In the project entitled “A research platform for data-driven democracy studies in Switzerland” (DemocraSci), we are performing a comprehensive analysis of the Swiss parliament archives. The project is a collaboration between the Chair of Systems Design at ETH Zürich, led by Prof. Frank Schweitzer, and the SDSC. Our aim is to create a database of who said what and when in both chambers of the Swiss parliament over the past 127 years. The Swiss Federal Archives (Schweizerisches Bundesarchiv) recently carried out the digitalization of the proceedings of both the National Council and the Council of States. Thanks to these efforts, we can now openly access over 40,000 documents pertaining to all votes, speeches, laws, amendments to laws, etc., from 1891 to the present day. However, without the right tools, it is unfeasible to perform a proper analysis of this corpus. The aim of the project is, therefore, to structure the corpus into a queryable database. This includes the identification of topics of debates or the analysis of speeches to map the political positions and opinions of the members of parliament. In this project, we will create a so-called knowledge graph out of the proceedings, a task never before carried out on such a vast corpus of political archives. This knowledge graph captures different relationships between political entities. Figure 1 gives a mock example of our planned knowledge graph. The graph consists of different nodes (circles in Figure 1) that connect to other nodes. Each line between two nodes indicates the relationship between them. For instance, in the knowledge graph depicted in Figure 1, we can see that Silvia Schenker is a member of the SP party and cosponsored an intervention proposed by Maya Graf.
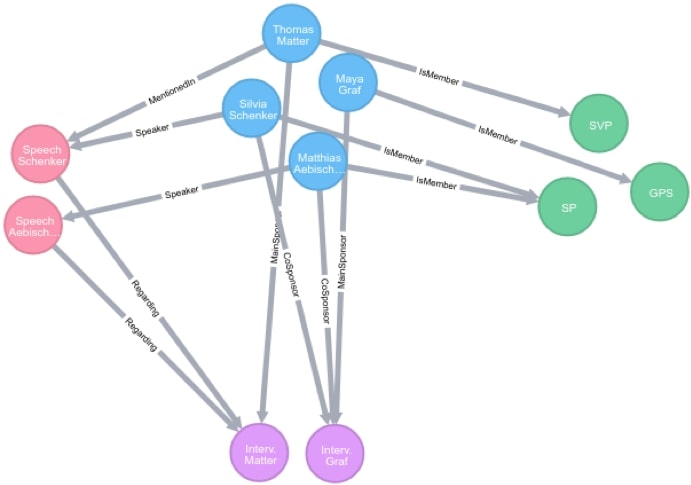
Our knowledge graph will capture different relationships and interactions between political entities such as politicians, parliamentary groups, committees, political parties, bills, interventions, votes, and speeches, for the whole time span from 1891 to today. Such a knowledge graph will be a valuable research tool for political scientists and historians. They will be able to answer a broad range of questions, such as how parties shift their focus over time, what type of conflicts of interest exist and how they arise, and which political topics drive polarization. Moreover, political scientists can analyze how socioeconomic events influence political decisions or study the trends of issues discussed in the councils, and even make predictions about expected voting outcomes or newly forming alliances. In a project of this magnitude, and with such involved data, we first need to curate and extract the useful information from the original raw files, enriching it with any extra information that may be useful for subsequent steps. These tasks are carried out in the first work package (WP) of the DemocraSci project. One important task within the first work package is labeling text lines of every document according to some pre-established categories (see Figure 2, left). For this, we need to detect margins, column separators, and other lines that help to define different sections of the text, as depicted in the right-hand side of Figure 2. Additionally, we need to ensure the proper ordering of all text lines, and their correct grouping into text boxes (see Figure 2, right). Hence, after performing this exhaustive preprocessing pipeline, we end up with a massive corpus of corrected text, mostly belonging to the speeches made by politicians during parliamentary sessions.
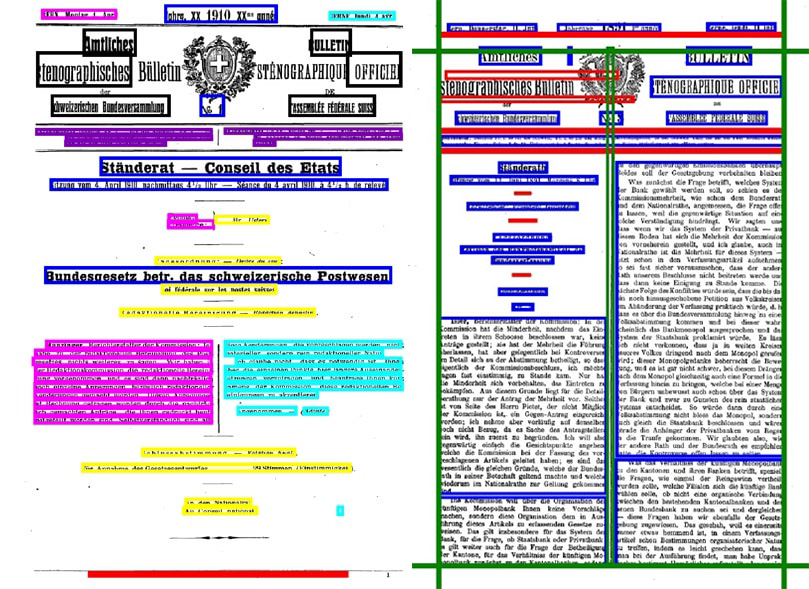
After the extraction of the corpus in WP1, we can proceed with the most interesting part of the project, the natural language processing. This constitutes WP2 of the DemocraSci project, where the main aim is to extract additional entities that further enrich the envisioned knowledge graph, such as topics discussed and their historical evolution, or opinion of politicians on different matters. For all these, there exist well-established techniques on which we can rely. For example, latent Dirichlet allocation (LDA) is, in a nutshell, a technique that allows extracting different lists of relevant words and their associations with the analyzed documents. Each of these lists comprises specific terms that can be assigned to a specific category, i.e., a topic. This way, we can quickly summarize the main topics discussed during each session as well as list the politicians proposing them and also those intervening in the discussions. Given the size and uniqueness of the data set, we can apply more advanced methods. For example, dynamic LDA will allow us to analyze how topics evolved through time, e.g., how the rhetoric on women’s rights or the Swiss energy policy changed over time. Also, with the use of deep recurrent neural networks, we will perform sentiment analysis on the speeches, to elucidate not only the topics discussed by specific politicians, but also their opinion on those subjects. All this rich information we gather from the corpus will be integrated into the knowledge graph. Our knowledge graph will comprise thousands of entities and relations between them. Once ready, this graph will be hosted on an interactive web application where researchers, journalists and the interested public can interact with the data and perform their own analyses. They will be able to go through the topics and find out what was discussed in parliament and when. Also, it will be possible to explore which arguments were used to win a discussion in parliament, or examine how politicians changed their positions over the course of their years of service. Besides, different machine learning methods could be trained on these data, in order to fit models that predict future political outcomes. There are challenging research opportunities based on this data set. By publishing it as fully open access upon successful completion of the project—including the methods and the documentation on how the processing was done—we hope to encourage other digitalization projects and strengthen scientific advances to better understand our past and help to shape our future.
Co-authors
- Lilian Gasser
- Laurence Brandenberger
- Prof. Schweitzer
- Prof. Frank Schweitzer