Summary
The food production industry is one of the largest CO2 emitters, accounting for 26% of all greenhouse gas emissions .
As we endeavor to reduce global CO2 emissions to avoid the worst impacts of climate change, a collaboration between the Swiss Data Science Center and the Swiss processing solution provider Bühler Group is laying the technical foundations to make real impacts on the industry’s emissions through an innovative approach to optimizing manufacturing operations.
Malting
Malting is the process of making a grain (typically barley) germinate by soaking in water and then halting the germination process by drying with hot air. It is subsequently processed into multiple food and beverage products such as beer, whiskey, or malted drinks. About 96% of the 16.5 million tons of malt made in the world is used as the main ingredient of beer . It is estimated that about 75-80 % of the world’s barley malt is produced using Bühler malting equipment .
In 2018, Heineken, one of the world’s largest beer producers, estimated that 7% of its CO2 emissions came from the malting process . In the UK, the malting sector produced around 1.5 million tons of malt, emitting about 340,000 tons of CO2. It is estimated that fuel usage represents 68% of malting CO2 emissions while electricity contributes 32% resulting in energy consumption (electricity + heat) ranging from around 600 to 1400 kWh/ton of malt.
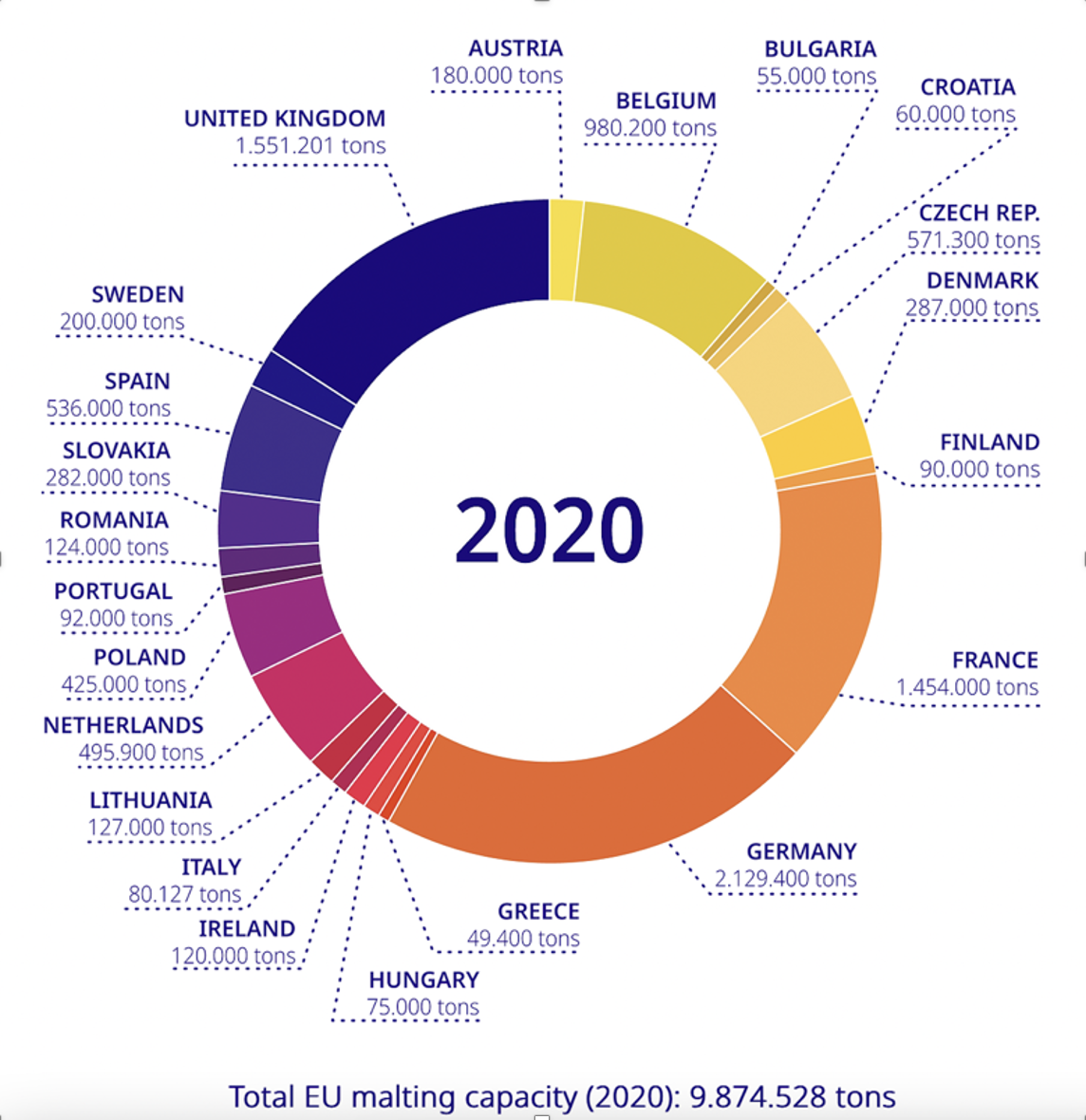
To address the emissions associated with the high energy demands of malting, MontBlanc was developed as a solution through a collaboration between Bühler AG and the Swiss Data Science Center. MontBlanc aims to decrease energy consumption and, therefore greenhouse gas (GHG) emissions in the malting process by optimizing a key component of the procedure.
The malting process
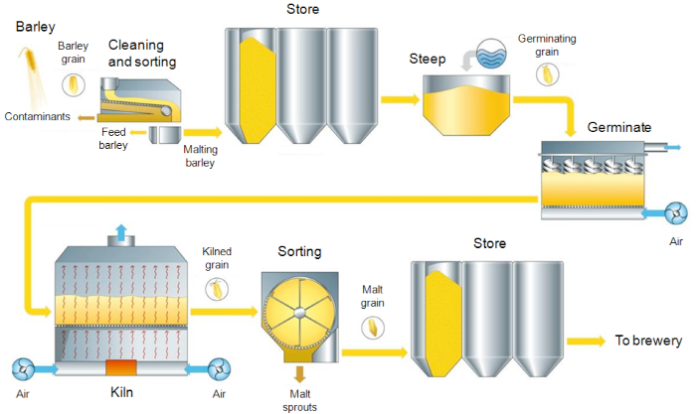
The malting process has three main steps:
- Steeping: The barley is soaked in water for several hours (~24-72h) to raise its moisture and allow grain germination.
- Germination: The soaked barley enters a germination chamber with a controlled temperature/humidity level allowing germination to progress. The process takes around 4-5 days.
- Kilning (drying): The germinated barley (also called green malt), which has up to ~45% moisture, is then dried to around ~5%. This step allows stabilization of the grain and long-term storage. In this step, the malt also takes on its color and flavor.
The kilning phase is the most energy-intensive of these steps, as the malt must be dried from 45% moisture to 5% within 24-48 hours. This process requires high airflow and high temperature.
Kilning
The kiln is a drying chamber designed to dry malt efficiently. It contains:
- A “product bed”: a perforated floor that allows air to pass through,
- A set of burners (using gas or other fuel) that heat up the incoming air (taken from outside),
- A system of electric fans that can move a large volume of air. This system is the primary driver of electricity costs in the malting plant.
The kilning step can be separated into three main phases:
- Withering: Lasts around 12 hours and most of the surface moisture of the germinated grain is removed. Humidity decreases from ~45% to ~15%.
- Curing: In this second phase which lasts several hours, the air temperature is increased to 80-90°C removing the last of the moisture and adding color to the malt.
- Cooling: In this final step, the malt is cooled using large volumes of air at ambient temperatures.
The MontBlanc solution
Multiple strategies are employed to save energy during the kilning process. Heat exchangers (mostly glass tubes) recover latent heat from the output air to preheat the ambient air entering the kiln, while the previously heated air is recirculated when its relative humidity is low. Seasonal process parameters and air-bypass in case of high ambient temperature may also be used. MontBlanc looks beyond the physical technologies deployed to save energy by exploiting the parameters utilized during the malting process.
In a typical malting plant, the kilning process follows a strict, pre-defined schedule with pre-defined process parameters. However, depending on batch characteristics (such as initial moisture level) and weather conditions (temperature, humidity, etc.), the process parameters do not always fit the pre-defined schedule, typically leading to an overestimation of the parameters to stay within schedule. This can result in the kiln staying idle while waiting for the next batch to be ready.
The main concept behind MontBlanc is to leverage a large set of variables (weather, plant environment, grain characteristics) to forecast the evolution of the kilning process for each batch of product. This allows the best parameters to be calculated to make the process as efficient as possible while fitting within the pre-defined schedule.
Physics tells us that reducing fan speed and increasing the drying time is more efficient and will lower energy consumption. A decrease of 10% in fan speed leads to a 10% increase in drying time. However, it also decreases the electrical energy consumption of the fan by 19%, as confirmed in the data below.
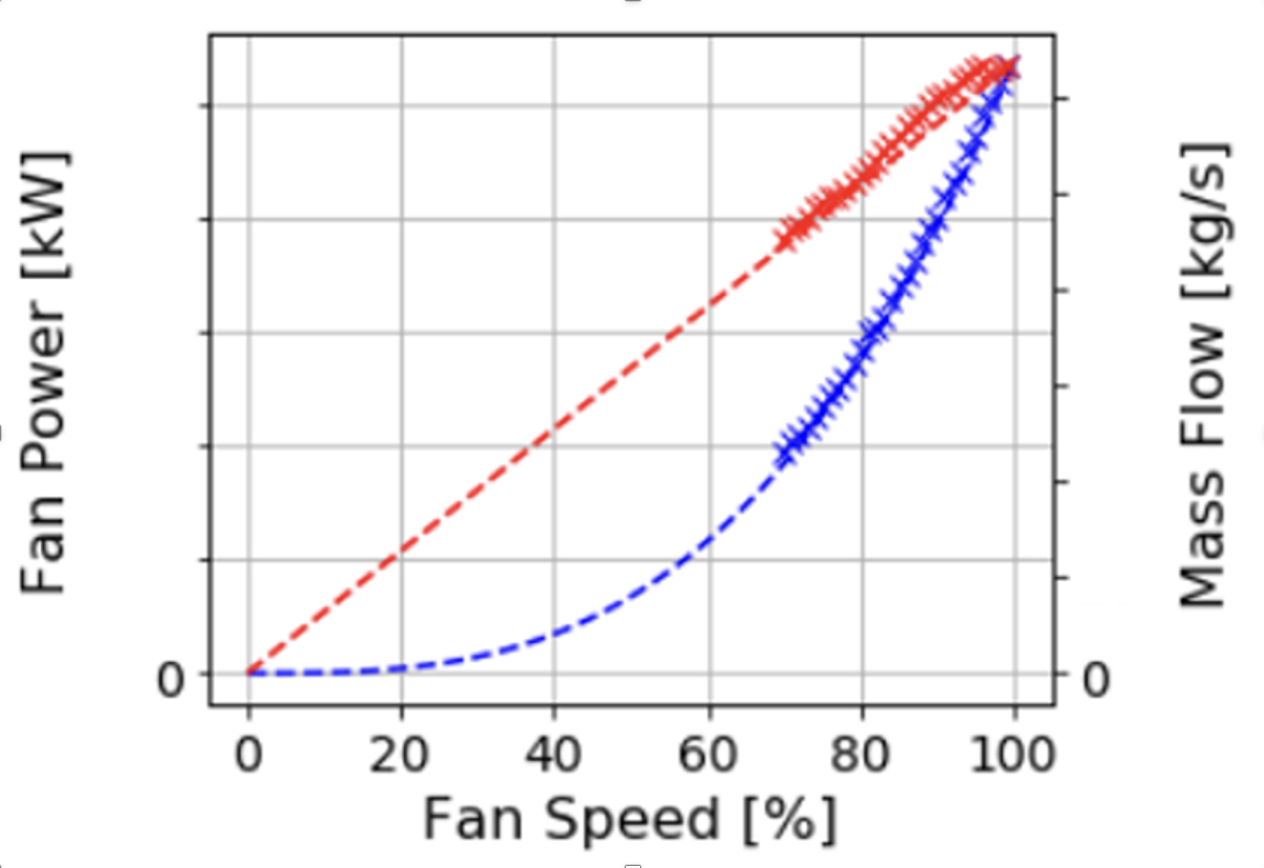
MontBlanc’s aim is to estimate the waiting time expected at the end of the next batch. It then computes the optimal fan speed necessary to reduce this expected waiting time and optimize the electrical power consumed.
Benefits
- Average of 16.4% reduction in electrical power consumption when using the MontBlanc service, validated over an 11-month industrial trial with 207 batches at an industrial pilot customer.
- A reduced waiting time, leading to efficiency gains for the operator and plant managers.
Future work
The food production domain is vast and complex, with thousands of processes and machines in use. MontBlanc only touches the surface of what may be possible within the manufacturing sector. SDSC and Bühler are working together to explore the potential of the MontBlanc approach, which could lead to vast energy efficiency gains across the sector, helping to optimize the large installed base of manufacturing lines and lead to significant reductions in global CO2 emissions.
Acknowledgments
The author would like to thank his colleagues at Bühler GQ Unit, particularly Matthias Graeber, Head of Data Science at Bühler Group for their support in developing MontBlanc and for their valuable feedback when writing this blog post, and Roberto Castello, Principal Data Scientist at the SDSC.
References
- Our World in Data | Food production is responsible for one-quarter of the world’s greenhouse gas emissions
- UK Malt | Unravelling the Mystery
- The Heineken Company | Annual Report 2018 | Page 126
- Euromalt | EUROMALT statistics
- European Commission | Report on different approaches to minimize the energy need for drying and kilning malt including description of their impact on beer flavour
- CarbonTrust. Industrial Energy Efficiency Accelerator - Guide to the maltings sector. Malting Sector Guide, CTG053.2011
- Bühler Group | Digital fan speed optimization for maltsters
MontBlanc in the news
- Food Ingredients 1st | Drinktec 2022: Bühler’s MontBlanc digital system slashes emissions in kilns
- Lang Creek Brewery | Bühler's digital Montblanc system reduces emissions in the brewing process
- Food Business Africa | Bühler develops new tech to cut emissions and improve efficiencies for beer producers