
Data science for enhancing resource efficiency in manufacturing processes


Clément became part of the SDSC team in January 2019, assuming the role of a Data Scientist with an emphasis on industry-oriented projects. He holds a Bachelor of Science degree in Physics, acquired in 2016 from the École Polytechnique Fédérale de Lausanne (EPFL) in Switzerland. Following this, he earned a Master of Science in Computational Science and Engineering in 2018, also from EPFL. Throughout his academic journey, Clément concentrated on leveraging Data Science and Machine Learning techniques to enhance efficiency in industrial processes. Subsequently, he developed a specialized interest in Generative AI, with a particular focus on Natural Language Processing (NLP), especially in the application of Large Language Models for innovation.

Context
About 96% of the 16.5 million tons of malt made in the world is used as the main ingredient for beer production. Bühler estimates that about 75-80 % of the world’s barley malt is produced using its malting equipment. The MontBlanc solution was developed to address the carbon emissions associated with the high energy demand of malting processes.
Objectives
MontBlanc aims at reducing the energy consumption, and therefore greenhouse gas (GHG) emissions, of the malting process by optimizing a key component of the procedure. In a typical plant, the kilning process (i.e., the drying of the malt) follows a strict, pre-defined schedule with defined process parameters. However, depending on batch characteristics (such as initial moisture level) and weather conditions, the process parameters do not always fit the pre-defined schedule. MontBlanc leverages a large set of variables (weather, plant environment, grain characteristics) to forecast the evolution of the kilning process for each batch of product. It estimates the waiting time expected at the end of the next batch and then it computes the optimal fan speed necessary to reduce this expected waiting time and thus optimizing the electrical power consumed.
Benefits
After deploying the MontBlanc service in an industrial trial at a pilot customer for a period of 11 months, about 16% reduction in electrical power consumption with respect to standard operations has been observed. The food production domain is vast and complex, with thousands of processes and machines in use. MontBlanc shows a tangible example of data science potential in the manufacturing sector.
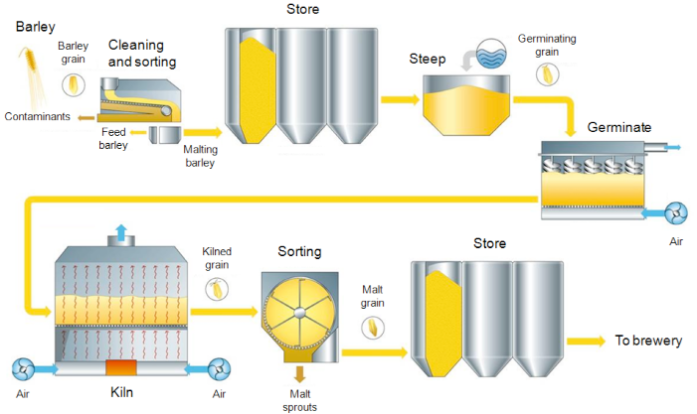
Notes
Special thanks to the Bühler GQ Unit, and particularly to Matthias Graeber, Head of Data Science at Bühler Group, for their support and exchanges during the MontBlanc project.
References
- Swiss Data Science Center | Blog about Montblanc
- Bühler Group | Digital fan speed optimization for maltsters
- Food Ingredients 1st | Drinktec 2022: Bühler’s MontBlanc digital system slashes emissions in kilns
More case studies
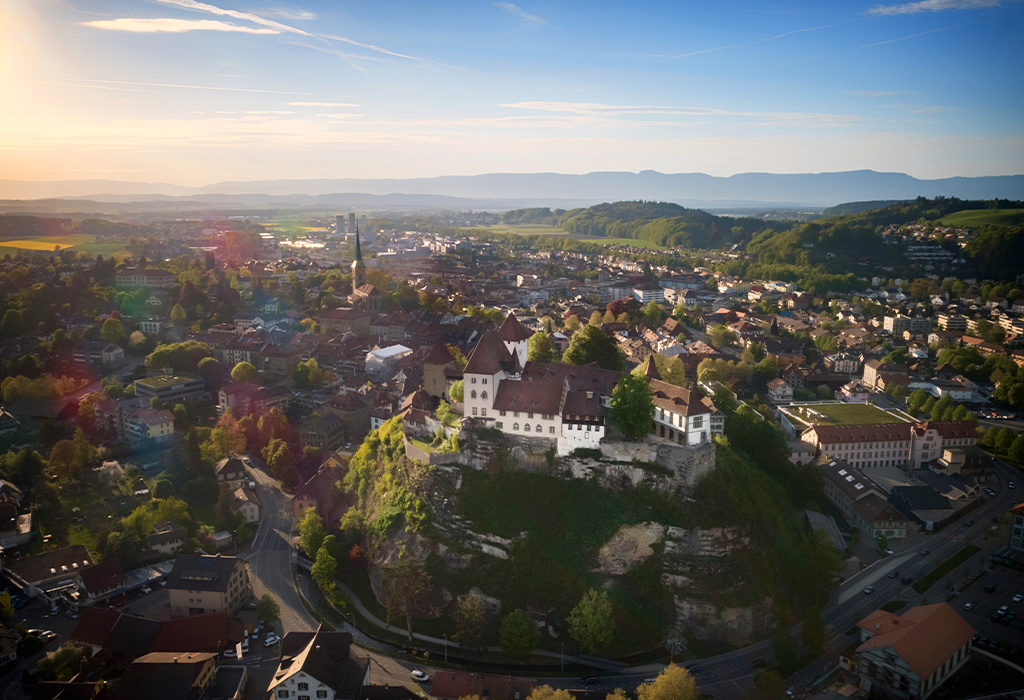
Smart Waste Collection with AI-Empowered Planning
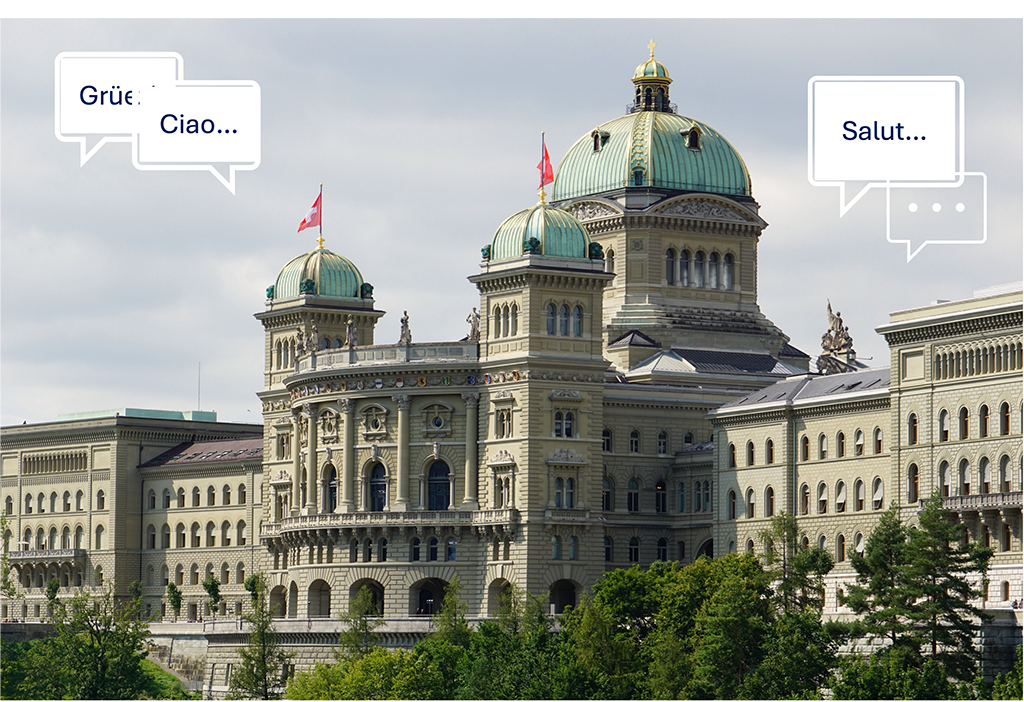
Enhancing Parliamentary Services with Generative AI

An artificial intelligence-based system for augmented cell & gene therapies
Contact us
Let’s talk Data Science
Do you need our services or expertise?
Contact us for your next Data Science project!