Biodiversity analyses in an Era of global changes
In order to conserve and manage biodiversity, we need an improved understanding of essential biodiversity drivers and improved predictions of resulting biodiversity patterns in space and time. Patterns and processes behind biodiversity are of utmost importance to mitigate potential threats from global change and support conservation decisions.
The most frequently used approach to study spatial biodiversity patterns is the method of species distribution models (SDMs; Guisan & Zimmermann 2000; Figure 1) that relate the observed species distribution (presences and absences) to a set of environmental predictors (usually derived from maps, such as temperature, precipitation, etc.) by means of statistical models (generalised linear models, generalised boosted models, decision trees, etc.; Figure 1). Because species are generally bound to particular environmental conditions, SDMs enable to characterize the species multidimensional niche volume (or species realized niche).
The state of the art in uncertainty assessment of species distribution models includes the evaluation of multiple predictor sets, of multiple statistical models and of the effect associated with training data choice. Such analyses allow for modelling biodiversity composition in space, for calculating risks of species and biodiversity loss and turnover under climate or land use change scenarios.
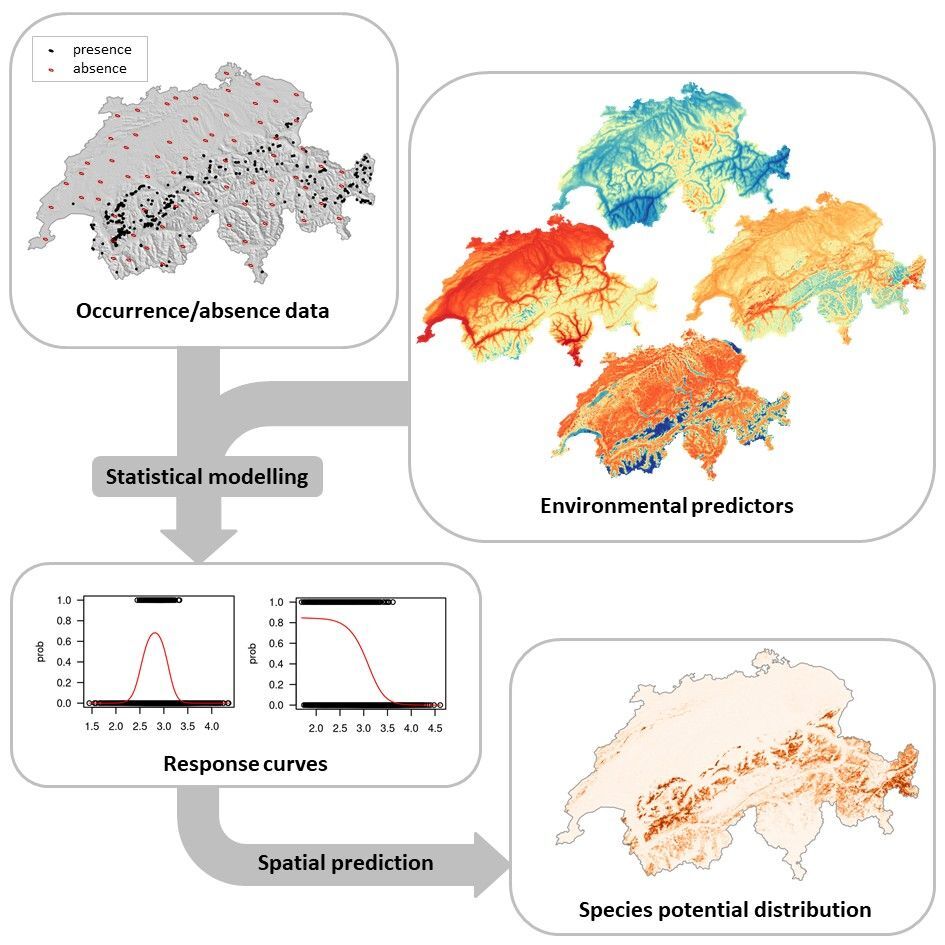
Current Shortcomings in biodiversity analyses
Several major shortcomings in such analyses constitute a problem. First, despite fast growing databases on species distribution, the completeness of species richness sampling is highly biased spatially, temporally and taxonomically (Meyer et al. 2016).
Second, although environmental information as well as species data are becoming available at increasingly finer spatial and temporal resolution (e.g. by means of remote sensing), many biodiversity modelling approaches do not yet make full use of such extensive information. Traditional approaches tend to use only a few predictors that are not too highly correlated and that are well interpretable, a shortcoming stemming rather from computational and analytical limitations.
Third, most biodiversity models focus on individual species and do not make full use of higher-level information. Hence, many congruent approaches are limited with respect to the form of species-environment relationships considered and ignore important additional information, such as trait similarity (e.g. similar leaf thickness, root type and height for plants) or phylogenetic relatedness (it e.g. shared evolutionary heritage) that could help the modelling of rare or poorly-monitored species. Using machine learning approaches to incorporate such additional information would facilitate understanding the potential distribution of species from biased spatial information.
Fourth, integrating all information available from many different data sources has become problematic, due to the sheer mass of possible predictors. A single species distribution framework bringing together all available information would substantially improve our knowledge of the state and threats of biodiversity, innovate our understating of the biodiversity drivers and guide future monitoring efforts of citizen sciences.
SpeedMind – Improving species biodiversity analyses and citizen science feedback through machine learning approaches
The Swiss Federal Institute for Forest, Snow and Landscape (WSL) and the Swiss Data Science Center (SDSC) are actively working towards the development and the study of the benefits of machine learning approaches for facilitating biodiversity assessments.
Applicable to any species group world-wide, SpeedMind focuses on floristic data from Switzerland as a pilot system and aims at predicting plant species distribution (SDMs) in Switzerland by incorporating an extensive amount of data from various sources in a novel way for domain science and to foster the link between ecological sciences and citizen science. While modelling rare plant species is a main challenge using traditional SDMs (because of low data availability), we aim at achieving this by jointly modelling rare species with the more widespread ones and by integrating information on species ecological and morphological similarities. More precisely, we are using Bayesian parametric (the model has a fixed number of parameters) and nonparametric (the number of parameters is unbounded) methods (Gopalan et al. 2015, Gopalan et al. 2014) to build joint species distribution models.
Further details of the developed models, the technical challenges and associated results will be discussed in a future blog post.
Improving data collection and feedbacks to citizen scientists
SpeedMind has a strong citizen science component, as it relies on the plant species reportings provided by InfoFlora, the national data and information centre of the Swiss flora (www.infoflora.ch/). InfoFlora relies on citizen science/crowdsourcing for achieving its goals. Plant occurrences across Switzerland are reported by thousands of citizen scientists in the field with the freely available InfoFlora mobile app (FloreApp). InfoFlora currently hosts millions of spatially explicit observations of ca. 3500 plant species across Switzerland. The recent development of user-friendly mobile app has strongly improved data transmission to biodiversity databases at an exponential rate (>50’000 records/month for InfoFlora).
However, most citizen scientists are not experts of the taxonomic groups they are reporting and generally only collect observations of species into easily accessible areas in the landscape (e.g. along roads and paths). Inability to correctly identify an observed species may lead to two detection problems: (i) non-detection (false negatives) if the citizen scientist does not report the species although present, and (ii) false positives if the citizen scientist reports the species with a wrong name. Identifying false positives in post hoc validation exercises is difficult and costly. For ensuring database integrity, it is thus of utmost importance to support app-users in accurately identifying species by providing feedbacks to citizen scientists on the plausibility of observing the species at a given location (species probability of presence based on SDMs), and to guide citizen scientists in the field to improve data collection (e.g. list of species “nice to check” in regions that are under sampled). In the era of big data, improving data management, collection and validation is crucial for enhancing data quality and for enabling sound scientific biodiversity assessments and analyses worldwide.
First developments and app implementation
Besides the development of new machine learning-based models of plant species distribution, we have integrated huge amounts of heterogeneous data sources (environmental data streams, maps, trait data, phylogenies, species occurrence data) in a standardised warehouse, representing an important contribution as it brings in one place disparate ecological, spatial and thematic information. In particular, SpeedMind developed new types of predictors sets at a very high spatial resolution (93 m), that are highly gained in precision and enable a better description of the species ecological niche.
First, we generated highly computational demanding maps of climate (temperature and precipitation) by downscaling CHELSA climate layers (Karger et al. 2017) from 1 km to 93 m spatial resolution in Switzerland. This pipeline will be further made available as an online tool to generate world level climate maps at 93 m resolution, which represents a major step in domain science for high resolution biodiversity studies.
Second, by combining a massive amount of plant data occurrences with expert-based ecological indicators of the plant ecology (classification of species ecological preferences for several climate and soil parameters), we used machine learning models (random forest) to generate eight ecologically meaningful predictors of plants (e.g. soil acidity, soil moisture, etc.). The resulting predictors outperformed traditional predictors used in domain science and increased our ability to predict the distribution of plant species in Switzerland, with an average improvement of 7.7% in model performance (delta TSS). In particular, species growing into moist habitats (e.g. Gentiana pneumonanthe) and growing along gradients of soil acidity (e.g. Oxytropis jacquinii, Ophrys holosericea) strongly benefited from this new set of predictors (Figure 2).
While getting more informative predictors of species distribution is a first step, the machine learning algorithms developed within SpeedMind will further enable to improve the modelling process and our understanding of the species ecological niche in a new and challenging way for domain science.
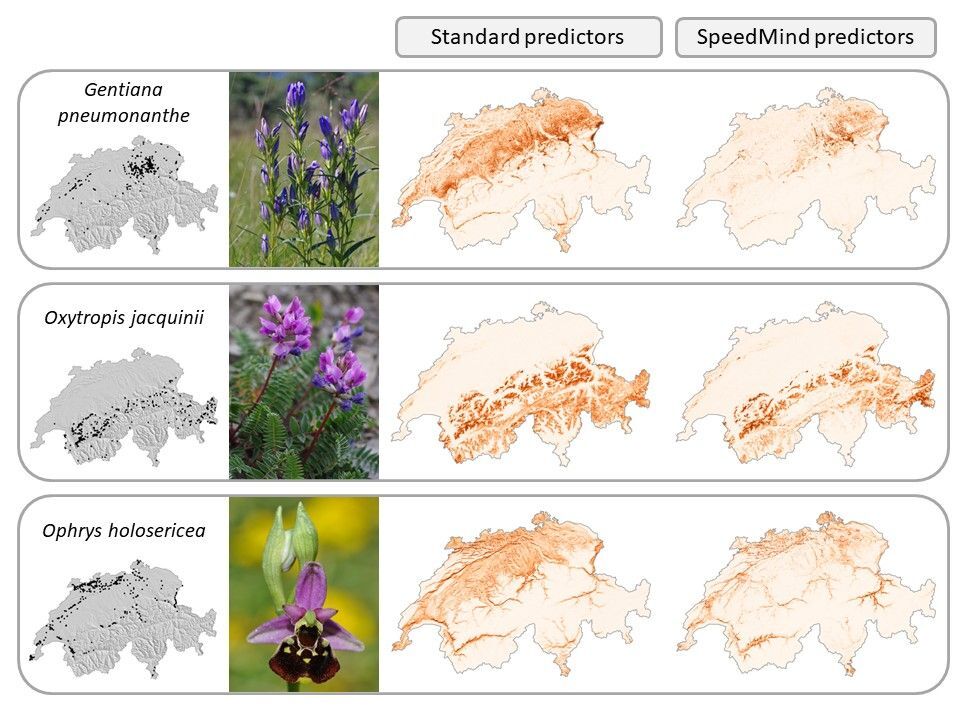
Besides scientific outcomes, SpeedMind aims at providing valuable feedbacks to citizen scientists in the field, which is a prerequisite for improving data collection, data quality and to foster the link between ecological sciences and citizen science. Extensive discussions between WSL and InfoFlora teams resulted in the definition of several sets of potential feedback that could be generated using SpeedMind SDMs and returned via FlorApp to citizen scientists in the field.
Among those, FlorApp will soon integrate direct offline feedbacks (currently implemented in a beta-testing mode; Figure 3) indicating if newly transmitted observations are (i) new for a specific region (data novelty), (ii) not been observed since a long period of time (historical data confirmation), and (iii) plausible according to the geographic position and the species ecological niche (data validation; Figure 3). This kind of information might strongly influence citizen scientists and motivate them to send more observations to biodiversity databases.
Future developments of FlorApp will aim at guiding citizen scientists into areas that are under sampled (data gap filling) and to provide a species list that is “nice to check” for a given location (new data acquisition). The main idea is that once a citizen submits an observation (these observation are the core data used in SpeedMind), s/he receives a feedback and a request to check up to 5 additional species. The five species (at the location from which the observation was sent) were selected to optimally improve the InfoFlora database and the models developed in SpeedMind (edges or gaps of knowledge in the species ecological niche).
Furthermore, this approach might also strengthen the collection of information on species absences (i.e. no observation of the species at this location), an information that is rarely collected and stored in biodiversity databases so far. While biodiversity databases are known to be highly biased spatially and taxonomically, SpeedMind will provide a technical and highly valuable solution for improving database integrity worldwide.
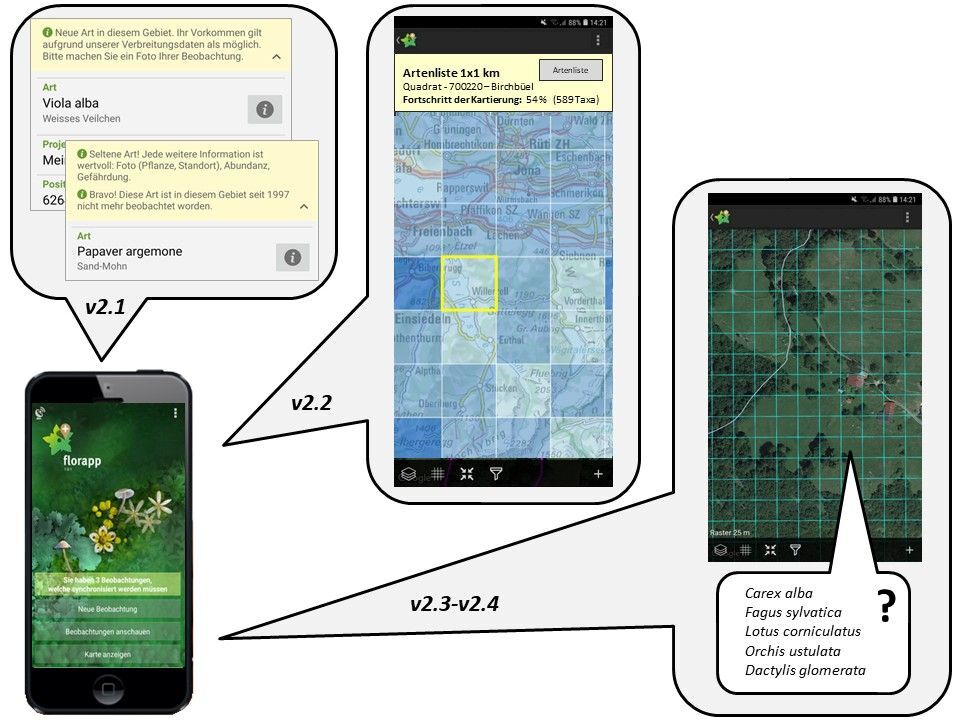
Co-authors
- Patrice Descombes, Postdoc, WSL
- Dirk N. Karger, Scientific staff member, WSL
- Damaris Zurell,Research Scientist, WSL
- Niklaus Zimmermann, Senior Scientist, WSL
References
- Gopalan, P., Hofman, J.M., Blei, D. (2015). Scalable Recommendation with Poisson Factorization. Proceedings of the Thirty-First Conference on Uncertainty in Artificial Intelligence.
- Gopalan, P., Ruiz, F.J.R., Ranganath, R., Blei, D. (2014). Bayesian Nonparametric Poisson Factorization for Recommendation Systems. Proceedings of the Seventeenth International Conference on Artificial Intelligence and Statistics.
- Guisan, A., Zimmermann, N. E (2000). Predictive habitat distribution models in ecology. Ecol. Model. 135, 147-186
- Karger, D.N., Conrad, O., Böhner, J., Kawohl, T., Kreft, H., Soria-Auza, R.W., Zimmermann, N.E., Linder, H.P. and Kessler, M. (2017). Climatologies at high resolution for the earth’s land surface areas. Scientific Data, 4, 170122.
- Meyer, C., Weigelt, P., Kreft, H. (2016). Multidimensional biases, gaps and uncertainties in global plant occurrence information. Ecol. Lett. 19, 992-1006.