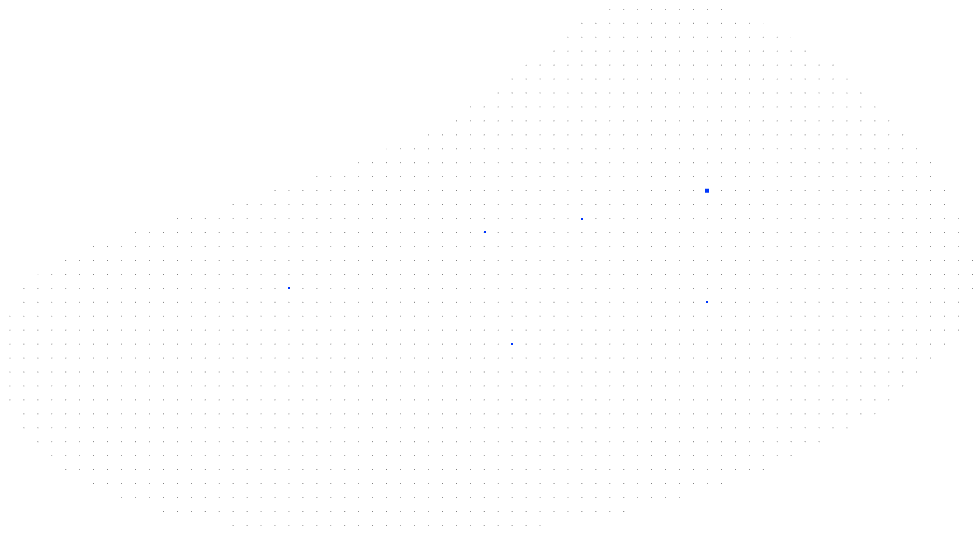
4D-Brains
Extracting Activity from Large 4D Whole-Brain Image Datasets

Abstract
Whole-brain recordings hold promise to revolutionize neuroscience. In the last decade, innovations in fast 3D microscopy, protein engineering, genetics, and microfluidics have allowed brain researchers to read out calcium activity at high temporal resolution from a large number of neurons in the brains of Caenorhabditis elegans, Danionella translucida, Hydra, and zebrafish simultaneously. This technology is considered to be a game changer for neuroscience as it leaves far fewer variables hidden than when only a small fraction of neuronal activities could be recorded. Many fundamental and challenging questions of neuroscience can now be pursued. For example: What global brain activity determines an organism's responses to stimuli? How are decisions computed by networks of neurons? What is the idle activity of an unstimulated brain?
The field suffers from a critical bottleneck. Neuronal activities are recorded as local intensity changes in 4D microscopy images. Extracting this information for a moving animal is very labor-intensive and requires expertise. The promise of whole-brain recordings cannot be fully realized unless the image analysis problem is solved.
There are several challenges: A) 3D images are generally difficult to annotate manually. B) The worm moves, rotates, bends, and compresses fast. C) To avoid blurring, the exposure time and the image quality are limited. D) The resolution in the z-direction is low.
People
Collaborators


Corinne joined the SDSC as a senior data scientist in December 2020. She graduated with a PhD in Statistics from the University of Washington in 2020. Her doctoral research focused on representation learning for partitioning problems. Prior to her PhD, she obtained bachelor's degrees in Math, Statistics, and Economics, along with a master's degree in Economics, from Penn State University. Her research interests include deep learning and kernel-based methods, with applications in fields ranging from computer vision to oceanography.


Isinsu joined the SDSC in May 2022. She obtained a Bachelor’s degree in Computer Science from Middle East Technical University, Turkey, and later received a Master’s degree in Computer Science from EPFL. She conducted her doctoral studies in the Computer Vision Lab at EPFL with a focus on human body pose estimation and motion prediction using deep learning-based methods. Her research interests include self- and semi-supervised learning for image-based analysis of the human body and motion, with applications ranging from athletic training to healthcare.
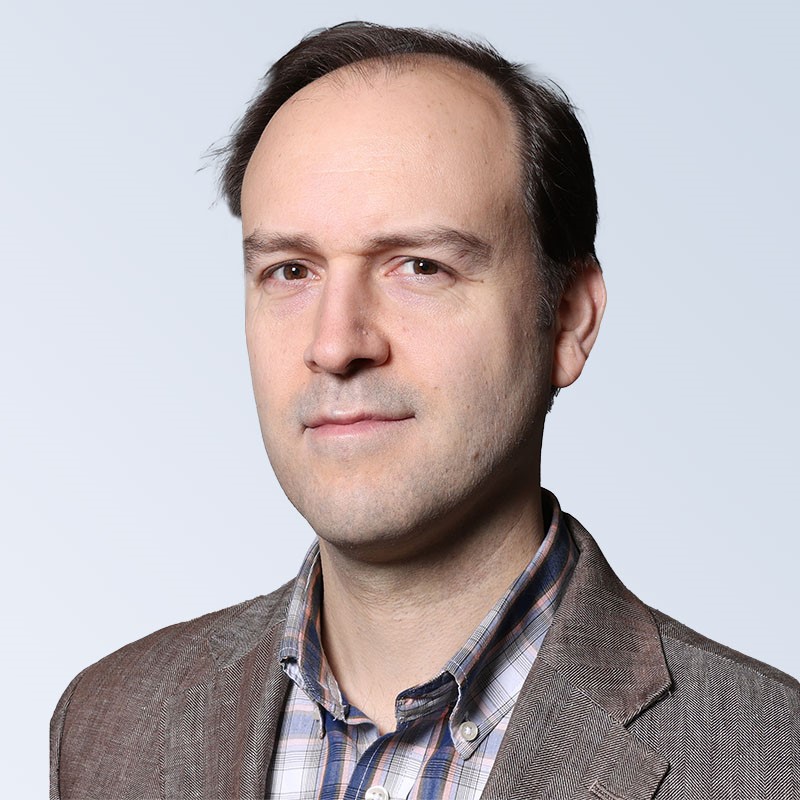
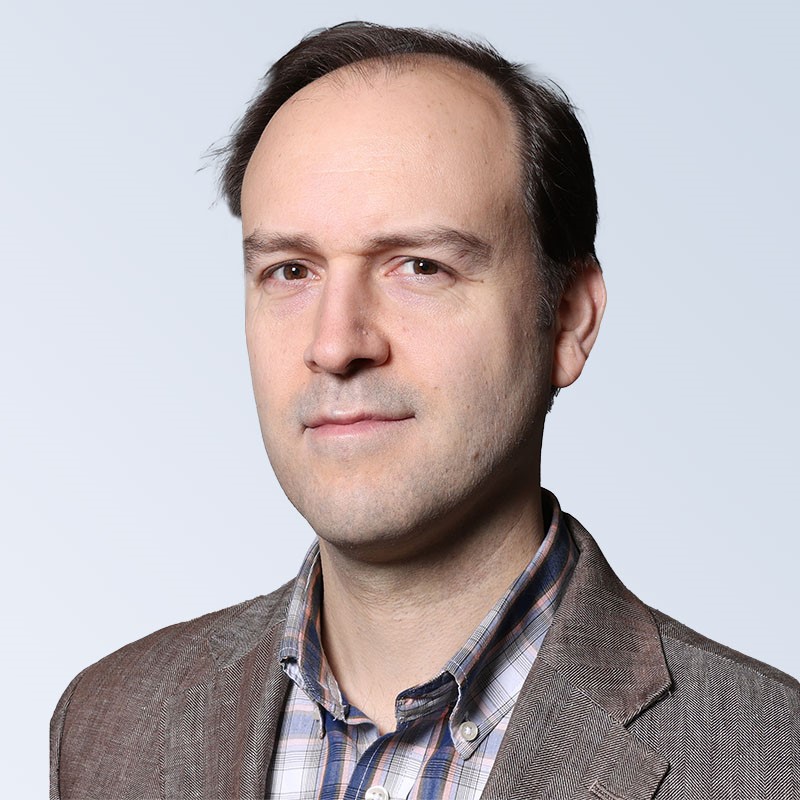
Guillaume Obozinski graduated with a PhD in Statistics from UC Berkeley in 2009. He did his postdoc and held until 2012 a researcher position in the Willow and Sierra teams at INRIA and Ecole Normale Supérieure in Paris. He was then Research Faculty at Ecole des Ponts ParisTech until 2018. Guillaume has broad interests in statistics and machine learning and worked over time on sparse modeling, optimization for large scale learning, graphical models, relational learning and semantic embeddings, with applications in various domains from computational biology to computer vision.
PI | Partners:
EPFL, Laboratory of the Physics of Biological Systems:
- Prof. Sahand Rahi
- Dr. Elif Gençtürk
- Alice Gross
- Mahsa Barzegarkeshteli
- Matthieu Schmidt
description
Motivation
Tracking cells and freely moving animals in timelapse recordings is critical to many areas of biology but continues to involve time-consuming manual labor. In our specific application in C. elegans neuroscience, novel genetically encoded calcium indicators and 4D microscopy techniques would make it possible to record neuronal activity at single-neuron resolution in the brains of naturally behaving C. elegans, if it was possible to reliably segment and track these neurons. While this 3D visualization technology opens up new avenues for investigating brain computations, segmenting and tracking imaged neurons over time (see Figure 1) is extremely challenging. The goals of the collaboration consist of identifying specific neurons across 4D images (segmentation & tracking), mapping every pixel in 4D images onto a 3D reference (registration) and speeding up the former tasks for real-time feedback to the animal.
Proposed Approach / Solution
In this project, we present an approach based on graph matching for tracking fluorescently-marked neurons in freely-moving C. elegans. In our approach, each frame is represented by a complete graph, with the somas and (parts of) neurites being the nodes. Our algorithm learns to use the node features (locations and shapes of the objects) and edge features (distances between the objects) to match the nodes in each frame with nodes in a reference frame. Neurites (and sometimes neurons) can be oversegmented into pieces at the preprocessing phase; our algorithm allows several segments to match the same reference neuron or neurite. In addition to this, we also propose to leverage generative deep learning models to create realistic synthetic datasets that are annotated automatically. The idea is to take a single image of C. elegans which is annotated manually and to deform non-linearly this image so as to produce a large number of other images of the same worm in different anatomical conformations, while preserving the image texture and the fluorescence, and transferring the annotations.
Impact
Efficient image analysis techniques would reduce the burden of manual annotation and unleash the growth of the field. Faster image analysis would mean that more and a more diverse range of experiments can be performed, and more animals can be analyzed, making results more statistically rigorous. Moreover, high-throughput neuroscience with freely moving animals will become possible, and new questions will become accessible, for example, individual differences between animals could be studied in a statistically rigorous way.
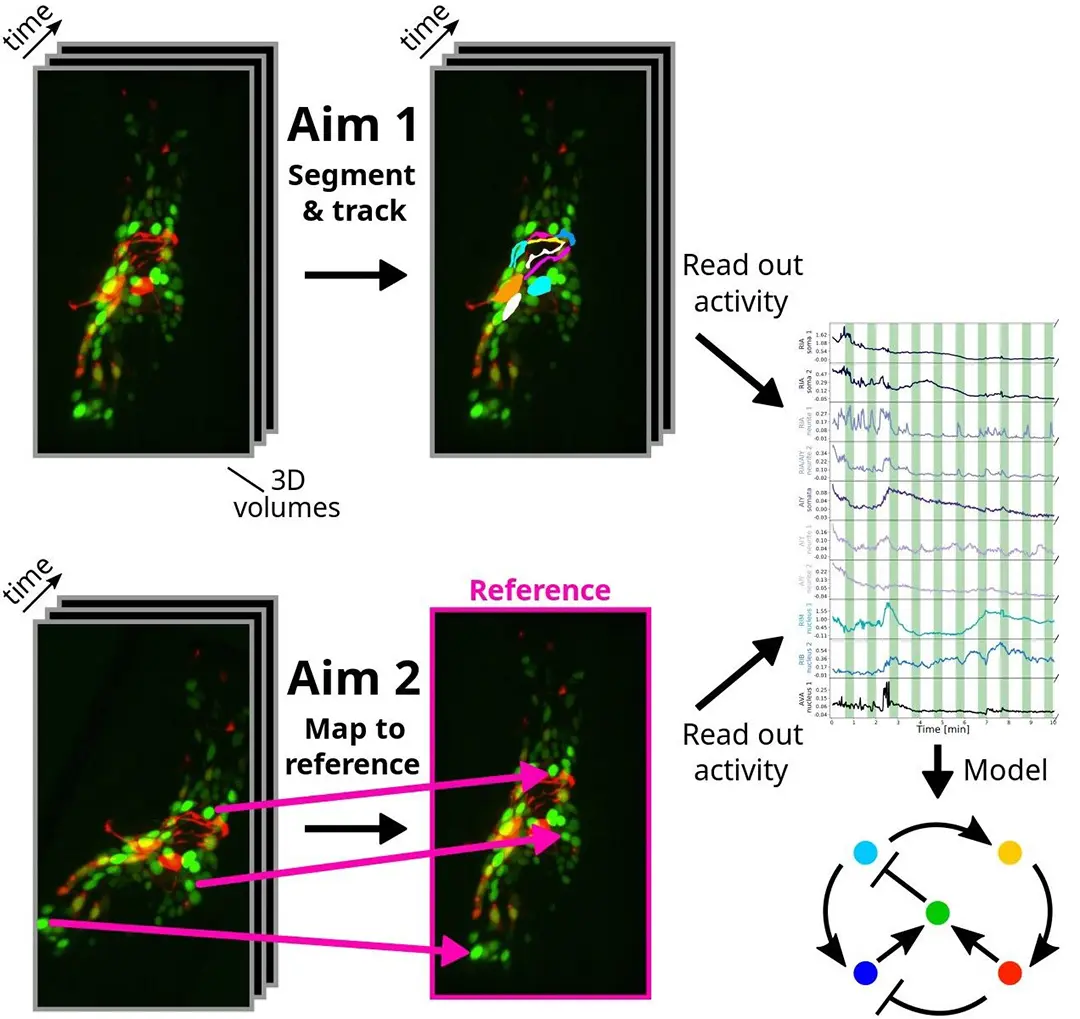
Presentation
Gallery
Annexe
Additional resources
Bibliography
- K. Chaitanya, N. Karani, C. F. Baumgartner, E. Erdil, A. Becker, O. Donati and E. Konukoglu. Semi-supervised task-driven data augmentation for medical image segmentation. In Medical Image Analysis, vol. 68, p. 101 934, 2021.
- S. Chaudhary, S. A. Lee, Y. Li, D. S. Patel, and H. Lu. Graphical-model framework for automated annotation of cell identities in dense cellular images. In eLife 10:e60321, Feb. 2021.
- S. Kato, H. S. Kaplan, T. Schrödel, S. Skora, T. H. Lindsay, E. Yemini, S. Lockery, and M. Zimmer. Global brain dynamics embed the motor command sequence of Caenorhabditis elegans. In Cell, 163 (3):656–669, Oct. 2015.
- J. Mu , S. D. Mello, Z. Yu, N. Vasconcelos, X. Wang, J. Kautz and S. Liu. CoordGAN: Self-Supervised Dense Correspondences Emerge from GANs. In IEEE/CVF Conference on Computer Vision and Pattern Recognition (CVPR), 2022.
- J. P. Nguyen, A. N. Linder, G. S. Plummer, J. W. Shaevitz, and A. M. Leifer. Automatically tracking neurons in a moving and deforming brain. In PLOS Computational Biology, 13(5):1–19, 05 2017.
- W. Peebles, J.-Y. Zhu, R. Zhang, A. Torralba, A. Efros, and E. Shechtman. GAN-Supervised Dense Visual Alignment. In IEEE Conference on Computer Vision and Pattern Recognition (CVPR), 2022.
- X. Yu, M. S. Creamer, F. Randi, A. K. Sharma, S. W. Linderman, and A. M. Leifer. Fast deep neural correspondence for tracking and identifying neurons in C. elegans using semi-synthetic training. In eLife, 10:e66410, Jul. 2021.
Publications
Related Pages
More projects
MAGNIFY
News
Latest news


First National Calls: 50 selected projects to start in 2025
First National Calls: 50 selected projects to start in 2025
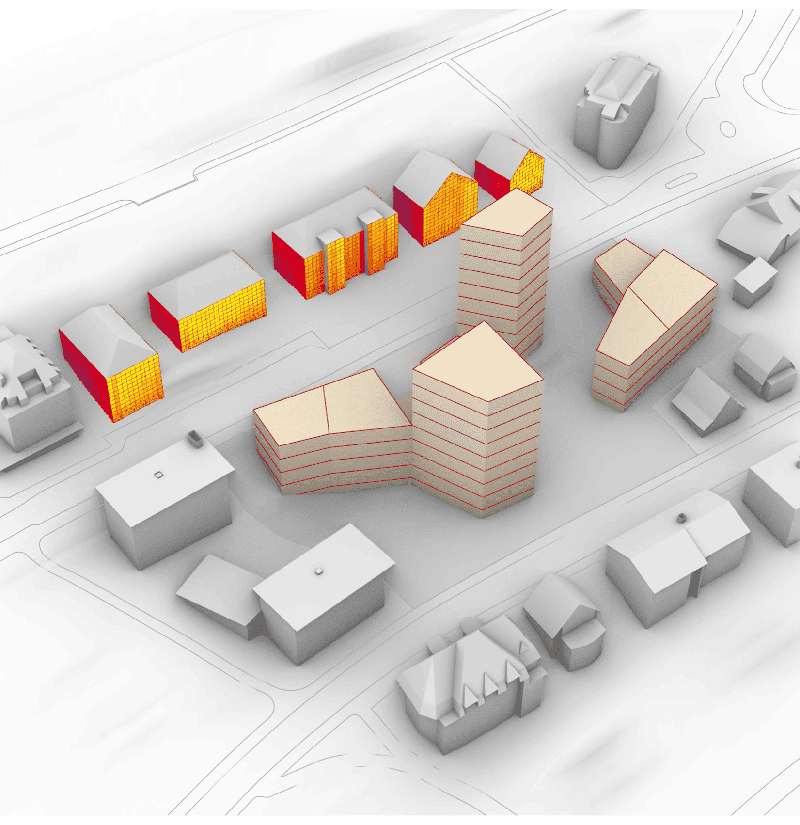
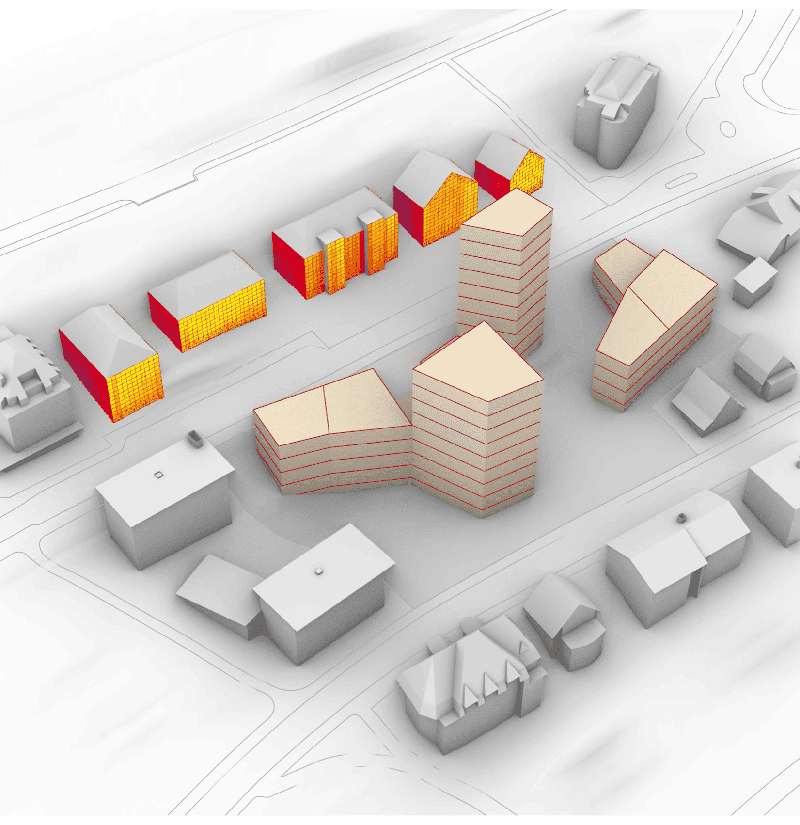
AIXD | Generative AI toolbox for architects and engineers
AIXD | Generative AI toolbox for architects and engineers


Smartair | An active learning algorithm for real-time acquisition and regression of flow field data
Smartair | An active learning algorithm for real-time acquisition and regression of flow field data
Contact us
Let’s talk Data Science
Do you need our services or expertise?
Contact us for your next Data Science project!