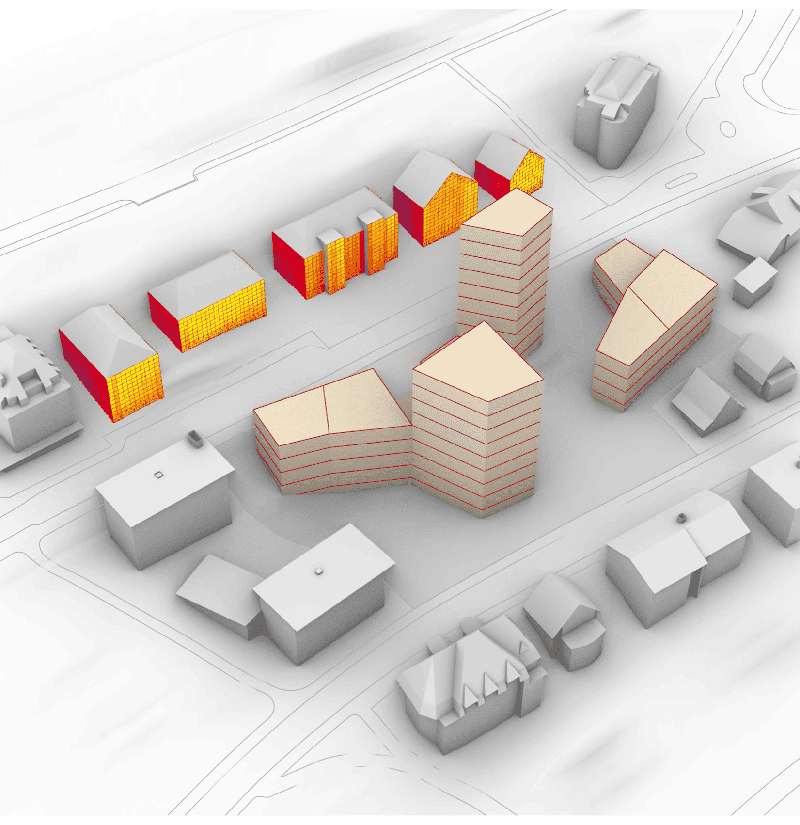
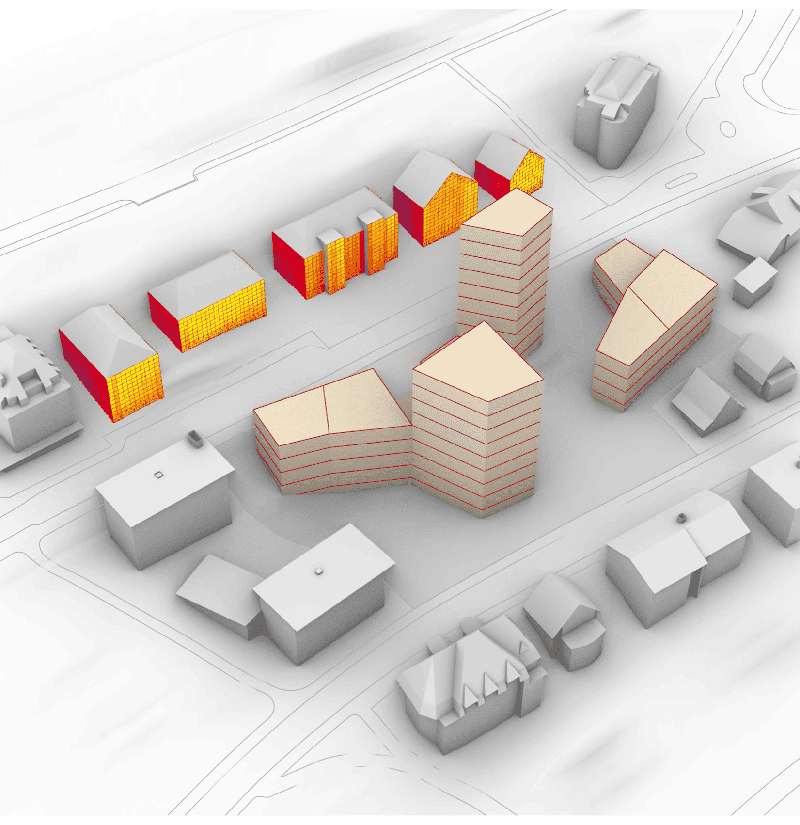
AIXD | Generative AI toolbox for architects and engineers
AIXD | Generative AI toolbox for architects and engineers
Contact us
Let’s talk Data Science
Do you need our services or expertise?
Contact us for your next Data Science project!